Spectral computed tomography parameters could be surrogate imaging markers to detect early perfusion changes in diabetic kidneys
Introduction
Diabetic kidney disease (DKD), which occurs in about 30–40% of patients with type 2 diabetes mellitus (DM), is currently the main cause of kidney failure on a global scale (1). In the last few decades, the prevalence and mortality related to DKD have significantly increased, which has become a global health concern. This is largely due to the asymptomatic nature of the disease in its early stages and its rapid progression during its later stages (2). Due to rapid aging and urbanization in recent decades, there has been an increasing incidence of DM. Consequently, DKD has developed into a significant public health problem in China (3).
Primary prevention is feasible if there are sensitive and specific biomarkers that enable early identification of DKD. Biomarkers such as estimated glomerular filtration rate (eGFR) and measured GFR (mGFR) have been established for the estimation of overall kidney damage (4,5). However, since the kidneys can partially compensate for lost function, kidney damage often precedes a positive result on biomarker tests in the early stage of DKD (6). Thus, these routinely used biomarkers neither offer insights into the degree of anatomical damage nor allow for risk stratification (7). Developing a new non-invasive modality to better understand the underlying pathophysiology in the early stage of DKD is of great importance and urgently required.
In the past few decades, magnetic resonance imaging (MRI) technique, as a non-invasive protocol, has been experimentally used to assess the pathophysiological status of different stage DKD, providing more comprehensive information such as microstructure damage, renal oxygenation, and hemodynamic measurements of renal perfusion (8-12). However, the strict indications required for magnetic resonance (MR) examination and time-consuming scanning limit the wider application of MRI techniques in DKD patients; moreover, different vendors and scanners may affect the stability and reproducibility of the diagnostic efficacy of MRI parameters (13).
Kidney microvasculopathy and renal hemodynamics changes have been demonstrated to be the baseline pathophysiological features of DKD, which precedes the appearance of structural and biochemistry disturbances (8,11,14). Dual-layer spectral detector computed tomography (DLCT) has been validated as a surrogate protocol for assessing organs’ perfusion disorder (15,16). We speculated that spectral CT parameters could identify the presence of microcirculatory disorder of DKD patients and contribute to the early diagnosis of DKD. In the clinical setting, perfusion assessment using spectral CT parameters is a novel and promising approach that can facilitate an early intervention when renal damage may be reversible and can slow down disease progression, especially for those people who are not suitable for an MRI examination. Therefore, the objective of this study was to validate the feasibility of spectral CT parameters to identify early changes of renal perfusion in patients with DM. We present this article in accordance with the STARD reporting checklist (available at https://qims.amegroups.com/article/view/10.21037/qims-22-1400/rc).
Methods
Participants
This retrospective study was conducted in our hospital between 1 August 2020 and 31 June 2022. Patients clinically diagnosed with type 2 DM were selected from the hospital patient pool. All patients underwent abdominal enhanced DLCT scanning for differential diagnosis of benign abdominal organ diseases in the Radiology Department of Nanxishan Hospital of Guangxi Zhuang Autonomous Region. The inclusion criteria were as follows: (I) aged 18–80 years; (II) with type 2 DM, per the 2007 American Diabetes Association diagnostic criteria (17); (III) abdominal enhanced DLCT scanning including whole kidney; (IV) complete clinical data related to type 2 DM; (V) normal albuminuria, eGFR of ≥80–120 mL/(min 1.73 m2). The exclusion criteria were as follows: (I) primary hypertension before or at the time of diagnosis with DM; (II) presence of other kidney diseases such as cysts, hamartomas, and calcification that influence the delineation of a region of interest (ROI); (III) severe systemic or organic diseases other than DKD diagnosed as the primary cause of impaired kidney function; (IV) poor image quality seriously affecting the measurements (Figure 1).
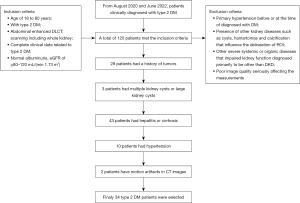
A total of 19 DM-free individuals who underwent abdominal (including the whole kidney) enhanced DLCT scanning in the radiology department of our hospital were selected as the control group. These cases had no renal or renal vascular diseases or hypertension and other severe systemic or organic diseases which may impair kidney function. All controls had normal albuminuria and normal serum creatinine levels, and they showed no morphological abnormalities in both kidneys on DLCT images.
Demographic data (including gender, age), medical history, physical examination [including blood pressure, weight, height, and body mass index (BMI)] and clinical laboratory measurements of blood samples [including creatinine, triglycerides, cholesterol, high density lipoprotein (HDL), low density lipoprotein (LDL), hemoglobin A1c (HbA1C)], and duration of DM were collected.
The study was conducted in accordance with the Declaration of Helsinki (as revised in 2013). The study was approved by the Ethics Committee of the Nanxishan Hospital of Guangxi Zhuang Autonomous Region (No. 2022KY-E-057) and the informed consent form was waived because it imposed no more than a minimal risk to the patients and involved no procedures for which written consent is normally required outside of the research context.
CT examination
The DLCT scans, which comprised both conventional non-enhanced CT and contrast-enhanced CT (cortical and medullary phases), were conducted using IQon spectral CT scanner (Philips Healthcare, Amsterdam, Netherlands).
