Improved [68Ga]Ga-PSMA-11 image qualities reconstructed by total variation regularized expectation maximization on total-body PET/CT
Introduction
Prostate-specific membrane antigen (PSMA) positron emission tomography (PET) is increasingly being applied to the imaging of prostate cancer (PCa) (1), which is the third most common cause of cancer death in Europe. In contrast to computed tomography (CT), magnetic resonance imaging (MRI), and choline-based PET imaging, [68Ga]Ga-PSMA PET has achieved improved detection performance by taking advantage of the specific biological characteristics of PSMA expression upregulated in PCa cells of varying disease stages (2). Afshar-Oromieh et al. showed that [68Ga]Ga-PSMA PET has better PCa detection performance compared to [18F]F-fluoromethylcholine PET (3). Correspondingly, Pfister et al. (4) found that [68Ga]Ga-PSMA PET performed better than [18F]F-fluoroethylcholine PET in individuals with biochemical recurrence. Studies have also highlighted the advantage of [68Ga]Ga-PSMA in high detection accuracy of the prostate primary tumor, metastasis (5), and tumor recurrence (6). In recent years, targeting [68Ga]Ga- or [18F]F-labeled PET agents to PSMA has become increasingly essential. [68Ga]Ga-PSMA-11 (syn. [68Ga]Ga-PSMA-HBED-CC), and the theranostic agents [68Ga]Ga-PSMA-617 and [68Ga]Ga-PSMA-I&T are the most extensively utilized [68Ga]Ga-labelled PSMA ligands for PET imaging (7,8). [18F]F-DCFBC (9,10), [18F]FDCFPyL (11), and [18F]F-PSMA 1007 are some of the agents that have been labeled with [18F]F (12). In this study, we aimed only to investigate [68Ga]Ga-PSMA-11.
Currently, statistical iterative reconstruction methods are the most widely used image reconstruction methods and the ordered subset expectation maximization (OSEM) algorithm is the gold standard. OSEM algorithms approach the acquired image by successive updated approximations, repeated until the difference between the projections of the reconstructed image and the actual recorded one falls below a specific level. The OSEM algorithm has a limited number of iterations and subsets to suppress image noise, which is a balance between noise and accuracy. This early stop leads to a bias in the final image estimate toward the initial image and to a decrease in contrast recovery (CR), signal-to-noise ratio (SNR), and image quality, which is partly accountable for the ineffective convergence of the algorithm. This may generate suboptimal image quality and inaccuracies in a quantitative assessment (13,14).
Total variation regularized expectation maximization (TVREM), a novel Bayesian penalized likelihood (BPL) reconstruction algorithm, can complete convergence while limiting image noise (15) and preserving edges (16) compared to the OSEM algorithm, due to the application of regularization strength parameter (β) that is added to the likelihood function. Thereby, it may further improve the sensitivity and accuracy in quantitative evaluation and shorten acquisition time (17) or reduce administrated dose. The penalization function is a difference and sum between neighboring voxels, which incorporates the pixel-to-pixel total variation, global noise equivalent counts, and local sensitivity profile. Until recently, abundant studies have shown promising results on [68Ga]Ga-PSMA conventional PET for BPL (18,19). The BPL reconstruction algorithm (Q.CLEAR) proposed by GE Healthcare (Chicago, IL, USA) claims to enhance image accuracy, and has a superior SNR to that of the conventional iterative reconstruction algorithm, according to quantitative evaluation (20,21). Ter Voert et al. reported an optimal SNR of β values between 400 and 550 for [68Ga]Ga-PSMA clinical scanning (18). However, the previous studies were performed on conventional PET/CT scanners with a standard axial field of view (AFOV) of 30 cm which limit the system sensitivity.
To overcome the limitation of low sensitivity and short AFOV in current clinical PET scanners, a 194-cm-long PET/CT system for total-body imaging (uEXPLORER; United Imaging Healthcare, Shanghai, China) was developed. Compared with conventional scanners, the massively increased sensitivity of the uEXPLORER substantially improves the PET image quality through a high SNR that supports the high spatial and temporal resolution and also provides much better lesion detection and region of interest (ROI) quantification results, which can be utilized to shorten the acquisition time while maintaining comparable image quality and lesion detectability in oncological studies (22).
The [68Ga]Ga has shown different uptake patterns due to the different positron energies, positron ranges, background activities, tumor-to-background ratios (TBR) (23), and pharmacodynamics in contrast to [18F]F-FDG PET. To our knowledge, no previous study had evaluated the [68Ga]Ga-PSMA-11 image quality reconstructed with TVREM on total-body PET. Therefore, the goal of this study was to evaluate the image quality of [68Ga]Ga-PSMA-11 on total-body PET and explore the optimal regularization parameter β in the TVREM algorithm to achieve better image quality.
