Prognostic impact of highly solid component in early-stage solid lung adenocarcinoma
Introduction
Primary lung cancer is one of the most common malignancies and the leading cause of cancer-related death in both sexes in the United States (1). Based on computed tomography (CT) findings, issued guidelines for the therapeutic management of lung cancer distinguish between subsolid and solid nodules, which mean nodules with ground-glass lesions or not. The prognosis of solid nodules is much worse than that of subsolid nodules, even if the nodules are subcentimeter in size (i.e., with a tumor size of ≤1 cm) (2,3). According to several studies, the maximum solid part size, rather than the total tumor size, is a better predictor of the invasiveness and prognosis of subsolid tumors (4-6). Therefore, the solid part size has been incorporated as a clinical T factor for lung cancer in the 8th edition of the Union for International Cancer Control tumor-node-metastasis (TNM) classification (7,8).
Although total and solid sizes were previously determined using a two-dimensional (2D) axial CT scan, multidetector CT now allows for a three-dimensional (3D) scan, and maximum and solid sizes could be calculated on 3D views. According to some studies, using a 3D solid volume of lung adenocarcinoma could help determine pathologic tumor invasiveness and lymph node metastases more accurately (9). Recently, it was discovered that 3D solid volume greater than 0 HU within the tumor predicts postoperative prognosis for patients with part-solid lung cancer better than 2D maximum solid diameter on axial imaging or 3D maximal solid size (10). Moreover, voxel-based histogram analysis of 3D CT by radiomics technology has gained popularity in recent years (11). There is, however, no systematic method for determining the prognosis of solid lung tumors on CT.
In this study, we evaluated the relationship between 3D volumetry value on a CT and postoperative disease-free survival (DFS) to discover the prognostic factor of early-stage solid lung adenocarcinoma, and compared the radiological and pathological findings for these cancers. We present this article in accordance with the STROBE reporting checklist (available at https://qims.amegroups.com/article/view/10.21037/qims-23-36/rc).
Methods
Study population
We retrospectively searched the database in our tertiary care hospital from January 2007 to December 2012. Patients with surgically resected lung adenocarcinoma harboring pathological stage I and radiological solid findings [consolidation to tumor ratio (C/T) =1.0] on thin-section CT were selected. The median follow-up time was 67 months. Patients were excluded if key clinical data were missing. The clinical records of all recruited patients were reviewed to obtain the clinicopathological and postoperative survival data to compare recurrent and non-recurrent patients. The Institutional Review Board of Nagoya University Hospital approved this retrospective, single-center study (No. 2018-0258). The study was conducted in accordance with the Declaration of Helsinki (as revised in 2013), and individual consent for this retrospective analysis was waived.
Three-dimensional CT scan protocol
Preoperatively, Aquilion 16 and Aquilion 64 (Toshiba Medical, Tokyo, Japan) were used to collect all noncontrast 3D CT images in the craniocaudal direction under the following conditions: X-ray tube voltage, 120 kVp; automatic tube current modulation, maximum of 500 mA; gantry rotation speed, 0.5 sec; and beam collimation, 1 mm or 0.5 mm. Using a high-spatial-frequency technique (FC 86 or FC52; Toshiba Medical), whole lung axial thin-section multidetector CT scans were reconstructed at the same increment with a section thickness of 1 or 0.5 mm.
Two- and three-dimensional calculation on a workstation
All images from the CT reconstruction were imported into a commercially accessible 3D workstation (VINCENT, version 4.3; Fujifilm Medical, Tokyo, Japan). Two oncologists (a thoracic surgeon with 17 years of experiences and a chest radiologist with 28 years of experiences) independently evaluated the axial multiplanar reconstructed and 3D images of excised tumors using window and width settings of 600 and 1,800 HU, respectively. The two readers were aware that the measuring target was solid lung cancer, but they were not aware of the pathologic findings or the prognosis. On the 3D workstation, the surgeon first drew a region of interest (ROI) for the primary tumor. Next, the radiologist corrected the ROI. Then, the ROI was finalized by consensus of the two.
The tumor size on the two-dimensional axial image (2DS) was measured by readers. Furthermore, the 3D tumor volume between −600 and 199 HU (3DV) and the 3D solid volume between 0 and 199 HU (3DSV) on multiplanar reconstructed images were automatically calculated on the workstation according to previous study (10). Additionally, 3DV and 3DSV-derived tumor diameters were calculated from volumes as if the tumors were sphere (12).
