Optimization and validation of voxel size-related radiomics variability by combatting batch effect harmonization in pulmonary nodules: a phantom and clinical study
Introduction
The recent rapid development of machine learning technology and computing power has enabled researchers to use big data of radiomics for precise clinical diagnosis (1). Radiomics refers to the high-throughput extraction of a large number of radiological image features of lesions, that is, the conversion of image information into digital information (2,3). Various studies have shown that the radiomics models have high diagnostic efficacy for pulmonary nodules in terms of differential diagnosis, nodule typing, preoperative prediction, or prognostic analysis (4-7). Lung cancer is one of the most serious diseases in the world, with a 5-year survival rate of around only 19% (8). The most common pathological subtype is lung adenocarcinoma (LUAD). However, it has been reported that the 5-year survival rate after complete surgery for preinvasive LUAD is close to 100% (9). Accurate preoperative diagnosis of invasive LUAD is conducive to clinical decision-making, such as in surgical determination. However, the selected features and radiomics models in previous studies have been inconsistent and with poor reproducibility, which has limited their application in real-world medical practice (10,11).
Advances in computed tomography (CT) technology has revolutionized diagnostic imaging, enabling consistent increases in image quality alongside the decrease of voxel size. Several studies have shown that ultra-high-resolution CT (U-HRCT, including 1,024×1,024, and 2,048×2,048 matrix size) scans can improve the image quality and the assessment of lung diseases in comparison with the 512×512 matrix size (12,13). Several recent studies on the effect of voxel size in the variability of radiomics have achieved inconsistent results. Paul et al. and Yang et al. found that voxel size strongly affected the reproducibility of the radiomics features (14,15), but Crandall et al. reported that the voxel size had a minimal effect in feature value (16).
Shafiq-Ul-Hassan et al. optimized the stability of radiomics features using image resampling (17). Image upsampling (increasing the voxel size) was generally used to explore whether it can reduce the radiomics variability, but it was shown that it could result in loss of valuable image information and affect the clinical diagnostic performance. Batch effects, which conceal biological signals and lead to deviations in subsequent data analysis (18), can be generated by either different experimental data sources or data collection processes of images with different voxel sizes. Although the combatting batch effect (ComBat) harmonization can reduce these radiomics variabilities (19,20), few relevant studies have been conducted to date.
In this study, we aimed to evaluate the ability of the ComBat harmonization to reduce variability of voxel size-related radiomics in the differential diagnosis of pulmonary nodules in both pulmonary phantom and actual patients.
Methods
This study was conducted in accordance with the Declaration of Helsinki (as revised in 2013). The study was approved by institutional ethics review boards of Shanghai Public Health Clinical Center, Shanghai Zhongshan Hospital, Beilun Second People’s Hospital, and Shanghai Changzheng Hospital (No. SK2020-174) and the requirement for individual consent for this retrospective analysis was waived.
Phantom image acquisition
The anthropomorphic thorax phantom simulating an intermediate-sized adult (PH-1; Kyoto Kagaku, Kyoto, Japan) was used in this study (https://www.kyotokagaku.com/lineup/). There were 22 artificial nodules embedded in the artificial vascular bundle in the chest phantom: (I) 1 irregular nodule 15 mm in diameter; (II) 7 concentric mixed ground glass nodules (mGGNs) of different diameters (15 and 20 mm) with different spherical solid components (3, 5, 7, 9 mm); (III) 2 double eccentric mGGN 20 mm in diameter with different spherical solid components (3, 5, 7 mm); (IV) 12 pure nodules, including 4 pure solid nodules and 8 pure ground glass nodules (pGGNs) (details in Figure S1).
All data acquisitions were performed on a Philips Brilliance 16 slice CT scanner (Philips, Amsterdam, Netherlands). Scanning parameters were divided into two parts based on different voxel sizes: the conventional scanning with 512×512 matrix and the target scanning with 1,024×1,024 matrix. The voxel sizes of phantom data were 0.68×0.68×2.00 and 0.18×0.18×1.00 mm3, respectively (details in Table S1).
Clinical image acquisition
In this study, a total of 303 patients with pulmonary nodules were retrospectively recruited from June 1, 2017 to May 30, 2020 at Beilun Second People’s Hospital. The patients were identified according to the recruitment pathway (Figure 1). Finally, a total of 134 patients (46 males and 88 females, age 59.46±10.48 years) who met the inclusion criteria were enrolled in the study (Table 1).
