Science mapping analysis of computed tomography-derived fractional flow reverse: a bibliometric review from 2012 to 2022
Introduction
Coronary heart disease (CHD) threatens public health globally as a major cause of fatality. Pathologically, atherosclerosis of the coronary artery results in luminal stenosis, contracture, and obstruction, which further leads to myocardial ischemia. Currently, there is a hybrid approach to assessing coronary ischemic lesions involving both anatomic and physiologic tests; the complementary nature of these tests could improve the strength of ischemic differentiation (1).
Although invasive coronary angiography (ICA) and non-invasive coronary computed tomography angiography (CCTA) are jointly established as class I recommendations for CHD diagnosis in the guidelines (2), their limitations have been exposed. The limitations of ICA, regarded as the gold standard for stenosis assessment, include its high expense, the invasiveness of the procedure, and the iatrogenic damage caused by the contrast agent (3); meanwhile, the merely anatomical evaluation of coronary stenosis provided by more conservative CCTA, which ignores the functional hemodynamics, fails in differentiating specific ischemia effectively (4). In general, flow reserve begins to abate when diameter stenosis is >50% and diminishes substantially when diameter stenosis is >75% (1,5). Vice versa, it has been reported that a non-negligible of number patients with mild coronary stenosis (<50%) could experience myocardial ischemia (6). The anatomical and functional tests show a poor mutual correlation thus generating misdiagnoses and missed diagnoses by CCTA.
Introduced as a hemodynamics index, fractional flow reserve (FFR) has emerged as the gold standard for the compatible assessment of ischemic lesions (7) and is defined as the ratio of blood flow distal to a stenotic lesion to theoretically normal maximal flow in the distribution supplied by the same artery. Functional significance has been considered when coronary stenosis has an FFR value <0.75 (5). However, as it involves the use of a pressure sensor-tipped guidewire in cardiac catheterization (8), FFR measurement is restricted by its invasiveness. As a result, computed tomography (CT)-derived FFR (CT-FFR) acts as an ideal alternative due to its non-invasiveness and lack of an adenosine injection.
In the measurement of ischemia, the fundamental step is to construct a three-dimensional (3D) anatomic model based on the CCTA image of a stenotic artery. Then, computational fluid dynamics (CFD) and the Navier-Stokes equation are used to perform the operation on the CCTA model (9). Through this algorithm, the maximal hyperemia could be simulated by the effect of intravenous adenosine (10). The computing results are visualized, with areas measured as having identical CT-FFR values endowed with the same color.
Multiple studies have suggested that CT-FFR values show good consistency with invasive FFR and better diagnostic performance than CCTA alone in diagnosing stenosis (11,12). Regarded as the gatekeeper of ICA, the negative CT-FFR threshold value is acknowledged as 0.80 (13-15). In recent years, several trials have validated the significant role of CT-FFR in guiding clinical decisions by identifying patients’ needs for revascularization and selecting appropriate strategies for revascularization (16-18).
The diagnostic performance of CT-FFR appears comparable in sensitivity, yet with greater specificity, to CCTA (19). When extensive calcification is more likely to lead to overrated stenosis severity, the machine learning (ML) algorithm of CT-FFR mitigates the influence of calcification on CCTA imaging, which is considered the Achilles heel of CCTA (20). Applied in cardiology, artificial intelligence (AI) has the potential to improve the efficiency of clinical workflow, provide objective and reproducible quantitative results, increase interpretative speed, and inform subsequent clinical pathways (21). With continuous advancements in AI, the combination of AI technology and medical diagnostics is inevitable and a milestone.
Methods
Data source and search
Retrieval of articles was conducted on April 23, 2022, from the Web of Science Core Collection (WoSCC), because it is recognized as one of the most authoritative databases comprising comprehensive publications in many scientific fields.
We set up the retrieval strategy based on terms identified in previously published articles. The full name of this technology based on CCTA is “fractional flow reserve computed/calculated from computed tomography” (common abbr. “FFRct”) (22). Other researchers would like to refer to it as “computed tomography-derived fractional flow reverse” (abbr. “CT-FFR”), and “non-invasive fractional flow reserve” is also mentioned in some articles (23). In this review, the searching strategy, based on lexical search, was as follows: (TS= [“computed tomography fractional flow reserve” OR “fractional flow reserve from computed tomography” OR “CT-FFR” OR “FFRct” OR “non-invasive fractional flow reserve”]) AND (Language= [English]). We restricted the time span of included literature to within the past 10 years (Jan. 2012 to Apr. 2022).
A total of 2,200 publications were found. Then, the exclusion was performed in terms of document types, including 209 meeting abstracts, 183 editorial materials, 42 letters, 26 early access, 4 corrections, 2 data papers, 20 case reports, 8 reference materials, 2 retractions, 10 book chapters, and 2 news items. After exclusion, 1,712 (1,350 articles and 362 reviews) records containing information of the authors, titles, abstracts, keywords, references, and so on were refined to export as “plain text file” format.
Search screening was conducted by two independent reviewers (XHZ and XPZ) to control the selection bias. Designed as a bibliometric review, this study did not require approval on either ethics or informed consent.
Scientometric analysis methods
We chose CiteSpace (http://cluster.cis.drexel.edu/~cchen/citespace/) to fabricate the knowledge mapping for its several views of interactive visualizations, including a cluster view, a timeline view, and a hierarchical view (24). CiteSpace5.8.R3 software is operated to process the secondary data and explore the two fundamental aspects of information science: intellectual bases and research fronts (25). Users can quickly access the research status, trends, and critical turning points of the research domain. The interpretation of the running results of CiteSpace can be summarized in four steps.
Firstly, the visualized knowledge maps are made up of nodes and links. Different nodes represent research items (i.e., institutions, countries, authors, keywords, etc.). Links connecting nodes represent relationships of co-occurrence or co-citation; the thicker links represent the higher correlation. Publication years are featured with various colors of each node and link (26); more specifically, the warmer the color tone, the more recent the term.
Secondly, there are two indicators to discern the transformative discoveries in a specialty. The betweenness centrality (BC) measures the percentage of the shortest paths in a network to which a given node belongs, commonly used to highlight potential pivotal points of paradigm shift over time (27). Burst detection, a computational technique to identify sharp increases of interest within a certain period, serves to detect abrupt changes and emerging trends (25).
Thirdly, cluster analysis also plays a prominent role in CiteSpace. Terms in the articles are classified into several clusters according to the inter-group similarity, and the central member of the cluster inferred by the algorithm as recurring themes in the cited articles will be generated as a label (28). CiteSpace distributes ID #0 to the largest cluster, and the clusters gradually shrink when the ID number increases. The resultant clusters use multidimensional scaling and color the members of these clusters accordingly (29).
Fourthly, in the timeline view, each cluster is shown horizontally and advances from left to right over time. The generation of annual labels based on the latent semantic indexing (LSI) algorithm is laid on the corresponding chronological line.