The contrast agent, iopromide (Bayer Healthcare Company Ltd., Leverkusen, Germany), was administered intravenously via the antecubital vein at a dose of 1.2 mL/kg body weight (80–100 mL) using a high-pressure syringe with an intended flow rate of 3.0 mL/s. The ROI was placed in the abdominal aorta with a trigger threshold of 180 Hounsfield units (HU). Monitoring began after 10 seconds of contrast injection, followed by a 6-second delay for cortical phase scanning after reaching the threshold and medullary phase scanning after 90 seconds of contrast injection. Parameters included tube voltages of 120 kVp, spectral CT adaptive current, collimator width of 64×0.625 mm, pitch of 1.234, 0.27 seconds rotation time, and matrix of 512×512. Following completion of the scans, data obtained from the enhanced dual phases were reconstructed using projected spatial spectral reconstruction (iDose 4). The image reconstruction had a thickness and spacing of 1 mm.
Imaging analysis
We obtained retrospective spectral-based image data from a Philips Spectral Diagnostic Suite 9.0 workstation (Philips Healthcare). The data were processed and analyzed by 2 radiologists with over 5 years of experience in interpreting abdominal images. To minimize potential biases, the investigators were blinded to all patients’ clinical data. ROIs with an approximate size of area of 10±1 mm2 were positioned at the upper, middle, and lower poles of both medulla and cortex of the both kidneys on the conventional CT images (Figure 2). The ROIs on conventional CT images were copied and pasted onto all spectral parameter maps, respectively, to ensure that the ROIs in different image maps had the same size, location, and shape. Manual adjustment was performed on the cortical ROIs to reduce interference from blood vessels, the renal pelvis, and the edge of the kidney wherever possible. The measurements were performed 3 times at consecutive image sections containing the maximum coronal-section as well as the upper and lower layers of each kidney, and the mean values were used for statistical analysis. In order to minimize the influence of the individual differences and scanning times, the 2 radiologists with over 5 years of experience in interpreting abdominal images (ID) values of kidneys were normalized to those of the aorta in the abdominal aorta to calculate the normalized iodine density (NID): NID=ID/ID aorta. In addition, the slope of the spectral HU curve (λHU, in Hounsfield units per kiloelectron-volt) was calculated as follows: λ= (CT number[40 keV] – CT number[70 keV])/(70–40) keV.
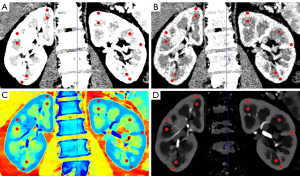
Statistical analysis
Intraclass correlation coefficient (ICC) tests were used to determine inter-operator reproducibility for measuring parameters among 2 readers. Interpreting the values of the ICC in this study followed established convention. An ICC value of less than 0.20 was considered poor agreement, 0.21–0.40 fair agreement, 0.41–0.60 for moderate agreement, 0.61–0.80 for good agreement, and ≥0.80 was considered excellent agreement (18). We conducted an a priori power analysis to test the adequacy of our sample size to independent sample t-test using G*Power (19). We specified an alpha level of 0.05, a 1-b error probability of 0.80, and an effect size (f =0.50) for an estimated medium effect. The results of the analysis suggested a total recommended sample size of 128. A post-hoc power analysis revealed that a sample size of 53 (DM:NC =34:19) resulted in a reported power of 0.531 to detect a medium effect (f =0.50) with an alpha level of 0.05.
We averaged all parameters measured by the 2 physicians. In this study, the missing value processing adopted the following method: if it is a numeric feature, the mean value is used to fill in; if it is a categorical feature, the mode value is used to fill in. We tested normality and homogeneity of variance using the Shapiro–Wilk and Bartlett’s tests, respectively. Data with normal distribution were described as mean ± standard deviation, whereas counting data were expressed as n (%). The differences in spectral CT parameters between groups were evaluated using a 2-sample t-test. For the group, the differences in spectral CT parameters between the left and right kidneys were assessed using the paired sample t-test. We evaluated the differences in spectral CT parameters between the upper, middle, and lower poles of both kidneys using a one-way analysis of variance (ANOVA). We used a chi-square test to evaluate the differences in gender frequency. Receiver operating characteristic (ROC) curves were utilized to assess the diagnostic performance of the imaging parameters for differentiating the DM group from the control group. We used the DeLong test to determine the statistical differences in the area under the ROC curves (AUC) among different parameters. Results were considered statistically significant if the 2-tailed P value was less than 0.05.
Results
Patient characteristics
Demographic and clinical variables reports are shown in Table 1. A total of 120 patients met the inclusion criteria. Of these, 28 patients had a history of tumors, 3 patients had multiple kidney cysts or large kidney cysts, 43 patients had hepatitis or cirrhosis, 10 had hypertension, and 2 patients have motion artifacts in their CT images; finally, 34 type 2 DM patients were selected in the present study (Figure 1). The average period from being diagnosed with DM to the current DLCT examination in the diabetic group was 7.3±5.6 years. This study had no missing values. No significant differences were found in none-enhanced CT numbers, enhanced CT numbers (Table 2), and gender distribution between DM group and control group participants.
