Diagnostic accuracy of glioma pseudoprogression identification with positron emission tomography imaging: a systematic review and meta-analysis
Introduction
Pseudoprogression (PsP) of glioma generally refers to a phenomenon of mimicking tumor progression, which is a consequence of a subacute treatment-related local tissue reaction, and can be caused by inflammation, edema, and increased permeability of the blood-brain barrier (BBB) (1,2). Studies in recent years have reported an incidence of glioma PsP exceeding 20% due to the increase of nonsurgical treatment, such as radiotherapy, chemotherapy, antiangiogenic therapy, checkpoint inhibitor immunotherapy, and targeted therapy (3-6). Importantly, there are substantial ramifications for patients and clinicians when PsP is not identified, as the appearance of the PsP phenomenon usually indicates the efficacy of early treatment, which may be associated with a better prognosis (7). In contrast, for those patients with true progression (TPR), it is necessary to terminate the current treatment and perform reoperation or make a new treatment plan.
At present, pathologic confirmation is still considered the most reliable method to differentiate PsP from TPR in glioma. However, to avoid unnecessary surgery, most clinicians evaluate the progression pattern according to the Response Assessment in Neuro-Oncology (RANO) criteria (2). Specifically, a diagnosis of TPR should be made when progressive contrast-enhancing lesions are noted on initial magnetic resonance imaging (MRI) and when further progression of contrast enhancement ensues at least 4 weeks later. By contrast, a diagnosis of PsP should be applied when follow-up MRI shows stabilization or regression of the contrast-enhanced lesions. Obviously, the extended period of follow-up from suspicious progression to the final diagnosis of PsP or TPR after first treatment of glioma poses a problem. Therefore, prognosis could be improved if the pattern of glioma progression could be identified at the earliest possible moment when clinicians first suspect progression.
Positron emission tomography (PET) imaging as a promising molecular neuroimaging technique that by using various radiotracers, could provide information on the metabolic glucose, amino acid, and lipid content of gliomas (8). In recent years, it has repeatedly been demonstrated that PET imaging with radiotracers such as 2-18F-fluoro-2-deoxy-D-glucose (18F-FDG), O-(2-18F-fluoroethyl)-L-tyrosine (18F-FET), (S-11C-methyl)-L-methionine (11C-MET), and 3,4-dihydroxy-6-18F-fluoro-L-phenylalanine (18F-FDOPA) has good diagnostic value for distinguishing PsP from TPR (9-12). Moreover, PET imaging has been proposed as a criterion for glioma management in addition to MRI, as stated in the joint guidelines of the RANO working group and recent joint European Association of Nuclear Medicine (EANM) and European Association of Neuro-Oncology (EANO) (13,14) recommendations. However, the sensitivity and accuracy of PET imaging with different radiotracers in identifying glioma PsP are controversial or unknown (15,16). Meanwhile, the challenge of demonstrating that radiotherapy planning based on PET is superior to traditional planning either in the first-line or in the recurrent setting remains unresolved (17). Therefore, it is necessary to conduct a meta-analysis to comprehensively evaluate the diagnostic performance of PET in distinguishing PsP from TPR.
Our meta-analysis is not the first attempt to verify the diagnostic value of PET imaging for glioma prognosis. However, previous studies only investigated the accuracy of PET imaging in distinguishing posttreatment-related changes from TPR and did not consider PsP from other treatment-related changes for specifical analysis (18-20). In order to objectively and realistically investigate the accuracy of PET imaging in distinguishing PsP from TPR, a stricter set of inclusion criteria was formulated for this meta-analysis. Specifically, all eligible patients were required to be suspected of glioma progression during the post therapeutic follow-up and ultimately defined as PsP or TPR according to the RANO criteria (2). We present this article in accordance with the PRISMA-DTA reporting checklist (21) (available at https://qims.amegroups.com/article/view/10.21037/qims-22-1340/rc).
Methods
The study protocol was prospectively registered in PROSPERO (International Prospective Register of Systematic Reviews; https://www.crd.york.ac.uk) under registration number CRD42022372687.