Before running CiteSpace, all operation metrics were set to default.
Results
Distribution of annual publications
To explore the pace of investigation in the CT-FFR domain, the distribution of annual publications comes as the first indicator. The annual distribution of 1,712 publications was shown as a column chart (Figure 1). A positive linear trend suggests the approximately increasing number of annual publications year by year, which means the CT-FFR domain is gradually being paid attention to. Especially in the past 6 years, the literature quantity hitting a higher level than before has accrued steadily, except for in 2018, where it slightly declined among the averagely increasing trend.
Distribution of spatial co-occurrence
Investigating countries/institutions can reflect the overall structure of a specific field and clarify the influence of different countries/institutions and the cooperative relationship between them. The co-occurrence maps and ranking of the top 5 countries/institutions are displayed in Figure 2A-2C and Table 1. According to Table 1, the top 5 countries and institutions contributed 1,457 articles (85%) and 354 articles (21%), respectively. The top 5 countries with the highest publication quantity also had high BC values (above 0.1) (30); they were the USA, the Netherlands, China, England, and Germany. The USA had the topmost publication quantity and BC, suggesting its outstanding role in CT-FFR research.
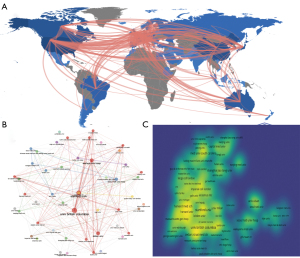
Table 1
Rank | Countries | Institutions | |||||
---|---|---|---|---|---|---|---|
Description | Quantity | Centrality | Description | Quantity | Centrality | ||
1 | USA | 638 | 0.28 | Univ British Columbia | 82 | 0.04 | |
2 | Netherlands | 226 | 0.11 | Stanford Univ | 78 | 0.61 | |
3 | China | 225 | 0.17 | Aarhus Univ Hosp | 72 | 0.02 | |
4 | England | 190 | 0.3 | Cedars Sinai Med Ctr | 64 | 0.06 | |
5 | Germany | 178 | 0.5 | Leiden Univ | 58 | 0 |
Indicated by Table 1, Stanford University, with the highest BC of 0.61, was considered the institution that had carried out the most scholarly communication and cooperation with others. In contrast, others showed relatively lower BC and interaction. Indisputably, the representation of the co-occurrence map cannot be the sole indicator to measure the position of the country/institution in the CT-FFR domain. The predominant publication of the USA and its research institutions may have led to the omission of European institutions in the top rankings.
Co-occurrence map of authors
A co-occurrence map of authors can provide information on influential research groups and help look for potential collaborators. A total of 509 researchers contributed 1,712 articles; the distribution of authors is presented in Figure 3, and the ranking of the top 10 productive authors is displayed in Table 2. As shown in Figure 3 and Table 2, the most significant node of the author was Jonathan Leipsic from the University of British Columbia, with the most publication frequency and the highest BC; James K. Min from Cornell University shared a cooperative relationship with Jonathan Leipsic.
Table 2
Rank | Authors | Quantity | Centrality |
---|---|---|---|
1 | Jonathon Leipsic | 64 | 0.24 |
2 | James K. Min | 62 | 0.14 |
3 | U. Joseph Schoepf | 55 | 0.21 |
4 | Koen Nieman | 53 | 0.13 |
5 | Gianluca Pontone | 50 | 0.07 |
6 | Bjarne L. Norgaard | 44 | 0.05 |
7 | Bonkwon Koo | 42 | 0.09 |
8 | Daniele Andreini | 41 | 0.11 |
9 | Bernard De Bruyne | 37 | 0.11 |
10 | Daniel S. Berman | 35 | 0.06 |
Besides the factors mentioned above, the co-occurrence map was relatively densely distributed, and 6 of the top 10 authors had high BC; we can infer that there was close cooperation in the CT-FFR domain.
Co-occurrence map of categories
Scientific literature is a collection of publications focusing on theoretical or practical issues in different fields (31). CiteSpace is designed to mitigate gaps in the knowledge domain and help researchers develop a good understanding of each category of skills and knowledge (28). Based on the co-occurrence map and the ranking of quantity, BC, and burst (Figure 4, Table 3), the development and use of CT-FFR involve multiple academic subjects other than the sub-disciplines belonging to clinical medicine, such as cardiology, radiology and medical imaging, and general and internal medicine. The terms with higher centrality (e.g., computer science, engineering, and mathematics) also a held vital role in CT-FFR. The technologies of CT-FFR and quantitative FFR both involve collaboration between the engineering and medical communities that apply fluid dynamic principles to calculate estimates of the invasively measured FFR value (32). As ML and AI are utilized to increase diagnostic accuracy and improve the modelling data quality of coronary physiology (33,34); computer science, a positive node in burst detection, was considered the frontier subject.
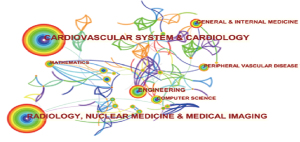
Table 3
Rank | Subject | Frequency | Centrality | Burst |
---|---|---|---|---|
1 | Cardiac & Cardiovascular Systems | 1,438 | 0.38 | 3.84 |
2 | Radiology, Nuclear Medicine, & Medical Imaging | 645 | 0.03 | 5.07 |
3 | Engineering | 131 | 0.35 | – |
4 | Computer Science | 108 | 0.68 | 3.78 |
5 | Mathematics | 100 | 0.3 | – |
Analysis of topic distribution
Keywords can effectively embody the central view of scientific literature. Aiming to delve further into hot topics and future frontier topics (35), the top 20 keywords with the highest frequency were ranked, as shown in Table 4. Combined with keyword distribution and burst detection, we summarized the main topic as four trends: demonstrating the CT-FFR’s non-inferiority or even the superiority of diagnostic and prognostic performance over ICA and CCTA (mentioned 913 times in 1,912 studies), the comparison between CT-FFR and other similar cardiac imaging tests [e.g., quantification FFR with 140 occurrences, magnetic resonance imaging (MRI) with 152 occurrences, emission CT with 119 occurrences, transluminal attenuation gradient (TAG) with 67 occurrences], the ever-expanding clinical application of CT-FFR (e.g., guiding the optimal medical therapy 106 times, guiding revascularization 93 times), and innovation of technical support (e.g., computed fluid dynamics with 93 occurrences, ML with 55 occurrences, or AI with 28 occurrences).
