The predictive value of a computed tomography-based radiomics model for the surgical separability of thymic epithelial tumors from the superior vena cava and the left innominate vein
Introduction
Thymic epithelial tumors (TETs) are the most common neoplasms in the anterior mediastinum, accounting for at least 50% of all anterior mediastinum neoplasms (1). To guide management of TETs, several staging systems have been developed, including the Masaoka-Koga classification and the tumor-node-metastasis (TNM) staging system from the International Thymic Malignancies Interest Group (ITMIG) and International Association for the Study of Lung Cancer (IASLC) (2). Generally, surgeons prefer minimally invasive thymectomy over open thymectomy due to its lower blood loss and fewer postoperative complications, especially in the respiratory system and heart (e.g., pneumonia, pleural effusion, and arrhythmias) (3-5). However, current clinical practice and research have shown that the resectability of TETs and the selection of surgical methods mainly relies on the evaluation of tumor metastasis, invasiveness, and tumor separability from surrounding organs and blood vessels. In cases where tumors invade surrounding blood vessels, replacement of the vessels may be necessary, while tumors that invade the lungs may require lung resection (6).
Computed tomography (CT) is the standard noninvasive diagnostic imaging modality used to assess the relationship between the tumor and surrounding vasculature. Recent research has focused on predicting vascular invasion in tumors using CT images. Two studies conducted on pancreatic cancer demonstrated that morphological changes in blood vessels and the length of contact between the tumor and the vessels were risk factors for vascular invasion (7,8). A study investigating mediastinal great vein invasion of TETs identified the irregularity of tumor margins, the interface between the tumor and great vein, and the absence of soft tissue in the interface as potential indicators of vascularly invasive TETs (9). However, merely determining whether a tumor has invaded the veins is not sufficient to inform operation-related decision-making. In clinical practice, when the tumor is tightly adherent to the great vessels due to the inflammatory reaction of the tumor, the surgeon may resect a portion of the vessel and perform angioplasty to achieve an R0 resection and prevent intraoperative and postoperatively bleeding. This can occur even if no vascular invasion is found pathologically, especially in veins with a relatively thin wall, such as the superior vena cava (SVC) and the left innominate vein (LIV). Given the technical challenges of vascular replacement during endoscopic surgery, misjudging the separability of the tumor and large blood vessels can present a significant obstacle for surgeons, further complicating the procedure and potentially leading to an increased conversion rate to thoracotomy.
Radiomics analysis is a radiological data-centric field, which entails extracting large amounts of image-related features and using these features to construct novel biomarkers for clinical endpoints. It is worth noting that unlike the interpretable semantic features extracted by radiologists, radiomics features are mostly nonsemantic and cannot be easily interpreted independently. In recent years, radiomics models have been developed in multiple solid tumors to predict pathological type, clinical stage, and prognosis (10-15). In TETs, radiomics analysis has been able to predict TNM stage and differentiate the type of thymic cyst (16-23). However, currently, no radiomics model exists that can predict surgically inseparable TETs from the great vein. Recently, radiomics analysis has made it possible to predict superior mesenteric artery invasion in pancreatic ductal adenocarcinoma. Therefore, we believe that preoperative prediction of the tumor relationship with the SCV and LIV (SVC-LIV) can be achieved through radiomics analysis, leading to a more reasonable arrangement of surgical modalities. Studies focusing on TETs and adjacent vessels have generally used contrast-enhanced CT (CECT). However, the computer’s ability to distinguish the grayscale values is far superior to that of observation of radiologists. Therefore, the contrast agent's dosage effect on the greyscale of blood vessels may cause deviations in the radiomics features. To construct a more refined and multidimensional model for the preoperative evaluation of TETs, we adopted a nonenhanced CT (NECT) scan to extract the radiomics features of TETs for analysis. To our knowledge, this is the first study on using radiomics analysis to predict vascularly inseparable TETs. We present this article in accordance with the TRIPOD reporting checklist (available at https://qims.amegroups.com/article/view/10.21037/qims-22-1050/rc).
Methods
Patient population
This study received approval from the Ethics Committee of West China Hospital of Sichuan University (No. 2021-578) and was conducted in accordance with the Declaration of Helsinki (as revised in 2013). Individual consent for this retrospective analysis was waived. In the retrospective analysis, patients who had undergone CT scans and were pathologically diagnosed with TETs at West China Hospital from April 2009 to September 2017 were selected consecutively in the primary inclusion. Patients were included if they met the following criteria: (I) diagnosis of thymoma or thymic carcinoma confirmed by pathologic report and (II) CT examination performed within 2 weeks prior to surgery. The exclusion criteria were as follows: (I) incomplete clinical data or CT images and (II) only an enhanced chest CT scan being performed. After inclusion, the baseline information was collected, including clinical characteristics of gender, age, TNM stage, operation selection, and image features, including calcification, heterogeneity, tumor lobulation, maximum diameter of the tumor, abutment degree of vessel circumference, irregular interface, irregularity of the tumor contour, the presence of a mediastinal fat layer, and the presence of space between the tumor and surrounding structures.
Definition of vascularly inseparable TETs
This study has defined vascular inseparable TETs based on interoperative exploration. Specifically, the interface between the tumor and SVC and LIV was classified into 3 categories: (I) without adherence, (II) adhering but separable, or (III) inseparable. To ensure the consistency of the classification and to prevent the overestimation of the invasion extent, this study only included the surgeries performed by surgeons with more than 10 years of experience in video-assisted thoracoscopic surgery (VATS).