Literature search strategy
A systematic search for potentially relevant articles was conducted in 5 international databases (Medline, Web of Science, Embase, Cochrane Library, and ClinicalTrials.gov) from inception until September 1, 2022. The keywords and medical subject headings (MeSH) terms were combined as follows: “glioma” and “PET” and “pseudoprogression” or “progression”. The retrieval formula is provided in Appendix 1. To include all eligible studies in the search, no language restriction was applied. Moreover, references provided from relevant articles were also manually examined to identify additional studies for inclusion.
Literature selection
The inclusion criteria for the literature were as follows: (I) clinical diagnostic test using PET imaging to distinguish PsP and TPR in patients with either adult or pediatric glioma; (II) patients suspected of glioma progression during the post therapeutic follow-up; (III) a progression pattern clearly distinguished in each case with the RANO criteria (2).
The exclusion criteria were as follows: (I) informal publication types (e.g., reviews or meta-analyses, clinical guideline, case reports, conference abstracts, letters to the editor, or comments); (II) cell or animal experiments; (III) with a sample size ≤10 patients; (IV) with insufficient data to obtain or deduce a 2×2 confusion matrix or the unavailability of the absolute numbers of true-positive (TP), false-positive (FP), true-negative (TN) and false-negative (FN) cases; (V) duplicate or overlapping cohorts (in the case of an overlapping cohort, the largest was enrolled and other overlapping studies were excluded); and (VI) inclusion of radiation necrosis or other treatment-related changes.
To minimize subjectivity, screening was completed by 2 independent reviewers (ZQ Ouyang, GR Zheng) and any discrepancies were adjudicated by a third reviewer (CD Liao).
Data extraction and quality assessment
The evaluation was based on a scale of 17 items. Quality assessment was performed independently by 2 reviewers (ZQ Ouyang, GR Zheng) using RevMan software (version 5.4, Cochrane; https://training.cochrane.org) based on the Quality Assessment of Diagnostic Accuracy Studies 2 (QUADAS-2) (22). The QUADAS-2 tool can assign a risk of bias rating of “low”, “high”, or “uncertain” based on a response of “yes”, “no”, or “uncertain” to the relevant flag questions included in each section. Specifically, if the answer to all the landmark questions in a range is “yes”, then it can be rated as low risk of bias; if all the informational questions are answered “no”, then the risk of bias is rated as “high” (23). Any disagreements in scoring were resolved through discussion.
Data extraction of the included studies proceeded according to a predesigned form as follows: (I) basic information of studies (author name, journal, year of publication, country of origin, study design, and begin and end time of investigation); (II) patients’ demographic and clinical characteristics (mean or median age, sample size, therapeutic regimen, World Health Organization (WHO) classification, O6-methylguanine-DNA methyl-transferase (MGMT) and isocitrate dehydrogenase [IDH(status)]; (III) PET protocol (PET modality, radiotracer type, radiotracer dose, time of PET scan after tracer injection, method of analysis, parameters, and cutoff value); and (IV) recorded or calculated absolute numbers of TP, FP, FN, and TN. The data extraction form was first piloted on 3 randomly selected studies and then completed for all items by 1 reviewer (ZQ Ouyang) and validated by another reviewer (CD Liao) to ensure a level of accuracy. The PET parameter with the highest accuracy of each study was selected. The screening protocol mandated that quantitative parameters take precedence over qualitative parameters, combined parameters, textural parameters, and radiomics parameters.
Statistical analysis
First, we evaluated the threshold effect by using Meta-Disc software (version 1.4; https://meta-disc.software.informer.com). In brief, the Spearman correlation coefficient between the logarithm of sensitivity and the logarithm of 1 − specificity was calculated to evaluate the threshold effect. This indicated that there was an obvious threshold effect when the Spearman correlation coefficient was >0.8 and P<0.05.
In Stata software (version MP17.0, StataCorp; https://www.stata.com), the pooled sensitivity, specificity, positive likelihood ratio (PLR), negative likelihood ratio (NLR), and diagnostic odds ratio (DOR) with their 95% confidence intervals (CIs) were calculated and presented using the random effects model. In addition, the hierarchical logistic regression model was used to generate the summary receiver operating characteristic (SROC) curve and area under the curve (AUC) for evaluating diagnostic accuracy.