Table 4
Rank | Keywords | Frequency |
---|---|---|
1 | Fractional flow reserve | 1,288 |
2 | Computed tomography angiography | 1,249 |
3 | Coronary artery disease | 1,224 |
4 | Diagnostic performance | 483 |
5 | Diagnostic accuracy | 330 |
6 | Computed tomography | 283 |
7 | Blood flow | 245 |
8 | Stenosis | 189 |
9 | Intervention | 187 |
10 | Prognostic value | 160 |
11 | Quantification | 140 |
12 | Myocardial perfusion | 137 |
13 | Emission computed tomography | 119 |
14 | Severity | 116 |
15 | Guideline | 107 |
16 | Medical therapy | 106 |
17 | Follow up | 105 |
18 | Intravascular ultrasound | 104 |
19 | Revascularization | 93 |
20 | Computational fluid dynamics | 93 |
Knowledge mapping of keywords
Figure 5A displays the 225 keywords with more than 15 occurrences, furthermore, to generalize the research topics, we exposed the mainstream by the word cloud view (Figure 5B). On the strength of the co-occurrence map, the network consisting of the 9 largest keywords clusters was obtained through the log-likelihood ratio (LLR) algorithm, as shown in Figure 5C. Table 5 enumerates each cluster. The silhouette is an index to measure the homogeneity of a cluster when the values are bigger than 0.7 (30), leading to efficient and reliable results, and the results can be reasonable when their values are bigger than 0.5 (36). Thus, the cluster in Figure 5B can be regarded as satisfactory.
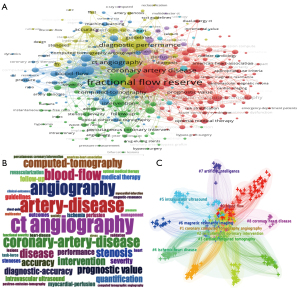
Table 5
Cluster ID | Size | Silhouette | Mean (year) | Label (LLR) |
---|---|---|---|---|
0 | 95 | 0.596 | 2015 | Blood flow |
1 | 87 | 0.651 | 2014 | Coronary computed tomography angiography |
2 | 77 | 0.585 | 2015 | Percutaneous coronary intervention |
3 | 64 | 0.584 | 2014 | Cardiac computed tomography |
4 | 53 | 0.741 | 2016 | Ischemic heart disease |
5 | 46 | 0.62 | 2015 | Intravascular ultrasound |
6 | 33 | 0.745 | 2015 | Magnetic resonance imaging |
7 | 26 | 0.761 | 2017 | Artificial intelligence |
8 | 13 | 0.886 | 2016 | Coronary heart disease |
LLR, log-likelihood ratio.
Burst detection of keywords
Burst detection explores the mentioned frequencies of dramatically increasing terms over a period, and its strength is related to the degree of attention being paid to it. Figure 6 enumerates the top 20 keywords with the strongest citation bursts, where the red column indicates the burst period of a research direction whereas the blue column indicates the whole decade span [2012–2022]. By analyzing Table 6, the research frontiers can be summarized as “emission computed tomography” [2012–2014], “American Heart Association” [2013–2015], “artery stenosis” [2013–2017], “syntax score” [2018–2019], “artificial intelligence” [2020–2022], “plaque characteristics” [2020–2022], and “deep learning” [2020–2022].
Table 6
Rank | Frequency | Centrality | Year | Journal | Author | Title |
---|---|---|---|---|---|---|
1 | 326 | 0.03 | 2014 | J Am Coll Cardiol | Norgaard BL | Diagnostic performance of noninvasive fractional flow reserve derived from coronary computed tomography angiography in suspected coronary artery disease |
2 | 252 | 0.02 | 2012 | JAMA-J Am Med Assoc | Min JK | Diagnostic accuracy of fractional flow reserve from anatomic CT angiography |
3 | 179 | 0.01 | 2012 | New Engl J Med | De Bruyne B | Fractional flow reserve–guided PCI versus medical therapy in stable coronary disease |
4 | 179 | 0.03 | 2011 | J Am Coll Cardiol | Koo BK | Diagnosis of ischemia-causing coronary stenoses by noninvasive fractional flow reserve computed from coronary computed tomographic angiograms |
5 | 176 | 0.02 | 2013 | J Am Coll Cardiol | Taylor CA | Computational fluid dynamics applied to cardiac computed tomography for noninvasive quantification of fractional flow reserve |
6 | 156 | 0.06 | 2015 | New Engl J Med | Douglas PS | Outcomes of anatomical versus functional testing for coronary artery disease |
7 | 150 | 0.05 | 2015 | Eur Heart J | Douglas PS | Clinical outcomes of fractional flow reserve by computed tomographic angiography-guided diagnostic strategies vs. usual care in patients with suspected coronary artery disease: the prospective longitudinal trial of FFRct: outcome and resource impacts study |
8 | 142 | 0.01 | 2020 | Eur Heart J | Knuuti J | 2019 ESC guidelines for the diagnosis and management of chronic coronary syndromes |
9 | 140 | 0.01 | 2013 | Eur Heart J | Montalescot G | 2013 ESC guidelines on the management of stable coronary artery disease |
10 | 124 | 0.02 | 2016 | J Am Coll Cardiol | Douglas PS | 1-year outcomes of FFRCT-guided care in patients with suspected coronary disease the PLATFORM study |
CT, computed tomography; FFRct, fractional flow reserve computed/calculated from computed tomography; PCI, percutaneous coronary intervention; ESC, European Society of Cardiology; FFRCT, fractional flow reserve computed from computed tomography; PLATFORM, Prospective Longitudinal Trial of FFRCT: Outcomes and Resource Impacts.
Analysis of co-citation reference
Co-citation analysis reveals the groundbreaking articles constituting the intellectual base and how research interests evolve and contribute to the development of the CT-FFR domain. Figure 7A shows the co-citation network, and the top 10 references cited most frequently are listed in Table 6.
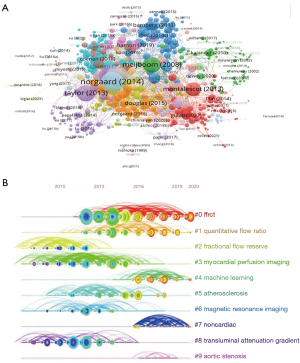
Nørgaard et al. (11) carried forward the Next Step (NXT) trial, a prospective, multi-center trial, which has indicated that CT-FFR has a better diagnostic performance than CCTA alone in the differentiation of ischemic lesions. Taylor accomplished a review of the principle of CFD modelling of coronary flow and pressure (9). Douglas compared CTA strategy and functional tests on patients with suspected CHD. He concluded that CTA does not improve clinical outcomes, emphasizing the necessity of advancing the non-invasive functional assessment (37). He also ran the Prospective Longitudinal Trial of FFRCT: Outcomes and 229 Resource Impacts (PLATFORM) trial to verify the role of CT-FFR in decreasing unnecessary ICA (14). The 2019 European Society of Cardiology (ESC) guidelines for chronic coronary syndrome also recommended that CT-FFR was non-inferior to ICA and FFR for decision-making and targets for revascularization (2).