Methods
Patients
A total of 51 patients [age 68.75±7.35 years, weight 67.87±9.84 kg, with a mean serum prostate-specific antigen (PSA) level of 29.48±35.99 ng/mL] who had been referred to undergo [68Ga]Ga-PSMA-11 total-body PET/CT were retrospectively enrolled.
The study was conducted in accordance with the Declaration of Helsinki (as revised in 2013). The study was approved by the Ethics Board of Renji Hospital, School of Medicine, Shanghai Jiao Tong University and informed consent was provided by all the patients. The patient information is displayed in Table 1. The selection criteria for patients were based on the following: (I) a surgical history of PCa or proven biopsy results; (II) a feasible serum PSA test; (III) the discovery of PSMA-positive malignant lesion/s on PET images. There were no adverse effects observed after injection of [68Ga]Ga-PSMA-11. In all reconstruction groups, a total of 84 PSMA-avid lesions were identified: 46 (55%) lesions in the soft tissue, 27 (32%) lesions in the lymph node, and 11 (13%) lesions in the bone.
Table 1
Characteristics | Value |
---|---|
Age (years) | 68.75±7.35 [50, 82] |
Height (m) | 1.70±0.05 [1.60, 1.83] |
Weight (kg) | 67.87±9.84 [51, 93] |
Body mass index (kg/m2) | 23.39±3.02 [16.28, 30.12] |
Uptake time (min) | 55±7 [45, 82] |
Injected dose (MBq) | 134.68±23.71 [87.34, 206.52] |
Injected dose per kg (MBq/kg) | 1.98±0.18 [1.59, 2.42] |
Serum PSA (ng/mL) | 29.48±35.99 [0.02, 172.09] |
Treatment-naïve patients (n) | 34 |
The values were presented as mean ± SD [range]. PSA, prostate-specific antigen; SD, standard deviation.
Data acquisition
All 51 cases underwent [68Ga]Ga-PSMA-11 total-body PET/CT scan using a digital time-of-flight (TOF) PET/CT scanner (uEXPLORER, United Imaging Healthcare). The scan was performed approximately 55 minutes after intravenous injection of [68Ga]Ga-PSMA-11 according to patient weight (1.98±0.18 MBq/kg). The routine clinical PET/CT protocol was applied with 5 minutes acquisition (total body), 600 mm transaxial field of view (FOV), 192×192 matrix, and 2.886 mm slice thickness with TOF and point-spread-function model, and standard corrections such as decay, scatter, normalization, random, attenuation, and dead time applied. The images were reconstructed with OSEM (3 iterations, 20 subsets, and 3 mm Gaussian postprocessing filter) and TVREM (5 penalization factors: 0.09, 0.18, 0.27, 0.36, and 0.45) respectively using 20-, 40-, 60-, 120-, and 300-seconds duration for the first part of the original scanning data acquisition from list-mode. We named these 30 groups O20, O40, O60, O120, and O300 for OSEM with simply 20-, 40-, 60-, 120-, and 300-second data; R20.09 to R20.45 for TVREM with 20 second acquisition and penalization factors of 0.09, 0.18, 0.27, 0.36, and 0.45, and the same rule applies to the naming of other groups (Table S1).
Quantitative evaluation of images
Image analysis was performed by an experienced nuclear medicine physician on the advantage workstation (uWS-MI R004, United Imaging Healthcare). To ensure consistency in the size and position of the drawing while drawing a volume of interest (VOI), the workstation was loaded with images that were reconstructed using various algorithms. The VOI on the liver was delineated by placing a 3 cm diameter sphere on the uniform tissue area in the right upper lobe, and the mean standard uptake value (SUVmean) and standard deviation (SD) were also measured for each patient. The image noise level was calculated by SD over SUVmean.
The ROIs around lesions were drawn manually and divided according to their maximum standard uptake value (SUVmax) in O300 reconstruction (<15 vs. ≥15), and localization (bone, lymph nodes, and soft tissue). For each lesion, the SUVmax was calculated and normalized by the ratio of SUVmax for TVREM to that for O300. The TBR and CR ratio were calculated respectively to evaluate the image quality. To calculate TBR, lesion SUVmax was divided by SUVmean of the background (liver), and we also defined CR by comparing the TBR of the TVREM reconstruction (numerator) to the TBR of the reference O300 reconstruction (denominator), according to the formula below:
In the formula, SUVmax is obtained from the lesion, SUVmean_bkgnd represents SUVmean from the liver background (‘bkgnd’), and ‘ref’ indicates the reference O300 reconstruction.