Table 1
Parameter | Group | P value | |
---|---|---|---|
Healthy controls (n=19) | Diabetic patients (n=34) | ||
Gender (male) | 12 [63] | 20 [51] | 0.095 |
Age (y) | 48.1±16.9 | 57.7±10.7 | 0.014 |
Weight (kg) | 59.2±4.7 | 62.0±11.4 | 0.329 |
BMI (kg/m2) | 22.9±3.0 | 22.7±3.0 | 0.838 |
eGFR (mL/min/1.73 m2) | 99.1±15.7 | 89.6±22.3 | 0.110 |
HbA1C (%) | 4.1±0.7 | 9.4±2.9 | <0.001* |
SP (mmHg) | 125.9±13.8 | 129.6±22.6 | 0.530 |
DP (mmHg) | 76.9±12.7 | 80.1±11.9 | 0.367 |
TC (mmol/L) | 3.9±1.0 | 4.2±1.1 | 0.510 |
TG (mmol/L) | 1.1±0.5 | 1.8±1.2 | 0.082 |
HDL (mmol/L) | 1.2±0.5 | 1.1±0.4 | 0.318 |
LDL (mmol/L) | 2.4±0.6 | 2.7±1.1 | 0.478 |
Duration of diabetes (years) | 0 | 7.3±5.6 |
Data are presented as n [%] or mean ± SD. *, the comparison with statistical significance. HbA1c, glycosylated hemoglobin; BMI, body mass index; eGFR, estimated glomerular filtration rate; SP, systolic pressure; DP, diastolic pressure; TC, total cholesterol; TG, triglyceride; HDL, high density lipoprotein; LDL, low density lipoprotein; SD, standard deviation.
Table 2
Parameters | Cortex | Medulla | |||||||
---|---|---|---|---|---|---|---|---|---|
Diabetic group | Control group | t-value | P value | Diabetic group | Control group | t-value | P value | ||
None enhancement CT number (HU) | 41.52±4.07 | 39.74±1.82 | 1.202 | 0.246 | 19.08±3.34 | 18.50±1.94 | 0.457 | 0.653 | |
Cortical phase | |||||||||
Enhancement CT number (HU) | 150.05±18.2 | 145.85±14.09 | 0.566 | 0.578 | 59.65±3.38 | 58.02±3.39 | 1.079 | 0.295 | |
λ40–70 | 8.87±1.14 | 7.54±0.74 | 5.079 | <0.001* | 2.40±0.78 | 2.00±0.63 | 1.913 | 0.061 | |
Zeff | 9.35±0.21 | 9.12±0.15 | 4.506 | 0.01* | 7.98±0.20 | 7.88±0.18 | 1.897 | 0.063 | |
ID | 4.43±0.57 | 3.78±0.42 | 4.385 | <0.001* | 1.18±0.38 | 0.98±0.32 | 1.954 | 0.056 | |
NID | 0.40±0.06 | 0.33±0.05 | 4.667 | <0.001* | 0.11±0.04 | 0.09±0.03 | 2.390 | 0.021* | |
Medullary phase | |||||||||
Enhancement CT number (HU) | 173.73±16.78 | 167.59±29.58 | 0.292 | 0.774 | 87.96±3.81 | 84.96±4.97 | 1.617 | 0.147 | |
λ40–70 | 11.29±2.08 | 9.53±1.61 | 3.201 | 0.002* | 4.88±1.16 | 4.38±0.77 | 1.683 | 0.098 | |
Zeff | 9.77±0.34 | 9.45±0.28 | 3.318 | 0.002* | 8.56±0.26 | 8.46±0.16 | 1.357 | 0.181 | |
ID | 5.65±1.02 | 4.76±0.84 | 3.261 | 0.002* | 2.45±0.59 | 2.16±0.38 | 1.898 | 0.063 | |
NID | 1.46±0.33 | 1.24±0.13 | 2.768 | 0.008* | 0.63±0.19 | 0.67±0.13 | 1.261 | 0.213 |
Data are presented as mean ± SD. *, the comparison with statistical significance. DLCT, dual-layer spectral detector computed tomography; CT, computed tomography; λ, the slope of the energy spectrum curves; Zeff, effective atomic number; ID, iodine density; NID, normalized iodine density; SD, standard deviation; HU, Hounsfield units.
Reliability of measurements
The measurements taken by both observers were highly consistent (P>0.05). The ICC was found to be 0.82, 0.837, 0.80, and 0.806 for effective atomic number (Zeff), ID, NID, and λ40–70, respectively.
Comparison of the spectral CT parameters in DM group and control participants
The mean medullary and cortical spectral CT parameter values of bilateral kidneys in dual phases are shown in Table 2. The results showed that Zeff, ID, NID, and λ40–70 of renal cortex in the DM patient group were significantly higher than those in the control group (P<0.05), both in cortical phase and medullary phase (Figures 3,4). There were no significant differences of Zeff, ID, or λ40–70 in the kidney medulla between the DM patients group and the control group (P>0.05) in the dual phase. NID of kidney medulla in the DM patient group was significantly higher than that in the control group (P=0.021), yet only in the cortical phase.