The timeline view is another two-dimensional (2D) feature of CiteSpace, which integrates cluster analysis and time slice; the results are shown in Figure 7B. Each cluster represents the leading research frontier emerging in chronological order.
As plentiful evidence has shown that merely anatomical without hemodynamic evaluation cannot support efficient diagnosis, researchers started to explore multiple imaging tools to perform the functional assessment of the suspected culprit artery. According to Figure 7B, earlier than in 2010, there were three test tools put into use. FFR (cluster#2) is acknowledged as the 1A recommendation for guiding percutaneous coronary revascularization by the 2021 American College of Cardiology (ACC)/American Heart Association (AHA)/Society for Cardiovascular Angiography and Interventions (SCAI) guidelines (38); however, its adoption into clinical work has limitations due to its invasiveness and secondary side effects. Myocardial perfusion imaging (MPI) (cluster#3) is a common way to quantify myocardial blood flow through CT and MRI (cluster#6). Perfusion cardiac magnetic resonance (CMR) is appealing for its high specificity, which addresses the high sensitivity but low specificity of CT-FFR, and these two examinations show similar overall diagnostic accuracy (39). TAG (cluster#8) is a derivative of CCTA imaging, which enables morphological and functional assessment concurrently in a non-invasive manner. Stuijfzand et al. did not find a difference in TAG value between the positive or negative value of CT-FFR and better diagnostic accuracy than CCTA alone, which might indicate suboptimal diagnostic performance (40).
Consequently, the scientific literature about CT-FFR (cluster#0) and quantitative flow ratio (QFR) (cluster#1) has surged until now. As a result of CFD having been applied to cardiology, CT-FFR and QFR are designed as qualified surrogates. QFR shows higher accuracy than CT-FFR, which can be explained by more essential details provided by angiographic images (41). Meanwhile, CT-FFR is more suitable for the outpatient population to avoid unnecessary invasive tests.
Atherosclerosis (cluster#5) is the adopted disease of the CT-FFR test; the correlational research was traced back to before 2010 and turned into hotspots between 2011 and 2016. In recent years, investigators have been dedicated to broadening the clinical application of CT-FFR, including the interpretation of plaque characteristics and aortic stenosis (cluster#9). Michail et al. implemented a prospective study demonstrating the safety and feasibility of applying CT-FFR to patients with severe aortic stenosis for the first time (42).
Since 2014, the ML (cluster#4) algorithm has been paid growing attention to for its superior modeling effect on CFD. Even as the limitations of AI modeling are objectives, such as the poor correlation of invasive FFR and considerable time to extract anatomical features from CCTA for ML (20), the combination of AI and medical science is still regarded as a milestone and future direction in the development of CT-FFR.
Discussion
Other than common reviews, bibliometrics analysis is characterized by systematical and comprehensive inclusion of correlated research, so as to locate landmark literature in this field, track current hotspots and trends, and predict the future direction of progression.
According to the knowledge mapping of the keywords, we classified the hotspots of present status as the following aspects.
The diagnostic performance and accuracy of CT-FFR
Although anatomical stenosis diagnosed by CCTA cannot predict some ischemic lesions which are observed better hemodynamically, as suggested by multicenter trial data, the CT-FFR value shows a remarkable correlation with invasive FFR; incorporating CT-FFR remarkably improves the diagnostic performance of ischemia and alters decision-making over CCTA alone (11,43,44). The deFACTO study found that CT-FFR enabled more effective discrimination and correct reclassification of per-vessel for intermediate ischemic lesions over measures of CT stenosis, which is significant for those who did not meet the traditional criteria of angiographically severe yet conferred hemodynamic importance (45). Besides that, the application of CT-FFR in the emergency room has been given less attention. A retrospective study by Chinnaiyan et al. found no statistically significant difference in major adverse cardiac events (MACEs) for patients with acute chest pain when CT-FFR was applied compared with CCTA alone (2.7% vs. 4.3%, P=0.310), and it is feasible to defer the revascularization by negative CT-FFR value (46).
The precise CT-FFR measurement relies on accurate luminal modelling, and the calcification of different severity adversely affects the artery boundary imaging (9). However, according to a prospective multicenter trial by Nørgaard et al., there is no statistically significant difference in the diagnostic performance of CT-FFR by CFD across all coronary artery calcification (CAC) score groups (11). This might be due to the flow-limiting degree of lesions (47). An FFR ≤0.80 is accepted as a definite threshold value in ischemic differentiation; nevertheless, the closest value to 0.80 of CT-FFR could mislead the dichotomous diagnosis. The diagnostic accuracy of 46% is restricted to the grey zone of non-invasive FFR value between 0.70 and 0.80, which requires more comprehensive tests to guide clinical decision-making (48). It is noteworthy that a few researchers are dedicated to determining the rejection rate of CT-FFR and identifying the correlates of thinner CT section thickness and lower patient heart rate, which has helped to refine this novel technology (49).
The prognostic value provided by CT-FFR
CT-FFR might be an independent predictor of prognosis for patients with CHD. A newly released meta-analysis conducted that the numerical value was inversely associated with unfavorable clinical outcomes (50). According to the PLATFORM, the accession of functional assessment of CT-FFR, which plays the role of gatekeeper of invasive examination and treatment, prevented the reported 50% increase in ICA and revascularizations and brought lower costs as well as better quality for life for patients (15,51). As for long-term follow-up, as reported by the Assessing Diagnostic Value of Non-invasive FFRCT in Coronary Care (ADVANCE) trial, the 1-year outcomes of the patients with negative CT-FFR show lower rates of revascularization and a trend toward less MACEs compared with patients with a positive test result (52). A median follow-up of 4.7 years organized by a sub-analysis of the NXT trial indicated that a CT-FFR value of 0.8 or less was a superior predictor compared with the presence of clinically significant stenosis (defined as greater than 70%) on coronary CT angiograms, which extended the safety of negative CT-FFR result to approximately 5 years (53).
Revascularization guided by CT-FFR
Complete revascularization in CHD aims to eliminate all ischemia-inducing lesions including percutaneous coronary intervention (PCI) and coronary artery bypass grafting (CABG). The PLATFORM study noticed that 230 patients (61%) of ICA in the CT-FFR group were cancelled safely and dramatically lowered the rate of finding non-obstructive CHD (14). As the research concerning CT-FFR evolves, it has been demonstrated that the application of CT-FFR is gradually expanding from diagnosis (the gatekeeper of ICA) to treatment (the road mapper of revascularization). In the SYNTAX III REVOLUTION trial, the heart team refined the decision-making for revascularization by correcting the overestimated severity of CHD from CCTA and changing treatment recommendations in 7% of patients (17). When CT-FFR is applied to guide PCI, the impact of an intervention on hemodynamics can be simulated and used to predict the likelihood of procedural success (54). The ongoing FASTTRACK CABG, a first-in-man study, is in the continuation of the SYNTAX III REVOLUTION trial, which probes into the feasibility of CT-FFR in cardio surgery by replacing ICA as a surgical guidance method for planning and execution of CABG in patients with 3-vessel disease (41). However, more outcome data is needed to support the ulterior recommendation for selecting CT-FFR in revascularization by guidelines.