Pathological evaluation
Hematoxylin and eosin-stained slides were independently reviewed and scored by two pathologists (YH and TT). The scoring was well-matched between the two pathologists. However, further discussion was performed to get a consensus in several cases. The diameter of the entire tumor and the invasive component were measured on the largest tumor cross-section. In those diagnosed with invasive mucinous adenocarcinoma and colloid adenocarcinoma, the invasive diameter was considered to be equal to the tumor diameter. In the previous studies (13-17), tumor volume in clinical CT images or in preclinical animal experiments was calculated as follows: tumor volume (mm3) = (major axis: mm) × (minor axis: mm)2 × 0.5236. This study applied this method for pathological invasive volume (PIV) evaluation as follows: PIV (mm3) = (major invasive axis: mm) × (minor invasive axis: mm)2 × 0.5236, which assumes both ellipsoid tumor configuration and invasive structure. Histological tumor grade was determined using the WHO classification of thoracic tumors, 5th edition (18). The tumor differentiation pattern was determined by dividing the tumor area into three categories: well-differentiated (lepidic), moderately differentiated (papillary or glandular), and poorly differentiated (solid or cribriform).
Statistical analysis
The features of the tumor and the patient were compared between recurrence and non-recurrence. Categorical variables are expressed as numbers [percentage (%)], and continuous variables are presented as medians (interquartile range). Age, tumor size, tumor volume, maximum standard uptake value (SUVmax), 18F fluorodeoxyglucose positron emission tomography/computed tomography (FDG-PET/CT), and serum carcinoembryonic antigen (CEA) level were compared using the Mann-Whitney test, whereas sex, epidermal growth factor receptor (EGFR) mutation status, pleural, lymphatic, and vascular invasion were compared with the Fisher’s exact test. DFS was calculated using the Kaplan-Meier analysis, and the survival curves for each subgroup based on the cutoff value of 2DS, 3DV, or 3DSV were evaluated using the log-rank test. DFS was defined as the time between surgery and the first recurrence or death for any reason. Recurrence was diagnosed by imaging examinations. In both univariate and multivariate analyses of DFS, the Cox proportional hazards model was applied to evaluate the effect of 2DS, 3DV, 3DSV, and clinicopathological characteristics. Based on the thresholds from previous studies, 2DS, 3DV, and 3DSV were simplified to a score of 0–3 using calculated 2DS, 3DV, and 3DSV-derived tumor diameters (12). Score 0, 1, 2, and 3 corresponds to diameters of ≤10, 11–20, 21–30, and >30 mm, respectively. The area under the curve (AUC) of the receiver operating characteristic (ROC) curve for a 5-year DFS rate based on (ridge) penalized Cox proportional hazards models were evaluated by repeated nested cross-validation. P values of <0.05 were considered statistically significant. R was used for statistical analysis.
Results
Baseline patient characteristics
Figure S1 depicts the flowchart of our research population. From January 2007 to December 2012, 709 patients who underwent complete resection were detected in our institution. As a pathological type, only adenocarcinoma (n=470) was chosen, and progressive diseases with more than stage II (n=151) were excluded. Additionally, part-solid and pure ground-glass opacity (GGO) types in CT findings (n=229) were ruled out. Finally, our cohort included 90 cases of lung adenocarcinoma and this cohort included 26 and 64 patients with and without recurrence, respectively. Table S1 displays the patient and tumor characteristics.
Difference between recurrent and non-recurrent lung cancers
Table 1 compares the characteristics of patients with recurrence and non-recurrence. Patient characteristics such as age, sex, and smoking were not different between the two groups. In the recurrence group, the proportion of patients with pleural invasion in the tumor was higher than in the non-recurrence group (P=0.010), but not lymphatic and vascular invasion. As a genetic feature, the proportion of EGFR mutants was significantly higher in the recurrence group than in the non-recurrence group (P=0.044). Additionally, 2DS, SUVmax on FDG-PET/CT, and serum CEA levels, which had previously been identified as prognostic factors in lung cancer patients (19-21), did not differ significantly between two groups.