Table 1
Characteristics | 512×512 matrix (n=134) | 1,024×1,024 matrix (n=134) | P value |
---|---|---|---|
Age (years) | 59.46±10.48 | – | |
Gender (F/M) | 88/46 | – | |
Nodule type | |||
pGGN | 54 (40.30) | – | |
mGGN | 75 (55.97) | – | |
Solid nodule | 5 (3.73) | – | |
Maximum diameter of nodule (mm) | 12.79±5.55 | 13.06±5.54 | 0.642 |
Minimum diameter of nodule (mm) | 9.89±3.99 | 10.06±4.07 | 0.766 |
Mean diameter of nodule (mm) | 11.34±4.53 | 11.56±4.59 | 0.676 |
Data are presented as mean ± standard deviation, n, or n (%). CT, computed tomography; F, female; M, male; pGGN, pure ground glass nodule; mGGN, mixed ground glass nodule.
As for the lung phantom, all images of patients were performed on the Philips Brilliance 16 slice CT scanner. The scanning parameters were also the same as those of the phantom data: conventional and target scanning. However, the patients were not retested because of the radiation dose. The voxel sizes of clinical data were 0.62×0.62×2.00–0.77×0.77×2.00 and 0.15×0.15×1.00–0.23×0.23×1.00 mm3, respectively (details in Table S1). A flow diagram of this study is shown in Figure 2.
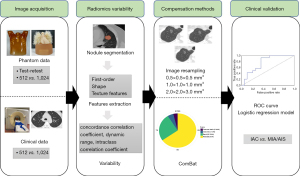
Region of interest segmentation and radiomics features extraction
Deep learning-based pulmonary nodule segmentation (both in phantom and clinical applications) was performed using the uAI Research Portal software (United Imaging Intelligence Inc., Shanghai, China) that was embedded into the widely used package-PyRadiomics (https://pyradiomics.readthedocs.io/en/latest/index.html). All segmented three-dimensional (3D) nodules were reviewed by two chest radiologists (Y Zhuo and Y Zhan) and manually adjusted if necessary. The uAI Research Portal software was used to extract radiomics features, including first-order statistics, shape, and texture features. A total of 14 filters were used during the process of features extraction (details in Appendix 1). Finally, a total of 2,600 radiomic features were extracted for each 3D nodule.
ComBat compensation method
To reduce radiomics variability from batch effects, the ComBat compensation method was performed on the BatchServer (https://lifeinfo.shinyapps.io/batchserver/) (21). Principal variance component analysis (PVCA) fits a mixed linear model to estimate the variation ratio of each factor, and was thus used to evaluate the effectiveness of batch effect correction. The emerging nonlinear dimensionality reduction method, uniform manifold approximation and projection (UMAP), was also used to evaluate the effectiveness of batch effect correction in this study.
Image resampling
The original phantom and clinical images had different voxel sizes, 0.15×0.15×1.00–0.77×0.77×2.00 mm3. To reduce voxel size-related radiomics variability, all images were resampled for 3 different times: 0.5×0.5×0.5, 1.0×1.0×1.0, and 2.0×2.0×3.0 mm3, which included not only the conventional upsampling but also the downsampling so as to preserve as much image information as possible.
Radiomics variability and correction analysis
In the phantom application, test-retest robustness was calculated on concordance correlation coefficient (CCC) and dynamic range (DR) (22-24). Radiomics features with good reproducibility were defined when CCC and DR ≥0.90 (22).
Both in phantom and clinical applications, radiomics variability was identified by intraclass correlation coefficient (ICC) between different voxel sizes. ICC was divided into 3 levels: ICC ≥0.75, 0.40≤ ICC <0.75, and ICC <0.40 (25,26). The radiomics features were defined as excellent, moderate, and poor based on CCC, DR, and ICC (Table 2).
Table 2
Type | Phantom data | Clinical data |
---|---|---|
Excellent | CCC ≥0.90 and DR ≥0.90 and ICC ≥0.75 | ICC ≥0.75 |
Moderate | CCC ≥0.90 and DR ≥0.90 and 0.40≤ ICC <0.75 | 0.40≤ ICC <0.75 |
Poor | CCC <0.90 or DR <0.90 or ICC <0.40 | ICC <0.40 |
CCC, concordance correlation coefficient; DR, dynamic range; ICC, intraclass correlation coefficient.