Conventional criteria and classification of radiologist assessment
In this study, 2 radiologists assessed the CT images of all patients independently. To ensure consistency and reliability, any discrepancies were resolved through discussion. The radiologists were blinded to any clinical information during the procedure. They were asked to collect the following features: calcification, heterogeneity, tumor lobulation, maximum diameter of the tumor, abutment degree of vessel circumference, irregular interface, irregularity of the tumor contour, the presence of a mediastinal fat layer, and the presence of a space between the tumor and surrounding structures. The criteria for determining potential great vein invasion or vascularly inseparable TETs according to CT images were the following: (I) an irregular interface between the tumor and surrounding structures or an irregular tumor margin, (II) absence of a space between the tumor and the surrounding tissue and (III) absence of a mediastinal fat layer (9). The results of the radiologist’s evaluation were used as the radiological model and were compared with the radiomics models.
Construction of radiomics model
To establish a radiomics model, the following steps are typically taken. Initially, the region of interest (ROI) of the CT image is delineated, and a radiomics label is established. Subsequently, the CT image is preprocessed and filtered to extract radiomics features that will be included in the models. However, to prevent overfitting, the features are selected before inclusion into the models. Additionally, imbalanced data are upsampled to ensure that the evaluation of the models is unbiased. Finally, machine learning classifiers are used to build the radiomics model using the selected radiomics features. This entire process helps in creating an accurate and reliable radiomics model.
Imaging techniques
The CT images of included patients were obtained before the initial treatment. The images were obtained with CT scanners from the following manufacturers: Siemens (Somatom Definition AS and Somatom Definition Flash, Siemens Healthineers, Erlangen, Germany), GE HealthCare (Revolution CT, Chicago, IL, USA), Philips (Brilliance 64, Philips Healthcare, Amsterdam, The Netherlands). The tube voltage of most CT scans was between 100 and 120 kV, and the tube current was between 100 and 650 mA. The pixel size of the acquired CT images ranged from 0.417×0.417 to 0.977×0.977 mm2. All CT images had a resolution of 512×512 with a slice thickness of 5 mm. The segmentation was performed in both lung settings, with a width of 1,200 Hounsfield units (HU) and a level of –600 HU; for the mediastinal settings, the width was 350 HU, and the level was 40 HU.
Tumor segmentation and feature extraction
ITK-SNAP 3.8.0 (http://www.itksnap.org) was used for tumor segmentation. Two radiologists with at least 10 years of experience in thoracic imaging performed this procedure. To select the slice with the maximum tumor-vein contact in two-dimensional (2D) segmentation, the selection was performed by 2 independent radiologists, and any discrepancy was resolved by discussion. In three-dimensional (3D) segmentation, a radiologist labeled the ROI that covered the total tumor area, and another radiologist labeled the tumor on 50 randomly selected patients (Figure 1). In the segmentation process, radiologists were blinded to intraoperative separability.
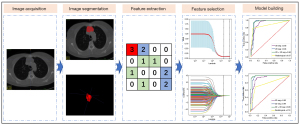
To perform image preprocessing and feature extraction, the “PyRadiomics” package (version 2.1; https://pyradiomics.readthedocs.io/en/latest/index.html) was installed in Python 3.7 software (Python Software Foundation, New York, USA) (24). Before feature extraction, the pixel spacing of images was resampled to 1×1 mm2, and a fixed bin width of 25 HU was applied for discretization. In all, 7 categories of features were extracted, including 16 shape-based features in 3D, 10 shape-based features in 2D, 19 first-order statistics features, 24 gray-level co-occurrence matrix (GLCM) features, 14 gray-level dependence matrix (GLDM) features, 16 gray-level run-length matrix (GLRLM) features, 16 gray-level size zone matrix (GLSZM) features, and 5 neighboring gray-tone difference matrix (NGTDM) features. In addition to the original CT image, first-order statistical features and texture features were also extracted from Laplacian of Gaussian (LoG) filters (sigma: 1.0, 2.0, 3.0, 4.0, and 5.0 mm) and wavelet filters in both 2D and 3D images. A total of 2,688 features were extracted. To test the interobserver reproducibility, the intraclass correlation coefficient (ICC) was calculated, and the features with ICC ≥0.8 were included in the subsequent analyses. The Z-score method was used to normalize the feature values, and the mathematical formula was as follows:
In this formula, xi refers to the feature value, refers to the mean value of all patients in this group, and s refers to the corresponding standard deviation.
Feature selection and radiomics signature building
Features were selected using univariate statistical tests (2-sample t-test) between the separable and the inseparable groups at first, and features with a P value <0.05 were chosen in the subsequent selection. The least absolute shrinkage and selection operator (LASSO) regression model was employed to reduce dimensionality and develop the radiomics signature. The cost function of LASSO method is as follows:
In this formula, m is the number of samples, xi is the value of the radiomic features, y(i) is the sample labels, λ is a tuning constant, θ is a coefficient vector, and n is the number of θ.
To balance the generation ability and minimize the overfitting risk, LASSO selection and radiomics signature building were performed based on repetitive stratified 5-fold cross-validation, and the number of iterations was set to 105 seconds. In each iteration, 4 folds of the cohort were used for training, and the remaining fold was used for validation (cross-validation fold). Following this, the entire training cohort was analyzed, and features with a nonzero coefficient were output.
Data balancing and classifier construction
Given the imbalance between cases free from vascular inseparable TETs and those defined as surgical inseparable TETs, we used adaptive synthetic sampling (ADASYN) methods to augment the minority group, thereby matching it with the majority class. ADASYN is based on the density of the minority group in the feature space, and it can adaptively generate instances to rebalance the minority and majority groups.
Support vector machine (SVM) with a Gaussian kernel was used as classifier to discriminate between the 2 groups in the selected feature space. The rationale behind using SVM was to generate hyperplanes that could maximize the margins between the plane and the nearest instances of the samples, while also taking the globally optimal solutions in account. To avoid overfitting, we used 5-fold cross-validation with 10 repetitions to evaluate and validate the performance (25). A receiver operating characteristic (ROC) curve was drawn, and the area under ROC curve (AUC) was used to illustrate the predictive performance.