Table 1
Variables | Recurrence (n=26) | Non-recurrence (n=64) | P value |
---|---|---|---|
Age (years) | 68 [62–71] | 68 [62–72] | 0.758 |
SUVmax on FDG-PET | 5.70 [3.50–7.67] | 5.09 [3.32–7.04] | 0.408 |
Serum CEA level | 4.00 [2.40–6.40] | 3.70 [2.18–7.70] | 0.841 |
2DS (mm) | 24.5 [18.3–29.8] | 21 [17.0–28.5] | 0.101 |
Sex (male), n (%) | 14 (53.8) | 47 (73.4) | 0.085 |
Smoking, n (%) | 0.124 | ||
Non-smoker | 13 (50.0) | 19 (29.7) | |
Ex-smoker | 10 (38.4) | 27 (58.6) | |
Current smoker | 3 (11.5) | 18 (28.1) | |
Procedure, n (%) | 0.270 | ||
Lobectomy | 25 (96.2) | 55 (85.9) | |
Segmentectomy/sublobar | 1 (0.8) | 9 (14.1) | |
Pleural invasion, n (%) | 0.010 | ||
+ | 20 (76.9) | 29 (45.3) | |
− | 6 (23.1) | 35 (54.7) | |
Lymphatic invasion, n (%) | 0.167 | ||
+ | 9 (34.6) | 12 (18.8) | |
− | 17 (65.4) | 52 (81.2) | |
Vascular invasion, n (%) | 0.285 | ||
+ | 5 (19.2) | 6 (9.4) | |
− | 21 (80.8) | 58 (90.6) | |
EGFR, n (%) | 0.044 | ||
Mutant | 12 (46.1) | 15 (23.4) | |
WT | 14 (53.9) | 49 (76.6) |
Data are shown as median [IQR] or n (%). SUVmax, maximum standard uptake value; FDG PET, [18F] fluorodeoxyglucose positron emission tomography; CEA, carcinoembryonic antigen; 2DS, tumor diameter on two-dimensional axial CT; EGFR, epidermal growth factor receptor; WT, wild type; IQR, interquartile range; CT, computed tomography.
Significance of the tumor volumetry on CT for the prognosis of patients with solid lung adenocarcinoma
Figure 1A depicts histograms based on 3D volumetry for solid lung adenocarcinoma, with each bar representing median tumor volumes of recurrent (red) or non-recurrent (blue) cases, which were segmentalized in increments of 10 HU. Total tumor 3DV was higher in the recurrent group compared to the non-recurrent group (P=0.019, Figure 1B). However, the significance of each subdivided volume differed between recurrent and non-recurrent cases, with the 3D volume with 0–199 HU (3DSV) being significantly higher in recurrent cases (P=0.009, Figure 1C, Table S2). Therefore, we hypothesized that 3DSV would be associated with solid tumor malignancy and affect the postoperative prognosis of the patient.
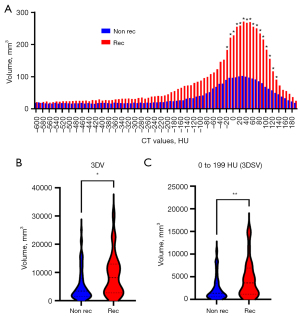
Survival analysis stratified by volumetry value
We performed DFS analysis based on several radiological values, including 2DS, 3DV, and 3DSV (Figure 2) and DFS was significantly differing only according to 3DSV (log-rank test for 2DS: P=0.332, 3DV: P=0.240, 3DSV: P=0.013). The univariate analysis indicated that pleural invasion [hazard ratio, 3.389; 95% confidence interval (CI), 1.360–8.449], EGFR mutation (hazard ratio: 2.313; 95% CI: 1.068–5.011), 3DSV (hazard ratio: 2.295; 95% CI: 1.307–4.028) and 3DSV/3DV (hazard ratio: 1.055; 95% CI: 1.010–1.012) were significantly related to DFS (Table 2). The multivariate analysis revealed a significant association between 3DSV (hazard ratio: 2.440; 95% CI: 1.110–5.361) and EGFR mutation (hazard ratio in Model 2DS: 4.471, 95% CI: 1.401–14.270; hazard ratio in Model 3DV: 4.477, 95% CI: 1.368–14.649; hazard ratio in Model 3DSV: 4.307, 95% CI: 1.328–13.977) and DFS (Table 3). Univariate ROC analysis for 5-year DFS was performed on the 2DS, 3DV, and 3DSV and 3DSV indicated highest AUC in these three radiological findings (2DS: AUC =0.653, 3DV: AUC =0.656, 3DSV: AUC =0.714) (Figure 3A). Multivariate ROC analysis was conducted for pleural invasion and EGFR mutation in addition to three radiological findings, and 3DSV showed the greatest AUC (2DS: AUC =0.783, 3DV: AUC =0.747, and 3DSV: AUC =0.805) (Figure 3B).