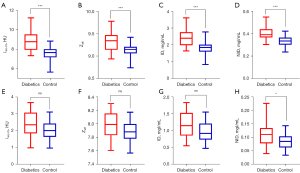
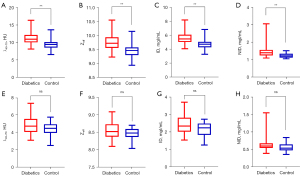
There was no statistical difference in the upper, middle, and lower poles of both kidneys, as well as in the groups in the same position (P>0.05) (Appendix 1, Figures S1-S6).
ROC analyses
The ROC analyses are presented in Table 3 and Figure 5. The diagnostic efficacy of cortical spectral CT parameters in discriminating the DM group from the control cases in the cortex phase were as follows: ID value: AUC =0.816, sensitivity =73.5%, specificity =89.5%, and Youden index =0.63 at the optimal cutoff value 4.14; Zeff value: AUC =0.800, sensitivity =67.6%, specificity =94.7%, and Youden index =0.623 at the optimal cutoff value 9.26; λ40–70 value: AUC =0.822, sensitivity =70.6%, specificity =89.5%, and Youden index =0.601 at the optimal cutoff value 8.26; NID value: AUC =0.851, sensitivity =70.6%, specificity =89.5%, and Youden index =0.601 at the optimal cutoff value 0.37.
Table 3
Enhancement phase | Cutoff value | AUC | Sensitivity (%) | Specificity (%) | 95% CI | P value |
---|---|---|---|---|---|---|
Cortical phase | ||||||
ID | 4.14 | 0.816 | 73.5 | 89.5 | 0.679–0.921 | <0.001* |
Zeff | 9.26 | 0.800 | 67.6 | 94.7 | 0.668–0.901 | <0.001* |
λ40–70 | 8.26 | 0.822 | 70.6 | 89.5 | 0.675–0.918 | <0.001* |
NID | 0.37 | 0.851 | 70.6 | 89.5 | 0.684–0.926 | <0.001* |
Medullary phase | ||||||
Zeff | 9.58 | 0.763 | 73.5 | 78.9 | 0.614–0.837 | <0.001* |
ID | 5.08 | 0.769 | 70.6 | 78.9 | 0.617–0.846 | <0.001* |
λ40–70 | 10.07 | 0.766 | 76.5 | 73.7 | 0.617–0.839 | <0.001* |
NID | 1.37 | 0.790 | 67.6 | 84.2 | 0.623–0.855 | <0.001* |
*, the comparison with statistical significance. Zeff, effective atomic number; λ, the slope of the energy spectrum curves; ID, iodine density; NID, normalized iodine density; CI, confidence interval; ROC, receiver operating characteristic; AUC, area under the curve.
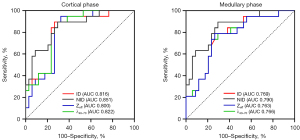
The diagnostic efficacy of cortical spectral CT parameters in discriminating the DM group from the control group in the medullary phase were as follows: ID value: AUC =0.769, sensitivity =70.6%, specificity =78.9%, and Youden index =0.495 at the optimal cutoff value 5.08; Zeff value: AUC =0.763, sensitivity =73.5%, specificity =78.9%, and Youden index =0.524 at the optimal cutoff value 9.58; λ40–70 value: AUC =0.766, sensitivity =76.5%, specificity =73.7%, and Youden index =0.502 at the optimal cutoff value 10.07; NID value: AUC =0.79, sensitivity =67.6%, specificity =84.2%, and Youden index =0.518 at the optimal cutoff value 1.37.
There were no significant differences of AUCs in Delong test both in cortex phase and medullary phase, P>0.05.
Discussion
In recent years, MRI techniques have demonstrated that microcirculation and microstructure changes have already occurred at the early stage of diabetes. The current study was the first to use spectral CT quantitative parameters to assess early changes in diabetic kidneys. The study results validated that DM patients with normal albuminuria and eGFR had experienced abnormal kidney cortex perfusion. This study confirmed that spectral CT could be an alternative protocol to identify early kidney injury in patients with DM.
The present study showed that the cortical spectral parameters of diabetic kidneys were higher than those of the control cases in dual enhanced phase, indicating an abnormal hyperperfusion in patients with DM prior to abnormal albuminuria and eGFR detection. Previous studies have shown that patients with DM have normal or mildly increased renal plasma flow and renal hyperperfusion even before proteinuria is detected (11,20,21). Pourghasem et al. proposed that hyperperfusion in diabetic kidneys may primarily cause structural and functional disorders in the kidney. Correction of perfusion abnormalities during the early stages of DM may prevent subsequent renal complications (22). During the initial phases of DKD, there is an elevation in blood volume and fluid accumulation in the kidneys (23). This phenomenon may be attributed to a number of contributing factors, such as elevated blood glucose levels, an increase in renal tubular flow and ultra-filtration volume initiated by the renin-angiotensin-aldosterone system, an expansion in blood vessels, and the comparatively wider caliber of the renal tubules (24). Another potential factor is microalbuminuria, which could be responsible for microvascular impairment and reduction in the clearance capacity of renal blood, consequently leading to improved renal perfusion (25). Renal hemodynamic disorders play a pivotal role in the development of diabetic kidney damage. There is a widely accepted notion that hyperperfusion, renal hyperfiltration, and an augmentation in capillary permeability in diabetic nephropathy occur prior to the onset of overt proteinuria (26). Renal microvascular hyperperfusion may contribute to the emergence of overt proteinuria in patients with DM until there is a reduction in renal filtration (25). A prior study (26) suggested that renal hyperperfusion can be observed only before the onset of overt proteinuria; however, it is uncertain how long such a state of increased renal perfusion can persist.