Qualitative evaluation of images
The reconstructed images were evaluated by 2 experienced nuclear radiologists independently on the advantage workstation (uWS-MI R004, United Imaging Healthcare) based on a 5-point scale. The scoring criteria are summarized in Table 2. The reviewers blindly rated the images and were unaware of the order and type of reconstruction methods. All images were anonymized, and the reading sequence was randomized to reduce the bias. We also calculated the SNR to verify our image-scoring results.
Table 2
Categories | Noise | Lesion detectability |
---|---|---|
1 | Poor | Not available |
2 | Marked | Poor |
3 | Routine clinical diagnosis available | Average |
4 | Clinical diagnosis irrelevant | Good |
5 | Almost none | Excellent |
Statistical analysis
The SUV of O300 served as the reference for the comparison between different reconstruction groups. The nonparametric Friedman test was used to examine the difference in liver SUVmean, SD, and lesion SUVmax between O300 and the other groups. The image quality scores of different reconstruction groups were compared with the nonparametric Friedman test. Bonferroni-Holm correction was used to adjust the P-value, which controlled the familywise error rate imposed by multiple comparisons. The inter-rater agreement was measured by Cohen’s κ. Data analysis was carried out using commercial software (SPSS 23.0; IBM Corp., Armonk, NY, USA) and graphics generated by GraphPad Prism 8 software (GraphPad Software Inc., San Diego, CA, USA). A P value of <0.05 was considered statistically significant.
Phantom study
Phantom preparation
For quantitative analysis of [68Ga]Ga-PSMA-11 PET/CT images, a National Electrical Manufacturers Association (NEMA) image quality (IQ) phantom was used in this study (24,25). A total of 6 hot spheres with diameters of 10-, 13-, 17-, 22-, 28-, and 37-mm were placed in the phantom, which were filled with a 4-fold background activity. According to the NEMA standard, the phantom was filled with 8.94 kBq/mL of background activity at the beginning of data acquisition.
Phantom data acquisition
The phantom was placed horizontally on the bed to ensure that the 6 spheres in the total-body PET/CT were located at the center of Unit 3 (uEXPLORER, United Imaging Healthcare). A CT scan was obtained first for attenuation correction, followed by a 5-minute PET scan. PET raw data were collected in list mode. All images were reconstructed using a 192×192 matrix, a FOV of 600 mm, a 3 mm Gaussian filter, a slice thickness of 2.886 mm, TOF, and the point spread function (PSF). The OSEM (3 iterations with 20 subsets) and TVREM (5 penalization factors: 0.09, 0.18, 0.27, 0.36, and 0.45) were applied to list-mode data with durations of 20-, 40-, 60-, 120-, and 300-second, which were consistent with our clinical studies, and named in the same rules (Table S1).
Phantom data evaluation
As illustrated in Figure S1, different ROIs were placed in reconstructed PET images for quantitative comparison. A total of 6 spherical ROIs were placed on the 6 spheres, and 12 ROIs were drawn as the background reference. The NEMA NU-2 2018 standard was used to calculate the contrast recovery coefficients (CRC) and background variability (BV) (Appendix 1) (24).
Results
Quantitative analysis of images
The CRs for TVREM groups with smaller penalization factors were higher than those of OSEM and TVREM groups with larger penalization factors (Figure 1). For the same duration of the list-mode data, the TVREM groups had higher CRs than OSEM except for R20.18 to R20.45, in which the image noise was decreased as the penalization factors increased (Figure 1). The image noise for the groups of R40.45, R60.36, R60.45, R120.27 to R120.45, and R300.09 to R300.45 was smaller than that of O300, yet only differences in Group R120.45 (P<0.001), R300.36 (P=0.011), and R300.45 (P<0.001) were statistically significant.
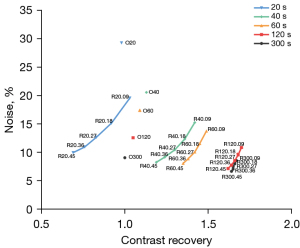
The image noise and the normalized SUVmax were decreased as the penalization factors increased (Figures 1,2A, and Table S2). At 40 seconds, the image noise of R40.09 to R40.27 decreased significantly (all P<0.05), whereas the lesions SUVmax increased 15.24–19.05% compared to O40. The R60.09 to R60.45 groups all had significantly decreased image noise (all P<0.05) whereas the lesions SUVmax were increased by 18.27–22.72% compared to O60. The R120.18 to R120.45 groups also had lower image noise (all P<0.05) and higher lesions SUVmax (increase of 26.73–27.72%) compared to O120. In contrast to O300, the image noise of R300.36 and R300.45 decreased significantly (all P<0.05) whereas the SUVmax of lesions increased by 26%.