Construction of the radiomics nomogram
Univariate logistic regression was used to select clinical image features for predicting vascular inseparable TETs. Features with a P value <0.05, as well as the radiomics score (radscore) of the best radiomics model, were input into a multivariable logistic regression to develop a radiomics nomogram. The performance of this nomogram was assessed with calibration curves and Hosmer-Lemeshow test for both the training and testing sets.
Statistical analysis
The differences in basic characteristics, such as sex, age, and TNM stage, between groups were analyzed using the Mann-Whitney test for continuous variables, while the analysis of variance (ANOVA) test was used for categorical variables. The difference in the ROC of these radiomics models and clinical imaging diagnosis was analyzed using the Delong test. Statistical analysis in this study was conducted using the “pandas” package in Python 3.7 software. All tests in this analysis were two-tailed, and P<0.05 was considered to indicate a significant difference.
Results
Patient characteristics
In total, 255 patients were identified histologically as having TETs; of these, 24 cases who underwent intravenous contrast CT scans, 10 cases lacking clinical variables, and 17 cases lacking CT images were excluded. Ultimately, 204 patients were included in this study (Figure 2). There were 105 males and 99 females, with a mean age of 51.53 years. In this cohort, 21 patients were diagnosed with vascularly inseparable TETs. A total of 172 patients had low-risk TETs (TNM stage I and II), and 32 patients had high-risk TETs (TNM stage III and IV). According to the World Health Organization (WHO) classification, a total of 174 thymomas with 9 thymic carcinomas were included in the separable TETs group, and 17 thymomas with 4 thymic carcinomas were included in the inseparable TETs group. Significant differences were observed in overall TNM stage, T stage, WHO classification, and operation section between the separable and inseparable TETs groups. During the 3D segmentation procedure, the mean slice of the ROI was 9.54 and 9.81 slices in the inseparable and separable groups, respectively. The characteristics of this cohort are summarized in Table 1. The cohort was randomly split into a training cohort (n=142) and a testing cohort (n=62) using a stratified random sampling ratio of 7:3. In the training cohort, there were 124 patients with separable TETs and 18 patients with inseparable TETs, while the testing cohort consisted of 59 patients with separable TETs and 3 patients with inseparable TETs.
Table 1
Variables | Separable (n=183) | Inseparable (n=21) | P value |
---|---|---|---|
Sex | 0.197 | ||
Male | 97 | 8 | |
Female | 86 | 13 | |
Age (years) | 51.89±12.17 | 49.3±10.18 | 0.333 |
Overall stage | <0.001 | ||
Stage I | 163 | 0 | |
Stage II | 9 | 0 | |
Stage IIIA | 9 | 19 | |
Stage IIIB | 0 | 1 | |
Stage IVA | 2 | 1 | |
T stage | <0.001 | ||
T1 | 162 | 0 | |
T2 | 10 | 0 | |
T3 | 10 | 19 | |
T4 | 1 | 2 | |
N stage | 0.632 | ||
N0 | 181 | 21 | |
N1 | 2 | 0 | |
N2 | 0 | 0 | |
M stage | 1.000 | ||
M0 | 183 | 21 | |
M1 | 0 | 0 | |
WHO classification | 0.005 | ||
A | 15 | 1 | |
AB | 76 | 3 | |
B1 | 35 | 4 | |
B2 | 36 | 4 | |
B3 | 12 | 5 | |
C | 9 | 4 | |
Operation | <0.001 | ||
Non-VATS operation | 46 | 15 | |
VATS operation | 137 | 6 | |
Collected image slice (slices) | 63.22±11.87 | 61.76±11.65 | 0.735 |
ROI slice in 3D segmentation (slices) | 9.81±3.49 | 9.54±4.43 | 0.231 |
Data are expressed as mean ± SD or frequency. WHO, World Health Organization; VATS, video-assisted thoracic surgery; SD, standard deviation; ROI, region of interest.
Feature selection and establishment of the radiomics model
In the 2D segmentation, 3D segmentation, and 2D + 3D segmentation, 1,354, 1,344, and 2,698 features were extracted, respectively; among these, 648, 728, and 1,376 features with high stability (ICC >0.8) were identified, respectively. Radiomics features for vascular inseparable TETs were selected using the LASSO model, and 20, 16, and 24 features were retained in the 2D, 3D, and 2D + 3D models, respectively (Table 2 and Figures 3-5).