Contrary to the above study findings, other studies have reported finding cortical kidney hypoperfusion in the diabetic patients with normal GFR compared with healthy controls. Mora-Gutiérrez et al. found that patients with normal eGFR exhibited a decrease in kidney perfusion as compared to nondiabetic individuals. These distinctions were not related to age, gender, or BMI (14). Rossi et al. also reported that cortical renal blood flow in mild chronic kidney disease (CKD) patients was significantly lower in comparison to that observed in healthy volunteers (27). The decline in kidney perfusion observed in diabetic patients may be attributed to an elevation in oxidative stress resulting from chronic hyperglycemia, and/or a reduction in nitric oxide concentration within the renal cortex (14). The imbalance between oxygen supply and consumption, which eventually leads to renal hypoxia, is primarily caused by a reduction in renal blood flow (28). In situations where there is kidney ischemia, cytokines such as interleukin-34 and colony-stimulating factor-1 promote macrophage proliferation, which can lead to renal fibrosis, and the transition from an acute to a chronic injury state (29).
The inconsistencies reported in the abovementioned studies may reflect that DKD is a dynamically progressive disease, and that patient sample selection at different disease stages exhibits different microcirculation states, presenting with hyperperfusion or hypoperfusion. Li et al. reported that, in the early stages of diabetes, glomerular hypertrophy and hyperfiltration induced by neoangiogenesis of microvessels were observed; however, in advanced diabetic nephropathy, the opposite was noted, which was characterized by capillary rarefaction accompanied by local tissue hypoxia (30). These alterations might be attributed to various pathological features. At the early stages of DKD, the GFR increases, and renal perfusion is mostly normal or elevated. As the disease progresses, there is an increase in renal arteriosclerosis, glomerular microarteriosclerosis, thickening of the glomerular capillary basement membrane, and narrowing or occlusion of the capillary lumen, resulting in a significant decline in renal perfusion, and eventually glomerulosclerosis. According to the pathological process of DKD, it is suggested that DKD results from the interaction between renal perfusion and cell damage. Both pathological states have different effects in distinct stages of DKD (31).
In this study, differences in spectral CT parameters mainly occurred in the renal cortex, and except NID (P=0.021), there were no significant differences of DLCT variables in the renal medulla, indicating that the perfusion abnormality of renal cortex is involved earlier than in the medulla. Previous studies have demonstrated that the levels of medullary blood oxygen in CKD patients were comparable to those in the control group, whereas the cortical blood oxygen levels remained unchanged or exhibited an increase (32). However, Yin et al. reported that medullary blood oxygen levels in DKD patients were lower than in the control group participants, which suggests that there might be inadequate medullary blood flow (33). Wang et al. reported that renal blood flow values of all renal regions of DM rats at day 3 and 7 after DM induction showed no statistically significant difference compared to baseline, but oxygen extraction fraction values increased compared with baseline, indicating enhanced oxygen consumption (12). Feng et al. reported that medullary perfusion in the kidneys of the DM normal albuminuria group was significantly increased compared to that in control groups; however, the medullary perfusion decreased from the normal albuminuria group to the mild albuminuria group and non-significant perfusion changes were observed between the mild albuminuria group and control groups (11). This finding suggests that there is a reduction in medullary high perfusion, and it is nearly normalized when moderate albuminuria is present.
The following causes may explain these inconsistent changes of cortical and medullary kidney perfusion. Firstly, the observed differences in cortical and medullary vascular responses may suggest the different expression of the relevant receptors family in renal cortex and medulla (34). Secondly, 90% of the renal blood volume is distributed to the cortex (35), endowing it with an abundant reserve capacity for handling damage, whereas the renal medulla lacks this ability. Furthermore, the medulla is vulnerable to injuries stemming from ischemic hypoxia, as well as the accumulation of toxic substances (36). Finally, increased Na+/K+-ATPase activity and active re-absorption of excess sodium in tubules leads to pronouncedly increased oxygen consumption, especially in the renal medulla (37). It is presumed that medullary hypoxia during the early stages of DKD is a critical mechanism in the onset and progression of the disease (38).
Another result was that there were no significant differences of spectral CT variables between the same ROI of the left and right kidney and the upper, middle, and lower poles of both kidneys (P>0.05). The results of this study indicate that DKD is a diffuse kidney disease in which microcirculation and microstructural changes occur uniformly throughout the whole kidney (39). Deng et al. reported a significant difference in limited movement of renal water molecules between the right and left kidneys in both the diabetic and healthy participant groups (23). This difference in results may be related to sample size (in their study, there were 19 patients in the diabetes group, 12 patients in control group). However, further validation of these findings is required based on larger samples or multi-center experimental studies.