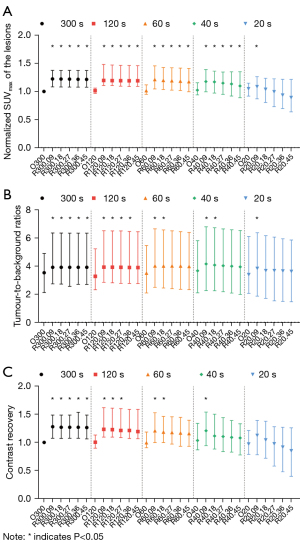
Compared to the O300 group, the image noise for the groups of R40.45, R60.36, R60.45, R120.27, R120.36, and R300.09 to R300.27 were lower with no statistically significant difference observed (all P>0.05), which were considered equivalent groups to O300. Moreover, the lesions SUVmax increased by 17% for R40.45, 24% for R60.36, 23% for R60.45, 28% for R120.27 and R120.36, 27% for R300.09, and 26% for R300.27 compared to O300, respectively (Figure 2A and Table S2).
The TBR values for TVREM were significantly higher than O300 (all P<0.05), except for R120.45, R60.27 to R60.45, R40.27 to R40.45, and R20.18 to R20.45 (Figure 2B). Besides, CR values were also significantly higher in TVREM compared to the O300 group (all P<0.05), except for R120.36 to R120.45, R60.27 to R60.45, R40.18 to R40.45, and R20.09 to R20.45 (Figure 2C).
The CR and TBR for higher [68Ga]Ga-PSMA-11 avidity, for which lesion SUVmax was ≥15 in O300, were significantly higher than O300 in all TVREM groups (Figure 3A,3B), whereas minor increases were observed for lesions with SUVmax <15 (Figure 3C,3D) due to the less significant variation in TVREM groups.
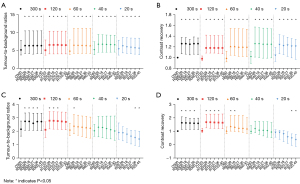
Qualitative evaluation of the image quality
The highest image quality score in terms of image noise, except for TVREM for 300 second groups, was assigned to R120.36 (5.00±0.00) for 120 second groups (Figure 4A), and the highest score of lesion detectability was assigned to R120.27, R120.36, and R120.45 (4.98±0.15) (Figure 4B). The image quality of the aforementioned reconstruction groups was considered equivalent to that of O300 (all P>0.05). To verify our image scoring results, we calculated the SNR and found that the highest SNR was in the R120.27 group, which increased by 41.46% compared to that of O120, and increased by 51.92% compared to that of O300 (Figures 5,6).
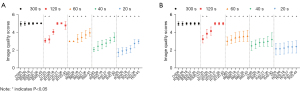
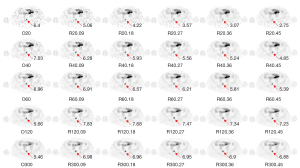
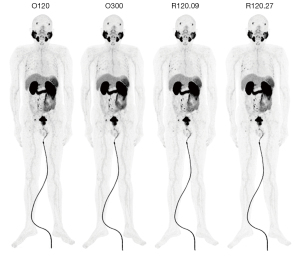
Phantom study
Figure S2A indicates that TVREM groups with smaller penalization factors achieved higher CRCs than OSEM and TVREM groups with larger penalization factors, and all TVREM groups outperformed OSEM under equivalent sphere diameter and acquisition time. Figure S2B shows that BVs decreased as penalization factors increased, and most TVREM groups had lower BVs than OSEM under equivalent sphere diameter and acquisition time. TVREM with acquisition times of 120 and 300 seconds had BVs comparable to O120 and O300, respectively, with differences ranging from 0 to 0.4%, except for R300.09 with the 10 mm diameter sphere. Furthermore, most TVREM algorithm groups demonstrated higher BVs than the O300 group, except for the R120.09–R120.45 results for the 28 and 37 mm spheres in the TVREM algorithm group, which had similar BV performance to the O300 group (Figure S2B), with differences limited to 0–0.4%.
Discussion
The study has shown that the TVREM reconstruction with proper penalization factor can significantly improve the image quality over the OSEM reconstruction algorithm in [68Ga]Ga-PSMA-11 total-body PET/CT. The TVREM 120 second group with a penalization factor in the range of 0.27 to 0.45 can achieve a 62–65% higher lesion contrast and 5–21% lower image noise compared to the OSEM 300 second group while saving 60% acquisition time. Moreover, the penalization factor between 0.27 and 0.36 in the TVREM 120 second group provided the optimal image quality for [68Ga]Ga-PSMA-11 total-body PET with a comparable to or even lower noise level and higher contrast for lesions than in the OSEM 300 second group.