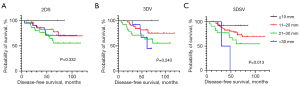
Table 2
Variables | Hazard ratio (95% CI) | P value |
---|---|---|
Age (years) | 0.994 (0.945–1.045) | 0.807 |
Female sex | 1.711 (0.791–3.701) | 0.172 |
Pleural invasion | 3.389 (1.360–8.449) | 0.009 |
Lymphatic invasion | 1.995 (0.885–4.500) | 0.096 |
Vascular invasion | 2.319 (0.869–6.188) | 0.093 |
SUVmax on FDG-PET | 1.495 (0.763–2.930) | 0.242 |
CEA | 1.077 (0.728–1.593) | 0.712 |
EGFR mutation | 2.313 (1.068–5.011) | 0.034 |
GGO | 1.931 (0.886–4.209) | 0.098 |
2DS | 1.292 (0.826–2.020) | 0.262 |
3DV* | 1.585 (0.986–2.548) | 0.057 |
3DSV** | 2.295 (1.307–4.028) | 0.004 |
3DSV/3DV (%) | 1.055 (1.010–1.012) | 0.016 |
*, converted diameter derived from 3DV; **, converted diameter derived from 3DSV. CI, confidence interval; SUVmax, maximum standard uptake value; FDG-PET, [18F] fluorodeoxyglucose positron emission tomography; CEA, carcinoembryonic antigen; EGFR, epidermal growth factor receptor; GGO, ground-glass opacity; 2DS, tumor size on the two-dimensional axial image; 3DV, three-dimensional tumor volume between –600 and 199 HU; 3DSV, three-dimensional solid volume between 0 and 199 HU.
Table 3
Variables | Model 2DS | Model 3DV | Model 3DSV | |||||
---|---|---|---|---|---|---|---|---|
Hazard ratio (95% CI) | P value | Hazard ratio (95% CI) | P value | Hazard ratio (95% CI) | P value | |||
Age (years) | 0.974 (0.914–1.039) | 0.428 | 0.982 (0.921–1.048) | 0.586 | 0.995 (0.933–1.060) | 0.875 | ||
Female | 0.978 (0.292–3.278) | 0.972 | 0.920 (0.276–3.063) | 0.892 | 0.816 (0.246–2.700) | 0.739 | ||
Pleural invasion | 2.776 (0.933–8.266) | 0.067 | 3.053 (0.992–9.397) | 0.052 | 2.862 (0.993–8.248) | 0.052 | ||
Lymphatic invasion | 1.727 (0.665–4.483) | 0.262 | 1.703 (0.649–4.473) | 0.280 | 1.569 (0.578–4.258) | 0.377 | ||
Vascular invasion | 2.453 (0.620–9.704) | 0.201 | 2.529 (0.613–10.428) | 0.199 | 1.889 (0.427–8.367) | 0.402 | ||
SUVmax | 1.428 (0.472–4.324) | 0.529 | 1.848 (0.604–5.653) | 0.282 | 1.234 (0.389–3.908) | 0.721 | ||
CEA | 0.946 (0.594–1.506) | 0.814 | 0.942 (0.608–1.461) | 0.791 | 0.979 (0.613–1.564) | 0.931 | ||
EGFR mutation | 4.471 (1.401–14.270) | 0.011 | 4.477 (1.368–14.649) | 0.013 | 4.307 (1.328–13.977) | 0.015 | ||
2DS | 1.678 (0.892–3.156) | 0.108 | – | – | – | – | ||
3DV† | – | – | 1.157 (0.585–2.286) | 0.675 | – | – | ||
3DSV‡ | – | – | – | – | 2.440 (1.110–5.361) | 0.026 |
†, converted diameter derived from 3DV; ‡, converted diameter derived from 3DSV. 2DS, tumor size on the two-dimensional axial image; 3DV, three-dimensional tumor volume between –600 and 199 HU; 3DSV, three-dimensional solid volume between 0 and 199 HU; CI, confidence interval; SUVmax, maximum standard uptake value; CEA, carcinoembryonic antigen; EGFR, epidermal growth factor receptor.
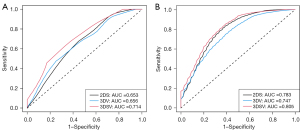
Cross-point between CT volumetry and pathological findings
Table 4 displays the pathological findings of 90 solid lung adenocarcinomas. The median values of both maximum tumor and invasive diameter did not differ significantly between the two groups. On the other hand, PIV and the proportion of moderate differentiated part were significantly higher in the recurrent group than in the non-recurrent group (invasive volume: P=0.034; moderate differentiated part: P=0.044). When PIV was compared to 3DV and 3DSV on multidetector CT, it was found to be very similar with 3DSV, but significantly lower than 3DV (P<0.001, mean square error for 3DSV: 0.420, mean square error for 3DV: 1.152, Figure 4A,4B). Figure 5 depicts a comparison of pathologic and CT findings. These two cases had similar 3DV (Figure 5A: 13,237 mm3, Figure 5B: 12,913 mm3), but the recurrent case had a much larger 3DSV (Figure 5C: 9,043 mm3, Figure 5D: 5,644 mm3). The recurrent case had no lepidic pattern in the pathologic finding (Figure 5E), whereas non-recurrent case had a lepidic pattern (Figure 5F), and high-power histopathologic images showed the difference between two cases more clearly (Figure 5G,5H). The lepidic growth region was excluded by 3DSV on CT, indicating the reproducibility between preoperative 3DSV and postoperative pathological invasiveness.