The Q test was evaluated to determine the between-study heterogeneity, while the discordance index (I2) was adopted as a measure of heterogeneity. Higgins et al. (24) suggest that heterogeneity be assessed as low, medium, and high, with upper limits for I2 of 25%, 50%, and 75%, respectively. For this meta-analysis, the potential influencing factors of heterogeneity included sample size (the included studies were divided by the median of sample size) (25), study design, WHO grade, gold standard, and radiotracer type. Therefore, meta-regression and subgroup analyses were performed to explore and explain the source of heterogeneity. Moreover, sensitivity analysis was performed to evaluate how robust the results were. The funnel plot was used to explore potential publication bias.
Results
Study selection
Figure 1 shows the PRISMA flowchart of this meta-analysis. A total of 1,293 records were identified, 4 of which (9,26-28) were manually retrieved from other sources. First, 401 records were removed due to being duplicates, and another 540 records were excluded based on the type of screening. The 352 remaining studies underwent tile and abstract eligibility assessment, among which 270 studies were excluded due to missing keywords. Then, the 82 remaining studies underwent full-text eligibility assessment, which culminated in a total inclusion of 20 studies for analysis in this review. The total number of included patients was 1,018, comprising 317 (30.9%) with PsP and 708 (69.1%) with TPR (the total number of included patients did not match the sum of PsP and TPR cases, as some patients were included twice in Ullrich et al.’s (29) study, while the other 2 patients were excluded from Skoblar Vidmar et al.’s (30) study for unknown reasons). The sample sizes ranged from 12 to 151 patients, and the median sample size was 40. The male to female ratio was about 1.6:1, and ages ranged from 2 to 83 years. There were 177 (17.4%) low-grade gliomas (LGGs; WHO I–II), 262 (25.7%) WHO grade III gliomas, 529 (52.0%) WHO grade IV gliomas, and 50 (4.9%) gliomas that were not specified. Among the included patients, 594 underwent detection for MGMT methylation status, and the ratio of methylated to unmethylated was about 1.4:1. Regarding IDH mutation status, the ratio of wild type to mutant type was 1.8:1.
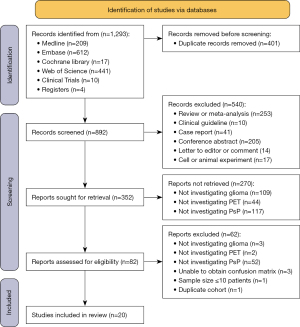
Study characteristics
Table 1 (9-12,16,26-40) demonstrates the characteristics of the included studies. Among the 20 included studies, 3 studies (11,12,31) had a prospective design. All studies used the RANO criteria as the gold standard to identify glioma PsP, with 14 studies (9-12,26,28,30-37) using pathology and MRI follow-up, 2 studies (27,29) using pathology as the sole reference standard, and 4 studies (16,38-40) using MRI follow-up alone. The most commonly evaluated radiotracer was 18F-FET; in addition, 3 studies tested 11C-MET (11,29,32), 2 tested 18F-FDOPA (12,16), and 1 tested 18F-FDG (9). Each study reported the treatment schemes of patients with glioma to different degrees. Except for the patients from 2 studies (28,35), in which the enrolled patients underwent additional targeted drug, immune checkpoint inhibitor, or tumor-treating field therapy, most of the patients underwent neurosurgery, radiotherapy, chemotherapy, or a combination thereof. Other characteristics of the included studies can be found in Table S1.