Several factors can affect the choice of the optimal penalization factor, including the setting of data collection, doctor preference, and adjustment of the reconstruction algorithm. Hence, in most cases, the optimal penalization factor is often provided as a range. TVREM has been shown in studies to maintain image quality in a relatively short acquisition time, with a penalty factor ranging from 0.14 to 0.35 (16). Yang et al. demonstrated that, as compared to OSEM, the TVREM algorithm can improve the contrast of [68Ga]Ga-PSMA-11 lesion images, decrease image noise, and obtain images at a rate of 2 min/bed while maintaining image quality during short-axis PET/CT (26). Consistent with those studies, our findings demonstrated that when the total body was obtained simultaneously, a factor within 0.27–0.45 for the TVREM 120 second group could generate higher contrast and lower noise levels compared to the OSEM 300 second group (P<0.05). Moreover, the factor between 0.27 and 0.36 for TVREM had equivalent image noise to the OSEM 300 second group when the acquisition time was shortened to 120 seconds (P>0.05). Therefore, the choice of optimal penalization factors may be a critical variable accompanied by the criteria for optimal image quality and acquisition settings.
A higher SUVmax provides a more precise and accurate quantitative evaluation, which is desired in clinical imaging. Our results indicate that parameters calculated by TVREM could more efficiently detect PCa lesions with varying [68Ga]Ga-PSMA-11 uptake. Numerous studies have showed that BPL algorithms could enhance the lesion quantification accuracy compared to OSEM due to their full iterative convergence without over-amplified noise; however, most of those studies were about [18F]F-FDG PET/CT (20,27,28). Our study further investigated the improvement of image quality of TVREM compared to OSEM in [68Ga]Ga-PSMA-11 total-body PET/CT. The TBR increased by 24%, the CR increased by 62–65%, and the lesion SUVmax increased by 28% with a penalization factor between 0.27 and 0.45 in the 120 second group compared to OSEM 300 seconds, maintaining the lower image noise ranging from 5% to 21%.
In a phantom study comparing the performance of the TVREM and OSEM algorithms, our findings demonstrated that the TVREM algorithm consistently outperformed the OSEM algorithm in terms of CRC. As the penalization factor β increases, there is a gradual decrease in CRC. This suggests that within the β range used in this study, the quantification results are stable, making TVREM more robust for clinical application. Additionally, the BV results showed that the TVREM algorithm provided higher uniformity in the reconstructed background compared to the OSEM algorithm under the same conditions. The uniformity of the background in images reconstructed with the 120-second TVREM algorithm is comparable to that of the 300-second OSEM algorithm, consistent with clinical results.
The 2 experienced nuclear radiologists evaluated all reconstruction images with sufficient confidence from 2 main aspects, including noise and lesion detectability. The results showed the highest mean quality score that both noise and lesion detectability was given when the penalization factor was 0.36 for 120 second acquisition time and also contained all TVREM for 300 second groups. In addition, the image quality of that penalization factor was 0.36 for the 120 second reconstruction group, which was considered equivalent to OSEM for the 300 second group, yet it was given a significantly higher image quality score than OSEM for 120 second group, which embodied the advantages of the TVREM algorithm in visual assessment of image reconstruction. However, the weighted kappa coefficient that evaluated the assessment consistency was equal to or lesser than 0.52 (Table S3) and indicated a modest inter-rater agreement, which implied lower stability than objective indicator results.
The fixed penalization factor is necessitated in clinical practice to maintain a consistent SUV as inconsistency in SUV ascribed to variation among scanners and PET imaging methods remains challenging in both clinical routine and research studies (29-31). Moreover, according to the results of our phantom experiment, the CRC of the TVREM algorithm was generally higher than that of the OSEM algorithm. Therefore, the reconstruction conditions such as the TVREM algorithm could more properly reflect tracer activity. To balance visual assessment and quantitative evaluation, our study suggested the optimal penalization factor of 0.27–0.36 in 120 second acquisition time, which may achieve the desirable SUV measurement and reflect the true tracer activity in tissues.
The shortening of acquisition time helps to reduce the possible patient motion artifacts, improve hospital throughput, and simplify the workflow for image reconstruction, transmission, and storage in clinical situations (32). A study on [18F]F-FDG PET revealed that the BPL algorithm has potential for shortening acquisition time (33,34). In line with this study, our results indicated that TVREM saved 60% acquisition time of [68Ga]Ga-PSMA-11 total-body PET/CT without compromising image quality. In addition, the superiority of total-body PET/CT systems and the advantages of the BPL algorithm in noise reduction and lesion detection enhancement might also contribute to dose reduction (26,35,36).