Table 4
Variables | Recurrence (n=26) | Non-recurrence (n=64) | P value |
---|---|---|---|
Maximum tumor diameter (mm) | 22.5 [18.3–26.8] | 19 [14.8–25.0] | 0.148 |
Maximum invasive diameter (mm) | 21.0 [16.0–26.8] | 18.0 [13.8–22.0] | 0.145 |
Invasive volume (mm3) | 1,943 [890–4,202] | 1,521 [668–2,580] | 0.034 |
Differentiation (%) | |||
Well | 10 [5–15] | 5 [0–15] | 0.818 |
Moderate | 75 [33–80] | 45 [20–75] | 0.044 |
Poor | 15 [5–59] | 50 [5–80] | 0.100 |
Grade, n (%) | 0.147 | ||
1 | 0 (0.0) | 1 (1.5) | |
2 | 14 (53.8) | 22 (34.3) | |
3 | 12 (46.2) | 34 (53.1) | |
Unknown | 0 (0.0) | 7 (10.9) |
Data are shown as median [IQR] or n (%). IQR, interquartile range.
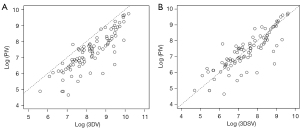
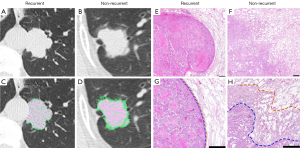
Discussion
Lung cancers can be classified as solid or subsolid based on the density features on CT scans, with markedly differing morphological and pathological characteristics (22,23). Solid lung cancerous nodules have poorer prognosis than subsolid nodules due to their faster growth and rapid metastases (24-26). Actually, patients with solid nodules showed a significantly worse postoperative prognosis than patient with subsolid nodules (12). In this study, however, over 70% of patients with stage I solid lung adenocarcinoma had no postoperative recurrence. Therefore, investigating the recurrence of early-stage solid lung cancer is important for efficiently distinguishing postoperative high-risk patients.
Since the 8th edition of the TNM classification system for lung cancer (27), T description has been determined by invasiveness rather than the largest diameter of the tumor. Several studies have been conducted to investigate the relationship between radiological C/T ratio and pathological findings in patients with lung adenocarcinoma (28-30). The most basic method for determining the size of a solid component is conventional 2D diametrical measurement. Tumor solid components, on the other hand, are frequently spherical and irregular in shape. Even if the maximum size of the multiplanar images was assessed, the diagnostic accuracy for predicting recurrence remained unchanged (31). As a result, 3D assessment of the solid component is critical to improving recurrence diagnosis accuracy in solid or subsolid lung tumors.
To decide on the optimal border of the CT number between recurrence and non-recurrence, we compared the volume distribution for solid lung adenocarcinoma and found that 3DSV indicated significant difference between two groups and this finding is consistent with a recent paper that found measuring 3D solid volume greater than 0 HU predicted the prognosis of part-solid lung cancer (10). Additionally, no recurrent patient has a 3DSV value less than 516 mm3. The value of 516 mm3 is close to 520 mm3, which is the volume of a sphere with a diameter of 10 mm and correspond to the TNM categorization boundary between T1a and T1b (7). Moreover, 3DSV had an optimal AUC value and was an independent prognostic predictor unlike serum CEA level and SUVmax on FDG-PET/CT, which were previously identified as predictors of postoperative survival (21,32,33). Furthermore, we investigated other prognostic factors, including the presence of the GGO component and the 3DSV/3DV ratio. However, the diagnostic performance of the above factors was not superior to 3DSV, probably because this study only enrolled patients with early-stage solid lung adenocarcinoma on CT. However, 3DSV worked well as a prognostic factor for this patient population. We need to continue the study for any kind of lung adenocarcinoma, including solid and part-solid nodules, to confirm the usefulness of 3DSV in clinical practice.