CT-FFR vs. QFR
QFR, another hemodynamic index, is a novel technique based on CFD for the rapid computation of FFR from radioactive ICA. Similar to CT-FFR, QFR has the significant advantage of higher safety and reduction of procedure time, risk, and costs of unnecessarily using a pressure guidewire or inducing maximum hyperemia. With FFR set as the baseline, a study showed that QFR had a positive discovery rate of 81% and a negative predictive value (NPV) of 90%, whereas CT-FFR had a positive predictive value (PPV) of 70% and an NPV of 82% (41). Despite the better potential in diagnostic accuracy of QFR, by contrast, CT-FFR is more appropriate for outpatients without evident coronary syndrome to reduce unnecessary ICA (55).
CT-FFR and CT-MPI
With the technical advancements of CT scanners, investigators’ interests have gradually shifted to performing CT-MPI, which aims to evaluate coronary blood flow effectively. This technique is used to image the transit of contrast material from the coronary arteries to the myocardium, and hypo-enhanced areas represent myocardial hypo-perfusion (56). The need for extra scanning and pharmacological stimulus is a limitation of CT-MPI compared with CT-FFR. Presented by a prospective study, the combination of CCTA+CT-FFR showed better sensitivity and NPV, but lower specificity, accuracy, and PPV over the combination of CCTA + CT-MPI, yet the CCTA + CT-FFR + CT-MPI elevated the efficiency on the above aspects (57). These two methods can be complementary to facilitate optimal decision-making.
Burst detection is the representative function of CiteSpace by elucidating respective research hotspots chronologically. At an early stage, the research focused on demonstrating the non-inferiority or even the superiority in differentiating ischemia of CT-FFR to other similar imaging examinations; until recently, more attention has been paid to amplifying the clinical application and innovative development of CT-FFR such as AI support.
Emission CT and CT-FFR
To evaluate myocardial perfusion, positron emission tomography (PET) for absolute quantification and single-photon emission CT (SPECT) for visual analysis are common reference standards (56). SPECT is the most commonly performed non-invasive stress test; compared with CT-FFR, which yields high diagnostic sensitivity, the correlation might be moderate (58). However, these two CT-based imaging tools are expected to combine to improve diagnostic accuracy and optimize the non-invasive method to guide clinical decisions.
TAG and CT-FFR
By definition, TAG, another hemodynamically non-invasive measurement of CCTA images, is the contrast opacification gradient along the lumen of a coronary artery on CCTA images (40), and the significant stenosis could enhance the contrast descent more dramatically. Yoon et al. found that CT-FFR showed better diagnostic accuracy than TAG in ischemic lesions (59). Also, compared to CT-FFR, the limitation of TAG lies in the effect of multiple heartbeats and severe calcification on the interpretation of the results.
ML and AI technology in CT-FFR
The deep neural network of the ML CT-FFR model is built on abundant CFD-based experiments (20). Compared to CFD modeling, ML-based CT-FFR is additionally trained by a specific subset of AI algorithms and is more capable of improving diagnostic accuracy. Coenen et al. reported that diagnostic accuracy improved from 58% by CTA to 78% by ML-based CT-FFR on a per-vessel basis (60). To overcome the major shortcoming of the too-long processing time of CT-FFR, the ML-based coronary CFD protocols allow the time to generate numerical results within a matter of hours, even if sent offsite for analysis (61). Applying AI technology to the clinical practice of cardiology is undoubtedly progressive, paradigm-shifting, and represents the future trend (34).
Plaque characteristics
The presence of adverse plaque characteristics, including positive remodeling, napkin-ring sign, low-attenuation plaque, and spotty calcification, is associated with plaque rupture (60). Lee et al. reported that culprit lesions in acute coronary syndrome exhibited lower CT-FFR and higher magnitude of ΔCT-FFR, the difference between the proximal and distal CT-FFR, showing the best incremental reclassification ability among different hemodynamic parameters (62).
This review is not without limitations. First, we chose WoSCC as the only database resource for its predominant collection of authoritative articles and because it is the only database directly matching the import format of CiteSpace. However, not using other databases could have led to an information gap and bias, which is an ineligible issue for this review. Second, the time lag, which takes the period for the accumulation of high cited frequency, would result in overlooking the detection of research fronts is the main challenge of co-citation analysis (24).
Conclusions
Due to the increasing morbidity and mortality of CHD, the earliest possible adjustment of lifestyle, pharmacological therapies, and non-invasive testing for suspected CHD patients is highly important. This review analyzed literature concerning CT-FFR published within the past decade on WoSCC in the form of a bibliometric and visual review. CiteSpace, an efficient data processing tool, was chosen to visualize the research status and frontiers through figures and tables.
For the first time, this review analyzed science literature about CT-FFR. It makes it more straightforward for researchers to grasp existing results from multiple dimensions, seek potential collaborators, and establish further research directions (30).
Acknowledgments
All the authors thank Guang’anmen Hospital, China Academy of Chinese Medical Sciences, for the support of this work and the reviewers for allowing us to improve the manuscript.
Funding: This work was supported by
Footnote
Conflicts of Interest: All authors have completed the ICMJE uniform disclosure form (available at https://qims.amegroups.com/article/view/10.21037/qims-22-1094/coif). All authors report that this work was supported by the Scientific and Technological Innovation Project of the China Academy of Chinese Medical Sciences (No. CI2021A03318). The authors have no other conflicts of interest to declare.
Ethical Statement: The authors are accountable for all aspects of the work in ensuring that questions related to the accuracy or integrity of any part of the work are appropriately investigated and resolved. Due to the literature review design of the present study, neither ethics approval nor informed consent was applicable.
Open Access Statement: This is an Open Access article distributed in accordance with the Creative Commons Attribution-NonCommercial-NoDerivs 4.0 International License (CC BY-NC-ND 4.0), which permits the non-commercial replication and distribution of the article with the strict proviso that no changes or edits are made and the original work is properly cited (including links to both the formal publication through the relevant DOI and the license). See: https://creativecommons.org/licenses/by-nc-nd/4.0/.