In the present study, Delong test showed that there were no significant differences between the diagnostic efficacy of cortical Zeff, λ40–70, ID, and NID both in cortex phase and medullary phase. This result indicated that all the parameters of spectral CT can effectively identify cortex perfusion abnormalities of diabetic patients in the early stage. Zeff is the attenuation of X-ray that can be used to calculate the atomic number of unknown elements. KeV map is a virtual single-energy image, equivalent to a single energy X-ray scan to obtain an attenuation image of matter. The ID represents the iodine concentration of each voxel that can be measured. ID images have the potential to quantify iodine enhancement and improve the visualization of contrast-enhanced iodine within tissues. As spectral parameters are derived by the spectral system from the same set of images and the same decomposition model, they provide the same information, explaining why they do not show significant differences between them. In this study, the NID of cortical phase showed higher AUC (0.851; sensitivity =70.6%, specificity =89.5%) than other spectral parameters. In previous research, different MR parameters showed different diagnostic efficacy in identifying early changes in the diabetic kidney. Deng et al. reported that diffusion coefficient value was the optimal parameter (AUC =0.752, sensitivity =61.1%, specificity =88.9%) for detecting diabetic kidney changes using intravoxel incoherent motion diffusion-weighted imaging (23). Pruijm et al. found that the ROC for the blood oxygenation level-dependent MRI variables (AUC of R2* slope: 0.80, 95% CI: 0.65–0.96) showed a strong association with the occurrence of major renal events in patients with CKD (40). Li et al. evaluated renal blood flow in CKD using arterial spin labeling perfusion, which reported an AUC for cortical blood flow of 0.98 (sensitivity =84.85%, specificity =100%) (41). A recent study reported that the AUC of mean apparent diffusion coefficient (ADC) values for detecting mild impairment of DKD was 0.93 (sensitivity =100%, specificity =80%) (42). However, in another study, the AUC for identifying diabetic kidneys from healthy controls using the mean ADC values was only 0.674 (95% CI: 0.560–0.775; sensitivity =52.5%, specificity =80%) (43). Although MRI parameters have been used to evaluate DKDs for more than 2 decades, the stability and reproducibility of the diagnostic efficacy of the above parameters for DKD evaluation has remained a problem that needs to be addressed. The current study verified that the spectral CT is a promising protocol for assessing the diabetic kidney damage. Even though the diagnostic performance of the spectral CT parameters in this study seems acceptable when compared to previous MRI studies, such a cross-sectional comparison may not have clinical significance. Further validation using the same dataset is needed to compare the efficacy of spectral CT and MRI in diagnosing DKD. In the clinical setting, spectral CT quantitative parameters are rarely used to assess renal damage in patients with DM, and the following issues need to be further addressed. First, further comparison of the diagnostic efficacy of spectral CT and MRI parameters inter- and intra-groups of diabetic patients in single-center should be conducted for verification. Second, the stability and reproducibility of the diagnostic efficacy of spectral CT parameters for assessing diabetic kidney damage should be verified internally and externally due to a lack of similar studies. In a study which analyzed the proportion of normal perfused lung areas, the AUC of optimal spectral CT parameter was only 0.67 (sensitivity 74%, specificity 55%) (15). Finally, a stable and reproducible cut-off value is a key point of spectral CT parameters used for clinical assessment of diabetic kidney impairment, but this requires further validation by multicenter, large-sample studies.
This study had several potential limitations. Firstly, this was a single center, single scanner, and single vendor retrospective study, which may have contributed to selection bias. Secondly, only diabetic patients with normal albuminuria and eGFR ≥80–120 mL were included in this study, sub-classifications of diabetic kidney were not evaluated. Thirdly, the sample size of this study is insufficient, a post-hoc power analysis revealed that a sample size of 53 (DM:NC =34:19) resulted in a reported power of 0.531 to detect a medium effect (f =0.50) with an alpha level of 0.05. Fourthly, the association between GFR and spectral CT parameters was not validated. Finally, 7 cases older than 70 years were included in the DM group, which potentially affected renal blood flow changes due to renal aging.
Conclusions
In conclusion, spectral CT imaging showed that iodine reaches the cortical kidney faster in patients with DM than in healthy controls, indicating that aberrant cortical kidney perfusion exists in patients with DM, regardless of their proteinuria and eGFR status. This study confirms the potential of spectral CT as a viable method to detect early kidney injury in patients with DM. Therefore, this study demonstrates the potential of spectral CT in detecting early kidney injury in diabetic patients.
Acknowledgments
We thank the Department of Radiology, Nanxishan Hospital of Guangxi Zhuang Autonomous Region, for their help and discussion.
Funding: None.
Footnote
Reporting Checklist: The authors have completed the STARD reporting checklist. Available at https://qims.amegroups.com/article/view/10.21037/qims-22-1400/rc
Conflicts of Interest: All authors have completed the ICMJE uniform disclosure form (available at https://qims.amegroups.com/article/view/10.21037/qims-22-1400/coif). The authors have no conflicts of interest to declare.
Ethical Statement:
Open Access Statement: This is an Open Access article distributed in accordance with the Creative Commons Attribution-NonCommercial-NoDerivs 4.0 International License (CC BY-NC-ND 4.0), which permits the non-commercial replication and distribution of the article with the strict proviso that no changes or edits are made and the original work is properly cited (including links to both the formal publication through the relevant DOI and the license). See: https://creativecommons.org/licenses/by-nc-nd/4.0/.