Clinical evaluation
The clinical evaluation dataset was enrolled from July 1, 2018 to June 30, 2020 at Beilun Second People’s Hospital and Shanghai Changzheng Hospital. Some studies suggested that sublobectomy could be an alternative approach for LUAD of no more than 2 cm in diameter (27-29). A clinical evaluation dataset with 512×512 matrix containing 186 lung nodules (pathologically confirmed LUAD) with a mean diameter of less than 2 cm was used in this study, including 88 invasive adenocarcinoma (IAC) and 98 adenocarcinoma in situ (AIS) and minimally invasive adenocarcinoma (MIA) (AIS-MIA group). In the MIA-AIS group, there were 73 cases of MIA and 25 of AIS (Table S2).
The radiomics features of all lung nodules were extracted and a logistic regression model was built after radiomics features selection. The models were also built with the excellent features after ComBat and image resampling correction. The receiver operating characteristic (ROC) curves were used to evaluate the performance of radiomics signature models.
Statistical analysis
The Wilcoxon rank-sum test was used for continuous variables between the two groups, and the categorical variables were compared with χ2 test. The SPSS software (version 20; IBM Corp., Armonk, NY, USA) was used to perform all statistical analysis. Statistical significance was indicated by a two-tailed P value of less than 0.05. Measurement data were expressed as mean ± standard deviation (SD).
We used two feature selection methods, max-relevance and min-redundancy (mRMR) and least absolute shrinkage and selection operator (LASSO), to select radiomics features. The LASSO method constructed a penalty function by adding constraint conditions, and a prediction model was constructed by performing 10-fold cross-validation. DeLong’s test was used between different ROC curves. Heml software (http://hemi.biocuckoo.org) was used to make a heatmap to visually show the radiomics variability. Hierarchical clustering analysis was used to evaluate the redundancy of CT radiomics features.
Results
Information of phantom and clinical data
The 22 artificial nodules of phantom included 8 pGGNs (36.36%), 9 mGGNs (40.91%), and 5 solid nodules (22.73%), of which 21 were spherical and 1 was irregular in shape.
The clinical and CT characteristics of 134 patients are shown in Table 1 and Figure S2, including 54 pGGNs (40.30%), 75 mGGNs (55.97%), and 5 solid nodules (3.73%).
Voxel size-related radiomics variability
In phantom application, among 2,600 features, 1,788 (68.77%) radiomics features had both CCC and DR ≥0.90. According to ICC value, there were 679 (26.12%) excellent, 325 (12.50%) moderate, and 1,596 (61.38%) poor features. Details are displayed in Table 3 and Figure 3.
Table 3
Data | Type | Before optimization | Image resampling (mm3) | ComBat | ||
---|---|---|---|---|---|---|
0.5×0.5×0.5 | 1.0×1.0×1.0 | 2.0×2.0×3.0 | ||||
Phantom data | Excellent | 679 (26.12) | 1,655 (63.65) | 1,558 (59.92) | 1,655 (63.65) | 1,085 (41.73) |
Moderate | 325 (12.50) | 502 (19.31) | 661 (25.43) | 654 (25.16) | 693 (26.65) | |
Poor | 1,596 (61.38) | 443 (17.04) | 381 (14.65) | 291 (11.19) | 822 (31.62) | |
Clinical data | Excellent | 840 (32.31) | 1,394 (53.62) | 1,519 (58.42) | 1,251 (48.12) | 2,365 (90.96) |
Moderate | 384 (14.77) | 637 (24.50) | 745 (28.66) | 1,116 (42.92) | 106 (4.08) | |
Poor | 1,321 (50.81) | 569 (21.88) | 336 (12.92) | 233 (8.96) | 129 (4.96) |
Data are presented as n (%). ComBat, combatting batch effect.
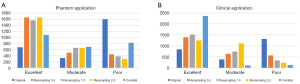
In clinical application, there were 840 (32.31%) excellent features, 384 (14.77%) moderate features, and 1,321 (50.81%) poor features according to ICC value (details in Table 3 and Figure 3).
ComBat compensation
The PVCA and UMAP analyses showed that ComBat optimization reduced batch effects from 46.39% to 14.90% in the phantom and from 25.76% to 14.17% in clinical application (Figure 4 and Figure S3).
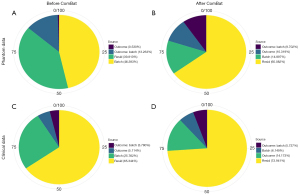
CT scanning on the phantom displayed 1,085 (41.73%) excellent, 693 (26.65%) moderate, and 822 (31.62%) poor features in contrast to 2,365 (90.96%) excellent, 106 (4.08%) moderate, and 129 (4.96%) poor features in patients based on ICC value after ComBat correction (Table 3 and Figure S4).