References
- Marcus ML, Skorton DJ, Johnson MR, Collins SM, Harrison DG, Kerber RE. Visual estimates of percent diameter coronary stenosis: "a battered gold standard". J Am Coll Cardiol 1988;11:882-5. [Crossref] [PubMed]
- Knuuti J, Wijns W, Saraste A, Capodanno D, Barbato E, Funck-Brentano C, et al. 2019 ESC Guidelines for the diagnosis and management of chronic coronary syndromes. Eur Heart J 2020;41:407-77. [Crossref] [PubMed]
- Pothineni NV, Shah NN, Rochlani Y, Nairooz R, Raina S, Leesar MA, Uretsky BF, Hakeem A U.S.. Trends in Inpatient Utilization of Fractional Flow Reserve and Percutaneous Coronary Intervention. J Am Coll Cardiol 2016;67:732-3. [Crossref] [PubMed]
- Shaw LJ, Hausleiter J, Achenbach S, Al-Mallah M, Berman DS, Budoff MJ, et al. Coronary computed tomographic angiography as a gatekeeper to invasive diagnostic and surgical procedures: results from the multicenter CONFIRM (Coronary CT Angiography Evaluation for Clinical Outcomes: an International Multicenter) registry. J Am Coll Cardiol 2012;60:2103-14. [Crossref] [PubMed]
- Meijboom WB, Van Mieghem CA, van Pelt N, Weustink A, Pugliese F, Mollet NR, Boersma E, Regar E, van Geuns RJ, de Jaegere PJ, Serruys PW, Krestin GP, de Feyter PJ. Comprehensive assessment of coronary artery stenoses: computed tomography coronary angiography versus conventional coronary angiography and correlation with fractional flow reserve in patients with stable angina. J Am Coll Cardiol 2008;52:636-43. [Crossref] [PubMed]
- Curzen N, Rana O, Nicholas Z, Golledge P, Zaman A, Oldroyd K, Hanratty C, Banning A, Wheatcroft S, Hobson A, Chitkara K, Hildick-Smith D, McKenzie D, Calver A, Dimitrov BD, Corbett S. Does routine pressure wire assessment influence management strategy at coronary angiography for diagnosis of chest pain?: the RIPCORD study. Circ Cardiovasc Interv 2014;7:248-55. [Crossref] [PubMed]
- Neumann FJ, Sousa-Uva M, Ahlsson A, Alfonso F, Banning AP, Benedetto U, et al. 2018 ESC/EACTS Guidelines on myocardial revascularization. Eur Heart J 2019;40:87-165. Erratum in: Eur Heart J 2019;40:3096. [Crossref] [PubMed]
- Koo BK. Fractional flow reserve for coronary bifurcation lesions: can fractional flow reserve-guided side branch intervention strategy improve clinical outcomes compared with angiography-guided strategy? JACC Cardiovasc Interv 2015;8:547-9. [Crossref] [PubMed]
- Taylor CA, Fonte TA, Min JK. Computational fluid dynamics applied to cardiac computed tomography for noninvasive quantification of fractional flow reserve: scientific basis. J Am Coll Cardiol 2013;61:2233-41. [Crossref] [PubMed]
- Dai X, Lu Z, Yu Y, Yu L, Xu H, Zhang J. The use of lesion-specific calcium morphology to guide the appropriate use of dynamic CT myocardial perfusion imaging and CT fractional flow reserve. Quant Imaging Med Surg 2022;12:1257-69. [Crossref] [PubMed]
- Nørgaard BL, Leipsic J, Gaur S, Seneviratne S, Ko BS, Ito H, et al. Diagnostic performance of noninvasive fractional flow reserve derived from coronary computed tomography angiography in suspected coronary artery disease: the NXT trial (Analysis of Coronary Blood Flow Using CT Angiography: Next Steps). J Am Coll Cardiol 2014;63:1145-55. [Crossref] [PubMed]
- Min JK, Leipsic J, Pencina MJ, Berman DS, Koo BK, van Mieghem C, Erglis A, Lin FY, Dunning AM, Apruzzese P, Budoff MJ, Cole JH, Jaffer FA, Leon MB, Malpeso J, Mancini GB, Park SJ, Schwartz RS, Shaw LJ, Mauri L. Diagnostic accuracy of fractional flow reserve from anatomic CT angiography. JAMA 2012;308:1237-45. [Crossref] [PubMed]
- Nørgaard BL, Hjort J, Gaur S, Hansson N, Bøtker HE, Leipsic J, Mathiassen ON, Grove EL, Pedersen K, Christiansen EH, Kaltoft A, Gormsen LC, Mæng M, Terkelsen CJ, Kristensen SD, Krusell LR, Jensen JM. Clinical Use of Coronary CTA-Derived FFR for Decision-Making in Stable CAD. JACC Cardiovasc Imaging 2017;10:541-50. [Crossref] [PubMed]
- Douglas PS, Pontone G, Hlatky MA, Patel MR, Norgaard BL, Byrne RA, et al. Clinical outcomes of fractional flow reserve by computed tomographic angiography-guided diagnostic strategies vs. usual care in patients with suspected coronary artery disease: the prospective longitudinal trial of FFR(CT): outcome and resource impacts study. Eur Heart J 2015;36:3359-67. [Crossref] [PubMed]
- Douglas PS, De Bruyne B, Pontone G, Patel MR, Norgaard BL, Byrne RA, et al. 1-Year Outcomes of FFRCT-Guided Care in Patients With Suspected Coronary Disease: The PLATFORM Study. J Am Coll Cardiol 2016;68:435-45. [Crossref] [PubMed]
- Tesche C, Vliegenthart R, Duguay TM, De Cecco CN, Albrecht MH, De Santis D, Langenbach MC, Varga-Szemes A, Jacobs BE, Jochheim D, Baquet M, Bayer RR. Nd, Litwin SE, Hoffmann E, Steinberg DH, Schoepf UJ. Coronary Computed Tomographic Angiography-Derived Fractional Flow Reserve for Therapeutic Decision Making. Am J Cardiol 2017;120:2121-7. [Crossref] [PubMed]
- Collet C, Onuma Y, Andreini D, Sonck J, Pompilio G, Mushtaq S, et al. Coronary computed tomography angiography for heart team decision-making in multivessel coronary artery disease. Eur Heart J 2018;39:3689-98. [Crossref] [PubMed]
- Mickley H, Veien KT, Gerke O, Lambrechtsen J, Rohold A, Steffensen FH, Husic M, Akkan D, Busk M, Jessen LB, Jensen LO, Diederichsen A, Øvrehus KA. Diagnostic and Clinical Value of FFR(CT) in Stable Chest Pain Patients With Extensive Coronary Calcification: The FACC Study. JACC Cardiovasc Imaging 2022;15:1046-58. [Crossref] [PubMed]
- Khav N, Ihdayhid AR, Ko B. CT-Derived Fractional Flow Reserve (CT-FFR) in the Evaluation of Coronary Artery Disease. Heart Lung Circ 2020;29:1621-32. [Crossref] [PubMed]
- Dey D, Lin A. Machine-Learning CT-FFR and Extensive Coronary Calcium: Overcoming the Achilles Heel of Coronary Computed Tomography Angiography. JACC Cardiovasc Imaging 2020;13:771-3. [Crossref] [PubMed]
- Lin A, Kolossváry M, Motwani M, Išgum I, Maurovich-Horvat P, Slomka PJ, Dey D. Artificial intelligence in cardiovascular CT: Current status and future implications. J Cardiovasc Comput Tomogr 2021;15:462-9. [Crossref] [PubMed]
- Min JK, Koo BK, Erglis A, Doh JH, Daniels DV, Jegere S, Kim HS, Dunning A, Defrance T, Leipsic J. Effect of image quality on diagnostic accuracy of noninvasive fractional flow reserve: results from the prospective multicenter international DISCOVER-FLOW study. J Cardiovasc Comput Tomogr 2012;6:191-9. [Crossref] [PubMed]
- Ferencik M, Lu MT, Mayrhofer T, Puchner SB, Liu T, Maurovich-Horvat P, Ghemigian K, Ivanov A, Adami E, Nagurney JT, Woodard PK, Truong QA, Udelson JE, Hoffmann U. Non-invasive fractional flow reserve derived from coronary computed tomography angiography in patients with acute chest pain: Subgroup analysis of the ROMICAT II trial. J Cardiovasc Comput Tomogr 2019;13:196-202. [Crossref] [PubMed]
- Chen C, Song M. Visualizing a field of research: A methodology of systematic scientometric reviews. PLoS One 2019;14:e0223994. [Crossref] [PubMed]
- Chen C. CiteSpace II: Detecting and visualizing emerging trends and transient patterns in scientific literature. J Am Soc Inf Sci Technol 2006;57:359-77.