References
- Alicic RZ, Rooney MT, Tuttle KR. Diabetic Kidney Disease: Challenges, Progress, and Possibilities. Clin J Am Soc Nephrol 2017;12:2032-45. [Crossref] [PubMed]
- Duan JY, Duan GC, Wang CJ, et al. Prevalence and risk factors of chronic kidney disease and diabetic kidney disease in a central Chinese urban population: a cross-sectional survey. BMC Nephrol 2020;21:115. [Crossref] [PubMed]
- Feng YZ, Ye YJ, Cheng ZY, et al. Non-invasive assessment of early stage diabetic nephropathy by DTI and BOLD MRI. Br J Radiol 2020;93:20190562. [Crossref] [PubMed]
- Luis-Lima S, Porrini E. An Overview of Errors and Flaws of Estimated GFR versus True GFR in Patients with Diabetes Mellitus. Nephron 2017;136:287-91. [Crossref] [PubMed]
- Stevens LA, Levey AS. Measured GFR as a confirmatory test for estimated GFR. J Am Soc Nephrol 2009;20:2305-13. [Crossref] [PubMed]
- Jufar AH, Lankadeva YR, May CN, et al. Renal functional reserve: from physiological phenomenon to clinical biomarker and beyond. Am J Physiol Regul Integr Comp Physiol 2020;319:R690-702. [Crossref] [PubMed]
- Inker LA, Levey AS, Pandya K, et al. Early change in proteinuria as a surrogate end point for kidney disease progression: an individual patient meta-analysis. Am J Kidney Dis 2014;64:74-85. [Crossref] [PubMed]
- Villa G, Ringgaard S, Hermann I, et al. Phase-contrast magnetic resonance imaging to assess renal perfusion: a systematic review and statement paper. MAGMA 2020;33:3-21. [Crossref] [PubMed]
- Wolf M, de Boer A, Sharma K, et al. Magnetic resonance imaging T1- and T2-mapping to assess renal structure and function: a systematic review and statement paper. Nephrol Dial Transplant 2018;33:ii41-50. [Crossref] [PubMed]
- Zhou H, Zhang J, Zhang XM, et al. Noninvasive evaluation of early diabetic nephropathy using diffusion kurtosis imaging: an experimental study. Eur Radiol 2021;31:2281-8. [Crossref] [PubMed]
- Feng YZ, Chen XQ, Yu J, et al. Intravoxel incoherent motion (IVIM) at 3.0 T: evaluation of early renal function changes in type 2 diabetic patients. Abdom Radiol (NY) 2018;43:2764-73. [Crossref] [PubMed]
- Wang R, Lin Z, Yang X, et al. Noninvasive Evaluation of Renal Hypoxia by Multiparametric Functional MRI in Early Diabetic Kidney Disease. J Magn Reson Imaging 2022;55:518-27. [Crossref] [PubMed]
- Makvandi K, Hockings PD, Jensen G, et al. Multiparametric magnetic resonance imaging allows non-invasive functional and structural evaluation of diabetic kidney disease. Clin Kidney J 2022;15:1387-402. [Crossref] [PubMed]
- Mora-Gutiérrez JM, Garcia-Fernandez N, Slon Roblero MF, et al. Arterial spin labeling MRI is able to detect early hemodynamic changes in diabetic nephropathy. J Magn Reson Imaging 2017;46:1810-7. [Crossref] [PubMed]
- Gertz RJ, Gerhardt F, Kröger JR, et al. Spectral Detector CT-Derived Pulmonary Perfusion Maps and Pulmonary Parenchyma Characteristics for the Semiautomated Classification of Pulmonary Hypertension. Front Cardiovasc Med 2022;9:835732. [Crossref] [PubMed]
- Mochizuki J, Nakaura T, Yoshida N, et al. Spectral imaging with dual-layer spectral detector computed tomography for the detection of perfusion defects in acute coronary syndrome. Heart Vessels 2022;37:1115-24. [Crossref] [PubMed]
- Consensus Committee. Consensus statement on the worldwide standardization of the hemoglobin A1C measurement: the American Diabetes Association, European Association for the Study of Diabetes, International Federation of Clinical Chemistry and Laboratory Medicine, and the International Diabetes Federation. Diabetes Care 2007;30:2399-400. [Crossref] [PubMed]
- Kundel HL, Polansky M. Measurement of observer agreement. Radiology 2003;228:303-8. [Crossref] [PubMed]
- Kang H. Sample size determination and power analysis using the G*Power software. J Educ Eval Health Prof 2021;18:17. [Crossref] [PubMed]
- Luik PT, Hoogenberg K, Van Der Kleij FG, et al. Short-term moderate sodium restriction induces relative hyperfiltration in normotensive normoalbuminuric Type I diabetes mellitus. Diabetologia 2002;45:535-41. [Crossref] [PubMed]
- Sigmund EE, Vivier PH, Sui D, et al. Intravoxel incoherent motion and diffusion-tensor imaging in renal tissue under hydration and furosemide flow challenges. Radiology 2012;263:758-69. [Crossref] [PubMed]
- Pourghasem M, Nasiri E, Shafi H. Early renal histological changes in alloxan-induced diabetic rats. Int J Mol Cell Med 2014;3:11-5.