Image resampling
The CT resampling images of the phantom and clinical images are shown in Figure 5. The 3 different image resampling sizes (0.5×0.5×0.5, 1.0×1.0×1.0, and 2.0×2.0×3.0 mm3) in the phantom showed that the numbers of features with CCC and DR ≥0.90 were 2,358 (90.69%), 2,298 (88.38%), and 2,351 (90.42%), respectively, including 1,655 (63.65%) excellent, 502 (19.31%) moderate, and 443 (17.04%) poor features based on ICC value after image resampling of 0.5×0.5×0.5 mm3. Among these features, the image resampling of 1.0×1.0×1.0 mm3 displayed 1,558 (59.92%) excellent, 661 (25.43%) moderate, and 381 (14.65%) poor features in contrast to 1,655 (63.65%) excellent, 654 (25.16%) moderate, and 291 (11.19%) poor features image resampling of 2.0×2.0×3.0 mm3 based on ICC value (Table 3 and Figure S5).
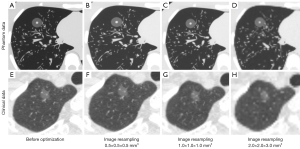
In clinical application, there were 1,394 (53.62%) excellent, 637 (24.50%) moderate, and 569 (21.88%) poor features after image resampling of 0.5×0.5×0.5 mm3, in contrast to 1,519 (58.42%) excellent, 745 (28.66%) moderate, and 336 (12.92%) poor features in image resampling of 1.0×1.0×1.0 mm3, and 1,251 (48.12%) excellent, 1,116 (42.92%) moderate, and 233 (8.96%) poor features in image resampling of 2.0×2.0×2.0 mm3 based on ICC value (Table 3 and Figure S6).
Redundancy of CT radiomics features
For excellent radiomics features, the de-redundancy hierarchical clustering analysis divided the original features into 10 groups in contrast to 15 groups by image resampling and ComBat compensation methods, respectively. The heatmap and hierarchical clustering analysis also showed that the first-order, shape, and texture features were 208 (30.63%), 275 (40.50%), and 196 (28.87%), respectively, among 679 excellent features in phantom data in contrast to 391 (46.55%), 250 (29.76%), and 199 (23.69%), respectively, among 840 excellent features in clinical data before optimization (Figure 6).
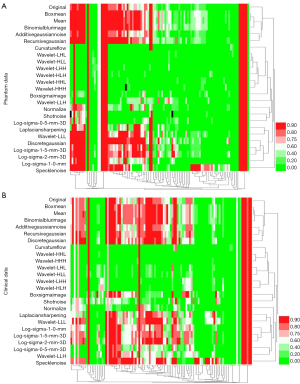
Clinical evaluation
A logistic regression model of pre-optimization was built using 11 selected radiomics features with non-zero coefficients; the area under the curve (AUC) of the training set was 0.998 (accuracy, 0.983; sensitivity, 0.984; specificity, 0.983), whereas that of testing set was 0.763 (accuracy, 0.545; sensitivity, 1.000; specificity, 0.211).
A logistic regression model of ComBat correction was built using 5 selected radiomics features with non-zero coefficients; the AUC of the training set was 0.997 (accuracy, 0.977; sensitivity, 0.976; specificity, 0.977), whereas that of testing set was 0.865 (accuracy, 0.736; sensitivity, 0.929; specificity, 0.630). The optimization effect of the ComBat compensation method was significantly better than image resampling correction of 0.5×0.5×0.5, 1.0×1.0×1.0, and 2.0×2.0×2.0 mm3 (P=0.012, 0.017, and 0.034, respectively; details in Table 4 and Figure S7).
Table 4
ROC | Before optimization | ComBat | Resampling 0.5 | Resampling 1.0 | Resampling 2.0 |
---|---|---|---|---|---|
Training set | |||||
AUC | 0.998 | 0.997 | 0.978 | 0.989 | 0.991 |
Accuracy | 0.983 | 0.977 | 0.930 | 0.947 | 0.953 |
Sensitivity | 0.984 | 0.976 | 0.929 | 0.960 | 0.960 |
Specificity | 0.983 | 0.977 | 0.897 | 0.937 | 0.949 |
Testing set | |||||
AUC | 0.763 | 0.865 | 0.711 | 0.778 | 0.812 |
Accuracy | 0.545 | 0.736 | 0.485 | 0.576 | 0.576 |
Sensitivity | 1.000 | 0.929 | 0.897 | 0.929 | 1.000 |
Specificity | 0.211 | 0.630 | 0.158 | 0.316 | 0.263 |
Resampling 0.5, image resampling of 0.5×0.5×0.5 mm3; resampling 1.0, image resampling of 1.0×1.0×1.0 mm3; resampling 2.0, image resampling of 2.0×2.0×3.0 mm3. ROC, receiver operating characteristic; ComBat, combatting batch effect; AUC, area under the curve.