On a CT scan, distinguish the GGO or noninvasive component from the solid component can be difficult. According to previous research, the boundary value for the visually assessed solid region on the lung window settings is −160 HU (5). However, intratumoral collapse, fibrosis, and oncologic invasive components were included in the visually assessed solid component. Based on the clinically significant results in our study, we assumed that 3DSV would be an important preoperative detector of precise invasive component. This finding demonstrated the importance of preoperative 3DSV evaluation in predicting postoperative prognosis and pathological malignancy of the tumor. Solid lung adenocarcinoma with high 3DSV has a high risk of postoperative recurrence, and even if it is pathological stage I, close follow-up with imaging and tumor markers may be required after surgery.
Our study had some limitations. First, this was a retrospective study with a single institution and a small number of patients. Consequently, additional research involving multiple centers may be required. Second, only DFS was used to assess patient survival since the prognosis for patients with recurrence has improved due to the recent introduction of a tyrosine kinase inhibitor that is effective against the majority of adenocarcinomas with certain genetic alterations (34). Third, 2D total size was subjectively assessed without the use of computer-assisted diagnosis. The survival analysis for 2DS should be enhanced if computer-aided diagnostics could determine the solid maximum diameter of the solid tumor.
Conclusions
The preoperative assessment of 3DSV, which is highly correlated with PIV, accurately predicted the postoperative prognosis in patients with early-stage solid lung adenocarcinoma.
Acknowledgments
Funding: None.
Footnote
Reporting Checklist: The authors have completed the STROBE reporting checklist. Available at https://qims.amegroups.com/article/view/10.21037/qims-23-36/rc
Conflicts of Interest: All authors have completed the ICMJE uniform disclosure form (available at https://qims.amegroups.com/article/view/10.21037/qims-23-36/coif). The authors have no conflicts of interest to declare.
Ethical Statement: The authors are accountable for all aspects of the work in ensuring that questions related to the accuracy or integrity of any part of the work are appropriately investigated and resolved. The study was conducted in accordance with the Declaration of Helsinki (as revised in 2013). The study was approved by the Institutional Review Board of Nagoya University Hospital (approval No. 2018-0258) and individual consent for this retrospective analysis was waived.
Open Access Statement: This is an Open Access article distributed in accordance with the Creative Commons Attribution-NonCommercial-NoDerivs 4.0 International License (CC BY-NC-ND 4.0), which permits the non-commercial replication and distribution of the article with the strict proviso that no changes or edits are made and the original work is properly cited (including links to both the formal publication through the relevant DOI and the license). See: https://creativecommons.org/licenses/by-nc-nd/4.0/.
References
- Siegel RL, Miller KD, Wagle NS, Jemal A. Cancer statistics, 2023. CA Cancer J Clin 2023;73:17-48. [Crossref] [PubMed]
- Li M, Zhu L, Lv Y, Shen L, Han Y, Ye B. Thin-slice computed tomography enables to classify pulmonary subsolid nodules into pre-invasive lesion/minimally invasive adenocarcinoma and invasive adenocarcinoma: a retrospective study. Sci Rep 2023;13:6999. [Crossref] [PubMed]
- Ridge CA, Yildirim A, Boiselle PM, Franquet T, Schaefer-Prokop CM, Tack D, Gevenois PA, Bankier AA. Differentiating between Subsolid and Solid Pulmonary Nodules at CT: Inter- and Intraobserver Agreement between Experienced Thoracic Radiologists. Radiology 2016;278:888-96. [Crossref] [PubMed]
- Nakamura S, Fukui T, Taniguchi T, Usami N, Kawaguchi K, Ishiguro F, Hirakawa A, Yokoi K. Prognostic impact of tumor size eliminating the ground glass opacity component: modified clinical T descriptors of the tumor, node, metastasis classification of lung cancer. J Thorac Oncol 2013;8:1551-7. [Crossref] [PubMed]
- Yoshida Y, Sakamoto M, Maeda E, Ohtsu H, Ota S, Asamura H, Nakajima J. Can image analysis on high-resolution computed tomography predict non-invasive growth in adenocarcinoma of the lung? Ann Thorac Cardiovasc Surg 2015;21:8-13. [Crossref] [PubMed]
- Liu Y, Sun H, Zhou F, Su C, Gao G, Ren S, Zhou C, Zhang Z, Shi J. Imaging features of TSCT predict the classification of pulmonary preinvasive lesion, minimally and invasive adenocarcinoma presented as ground glass nodules. Lung Cancer 2017;108:192-7. [Crossref] [PubMed]
- Travis WD, Asamura H, Bankier AA, Beasley MB, Detterbeck F, Flieder DB, Goo JM, MacMahon H, Naidich D, Nicholson AG, Powell CA, Prokop M, Rami-Porta R, Rusch V, van Schil P, Yatabe Y; . The IASLC Lung Cancer Staging Project: Proposals for Coding T Categories for Subsolid Nodules and Assessment of Tumor Size in Part-Solid Tumors in the Forthcoming Eighth Edition of the TNM Classification of Lung Cancer. J Thorac Oncol 2016;11:1204-23.