- Xie P. Study of international anticancer research trends via co-word and document co-citation visualization analysis. Scientometrics 2015;105:611-22.
- Freeman LC. Centrality in social networks: Conceptual clarification. Social Networks 1979;1:215-39.
- Chen C. Science mapping: a systematic review of the literature. Journal of Data and Information Science 2017;2:1-40.
- Ping Q, He J, Chen C. How many ways to use CiteSpace? A study of user interactive events over 14 months. J Assoc Inf Sci Technol 2017;68:1234-56.
- Chen C, Hu Z, Liu S, Tseng H. Emerging trends in regenerative medicine: a scientometric analysis in CiteSpace. Expert Opin Biol Ther 2012;12:593-608. [Crossref] [PubMed]
- Chen C. Searching for intellectual turning points: progressive knowledge domain visualization. Proc Natl Acad Sci U S A 2004;101:5303-10. [Crossref] [PubMed]
- Hirshfeld JW Jr, Nathan AS. QFR and FFR(CT): Accurate Enough? JACC Cardiovasc Interv 2019;12:2060-3. [Crossref] [PubMed]
- Itu L, Rapaka S, Passerini T, Georgescu B, Schwemmer C, Schoebinger M, Flohr T, Sharma P, Comaniciu D. A machine-learning approach for computation of fractional flow reserve from coronary computed tomography. J Appl Physiol (1985) 2016;121:42-52. [Crossref] [PubMed]
- Davies JE, Cook CM. Is Now the Time to Debate Traditional Fractional Flow Reserve/Instantaneous Wave-Free Ratio Cut Points? Circ Cardiovasc Interv 2018;11:e007562. [Crossref] [PubMed]
- Li Y, Gao Q, Chen N, Zhang Y, Wang J, Li C, He X, Jiao Y, Zhang Z. Clinical studies of magnetic resonance elastography from 1995 to 2021: Scientometric and visualization analysis based on CiteSpace. Quant Imaging Med Surg 2022;12:5080-100. [Crossref] [PubMed]
- Zhou W, Chen J, Huang Y. Co-Citation analysis and burst detection on financial bubbles with scientometrics approach. Economic Research-Ekonomska Istraživanja 2019;32:2310-28.
- Douglas PS, Hoffmann U, Patel MR, Mark DB, Al-Khalidi HR, Cavanaugh B, et al. Outcomes of anatomical versus functional testing for coronary artery disease. N Engl J Med 2015;372:1291-300. [Crossref] [PubMed]
- Lawton JS, Tamis-Holland JE, Bangalore S, Bates ER, Beckie TM, et al. 2021 ACC/AHA/SCAI Guideline for Coronary Artery Revascularization: Executive Summary: A Report of the American College of Cardiology/American Heart Association Joint Committee on Clinical Practice Guidelines. J Am Coll Cardiol 2022;79:197-215. [Crossref] [PubMed]
- Leiner T, Takx RAP. Predicting the Need for Revascularization in Stable Coronary Artery Disease: Protons or Photons? JACC Cardiovasc Imaging 2020;13:1005-7. [Crossref] [PubMed]
- Stuijfzand WJ, Danad I, Raijmakers PG, Marcu CB, Heymans MW, van Kuijk CC, van Rossum AC, Nieman K, Min JK, Leipsic J, van Royen N, Knaapen P. Additional value of transluminal attenuation gradient in CT angiography to predict hemodynamic significance of coronary artery stenosis. JACC Cardiovasc Imaging 2014;7:374-86. [Crossref] [PubMed]
- Tanigaki T, Emori H, Kawase Y, Kubo T, Omori H, Shiono Y, Sobue Y, Shimamura K, Hirata T, Matsuo Y, Ota H, Kitabata H, Okubo M, Ino Y, Matsuo H, Akasaka T. QFR Versus FFR Derived From Computed Tomography for Functional Assessment of Coronary Artery Stenosis. JACC Cardiovasc Interv 2019;12:2050-9. [Crossref] [PubMed]
- Michail M, Ihdayhid AR, Comella A, Thakur U, Cameron JD, McCormick LM, Gooley RP, Nicholls SJ, Mathur A, Hughes AD, Ko BS, Brown AJ. Feasibility and Validity of Computed Tomography-Derived Fractional Flow Reserve in Patients With Severe Aortic Stenosis: The CAST-FFR Study. Circ Cardiovasc Interv 2021;14:e009586. [Crossref] [PubMed]
- Di Jiang M, Zhang XL, Liu H, Tang CX, Li JH, Wang YN, et al. The effect of coronary calcification on diagnostic performance of machine learning-based CT-FFR: a Chinese multicenter study. Eur Radiol 2021;31:1482-93. [Crossref] [PubMed]
- Curzen N, Nicholas Z, Stuart B, Wilding S, Hill K, Shambrook J, et al. Fractional flow reserve derived from computed tomography coronary angiography in the assessment and management of stable chest pain: the FORECAST randomized trial. Eur Heart J 2021;42:3844-52. [Crossref] [PubMed]
- Nakazato R, Park HB, Berman DS, Gransar H, Koo BK, Erglis A, Lin FY, Dunning AM, Budoff MJ, Malpeso J, Leipsic J, Min JK. Noninvasive fractional flow reserve derived from computed tomography angiography for coronary lesions of intermediate stenosis severity: results from the DeFACTO study. Circ Cardiovasc Imaging 2013;6:881-9. [Crossref] [PubMed]
- Chinnaiyan KM, Safian RD, Gallagher ML, George J, Dixon SR, Bilolikar AN, Abbas AE, Shoukfeh M, Brodsky M, Stewart J, Cami E, Forst D, Timmis S, Crile J, Raff GL. Clinical Use of CT-Derived Fractional Flow Reserve in the Emergency Department. JACC Cardiovasc Imaging 2020;13:452-61. [Crossref] [PubMed]