Table 2
Feature | Coefficient |
---|---|
2D segmentation model | |
original_shape_Elongation | −0.10011205 |
log-sigma-2-0-mm-2D_firstorder_90Percentile | 0.01284269 |
log-sigma-2-0-mm-2D_glcm_Imc1 | −0.10431494 |
log-sigma-2-0-mm-2D_glrlm_ShortRunHighGrayLevelEmphasis | −0.08237211 |
log-sigma-2-0-mm-2D_glrlm_ShortRunLowGrayLevelEmphasis | 0.05194436 |
log-sigma-2-0-mm-2D_glszm_GrayLevelVariance | 0.0078466 |
log-sigma-5-0-mm-2D_glcm_Autocorrelation | 0.0078466 |
log-sigma-5-0-mm-2D_glcm_Id | 0.00351167 |
log-sigma-5-0-mm-2D_glrlm_ShortRunLowGrayLevelEmphasis | 0.135122 |
wavelet-LLH_glcm_ClusterShade | −0.02100059 |
wavelet-LLH_glcm_SumSquares | 0.04015058 |
wavelet-LLH_gldm_HighGrayLevelEmphasis | 0.09444635 |
wavelet-LLH_gldm_SmallDependenceLowGrayLevelEmphasis | −0.02332301 |
wavelet-LLH_glszm_ZonePercentage | −0.03189313 |
wavelet-LHL_glcm_ClusterShade | −0.05094128 |
wavelet-LHL_glrlm_LongRunHighGrayLevelEmphasis | 0.07348392 |
wavelet-LHH_glszm_SmallAreaLowGrayLevelEmphasis | −0.07796041 |
wavelet-HLH_glcm_Imc2 | −0.03515943 |
wavelet-HLH_glszm_SmallAreaEmphasis | −0.02972123 |
wavelet-HLH_glszm_SmallAreaLowGrayLevelEmphasis | −0.0398179 |
3D segmentation model | |
original_shape_Sphericity | −0.02572743 |
log-sigma-1-0-mm-3D_glcm_ClusterShade | −0.03052404 |
log-sigma-1-0-mm-3D_glrlm_ShortRunHighGrayLevelEmphasis | −0.06850541 |
log-sigma-1-0-mm-3D_glszm_ZoneEntropy | 0.10999916 |
log-sigma-2-0-mm-3D_firstorder_90Percentile | 0.11341436 |
log-sigma-2-0-mm-3D_firstorder_Range | 0.0003084 |
log-sigma-2-0-mm-3D_glcm_Imc1 | −0.06719718 |
log-sigma-3-0-mm-3D_glcm_DifferenceVariance | −0.06686246 |
log-sigma-3-0-mm-3D_glrlm_ShortRunHighGrayLevelEmphasis | −0.03303138 |
log-sigma-4-0-mm-3D_gldm_LargeDependenceLowGrayLevelEmphasis | −0.14331302 |
log-sigma-5-0-mm-3D_glcm_SumEntropy | −0.10301179 |
wavelet-LLH_glszm_SizeZoneNonUniformity | 0.046551 |
wavelet-LLH_glszm_SizeZoneNonUniformityNormalized | 0.02108074 |
wavelet-LLH_glszm_ZoneEntropy | −0.09888323 |
wavelet-HHH_firstorder_Kurtosis | 0.10056921 |
wavelet-LLL_firstorder_Maximum | 0.07155026 |
2D + 3D segmentation model | |
2D features | |
original_shape_Elongation | 0.06653255 |
log-sigma-1-0-mm-2D_glcm_ClusterShade | −0.08421055 |
log-sigma-1-0-mm-2D_glszm_ZoneEntropy | 0.01628199 |
log-sigma-2-0-mm-2D_firstorder_Minimum | −0.06231016 |
log-sigma-2-0-mm-2D_glcm_Imc1 | −0.00321245 |
log-sigma-3-0-mm-2D_glcm_DifferenceEntropy | −0.05915957 |
log-sigma-3-0-mm-2D_glrlm_ShortRunHighGrayLevelEmphasis | −0.04130194 |
log-sigma-4-0-mm-2D_gldm_LargeDependenceLowGrayLevelEmphasis | −0.06126603 |
log-sigma-5-0-mm-2D_glcm_JointEntropy.1 | −0.01378587 |
wavelet-HLH_glcm_ClusterTendency | 0.0444266 |
wavelet-HHH_firstorder_Kurtosis | 0.06211867 |
wavelet-LLL_firstorder_Maximum | 0.05245455 |
3D features | |
original_shape_Elongation | −0.1055529 |
log-sigma-1-0-mm-3D_firstorder_90Percentile | 0.0798177 |
log-sigma-2-0-mm-3D_glcm_Correlation | 0.13683448 |
log-sigma-5-0-mm-3D_glcm_DifferenceEntropy | −0.03972627 |
log-sigma-5-0-mm-3D_glrlm_ShortRunLowGrayLevelEmphasis | 0.07684226 |
wavelet-LLH_glcm_ClusterShade | −0.12886596 |
wavelet-LLH_glcm_SumSquares | 0.06104846 |
wavelet-LHL_glszm_HighGrayLevelZoneEmphasis | −0.04477988 |
wavelet-LHH_gldm_DependenceVariance | 0.07270085 |
wavelet-LHH_glrlm_RunVariance | 0.04231245 |
wavelet-HLL_gldm_DependenceEntropy | 0.07390282 |
wavelet-HLL_glszm_ZoneEntropy | 0.00640498 |
glcm, gray-level co-occurrence matrix; glrlm, gray-level run-length matrix; glszm, gray-level size zone matrix; gldm, gray-level dependence matrix; L (in the wavelet filters), low-pass filter; H (in the wavelet filters), high-pass filter; 2D, two-dimensional; 3D, three-dimensional.
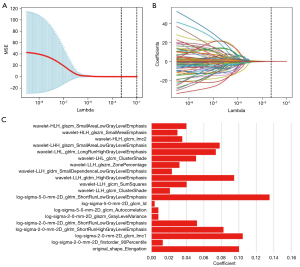
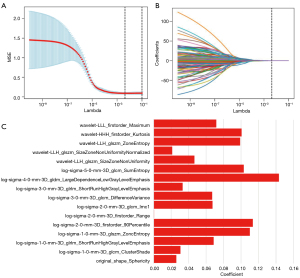
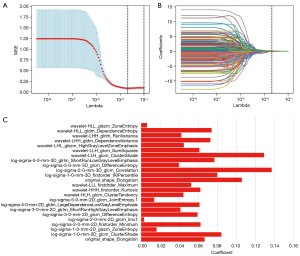
In the 2D model, 1 first-order feature, 1 morphological feature, and 18 texture features were selected. The 3D model included 4 first-order features, 1 morphological feature, and 11 texture features. In the 2D + 3D model, 3 first-order features, 1 morphological feature, and 8 texture features were selected in the 2D feature group, while 1 first-order feature, 1 morphological feature, and 10 textures were selected in the 3D feature group. The AUCs of these models (2D model, 3D model, and 2D + 3D model) were 94.1%, 91.7%, and 94.6% in the training cohort, and 95.0%, 91.6%, and 97.7% in the testing cohort, respectively. The AUC of the clinical imaging diagnosis was 0.868 and 0.777 in training and testing sets, respectively (Table 3). The CT images of some representative TETs cases are shown in Figure 6, including a separable TET (Figure 6A) and an inseparable TET (Figure 6B) misdiagnosed by radiologists but diagnosed correctly by the radiomics model. The radiomics models showed significant improvement compared with the radiological model, with P value of <0.001, 0.012, and 0.013 for the 2D, 3D and 2D + 3D models, respectively (Figures 7,8). However, no significant differences were observed among the 3 radiomics models. The P value of the Delong test between the 2D model and 3D model was 0.109, and the P values between the 2D with the 2D + 3D and the 3D with the 2D + 3D were 0.087 and 0.263, respectively.