There were still several limitations to our study. First, the study population did not include biochemical recurrence patients with lower PSA levels; future research should be expanded to include such patients. Secondly, we did not explore whether the increased CR and TBR of the TVREM algorithm can contribute to the early detection of prostate tumors. Besides, our analysis was performed solely on [68Ga]Ga-PSMA-11 total-body PET/CT; more evaluations and validations are necessary to further verify our results. Furthermore, the application of total-body PET/CT with the TVREM algorithm in human research would contribute to the investigation of multiorgan diseases, or cancer and other systemic diseases. Hopefully, the total-body PET/CT systems can become commercially available and economically reasonable, and even be supported in some basis of clinical care.
Conclusions
The TVREM reconstruction algorithm can remarkably improve lesion contrast and reduce image noise compared to OSEM in [68Ga]Ga-PSMA-11 total-body PET/CT, hence enabling shorter acquisition time while maintaining a similar image quality.
Acknowledgments
The authors want to thank all the clinical and research staff at the Department of Nuclear Medicine, Renji Hospital, Shanghai Jiao Tong University for their technical assistance and helpful discussions.
Funding: This work was supported in part by the National Key Research and Development Program of China (Grant Nos. 2020YFA0909000 and 2021YFA0910000), the National Natural Science Foundation of China (Grant Nos. 82001878 and 82171972), and the Shanghai Rising-Star Program (Grant No. 20QA1406100).
Footnote
Conflicts of Interest: All authors have completed the ICMJE uniform disclosure form (available at https://qims.amegroups.com/article/view/10.21037/qims-22-1341/coif). XY, DH, HS, QG, YLN, and YZ are employees of United Imaging Healthcare Group Co., Ltd. The other authors have no conflicts of interest to declare.
Ethical Statement: The authors are accountable for all aspects of the work in ensuring that questions related to the accuracy or integrity of any part of the work are appropriately investigated and resolved. The study was conducted in accordance with the Declaration of Helsinki (as revised in 2013). The study was approved by the Ethics Board of Renji Hospital, School of Medicine, Shanghai Jiao Tong University and informed consent was provided by all the patients.
Open Access Statement: This is an Open Access article distributed in accordance with the Creative Commons Attribution-NonCommercial-NoDerivs 4.0 International License (CC BY-NC-ND 4.0), which permits the non-commercial replication and distribution of the article with the strict proviso that no changes or edits are made and the original work is properly cited (including links to both the formal publication through the relevant DOI and the license). See: https://creativecommons.org/licenses/by-nc-nd/4.0/.
References
- Fendler WP, Eiber M, Beheshti M, Bomanji J, Ceci F, Cho S, Giesel F, Haberkorn U, Hope TA, Kopka K, Krause BJ, Mottaghy FM, Schöder H, Sunderland J, Wan S, Wester HJ, Fanti S, Herrmann K. (68)Ga-PSMA PET/CT: Joint EANM and SNMMI procedure guideline for prostate cancer imaging: version 1.0. Eur J Nucl Med Mol Imaging 2017;44:1014-24. [Crossref] [PubMed]
- Perner S, Hofer MD, Kim R, Shah RB, Li H, Möller P, Hautmann RE, Gschwend JE, Kuefer R, Rubin MA. Prostate-specific membrane antigen expression as a predictor of prostate cancer progression. Hum Pathol 2007;38:696-701. [Crossref] [PubMed]
- Afshar-Oromieh A, Zechmann CM, Malcher A, Eder M, Eisenhut M, Linhart HG, Holland-Letz T, Hadaschik BA, Giesel FL, Debus J, Haberkorn U. Comparison of PET imaging with a (68)Ga-labelled PSMA ligand and (18)F-choline-based PET/CT for the diagnosis of recurrent prostate cancer. Eur J Nucl Med Mol Imaging 2014;41:11-20. [Crossref] [PubMed]
- Pfister D, Porres D, Heidenreich A, Heidegger I, Knuechel R, Steib F, Behrendt FF, Verburg FA. Detection of recurrent prostate cancer lesions before salvage lymphadenectomy is more accurate with (68)Ga-PSMA-HBED-CC than with (18)F-Fluoroethylcholine PET/CT. Eur J Nucl Med Mol Imaging 2016;43:1410-7. [Crossref] [PubMed]