- Zhao N, Gao Y, Xu B, Yang W, Song L, Jiang T, Xu L, Hu H, Li L, Chen W, Li D, Zhang F, Fan L, Lu B. Effect of Coronary Calcification Severity on Measurements and Diagnostic Performance of CT-FFR With Computational Fluid Dynamics: Results From CT-FFR CHINA Trial. Front Cardiovasc Med 2021;8:810625. [Crossref] [PubMed]
- Cook CM, Petraco R, Shun-Shin MJ, Ahmad Y, Nijjer S, Al-Lamee R, Kikuta Y, Shiono Y, Mayet J, Francis DP, Sen S, Davies JE. Diagnostic Accuracy of Computed Tomography-Derived Fractional Flow Reserve: A Systematic Review. JAMA Cardiol 2017;2:803-10. [Crossref] [PubMed]
- Pontone G, Weir-McCall JR, Baggiano A, Del Torto A, Fusini L, Guglielmo M, et al. Determinants of Rejection Rate for Coronary CT Angiography Fractional Flow Reserve Analysis. Radiology 2019;292:597-605. [Crossref] [PubMed]
- Nørgaard BL, Gaur S, Fairbairn TA, Douglas PS, Jensen JM, Patel MR, Ihdayhid AR, Ko BSH, Sellers SL, Weir-McCall J, Matsuo H, Sand NPR, Øvrehus KA, Rogers C, Mullen S, Nieman K, Parner E, Leipsic J, Abdulla J. Prognostic value of coronary computed tomography angiographic derived fractional flow reserve: a systematic review and meta-analysis. Heart 2022;108:194-202. [Crossref] [PubMed]
- Hlatky MA, De Bruyne B, Pontone G, Patel MR, Norgaard BL, Byrne RA, et al. Quality-of-Life and Economic Outcomes of Assessing Fractional Flow Reserve With Computed Tomography Angiography: PLATFORM. J Am Coll Cardiol 2015;66:2315-23. [Crossref] [PubMed]
- Patel MR, Nørgaard BL, Fairbairn TA, Nieman K, Akasaka T, Berman DS, et al. 1-Year Impact on Medical Practice and Clinical Outcomes of FFR(CT): The ADVANCE Registry. JACC Cardiovasc Imaging 2020;13:97-105. [Crossref] [PubMed]
- Ihdayhid AR, Norgaard BL, Gaur S, Leipsic J, Nerlekar N, Osawa K, Miyoshi T, Jensen JM, Kimura T, Shiomi H, Erglis A, Jegere S, Oldroyd KG, Botker HE, Seneviratne SK, Achenbach S, Ko BS. Prognostic Value and Risk Continuum of Noninvasive Fractional Flow Reserve Derived from Coronary CT Angiography. Radiology 2019;292:343-51. [Crossref] [PubMed]
- Kawashima H, Pompilio G, Andreini D, Bartorelli AL, Mushtaq S, Ferrari E, et al. Safety and feasibility evaluation of planning and execution of surgical revascularisation solely based on coronary CTA and FFR(CT) in patients with complex coronary artery disease: study protocol of the FASTTRACK CABG study. BMJ Open 2020;10:e038152. [Crossref] [PubMed]
- Shiono Y, Matsuo H, Kawasaki T, Amano T, Kitabata H, Kubo T, et al. Clinical Impact of Coronary Computed Tomography Angiography-Derived Fractional Flow Reserve on Japanese Population in the ADVANCE Registry. Circ J 2019;83:1293-301. [Crossref] [PubMed]
- Rossi A, Merkus D, Klotz E, Mollet N, de Feyter PJ, Krestin GP. Stress myocardial perfusion: imaging with multidetector CT. Radiology 2014;270:25-46. [Crossref] [PubMed]
- Pontone G, Baggiano A, Andreini D, Guaricci AI, Guglielmo M, Muscogiuri G, et al. Dynamic Stress Computed Tomography Perfusion With a Whole-Heart Coverage Scanner in Addition to Coronary Computed Tomography Angiography and Fractional Flow Reserve Computed Tomography Derived. JACC Cardiovasc Imaging 2019;12:2460-71. [Crossref] [PubMed]
- Danad I, Szymonifka J, Twisk JWR, Norgaard BL, Zarins CK, Knaapen P, Min JK. Diagnostic performance of cardiac imaging methods to diagnose ischaemia-causing coronary artery disease when directly compared with fractional flow reserve as a reference standard: a meta-analysis. Eur Heart J 2017;38:991-8. [Crossref] [PubMed]
- Yoon YE, Choi JH, Kim JH, Park KW, Doh JH, Kim YJ, Koo BK, Min JK, Erglis A, Gwon HC, Choe YH, Choi DJ, Kim HS, Oh BH, Park YB. Noninvasive diagnosis of ischemia-causing coronary stenosis using CT angiography: diagnostic value of transluminal attenuation gradient and fractional flow reserve computed from coronary CT angiography compared to invasively measured fractional flow reserve. JACC Cardiovasc Imaging 2012;5:1088-96. [Crossref] [PubMed]
- Coenen A, Kim YH, Kruk M, Tesche C, De Geer J, Kurata A, Lubbers ML, Daemen J, Itu L, Rapaka S, Sharma P, Schwemmer C, Persson A, Schoepf UJ, Kepka C, Hyun Yang D, Nieman K. Diagnostic Accuracy of a Machine-Learning Approach to Coronary Computed Tomographic Angiography-Based Fractional Flow Reserve: Result From the MACHINE Consortium. Circ Cardiovasc Imaging 2018;11:e007217. [Crossref] [PubMed]
- Nicol ED, Norgaard BL, Blanke P, Ahmadi A, Weir-McCall J, Horvat PM, Han K, Bax JJ, Leipsic J. The Future of Cardiovascular Computed Tomography: Advanced Analytics and Clinical Insights. JACC Cardiovasc Imaging 2019;12:1058-72. [Crossref] [PubMed]
- Lee JM, Choi G, Koo BK, Hwang D, Park J, Zhang J, et al. Identification of High-Risk Plaques Destined to Cause Acute Coronary Syndrome Using Coronary Computed Tomographic Angiography and Computational Fluid Dynamics. JACC Cardiovasc Imaging 2019;12:1032-43. [Crossref] [PubMed]