Table 3
Models | Group | AUC (95% CI) | Sensitivity (%) | Specificity (%) | Significance level (Delong test vs. radiologists’ diagnosis) |
---|---|---|---|---|---|
Radiological model | Train | 0.868 (0.826, 0.910) | 77.8 | 96.0 | – |
Test | 0.777 (0.671, 0.883) | 66.7 | 82.1 | – | |
2D segmentation | Train | 0.941 (0.917, 0.975) | 98.3 | 88.2 | Z score =3.395; P=0.001 |
Test | 0.950 (0.918, 0.997) | 96.9 | 88.9 | Z score =3.600; P<0.001 | |
3D segmentation | Train | 0.917 (0.863, 0.943) | 98.3 | 79.2 | Z score =3.059; P=0.002 |
Test | 0.916 (0.859, 0.965) | 96.9 | 87.9 | Z score =2.524; P=0.012 | |
2D + 3D segmentation | Train | 0.946 (0.935, 0.985) | 98.3 | 92.9 | Z score =3.040; P=0.002 |
Test | 0.977 (0.950, 0.992) | 97.9 | 91.2 | Z score =2.493; P=0.013 |
AUC, area under curve; CI, confidence interval; 2D, two-dimensional; 3D, three-dimensional.
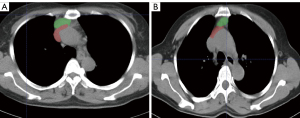
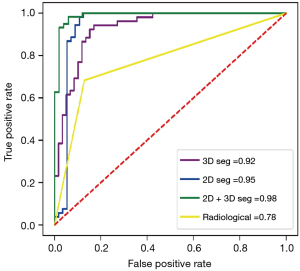
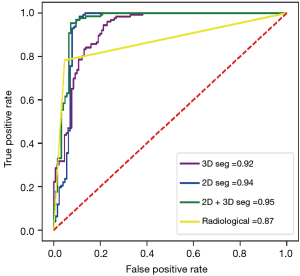
Nomogram building and validation
The nomogram was constructed based on the clinical image features and radscore to visualize the results of multivariable logistic regression analysis for vascular inseparable TETs (Figure 9). Ultimately, 3 clinical imaging features and a radscore were included in the nomogram, and the univariable and multivariable analyses of the significant features in the univariate logistic regression and radscore are shown in Table 4 and Table 5. In both the training and testing sets, the calibration curves and Hosmer-Lemeshow test of this nomogram indicated high accuracy. The ROC plot was drawn to show the sensitivity, specificity, and accuracy of the nomogram (Figure 10). However, the ROC plot of nomogram did not indicate there to be a significant improvement with the 3 radiomics models in the Delong test (P=0.297 for the 2D model, P=0.260 for 3D model, P=0.105 for 2D + 3D model).
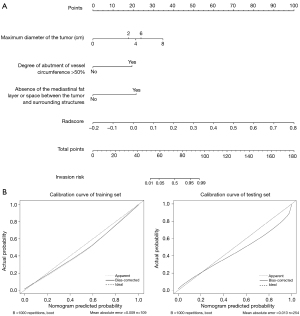
Table 4
Features | Z score (Wald test) | P value |
---|---|---|
Sex (male) | −1.65 | 0.099 |
Age (years) | 1.80 | 0.073 |
Maximum diameter of the tumor | ||
0–2 cm | 3.19 | 0.001 |
2–4 cm | 4.53 | <0.001 |
4–6 cm | 5.76 | <0.001 |
6–8 cm | 2.54 | 0.011 |
Calcification | 3.43 | <0.001 |
Lobulated | 6.99 | 0.001 |
Heterogeneous | 7.79 | <0.001 |
Degree of abutment of vessel circumference >50% | 8.55 | <0.001 |
Irregularity of the tumor contour and/or irregular interface | 4.78 | <0.001 |
Absence of the mediastinal fat layer or space between the tumor and surrounding structures | 5.85 | <0.001 |
Table 5
Features | Z score (Wald test) | P value |
---|---|---|
Maximum diameter of the tumor | ||
0–2 cm | 2.11 | 0.035 |
2–4 cm | 2.53 | 0.014 |
4–6 cm | 2.46 | 0.014 |
6–8 cm | 3.10 | 0.002 |
Calcification | 0.82 | 0.413 |
Lobulated | −0.31 | 0.756 |
Heterogeneous | −1.63 | 0.103 |
Degree of abutment of vessel circumference >50% | 3.09 | 0.002 |
Irregularity of the tumor contour and/or irregular interface | 3.53 | >0.001 |
Absence of the mediastinal fat layer or space between the tumor and surrounding structures | 3.53 | >0.001 |
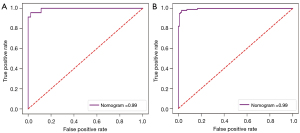
Discussion
Currently, the preferred treatment for patients with TETs with a mediastinal large vein is neoadjuvant therapy to facilitate tumor shrinkage before surgical resection (26-28). If the surgeon is unable to separate the tumor and great vein, additional angioplasty may be necessary. Therefore, an accurate preoperative prediction of surgical inseparable TETs could help surgeons to choose the most suitable treatment modality to plan their surgical approach preoperatively, thus avoiding unexpected intraoperative events. This study aimed to develop a radiomics analysis based on preoperative CT images capable of predicting surgically inseparable TETs. We had the additional objective of comparing the effectiveness of the radiomics models and radiologists’ diagnosis in predicting vascularly inseparable TETs. Our study demonstrated that the preoperative prediction using radiomics analysis based on NECT images could achieve a relatively high level of accuracy. In addition, the radiomics models and the nomogram, which combine image and radiomics features, demonstrated significant improvement over the radiologists’ diagnosis. Therefore, radiomics analysis can assist in preoperatively assessing the risk of surgery and determining a suitable surgical approach for patients with TETs.