- Deng Y, Yang B, Peng Y, et al. Use of intravoxel incoherent motion diffusion-weighted imaging to detect early changes in diabetic kidneys. Abdom Radiol (NY) 2018;43:2728-33. [Crossref] [PubMed]
- Caramori ML, Fioretto P, Mauer M. Low glomerular filtration rate in normoalbuminuric type 1 diabetic patients: an indicator of more advanced glomerular lesions. Diabetes 2003;52:1036-40. [Crossref] [PubMed]
- Wang L, Wu J, Cheng JF, et al. Diagnostic value of quantitative contrast-enhanced ultrasound (CEUS) for early detection of renal hyperperfusion in diabetic kidney disease. J Nephrol 2015;28:669-78. [Crossref] [PubMed]
- Raptis AE, Viberti G. Pathogenesis of diabetic nephropathy. Exp Clin Endocrinol Diabetes 2001;109:S424-37. [Crossref] [PubMed]
- Rossi C, Artunc F, Martirosian P, et al. Histogram analysis of renal arterial spin labeling perfusion data reveals differences between volunteers and patients with mild chronic kidney disease. Invest Radiol 2012;47:490-6. [Crossref] [PubMed]
- Legrand M, Mik EG, Johannes T, et al. Renal hypoxia and dysoxia after reperfusion of the ischemic kidney. Mol Med 2008;14:502-16. [Crossref] [PubMed]
- Baek JH, Zeng R, Weinmann-Menke J, et al. IL-34 mediates acute kidney injury and worsens subsequent chronic kidney disease. J Clin Invest 2015;125:3198-214. [Crossref] [PubMed]
- Li S, Wang F, Sun D. The renal microcirculation in chronic kidney disease: novel diagnostic methods and therapeutic perspectives. Cell Biosci 2021;11:90. [Crossref] [PubMed]
- Caramori ML, Fioretto P, Mauer M. Low glomerular filtration rate in normoalbuminuric type 1 diabetic patients: an indicator of more advanced glomerular lesions. Diabetes 2003;52:1036-40. [Crossref] [PubMed]
- Thacker JM, Li LP, Li W, et al. Renal Blood Oxygenation Level-Dependent Magnetic Resonance Imaging: A Sensitive and Objective Analysis. Invest Radiol 2015;50:821-7. [Crossref] [PubMed]
- Yin WJ, Liu F, Li XM, et al. Noninvasive evaluation of renal oxygenation in diabetic nephropathy by BOLD-MRI. Eur J Radiol 2012;81:1426-31. [Crossref] [PubMed]
- Kreft E, Sałaga-Zaleska K, Sakowicz-Burkiewicz M, et al. Diabetes Affects the A1 Adenosine Receptor-Dependent Action of Diadenosine Tetraphosphate (Ap4A) on Cortical and Medullary Renal Blood Flow. J Vasc Res 2021;58:38-48. [Crossref] [PubMed]
- Lee VS, Rusinek H, Bokacheva L, et al. Renal function measurements from MR renography and a simplified multicompartmental model. Am J Physiol Renal Physiol 2007;292:F1548-59. [Crossref] [PubMed]
- Peng XG, Bai YY, Fang F, et al. Renal lipids and oxygenation in diabetic mice: noninvasive quantification with MR imaging. Radiology 2013;269:748-57. [Crossref] [PubMed]
- Radenković M, Stojanović M, Prostran M. Experimental diabetes induced by alloxan and streptozotocin: The current state of the art. J Pharmacol Toxicol Methods 2016;78:13-31. [Crossref] [PubMed]
- Zheng SS, He YM, Lu J. Noninvasive evaluation of diabetic patients with high fasting blood glucose using DWI and BOLD MRI. Abdom Radiol (NY) 2021;46:1659-69. [Crossref] [PubMed]
- Cai YZ, Li ZC, Zuo PL, et al. Diagnostic value of renal perfusion in patients with chronic kidney disease using 3D arterial spin labeling. J Magn Reson Imaging 2017;46:589-94. [Crossref] [PubMed]
- Pruijm M, Milani B, Pivin E, et al. Reduced cortical oxygenation predicts a progressive decline of renal function in patients with chronic kidney disease. Kidney Int 2018;93:932-40. [Crossref] [PubMed]
- Li LP, Tan H, Thacker JM, et al. Evaluation of Renal Blood Flow in Chronic Kidney Disease Using Arterial Spin Labeling Perfusion Magnetic Resonance Imaging. Kidney Int Rep 2017;2:36-43. [Crossref] [PubMed]
- Mohamed Osman NM, Abdel Kader M, Aziz Nasr TAEL, et al. The Role of Diffusion-Weighted MRI and Apparent Diffusion Coefficient in Assessment of Diabetic Kidney Disease: Preliminary Experience Study. Int J Nephrol Renovasc Dis 2021;14:1-10. [Crossref] [PubMed]
- Şahan MH, Özdemir A, Asal N, et al. Pancreas and kidney changes in type 2 diabetes patients: the role of diffusion-weighted imaging. Turk J Med Sci 2021;51:1289-95. [Crossref] [PubMed]