Discussion
Our study demonstrated that the voxel size affects the variability of radiomics features of phantom and clinical applications, which can be significantly improved by ComBat and image resampling correction. The image resampling was superior to ComBat compensation in phantom application, whereas ComBat compensation was significantly better than image resampling in clinical application. In addition, the hierarchical clustering analysis showed that the first-order and shape features of the original images had better robustness than those of texture features with different filters.
In this study, scanning parameters were divided into two parts based on different voxel sizes: the conventional scanning with 512×512 matrix, and the target scanning with 1,024×1,024 matrix that is a local scanning for nodules with the advantage of resolution improvement to provide more detailed features and simplify qualitative diagnosis, as indicated by previous reports about U-HRCT imaging (12,13).
Radiomics can provide a large amount of information to help clinical decision-making, but variability is a hinderance to its clinical application requiring urgent rectification. The variability in CT scans is mainly due to large differences in CT scan parameters, particularly the differences in voxel size. In our study, more than half of the features were poor after changing the voxel size both in phantom and clinical applications. Yang et al. found that voxel size strongly affected the robustness of positron emission tomography/magnetic resonance (PET/MR) radiomics features, and that 0.5×0.5×1.0 mm3 of voxel size was optimal for features in the primary tumor of nasopharyngeal carcinoma (15). The variability of the voxel size can be obtained by analyzing the pixel size and slice spacing. Paul et al. found that most deep features changed with the pixel size, which was the same for many traditional radiomics features (14). Our findings were consistent with those of the above reports. However, Crandall et al. found that the reproducibility of most PET/CT features was barely affected by changes in voxel size in cervical cancer (16).
Both image resampling and the ComBat harmonization could optimize voxel size-related CT radiomics variability in our study, but the diagnostic performance of the model with ComBat compensation method was significantly better than that of models with image resampling correction and model without optimization in the testing set (P<0.05) in clinical evaluation. The resampling method was traditionally considered to resolve the source of variation caused by different voxel sizes. Shafiq-Ul-Hassan et al. found that image resampling was an appropriate preprocessing step to obtain more reproducible CT radiomics features (17,30). Another study had shown that image resampling accompanied by Butterworth low pass filtering could effectively reduce the variability due to different voxel sizes (31).
The ComBat method was derived from genomics research, and the intended function was to reduce batch effects of data collection processes (32). Orlhac et al. compared the radiomics feature distributions before and after application of the ComBat compensation method and showed that the cluster distribution of each texture feature corresponding to different imaging parameters was corrected after fitting (20). Orlhac et al. also used the ComBat method to effectively adjust the PET radiomics feature distributions under 3 different imaging parameter settings, making multi-center joint research possible (19). Ligero et al. explored resampling, ComBat, and singular value decomposition (SVD) compensation methods for reducing CT radiomics variability, and found that ComBat showed the highest improvement of feature classification (33). In our study, not only the AUC but also the sensitivity and specificity of the ComBat model were higher than those of other models after ComBat optimization, because the radiomics features with small variability were selected after ComBat optimization, and the established model was more stable and more universal. The ComBat optimization method can not only remove the batch effect caused by different voxels, but also remove the batch effect caused by other factors, such as CT vendors (33). This might be one of the reasons for ComBat compensation performing significantly better than image resampling in our clinical application.
Our hierarchical clustering analysis showed that the first-order and shape features of the original images had better robustness than those of texture features with different filters, which was consistent with previous reports (34,35). A systematic review showed that first-order CT features had higher repeatability and reproducibility than shape and texture features according to 41 full-text articles (34). Tunali et al. found that statistical, histogram, and a subset of texture features tended to be stable in peritumoral regions of lung cancer lesions (35). The first-order feature describes the distribution of individual voxel values, regardless of the spatial position relationship, whereas the texture features describe the relationship between voxels and regional contrast, which can be used to assess tumor heterogeneity. The high-level features emphasize the use of filters on the image processing and features extraction. Therefore, in comparison with high-order features and texture, the first-order features obtained from the histogram are more stable.