- Hofman MS, Lawrentschuk N, Francis RJ, Tang C, Vela I, Thomas P, et al. Prostate-specific membrane antigen PET-CT in patients with high-risk prostate cancer before curative-intent surgery or radiotherapy (proPSMA): a prospective, randomised, multicentre study. Lancet 2020;395:1208-16. [Crossref] [PubMed]
- Maurer T, Eiber M, Schwaiger M, Gschwend JE. Current use of PSMA-PET in prostate cancer management. Nat Rev Urol 2016;13:226-35. [Crossref] [PubMed]
- Eder M, Schäfer M, Bauder-Wüst U, Hull WE, Wängler C, Mier W, Haberkorn U, Eisenhut M. 68Ga-complex lipophilicity and the targeting property of a urea-based PSMA inhibitor for PET imaging. Bioconjug Chem 2012;23:688-97. [Crossref] [PubMed]
- Weineisen M, Schottelius M, Simecek J, Baum RP, Yildiz A, Beykan S, Kulkarni HR, Lassmann M, Klette I, Eiber M, Schwaiger M, Wester HJ. 68Ga- and 177Lu-Labeled PSMA I&T: Optimization of a PSMA-Targeted Theranostic Concept and First Proof-of-Concept Human Studies. J Nucl Med 2015;56:1169-76. [Crossref] [PubMed]
- Cho SY, Gage KL, Mease RC, Senthamizhchelvan S, Holt DP, Jeffrey-Kwanisai A, Endres CJ, Dannals RF, Sgouros G, Lodge M, Eisenberger MA, Rodriguez R, Carducci MA, Rojas C, Slusher BS, Kozikowski AP, Pomper MG. Biodistribution, tumor detection, and radiation dosimetry of 18F-DCFBC, a low-molecular-weight inhibitor of prostate-specific membrane antigen, in patients with metastatic prostate cancer. J Nucl Med 2012;53:1883-91. [Crossref] [PubMed]
- Rowe SP, Gage KL, Faraj SF, Macura KJ, Cornish TC, Gonzalez-Roibon N, et al. 18F-DCFBC PET/CT for PSMA-Based Detection and Characterization of Primary Prostate Cancer. J Nucl Med 2015;56:1003-10. [Crossref] [PubMed]
- Rowe SP, Macura KJ, Mena E, Blackford AL, Nadal R, Antonarakis ES, Eisenberger M, Carducci M, Fan H, Dannals RF, Chen Y, Mease RC, Szabo Z, Pomper MG, Cho SY. PSMA-Based [(18)F]DCFPyL PET/CT Is Superior to Conventional Imaging for Lesion Detection in Patients with Metastatic Prostate Cancer. Mol Imaging Biol 2016;18:411-9. [Crossref] [PubMed]
- Giesel FL, Hadaschik B, Cardinale J, Radtke J, Vinsensia M, Lehnert W, Kesch C, Tolstov Y, Singer S, Grabe N, Duensing S, Schäfer M, Neels OC, Mier W, Haberkorn U, Kopka K, Kratochwil C. F-18 labelled PSMA-1007: biodistribution, radiation dosimetry and histopathological validation of tumor lesions in prostate cancer patients. Eur J Nucl Med Mol Imaging 2017;44:678-88. [Crossref] [PubMed]
- Qi J, Leahy RM. Iterative reconstruction techniques in emission computed tomography. Phys Med Biol 2006;51:R541-78. [Crossref] [PubMed]
- Iriarte A, Marabini R, Matej S, Sorzano CO, Lewitt RM. System models for PET statistical iterative reconstruction: A review. Comput Med Imaging Graph 2016;48:30-48. [Crossref] [PubMed]
- Gao J, Liu Q, Zhou C, Zhang W, Wan Q, Hu C, Gu Z, Liang D, Liu X, Yang Y, Zheng H, Hu Z, Zhang N. An improved patch-based regularization method for PET image reconstruction. Quant Imaging Med Surg 2021;11:556-70. [Crossref] [PubMed]
- Wernick MN, Infusino EJ, Milosević M. Fast spatio-temporal image reconstruction for dynamic PET. IEEE Trans Med Imaging 1999;18:185-95. [Crossref] [PubMed]
- Liu L, Liu H, Xu S, Zhang S, Tao Y, Mok GSP, Chen Y. The Impact of Total Variation Regularized Expectation Maximization Reconstruction on (68)Ga-DOTA-TATE PET/CT Images in Patients With Neuroendocrine Tumor. Front Med (Lausanne) 2022;9:845806. [Crossref] [PubMed]
- Ter Voert EEGW, Muehlematter UJ, Delso G, Pizzuto DA, Müller J, Nagel HW, Burger IA. Quantitative performance and optimal regularization parameter in block sequential regularized expectation maximization reconstructions in clinical (68)Ga-PSMA PET/MR. EJNMMI Res 2018;8:70. [Crossref] [PubMed]
- Krokos G, Pike LC, Cook GJR, Marsden PK. Standardisation of conventional and advanced iterative reconstruction methods for Gallium-68 multi-centre PET-CT trials. EJNMMI Phys 2021;8:52. [Crossref] [PubMed]
- Teoh EJ, McGowan DR, Macpherson RE, Bradley KM, Gleeson FV. Phantom and Clinical Evaluation of the Bayesian Penalized Likelihood Reconstruction Algorithm Q.Clear on an LYSO PET/CT System. J Nucl Med 2015;56:1447-52. [Crossref] [PubMed]
- Roef MJ, Rijnsdorp S, Brouwer C, Wyndaele DN, Arends AJ. Evaluation of Quantitative Ga-68 PSMA PET/CT Repeatability of Recurrent Prostate Cancer Lesions Using Both OSEM and Bayesian Penalized Likelihood Reconstruction Algorithms. Diagnostics (Basel) 2021.