CT and MRI are commonly used to assist in determining the tumor size, malignancy, and extent of invasion to adjacent organs. Few studies have been conducted on evaluating the spatial relationship between TETs and the surrounding vein using CT images. Kuriyama et al. used the length of the tumor vessel interface and the maximum diameter of the tumor to judge vascular invasion based on these tumor characteristics and achieved good accuracy (AUC =0.764) (9). In our study, clinical diagnosis achieved a similar accuracy (AUC =0.777). We used radiomics methods to establish relevant models by analyzing the first-order, shape, and texture features of the images. The results suggested that the accuracy of these models was better than that of semantic features (Table 3). Furthermore, the comparison with the evaluation of radiologists indicated that the greatest advantage of the radiomics model is its higher sensitivity. This is important for clinical practice in avoiding recommending the operation of TETs that cannot be separated during the operation, thus reducing the operation risk. Through observation of the judgment of the radiomics model and radiologists, we found that 52.9% of the tumors were distant from the veins (108/204). Further observation revealed that differences in prediction ability between the radiological model and the radiologist were mostly likely to occur in cases where the tumor boundary and vein boundary were not very clear. For TETs that are obviously distant from the vein, the prediction ability of the radiologist was similar to that of the radiomics model. In addition, since the semantic features were judged by radiologists, the difference in individual experience between doctors might have led to heterogeneity and instability. However, the radiomics model had the characteristics of stability, so the diagnostic efficacy and relevance of radiomics model were greater compared to those of semantic features.
In this study, it was found that the GLRLM, GLCM, and GLSZM features in the 2D segmentation model were strongly correlated with vascularly inseparable TETs. As the vascular invasion of TETs was the predominant reason for inseparable TETs, the selected features can be considered as representing the vascular aggressiveness of the tumor. GLRLM-small runs low gray-level emphasis indicates that the texture is dominated by short runs of low gray-levels, leading to increased roughness. This suggests that when the internal roughness of the tumor increases, the likelihood of vascular invasion also increases. Similarly, GLCM-informational measure of correlation 1 (IMC1) quantifies the complexity of texture using gray-level co-occurrence entropy, reflecting the correlation of gray values around voxels. Higher values indicate that the gray values around voxels are more similar, resulting in a more uniform image. Therefore, GLCM-IMC1 was negatively correlated with vascular invasion in the three models in this study, and the strong correlation observed in this study between GLSZM-zone entropy and vascular invasion further confirms this point. A study on superior mesenteric vein invasion in pancreatic ductal adenocarcinoma reached similar conclusions (29). Additionally, several tumors were inseparable due to tight adhesion with veins, resulting in unsafe separation. This might have been due to the intratumoral heterogeneity being associated with the inflammatory response of tumor microenvironment. Greater intratumoral heterogeneity may lead to greater inflammation in the tumor microenvironment and inseparable adhesions. However, there is currently no relevant literature to support this point, and more targeted studies are required.
Most of the studies related to TETs conducted thus far have focused on CECT images. While CECT does offer advantages over NECT images for visual observation in the extraction of semantic features, the results of CECT are not always stable due to the variability in distribution and injection sites of contrast agents. Some studies on radiomics analysis related to TETs have suggested that the accuracy of NECT is not inferior to that of CECT (30,31). Furthermore, we have observed that contrast agents can cause an allergic reaction in some patients. Therefore, the use of the radiomics model based on NECT can provide more reliable and valuable clinical data for these patients.
At present, the optimal method of lesion segmentation in radiomics analysis remains controversial. While some researchers believe that performing multilayer segmentation can obtain more complete lesion information, others argue that one-layer segmentation is more efficient, less complex, and repeatable (32,33). In a CT radiomics study, a 2D segmentation model demonstrated a significant advantage over the 3D segmentation model in predicting lymphovascular invasion in lung adenocarcinoma. Another study which employed positron emission tomography-CT (PET-CT) radiomics analysis to predict lymphovascular invasion in lung cancer found there to be no significant difference between the 2D segmentation model and the 3D segmentation model (32,33). In this study, the AUCs obtained by the 2D segmentation model in the training set and test set were 0.941 and 0.950, respectively, and those of the 3D segmentation model were 0.917 and 0.916, respectively. In the Delong test, no significant difference was found between these models. Therefore, our study suggests that the 2D segmentation model does not lose much information compared with the 3D segmentation model in predicting vascular inseparable TETs.
A nomogram can illustrate the results of multivariable logistic regression. In our study, the nomogram incorporated 3 image features: maximum diameter of the tumor, abutment degree of the vessel circumference, and absence of the mediastinal fat layer or space between the tumor and surrounding structures. Two of these features are conventional imaging diagnostic criteria. However, the nomogram indicated that the imaging features held less weight in determining the final total score, which was primarily determined by the radscore. In the comparison of the ROC curves of the nomogram model and those of the pure radiomics models, no significant difference was found. This may be explained by the fact that the radiomics characteristics partly reflected some information regarding the tumor boundary, rendering the use of radiomics model alone sufficient in predicting whether the doctor can separate the tumor from the blood vessels during the operation.
Some limitations to our study should be noted. First, a single-center design was employed, and both the training set and the test set were obtained from West China Hospital of Sichuan University, which limits the generalizability of the findings. In addition, more models and feature selection methods could have been introduced in this study, as our study only adopted radiomics methods for predicting the vascularly inseparable TETs. Given that this method can be applied to other research fields, our study should be generalized conservatively.