- Aokage K, Miyoshi T, Ishii G, Kusumoto M, Nomura S, Katsumata S, Sekihara K, Hishida T, Tsuboi M. Clinical and Pathological Staging Validation in the Eighth Edition of the TNM Classification for Lung Cancer: Correlation between Solid Size on Thin-Section Computed Tomography and Invasive Size in Pathological Findings in the New T Classification. J Thorac Oncol 2017;12:1403-12.
- Shikuma K, Menju T, Chen F, Kubo T, Muro S, Sumiyoshi S, Ohata K, Sowa T, Nakanishi T, Cho H, Neri S, Aoyama A, Sato T, Sonobe M, Date H. Is volumetric 3-dimensional computed tomography useful to predict histological tumour invasiveness? Analysis of 211 lesions of cT1N0M0 lung adenocarcinoma. Interact Cardiovasc Thorac Surg 2016;22:831-8. [Crossref] [PubMed]
- Kamiya S, Iwano S, Umakoshi H, Ito R, Shimamoto H, Nakamura S, Naganawa S. Computer-aided Volumetry of Part-Solid Lung Cancers by Using CT: Solid Component Size Predicts Prognosis. Radiology 2018;287:1030-40. [Crossref] [PubMed]
- Yoshiyasu N, Kojima F, Hayashi K, Bando T. Radiomics technology for identifying early-stage lung adenocarcinomas suitable for sublobar resection. J Thorac Cardiovasc Surg 2021;162:477-485.e1. [Crossref] [PubMed]
- Iwano S, Umakoshi H, Kamiya S, Yokoi K, Kawaguchi K, Fukui T, Naganawa S. Postoperative recurrence of clinical early-stage non-small cell lung cancers: a comparison between solid and subsolid nodules. Cancer Imaging 2019;19:33. [Crossref] [PubMed]
- Wang S, Lin D, Yang X, Zhan C, Zhao S, Luo R, Wang Q, Tan L. Clinical significance of PET/CT uptake for peripheral clinical N0 non-small cell lung cancer. Cancer Med 2020;9:2445-53. [Crossref] [PubMed]
- Lindell RM, Hartman TE, Swensen SJ, Jett JR, Midthun DE, Mandrekar JN. 5-year lung cancer screening experience: growth curves of 18 lung cancers compared to histologic type, CT attenuation, stage, survival, and size. Chest 2009;136:1586-95. [Crossref] [PubMed]
- Wang L, Fu JN, Wang JY, Jin CJ, Ren XY, Tan Q, Li J, Yin HW, Xiong K, Wang TY, Liu XM, Zeng HH. Selenium-containing thioredoxin reductase inhibitor ethaselen sensitizes non-small cell lung cancer to radiotherapy. Anticancer Drugs 2011;22:732-40. [Crossref] [PubMed]
- Wang L, Li X, Song YM, Wang B, Zhang FR, Yang R, Wang HQ, Zhang GJ. Ginsenoside Rg3 sensitizes human non-small cell lung cancer cells to γ-radiation by targeting the nuclear factor-κB pathway. Mol Med Rep 2015;12:609-14. [Crossref] [PubMed]
- Jia X, Gu Z, Chen W, Jiao J. Tigecycline targets nonsmall cell lung cancer through inhibition of mitochondrial function. Fundam Clin Pharmacol 2016;30:297-306. [Crossref] [PubMed]
- WHO Classification of Tumours Editorial Board. Thoracic Tumors. 5th ed. International Agency for Research on Cancer, Lyon, France; 2021.
- Hammarström S. The carcinoembryonic antigen (CEA) family: structures, suggested functions and expression in normal and malignant tissues. Semin Cancer Biol 1999;9:67-81. [Crossref] [PubMed]
- Nasralla A, Lee J, Dang J, Turner S. Elevated preoperative CEA is associated with subclinical nodal involvement and worse survival in stage I non-small cell lung cancer: a systematic review and meta-analysis. J Cardiothorac Surg 2020;15:318. [Crossref] [PubMed]
- Shigefuku S, Ito H, Miura J, Kikuchi A, Isaka T, Adachi H, Nakayama H, Ikeda N. Prognostic Significance of the Maximum Standardized Uptake Value on the Prognosis of Clinical Stage IA Lung Adenocarcinoma Based on the 8th Edition TNM Classification. Ann Surg Oncol 2023;30:830-8.