Table 1
First author | Nation | Year | Study design | Sample size (male number) | Age [range], years | WHO classification | TPR/PsP | Reference standard (RANO) | Radiotracer type | Quantitative parameter | Cutoff | TP | FP | FN | TN |
---|---|---|---|---|---|---|---|---|---|---|---|---|---|---|---|
Bag (32) | USA | 2022 | Retrospective | 27 (n=16) | 14# [2–25] | III (n=23), IV (n=4) | 22/5 | Pathology & MRI | 11C-MET | MTV | 0.98 cm2 | 4 | 2 | 1 | 20 |
Galldiks (27) | GER | 2013 | Retrospective | 27 (n=19) | 44# [11–64] | II (n=27) | 18/9 | Pathology | 18F-FET | Relative changes of TBRmax | 33% | 8 | 5 | 1 | 13 |
Galldiks (10) | GER | 2015 | Retrospective | 22 (n=14) | 56# [34–76] | IV (n=22) | 11/11 | Pathology & MRI | 18F-FET | TBRmax | 2.3 | 11 | 1 | 0 | 10 |
Imani (9) | USA | 2014 | Retrospective | 12 (n=5) | 39* [25–70] | II (n=6), III (n=6) | 5/7 | Pathology & MRI | 18F-FDG | nSUVmax | 1.9 | 6 | 1 | 1 | 4 |
Kebir (38) | GER | 2016 | Retrospective | 26 (n=21) | 56* [23–76] | IV (n=26) | 19/7 | MRI | 18F-FET | TBRmax | 1.9 | 6 | 3 | 1 | 16 |
Kebir (39) | GER | 2017 | Retrospective | 14 (n=9) | 52* [29–70] | III (n=3), IV (n=11) | 10/4 | MRI | 18F-FET | TBRmax | 2.1 | 4 | 3 | 0 | 7 |
Kebir (40) | GER | 2020 | Retrospective | 44 (n=34) | 55* [34–79] | IV (n=44) | 30/14 | MRI | 18F-FET | TBRmean | 1.82 | 5 | 0 | 9 | 30 |
Kertels (33) | GER | 2019 | Retrospective | 36 (n=22) | 54# [24–75] | IV (n=36) | 28/8 | Pathology & MRI | 18F-FET | TBRmax1 | 3.44 | 7 | 4 | 1 | 24 |
Lohmann (31) | GER | 2020 | Prospective | 34 (n=21) | 57# [24–79] | III (n=1), IV (n=33) | 18/16 | Pathology & MRI | 18F-FET | TBRmax | 2.25 | 13 | 6 | 3 | 12 |
Maurer (28) | GER | 2020 | Retrospective | 127 (n=83) | 50# [20–78] | II (n=21), III (n=36), IV (n=68), NS (n=2) | 94/33 | Pathology & MRI | 18F-FET | TBRmax | 1.95 | 23 | 28 | 10 | 66 |
Müller (26) | GER | 2022 | Retrospective | 151 (n=97) | 52* [20–78] | II (n=28), III (n=40), IV (n=83) | 114/37 | Pathology & MRI | 18F-FET | TBRmean + TBRmax | NA | 8 | 9 | 4 | 37 |
Paprottka (34) | GER | 2021 | Retrospective | 74 (n=41) | 55* [NR] | II (n=4), III (n=19), IV (n=51) | 57/17 | Pathology & MRI | 18F-FET | TBRmean | 2 | 14 | 11 | 3 | 46 |
Pellerin (12) | FRA | 2021 | Prospective | 58 (n=34) | 53# [NR] | II (n=10), III (n=21), IV (n=27) | 34/24 | Pathology & MRI | 18F-FDOPA | T-map and isocontour map | NA | 22 | 2 | 2 | 32 |
Skoblar Vidmar (30) | SI | 2022 | Retrospective | 44 (n=27) | 44* [17–72] | NS (n=44) | 31/11 | Pathology & MRI | 18F-FET | TBRmax | 3.03 | 9 | 7 | 2 | 24 |
Skvortsova (11) | RUS | 2014 | Prospective | 72 (n=35) | 36* [3–68] | I (n=17), II (n=17), III (n=34), NS (n=4) | 30/42 | Pathology & MRI | 11C-MET | TBRmax | 1.9 | 41 | 5 | 1 | 25 |
Steidl (35) | GER | 2021 | Retrospective | 104 (n=68) | 52* [20–78] | II (n=10), III (n=24), IV (n=70) | 83/21 | Pathology & MRI | 18F-FET | Slope | 0.69 SUV/h | 13 | 13 | 8 | 70 |
Ullrich (29) | GER | 2009 | Retrospective | 24 (n=14) | 40# [NR] | II (n=18), III (n=6) | 20/13 | Pathology | 11C-MET | Relative changes of TBR | 14.6% | 12 | 2 | 1 | 18 |