Conclusions
Overall, this study evaluated the ability for a radiomics approach to predict those TETs that would be vascularly inseparable in an operation. Data analysis revealed that compared to the radiologists diagnosis, the radiomics model was significantly superior in predicting the vascularly inseparable TETs.
Acknowledgments
Funding: None.
Footnote
Reporting Checklist: The authors have completed the TRIPOD reporting checklist. Available at https://qims.amegroups.com/article/view/10.21037/qims-22-1050/rc
Conflicts of Interest: All authors have completed the ICMJE uniform disclosure form (available at https://qims.amegroups.com/article/view/10.21037/qims-22-1050/coif). The authors have no conflicts of interest to declare.
Ethical Statement: The authors are accountable for all aspects of the work in ensuring that questions related to the accuracy or integrity of any part of the work are appropriately investigated and resolved. This study was approved by the Ethics Committee of West China Hospital, Sichuan University (No. 2021-578) and was conducted in accordance with the Declaration of Helsinki (as revised in 2013). Individual consent for this retrospective analysis was waived.
Open Access Statement: This is an Open Access article distributed in accordance with the Creative Commons Attribution-NonCommercial-NoDerivs 4.0 International License (CC BY-NC-ND 4.0), which permits the non-commercial replication and distribution of the article with the strict proviso that no changes or edits are made and the original work is properly cited (including links to both the formal publication through the relevant DOI and the license). See: https://creativecommons.org/licenses/by-nc-nd/4.0/.
References
- Marom EM. Advances in thymoma imaging. J Thorac Imaging 2013;28:69-80; quiz 81-3. [Crossref] [PubMed]
- Marx A, Chan JK, Coindre JM, Detterbeck F, Girard N, Harris NL, Jaffe ES, Kurrer MO, Marom EM, Moreira AL, Mukai K, Orazi A, Ströbel P. The 2015 World Health Organization Classification of Tumors of the Thymus: Continuity and Changes. J Thorac Oncol 2015;10:1383-95. [Crossref] [PubMed]
- Friedant AJ, Handorf EA, Su S, Scott WJ. Minimally Invasive versus Open Thymectomy for Thymic Malignancies: Systematic Review and Meta-Analysis. J Thorac Oncol 2016;11:30-8. [Crossref] [PubMed]
- Ruffini E, Filosso PL, Guerrera F, Lausi P, Lyberis P, Oliaro A. Optimal surgical approach to thymic malignancies: New trends challenging old dogmas. Lung Cancer 2018;118:161-70. [Crossref] [PubMed]
- Raza A, Woo E. Video-assisted thoracoscopic surgery versus sternotomy in thymectomy for thymoma and myasthenia gravis. Ann Cardiothorac Surg 2016;5:33-7. [Crossref] [PubMed]
- Zhang Y, Lin D, Aramini B, Yang F, Chen X, Wang X, Wu L, Huang W, Fan J. Thymoma and Thymic Carcinoma: Surgical Resection and Multidisciplinary Treatment. Cancers (Basel) 2023;15:1953. [Crossref] [PubMed]
- Ahmed SA, Mourad AF, Hassan RA, Ibrahim MAE, Soliman A, Aboeleuon E, Elbadee OMA, Hetta HF, Jabir MA. Preoperative CT staging of borderline pancreatic cancer patients after neoadjuvant treatment: accuracy in the prediction of vascular invasion and resectability. Abdom Radiol (NY) 2021;46:280-9. [Crossref] [PubMed]
- Lapshyn H, Schulte T, Sondermann S, May K, Petrova E, Honselmann KC, Braun R, Zemskov S, Keck T, Wellner UF, Bausch D, Bolm L. Radiological prediction of portal vein infiltration in patients with pancreatic ductal adenocarcinoma. Pancreatology 2021;21:155-62. [Crossref] [PubMed]
- Kuriyama S, Imai K, Ishiyama K, Takashima S, Atari M, Matsuo T, Ishii Y, Harata Y, Sato Y, Motoyama S, Nomura K, Hashimoto M, Minamiya Y. Using CT to evaluate mediastinal great vein invasion by thymic epithelial tumors: measurement of the interface between the tumor and neighboring structures. Eur Radiol 2022;32:1891-901. [Crossref] [PubMed]
- Chetan MR, Gleeson FV. Radiomics in predicting treatment response in non-small-cell lung cancer: current status, challenges and future perspectives. Eur Radiol 2021;31:1049-58. [Crossref] [PubMed]
- Dalal V, Carmicheal J, Dhaliwal A, Jain M, Kaur S, Batra SK. Radiomics in stratification of pancreatic cystic lesions: Machine learning in action. Cancer Lett 2020;469:228-37. [Crossref] [PubMed]
- Kocher M, Ruge MI, Galldiks N, Lohmann P. Applications of radiomics and machine learning for radiotherapy of malignant brain tumors. Strahlenther Onkol 2020;196:856-67. [Crossref] [PubMed]
- Sun Y, Li C, Jin L, Gao P, Zhao W, Ma W, Tan M, Wu W, Duan S, Shan Y, Li M. Radiomics for lung adenocarcinoma manifesting as pure ground-glass nodules: invasive prediction. Eur Radiol 2020;30:3650-9. [Crossref] [PubMed]
- Yan M, Wang W. A radiomics model of predicting tumor volume change of patients with stage III non-small cell lung cancer after radiotherapy. Sci Prog 2021;104:36850421997295. [Crossref] [PubMed]