- Cherry SR, Jones T, Karp JS, Qi J, Moses WW, Badawi RD. Total-Body PET: Maximizing Sensitivity to Create New Opportunities for Clinical Research and Patient Care. J Nucl Med 2018;59:3-12. [Crossref] [PubMed]
- Bertolli O, Eleftheriou A, Cecchetti M, Camarlinghi N, Belcari N, Tsoumpas C. PET iterative reconstruction incorporating an efficient positron range correction method. Phys Med 2016;32:323-30. [Crossref] [PubMed]
- National Electrical Manufactures Association. NEMA standards publication NU 2-2012: performance measurements of positron emission tomographs. Rosslyn, VA: National Electrical Manufactures Association 2012.
- Pasawang P, Sontrapornpol T, Krisanachinda A. Experience on performance measurements of positron emission tomographs: NEMA NU2–2018. Medical Physics 2019;7.
- Yang FJ, Ai SY, Wu R, Lv Y, Xie HF, Dong Y, Meng QL, Wang F. Impact of total variation regularized expectation maximization reconstruction on the image quality of (68)Ga-PSMA PET: a phantom and patient study. Br J Radiol 2021;94:20201356. [Crossref] [PubMed]
- Ahn S, Ross SG, Asma E, Miao J, Jin X, Cheng L, Wollenweber SD, Manjeshwar RM. Quantitative comparison of OSEM and penalized likelihood image reconstruction using relative difference penalties for clinical PET. Phys Med Biol 2015;60:5733-51. [Crossref] [PubMed]
- Teoh EJ, McGowan DR, Bradley KM, Belcher E, Black E, Gleeson FV. Novel penalised likelihood reconstruction of PET in the assessment of histologically verified small pulmonary nodules. Eur Radiol 2016;26:576-84. [Crossref] [PubMed]
- Jaskowiak CJ, Bianco JA, Perlman SB, Fine JP. Influence of reconstruction iterations on 18F-FDG PET/CT standardized uptake values. J Nucl Med 2005;46:424-8. [PubMed]
- Adams MC, Turkington TG, Wilson JM, Wong TZ. A systematic review of the factors affecting accuracy of SUV measurements. AJR Am J Roentgenol 2010;195:310-20. [Crossref] [PubMed]
- Kinahan PE, Fletcher JW. Positron emission tomography-computed tomography standardized uptake values in clinical practice and assessing response to therapy. Semin Ultrasound CT MR 2010;31:496-505. [Crossref] [PubMed]
- Liu G, Hu P, Yu H, Tan H, Zhang Y, Yin H, Hu Y, Gu J, Shi H. Ultra-low-activity total-body dynamic PET imaging allows equal performance to full-activity PET imaging for investigating kinetic metrics of (18)F-FDG in healthy volunteers. Eur J Nucl Med Mol Imaging 2021;48:2373-83. [Crossref] [PubMed]
- Lindström E, Sundin A, Trampal C, Lindsjö L, Ilan E, Danfors T, Antoni G, Sörensen J, Lubberink M. Evaluation of Penalized-Likelihood Estimation Reconstruction on a Digital Time-of-Flight PET/CT Scanner for (18)F-FDG Whole-Body Examinations. J Nucl Med 2018;59:1152-8. [Crossref] [PubMed]
- Trägårdh E, Minarik D, Almquist H, Bitzén U, Garpered S, Hvittfelt E, Olsson B, Oddstig J. Impact of acquisition time and penalizing factor in a block-sequential regularized expectation maximization reconstruction algorithm on a Si-photomultiplier-based PET-CT system for (18)F-FDG. EJNMMI Res 2019;9:64. [Crossref] [PubMed]
- Geismar JH, Stolzmann P, Sah BR, Burger IA, Seifert B, Delso G, von Schulthess GK, Veit-Haibach P, Husmann L. Intra-individual comparison of PET/CT with different body weight-adapted FDG dosage regimens. Acta Radiol Open 2015;4:2047981614560076. [Crossref] [PubMed]
- Donswijk ML, Morigi JJ, Little A, Vogel WV, van Leeuwen PJ. Where to next prostate-specific membrane antigen PET imaging frontiers? Curr Opin Urol 2020;30:672-8. [Crossref] [PubMed]