There were several limitations to this study. First, this study investigated the variability of voxel size-related CT radiomics features, but there would be other variables in the research process, such as the error of nodule segmentation, which could also affect the results. Second, we took voxel size as a whole, and did not conduct a more detailed study of pixel size and slice spacing. Third, different CT vendors and scanning parameters will result in more batch effects in radiomics analysis, which can be reduced by ComBat (33); multi-center validations are needed to evaluate the ability of ComBat harmonization. In addition, there were statistical differences between the gender, age, and nodule type of patients in the IAC and MIA-AIS groups in clinical evaluation. Although these factors may have certain impacts on the results, the data collected in a continuous period of time could better reflect the actual clinical problem.
In conclusion, the ComBat harmonization can optimize voxel size-related variability of CT radiomics both in phantom and clinical application, and the diagnostic efficiency and generalization of the optimized features are improved based on clinical evaluation. According to the results of this prospective multicenter large-scale study in which the variability of the scanning parameter was a major limitation, the ComBat method is easy to apply and endows the potential of radiomics.
Acknowledgments
Funding: This work was supported by
Footnote
Conflicts of Interest: All authors have completed the ICMJE uniform disclosure form (available at https://qims.amegroups.com/article/view/10.21037/qims-22-992/coif). The authors have no conflicts of interest to declare.
Ethical Statement: The authors are accountable for all aspects of the work in ensuring that questions related to the accuracy or integrity of any part of the work are appropriately investigated and resolved. The study was conducted in accordance with the Declaration of Helsinki (as revised in 2013). The study was approved by the institutional ethics review boards of Shanghai Public Health Clinical Center, Shanghai Zhongshan Hospital, Beilun Second People’s Hospital and Shanghai Changzheng Hospital (No. SK2020-174) and the requirement for individual consent for this retrospective analysis was waived.
Open Access Statement: This is an Open Access article distributed in accordance with the Creative Commons Attribution-NonCommercial-NoDerivs 4.0 International License (CC BY-NC-ND 4.0), which permits the non-commercial replication and distribution of the article with the strict proviso that no changes or edits are made and the original work is properly cited (including links to both the formal publication through the relevant DOI and the license). See: https://creativecommons.org/licenses/by-nc-nd/4.0/.
References
- Obermeyer Z, Emanuel EJ. Predicting the Future - Big Data, Machine Learning, and Clinical Medicine. N Engl J Med 2016;375:1216-9. [Crossref] [PubMed]
- Lee G, Lee HY, Park H, Schiebler ML, van Beek EJR, Ohno Y, Seo JB, Leung A. Radiomics and its emerging role in lung cancer research, imaging biomarkers and clinical management: State of the art. Eur J Radiol 2017;86:297-307. [Crossref] [PubMed]
- Rizzo S, Botta F, Raimondi S, Origgi D, Fanciullo C, Morganti AG, Bellomi M. Radiomics: the facts and the challenges of image analysis. Eur Radiol Exp 2018;2:36. [Crossref] [PubMed]
- Fan L, Fang M, Li Z, Tu W, Wang S, Chen W, Tian J, Dong D, Liu S. Radiomics signature: a biomarker for the preoperative discrimination of lung invasive adenocarcinoma manifesting as a ground-glass nodule. Eur Radiol 2019;29:889-97. [Crossref] [PubMed]
- She Y, Zhang L, Zhu H, Dai C, Xie D, Xie H, Zhang W, Zhao L, Zou L, Fei K, Sun X, Chen C. The predictive value of CT-based radiomics in differentiating indolent from invasive lung adenocarcinoma in patients with pulmonary nodules. Eur Radiol 2018;28:5121-8. [Crossref] [PubMed]