- Yu Y, He Z, Ouyang J, Tan Y, Chen Y, Gu Y, et al. Magnetic resonance imaging radiomics predicts preoperative axillary lymph node metastasis to support surgical decisions and is associated with tumor microenvironment in invasive breast cancer: A machine learning, multicenter study. EBioMedicine 2021;69:103460. [Crossref] [PubMed]
- Blüthgen C, Patella M, Euler A, Baessler B, Martini K, von Spiczak J, Schneiter D, Opitz I, Frauenfelder T. Computed tomography radiomics for the prediction of thymic epithelial tumor histology, TNM stage and myasthenia gravis. PLoS One 2021;16:e0261401. [Crossref] [PubMed]
- Chen X, Feng B, Li C, Duan X, Chen Y, Li Z, Liu Z, Zhang C, Long W. A radiomics model to predict the invasiveness of thymic epithelial tumors based on contrast-enhanced computed tomography. Oncol Rep 2020;43:1256-66. [Crossref] [PubMed]
- Liu J, Yin P, Wang S, Liu T, Sun C, Hong N. CT-Based Radiomics Signatures for Predicting the Risk Categorization of Thymic Epithelial Tumors. Front Oncol 2021;11:628534. [Crossref] [PubMed]
- Ohira R, Yanagawa M, Suzuki Y, Hata A, Miyata T, Kikuchi N, Yoshida Y, Yamagata K, Doi S, Ninomiya K, Tomiyama N. CT-based radiomics analysis for differentiation between thymoma and thymic carcinoma. J Thorac Dis 2022;14:1342-52. [Crossref] [PubMed]
- Ozawa Y, Hara M, Shibamoto Y. The impact of radiomics in predicting oncologic behavior of thymic epithelial tumors. Mediastinum 2019;3:27. [Crossref] [PubMed]
- Rajamohan N, Goyal A, Kandasamy D, Bhalla AS, Parshad R, Jain D, Sharma R. CT texture analysis in evaluation of thymic tumors and thymic hyperplasia: correlation with the international thymic malignancy interest group (ITMIG) stage and WHO grade. Br J Radiol 2021;94:20210583. [Crossref] [PubMed]
- Xiao G, Rong WC, Hu YC, Shi ZQ, Yang Y, Ren JL, Cui GB. MRI Radiomics Analysis for Predicting the Pathologic Classification and TNM Staging of Thymic Epithelial Tumors: A Pilot Study. AJR Am J Roentgenol 2020;214:328-40. [Crossref] [PubMed]
- Zhang C, Yang Q, Lin F, Ma H, Zhang H, Zhang R, Wang P, Mao N. CT-Based Radiomics Nomogram for Differentiation of Anterior Mediastinal Thymic Cyst From Thymic Epithelial Tumor. Front Oncol 2021;11:744021. [Crossref] [PubMed]
- van Griethuysen JJM, Fedorov A, Parmar C, Hosny A, Aucoin N, Narayan V, Beets-Tan RGH, Fillion-Robin JC, Pieper S, Aerts HJWL. Computational Radiomics System to Decode the Radiographic Phenotype. Cancer Res 2017;77:e104-7. [Crossref] [PubMed]
- He H, Bai Y, Garcia EA, Li S. ADASYN: Adaptive synthetic sampling approach for imbalanced learning. 2008 IEEE International Joint Conference on Neural Networks (IEEE World Congress on Computational Intelligence); 01-08 June 2008; Hong Kong. IEEE; 2008:1322-8.
- Bertolaccini L, Prisciandaro E, Galetta D, Casiraghi M, Guarize J, Petrella F, Sedda G, Lo Iacono G, Brambilla D, Spaggiari L. Outcomes and Safety Analysis in Superior Vena Cava Resection for Extended Thymic Epithelial Tumors. Ann Thorac Surg 2021;112:271-7. [Crossref] [PubMed]
- Li Z, Wu X, Zhao Y, Xiao Y, Zhao Y, Zhang T, Li H, Sha F, Wang Y, Deng L, Ma X. Clinical benefit of neoadjuvant anti-PD-1/PD-L1 utilization among different tumors. MedComm (2020) 2021;2:60-8. [Crossref] [PubMed]
- Toker A, Hayanga JWA, Dhamija A, Kaba E, Ozkan B, Ayalp K, Cimenoglu B, Abbas G. Superior Vena Cava Reconstruction in Masaoka Stage III and IVa Thymic Epithelial Tumors. Ann Thorac Surg 2022;113:1882-90. [Crossref] [PubMed]
- Chen F, Zhou Y, Qi X, Zhang R, Gao X, Xia W, Zhang L. Radiomics-Assisted Presurgical Prediction for Surgical Portal Vein-Superior Mesenteric Vein Invasion in Pancreatic Ductal Adenocarcinoma. Front Oncol 2020;10:523543. [Crossref] [PubMed]
- Feng XL, Wang SZ, Chen HH, Huang YX, Xin YK, Zhang T, Cheng DL, Mao L, Li XL, Liu CX, Hu YC, Wang W, Cui GB, Nan HY. Optimizing the radiomics-machine-learning model based on non-contrast enhanced CT for the simplified risk categorization of thymic epithelial tumors: A large cohort retrospective study. Lung Cancer 2022;166:150-60. [Crossref] [PubMed]
- Wang X, Sun W, Liang H, Mao X, Lu Z. Radiomics Signatures of Computed Tomography Imaging for Predicting Risk Categorization and Clinical Stage of Thymomas. Biomed Res Int 2019;2019:3616852. [Crossref] [PubMed]
- Yang G, Nie P, Zhao L, Guo J, Xue W, Yan L, Cui J, Wang Z. 2D and 3D texture analysis to predict lymphovascular invasion in lung adenocarcinoma. Eur J Radiol 2020;129:109111. [Crossref] [PubMed]
- Shen C, Liu Z, Guan M, Song J, Lian Y, Wang S, Tang Z, Dong D, Kong L, Wang M, Shi D, Tian J. 2D and 3D CT Radiomics Features Prognostic Performance Comparison in Non-Small Cell Lung Cancer. Transl Oncol 2017;10:886-94. [Crossref] [PubMed]