- Naidich DP, Bankier AA, MacMahon H, Schaefer-Prokop CM, Pistolesi M, Goo JM, Macchiarini P, Crapo JD, Herold CJ, Austin JH, Travis WD. Recommendations for the management of subsolid pulmonary nodules detected at CT: a statement from the Fleischner Society. Radiology 2013;266:304-17. [Crossref] [PubMed]
- Chu ZG, Zhang Y, Li WJ, Li Q, Zheng YN, Lv FJ. Primary solid lung cancerous nodules with different sizes: computed tomography features and their variations. BMC Cancer 2019;19:1060. [Crossref] [PubMed]
- Oda S, Awai K, Murao K, Ozawa A, Utsunomiya D, Yanaga Y, Kawanaka K, Yamashita Y. Volume-doubling time of pulmonary nodules with ground glass opacity at multidetector CT: Assessment with computer-aided three-dimensional volumetry. Acad Radiol 2011;18:63-9. [Crossref] [PubMed]
- Wolf AS, Richards WG, Jaklitsch MT, Gill R, Chirieac LR, Colson YL, Mohiuddin K, Mentzer SJ, Bueno R, Sugarbaker DJ, Swanson SJ. Lobectomy versus sublobar resection for small (2 cm or less) non-small cell lung cancers. Ann Thorac Surg 2011;92:1819-23; discussion 1824-5. [Crossref] [PubMed]
- Lee SM, Park CM, Paeng JC, Im HJ, Goo JM, Lee HJ, Kang CH, Kim YW, Kim JI. Accuracy and predictive features of FDG-PET/CT and CT for diagnosis of lymph node metastasis of T1 non-small-cell lung cancer manifesting as a subsolid nodule. Eur Radiol 2012;22:1556-63. [Crossref] [PubMed]
- Goldstraw P, Chansky K, Crowley J, Rami-Porta R, Asamura H, Eberhardt WE, Nicholson AG, Groome P, Mitchell A, Bolejack V. The IASLC Lung Cancer Staging Project: Proposals for Revision of the TNM Stage Groupings in the Forthcoming (Eighth) Edition of the TNM Classification for Lung Cancer. J Thorac Oncol 2016;11:39-51. [Crossref] [PubMed]
- Hattori A, Matsunaga T, Takamochi K, Oh S, Suzuki K. Oncological Characteristics of Radiological Invasive Adenocarcinoma with Additional Ground-Glass Nodules on Initial Thin-Section Computed Tomography: Comparison with Solitary Invasive Adenocarcinoma. J Thorac Oncol 2016;11:729-36. [Crossref] [PubMed]
- Katsumata S, Aokage K, Nakasone S, Sakai T, Okada S, Miyoshi T, Tane K, Hayashi R, Ishii G, Tsuboi M. Radiologic Criteria in Predicting Pathologic Less Invasive Lung Cancer According to TNM 8th Edition. Clin Lung Cancer 2019;20:e163-70.
- Suzuki K, Koike T, Asakawa T, Kusumoto M, Asamura H, Nagai K, Tada H, Mitsudomi T, Tsuboi M, Shibata T, Fukuda H, Kato HJapan Lung Cancer Surgical Study Group. (JCOG LCSSG). A prospective radiological study of thin-section computed tomography to predict pathological noninvasiveness in peripheral clinical IA lung cancer (Japan Clinical Oncology Group 0201). J Thorac Oncol 2011;6:751-6. [Crossref] [PubMed]
- Jeong B, Lee SM, Park S, Choe J, Choi S, Do KH, Seo JB. Prognostic performance in lung cancer according to tumor size: Comparison of axial, multiplanar, and 3-dimensional CT measurement to pathological size. Eur J Radiol 2021;144:109976. [Crossref] [PubMed]
- Xu H, Zhao G, Lin J, Ye Q, Xiang J, Yan B. A combined preoperative red cell distribution width and carcinoembryonic antigen score contribute to prognosis prediction in stage I lung adenocarcinoma. World J Surg Oncol 2023;21:56. [Crossref] [PubMed]
- Chen HH, Chiu NT, Su WC, Guo HR, Lee BF. Prognostic value of whole-body total lesion glycolysis at pretreatment FDG PET/CT in non-small cell lung cancer. Radiology 2012;264:559-66. [Crossref] [PubMed]
- Huang Q, Li J, Sun Y, Wang R, Cheng X, Chen H. Efficacy of EGFR tyrosine kinase inhibitors in the adjuvant treatment for operable non-small cell lung cancer by a meta-analysis. Chest 2016;149:1384-92. [Crossref] [PubMed]