- Wu G, Woodruff HC, Sanduleanu S, Refaee T, Jochems A, Leijenaar R, Gietema H, Shen J, Wang R, Xiong J, Bian J, Wu J, Lambin P. Preoperative CT-based radiomics combined with intraoperative frozen section is predictive of invasive adenocarcinoma in pulmonary nodules: a multicenter study. Eur Radiol 2020;30:2680-91. [Crossref] [PubMed]
- Zhao W, Xu Y, Yang Z, Sun Y, Li C, Jin L, Gao P, He W, Wang P, Shi H, Hua Y, Li M. Development and validation of a radiomics nomogram for identifying invasiveness of pulmonary adenocarcinomas appearing as subcentimeter ground-glass opacity nodules. Eur J Radiol 2019;112:161-8. [Crossref] [PubMed]
- Siegel RL, Miller KD, Jemal A. Cancer statistics, 2020. CA Cancer J Clin 2020;70:7-30. [Crossref] [PubMed]
- Zhang Y, Ma X, Shen X, Wang S, Li Y, Hu H, Chen H. Surgery for pre- and minimally invasive lung adenocarcinoma. J Thorac Cardiovasc Surg 2022;163:456-64. [Crossref] [PubMed]
- Lee SH, Cho HH, Lee HY, Park H. Clinical impact of variability on CT radiomics and suggestions for suitable feature selection: a focus on lung cancer. Cancer Imaging 2019;19:54. [Crossref] [PubMed]
- Mackin D, Fave X, Zhang L, Fried D, Yang J, Taylor B, Rodriguez-Rivera E, Dodge C, Jones AK, Court L. Measuring Computed Tomography Scanner Variability of Radiomics Features. Invest Radiol 2015;50:757-65. [Crossref] [PubMed]
- Hata A, Yanagawa M, Honda O, Kikuchi N, Miyata T, Tsukagoshi S, Uranishi A, Tomiyama N. Effect of Matrix Size on the Image Quality of Ultra-high-resolution CT of the Lung: Comparison of 512 × 512, 1024 × 1024, and 2048 × 2048. Acad Radiol 2018;25:869-76. [Crossref] [PubMed]
- Yanagawa M, Tsubamoto M, Satoh Y, Hata A, Miyata T, Yoshida Y, Kikuchi N, Kurakami H, Tomiyama N. Lung Adenocarcinoma at CT with 0.25-mm Section Thickness and a 2048 Matrix: High-Spatial-Resolution Imaging for Predicting Invasiveness. Radiology 2020;297:462-71. [Crossref] [PubMed]
- Paul R, Shafiq-Ul Hassan M, Moros EG, Gillies RJ, Hall LO, Goldgof DB. Deep Feature Stability Analysis Using CT Images of a Physical Phantom Across Scanner Manufacturers, Cartridges, Pixel Sizes, and Slice Thickness. Tomography 2020;6:250-60. [Crossref] [PubMed]
- Yang P, Xu L, Cao Z, Wan Y, Xue Y, Jiang Y, Yen E, Luo C, Wang J, Rong Y, Niu T. Extracting and Selecting Robust Radiomic Features from PET/MR Images in Nasopharyngeal Carcinoma. Mol Imaging Biol 2020;22:1581-91. [Crossref] [PubMed]
- Crandall JP, Fraum TJ, Lee M, Jiang L, Grigsby P, Wahl RL. Repeatability of (18)F-FDG PET Radiomic Features in Cervical Cancer. J Nucl Med 2021;62:707-15. [Crossref] [PubMed]
- Shafiq-Ul-Hassan M, Zhang GG, Latifi K, Ullah G, Hunt DC, Balagurunathan Y, Abdalah MA, Schabath MB, Goldgof DG, Mackin D, Court LE, Gillies RJ, Moros EG. Intrinsic dependencies of CT radiomic features on voxel size and number of gray levels. Med Phys 2017;44:1050-62. [Crossref] [PubMed]
- Lazar C, Meganck S, Taminau J, Steenhoff D, Coletta A, Molter C, Weiss-Solís DY, Duque R, Bersini H, Nowé A. Batch effect removal methods for microarray gene expression data integration: a survey. Brief Bioinform 2013;14:469-90. [Crossref] [PubMed]
- Orlhac F, Boughdad S, Philippe C, Stalla-Bourdillon H, Nioche C, Champion L, Soussan M, Frouin F, Frouin V, Buvat I. A Postreconstruction Harmonization Method for Multicenter Radiomic Studies in PET. J Nucl Med 2018;59:1321-8. [Crossref] [PubMed]
- Orlhac F, Frouin F, Nioche C, Ayache N, Buvat I. Validation of A Method to Compensate Multicenter Effects Affecting CT Radiomics. Radiology 2019;291:53-9. [Crossref] [PubMed]
- Zhu T, Sun R, Zhang F, Chen GB, Yi X, Ruan G, Yuan C, Zhou S, Guo T. BatchServer: A Web Server for Batch Effect Evaluation, Visualization, and Correction. J Proteome Res 2021;20:1079-86. [Crossref] [PubMed]
- Balagurunathan Y, Kumar V, Gu Y, Kim J, Wang H, Liu Y, Goldgof DB, Hall LO, Korn R, Zhao B, Schwartz LH, Basu S, Eschrich S, Gatenby RA, Gillies RJ. Test-retest reproducibility analysis of lung CT image features. J Digit Imaging 2014;27:805-23. [Crossref] [PubMed]
- Schuster DP. The opportunities and challenges of developing imaging biomarkers to study lung function and disease. Am J Respir Crit Care Med 2007;176:224-30. [Crossref] [PubMed]
- Zhao B, Tan Y, Tsai WY, Qi J, Xie C, Lu L, Schwartz LH. Reproducibility of radiomics for deciphering tumor phenotype with imaging. Sci Rep 2016;6:23428. [Crossref] [PubMed]
- Khan JN, Singh A, Nazir SA, Kanagala P, Gershlick AH, McCann GP. Comparison of cardiovascular magnetic resonance feature tracking and tagging for the assessment of left ventricular systolic strain in acute myocardial infarction. Eur J Radiol 2015;84:840-8. [Crossref] [PubMed]
- Schmidt B, Dick A, Treutlein M, Schiller P, Bunck AC, Maintz D, Baeßler B. Intra- and inter-observer reproducibility of global and regional magnetic resonance feature tracking derived strain parameters of the left and right ventricle. Eur J Radiol 2017;89:97-105. [Crossref] [PubMed]
- Nakamura K, Saji H, Nakajima R, Okada M, Asamura H, Shibata T, Nakamura S, Tada H, Tsuboi M. A phase III randomized trial of lobectomy versus limited resection for small-sized peripheral non-small cell lung cancer (JCOG0802/WJOG4607L). Jpn J Clin Oncol 2010;40:271-4. [Crossref] [PubMed]
- Stamatis G, Leschber G, Schwarz B, Brintrup DL, Ose C, Weinreich G, Passlick B, Hecker E, Kugler C, Dienemann H, Krbek T, Eggeling S, Hatz R, Müller MR, Weder W, Aigner C, Jöckel KH. Perioperative course and quality of life in a prospective randomized multicenter phase III trial, comparing standard lobectomy versus anatomical segmentectomy in patients with non-small cell lung cancer up to 2 cm, stage IA (7th edition of TNM staging system). Lung Cancer 2019;138:19-26.
- Wen Z, Zhao Y, Fu F, Hu H, Sun Y, Zhang Y, Chen H. Comparison of outcomes following segmentectomy or lobectomy for patients with clinical N0 invasive lung adenocarcinoma of 2 cm or less in diameter. J Cancer Res Clin Oncol 2020;146:1603-13.
- Shafiq-Ul-Hassan M, Latifi K, Zhang G, Ullah G, Gillies R, Moros E. Voxel size and gray level normalization of CT radiomic features in lung cancer. Sci Rep 2018;8:10545. [Crossref] [PubMed]
- Mackin D, Fave X, Zhang L, Yang J, Jones AK, Ng CS, Court L. Harmonizing the pixel size in retrospective computed tomography radiomics studies. PLoS One 2017;12:e0178524. [Crossref] [PubMed]
- Da-Ano R, Masson I, Lucia F, Doré M, Robin P, Alfieri J, Rousseau C, Mervoyer A, Reinhold C, Castelli J, De Crevoisier R, Rameé JF, Pradier O, Schick U, Visvikis D, Hatt M. Performance comparison of modified ComBat for harmonization of radiomic features for multicenter studies. Sci Rep 2020;10:10248. [Crossref] [PubMed]
- Ligero M, Jordi-Ollero O, Bernatowicz K, Garcia-Ruiz A, Delgado-Muñoz E, Leiva D, Mast R, Suarez C, Sala-Llonch R, Calvo N, Escobar M, Navarro-Martin A, Villacampa G, Dienstmann R, Perez-Lopez R. Minimizing acquisition-related radiomics variability by image resampling and batch effect correction to allow for large-scale data analysis. Eur Radiol 2021;31:1460-70. [Crossref] [PubMed]
- Traverso A, Wee L, Dekker A, Gillies R. Repeatability and Reproducibility of Radiomic Features: A Systematic Review. Int J Radiat Oncol Biol Phys 2018;102:1143-58. [Crossref] [PubMed]
- Tunali I, Hall LO, Napel S, Cherezov D, Guvenis A, Gillies RJ, Schabath MB. Stability and reproducibility of computed tomography radiomic features extracted from peritumoral regions of lung cancer lesions. Med Phys 2019;46:5075-85. [Crossref] [PubMed]