Werner (36) | GER | 2019 | Retrospective | 48 (n=29) | 50# [20–83] | II (n=1), III (n=8), IV (n=39) | 38/10 | Pathology & MRI | 18F-FET | TBRmax | 1.95 | 10 | 8 | 0 | 30 |
Werner (37) | GER | 2021 | Retrospective | 23 (n=13) | 58# [38–71] | IV (n=23) | 12/11 | Pathology & MRI | 18F-FET | TBRmean | 1.95 | 9 | 1 | 2 | 11 |
Zaragori (16) | FRA | 2020 | Retrospective | 51 (n=28) | 51* [21–75] | II (n=18), III (n=8), IV (n=25) | 34/17 | MRI | 18F-FDOPA | TBRmax | 1.61 | 16 | 1 | 1 | 33 |
The studies are arranged by the first author’ s last name. #, mean age; *, median age. 11C-MET, (S-11C-methyl)-L-methionine; 18F-FDG, 2-18F-fluoro-2-deoxy-D-glucose; 18F-FDOPA, 3,4-dihydroxy-6-18F-fluoro-L-phenylalanine; 18F-FET, O-(2-18F-fluoroethyl)-L-tyrosine; FN, false negative; FP, false positive; MRI, magnetic resonance imaging; MTV, metabolic tumor volume; NA, not applicable; NR, not reported; NS, not specified; nSUV, standardized uptake values of the lesion divided by standardized uptake values of the normal white matter; PsP, pseudoprogression; SUV, standardized uptake value; TBRmax, maximum tumor-to-brain ratio; TBRmean, mean tumor-to-brain ratio; TN, true negative; TP, true positive; TPR, true glioma progression; WHO, World Health Organization.
Quality assessment
The QUADAS-2 evaluation of the included studies is shown in Figure 2. The 2 independent reviewers agreed that most of the studies did not specify whether patients were enrolled from a random group or a consecutive group, whether the results of PET or the gold standard were interpreted based on the double-blind method, or whether there was an appropriate interval between PET scanning and application of the gold standard, resulting in the risk of bias for patient selection, index test, reference standard, and flow and timing being unclear in 11 (55%), 15 (75%), 18 (90%), and 15 studies (75%), respectively (Table 2).
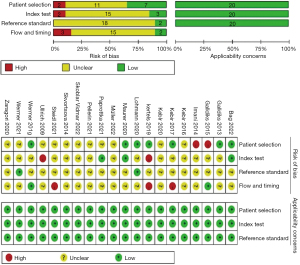
Table 2
Subgroup | Number of studies | Pooled AUC (95% CI) | Pooled sensitivity (95% CI) | P1 | Pooled specificity (95% CI) | P2 |
---|---|---|---|---|---|---|
Sample size | 0.59 | <0.001 | ||||
<40 patients | 10 | 0.92 (0.89–0.94) | 0.90 (0.82–0.98) | 0.83 (0.76 to 0.91) | ||
≥40 patients | 10 | 0.91 (0.88–0.93) | 0.82 (0.73–0.92) | 0.85 (0.79 to 0.90) | ||
Study design | 0.79 | 0.04 | ||||
Prospective | 3 | NA | 0.93 (0.85–1.00) | 0.84 (0.73 to 0.95) | ||
Retrospective | 17 | 0.90 (0.87–0.92) | 0.83 (0.75–0.91) | 0.84 (0.80 to 0.89) | ||
WHO grade | 0.09 | 0.01 | ||||
HGG | 8 | 0.90 (0.87–0.93) | 0.83 (0.70–0.96) | 0.87 (0.81 to 0.93) | ||
LGG and HGG | 12 | 0.92 (0.89–0.94) | 0.87 (0.79–0.95) | 0.83 (0.78 to 0.88) | ||
Gold standard | 0.37 | <0.001 | ||||
Pathology & MRI | 14 | 0.89 (0.86–0.91) | 0.86 (0.78–0.94) | 0.82 (0.77 to 0.87) | ||
Pathology or MRI | 6 | 0.96 (0.93–0.97) | 0.85 (0.71–0.99) | 0.89 (0.83 to 0.95) | ||
Amino acid radiotracer | 0.65 | 0.60 | ||||
Yes | 19 | 0.91 (0.88–0.93) | 0.86 (0.79–0.93) | 0.84 (0.80 to 0.89) | ||
No | 1 | NA | 0.88 (0.57–1.00) | 0.81 (0.44 to 1.00) | ||
18F-FET PET | <0.001 | <0.001 | ||||
Yes | 14 | 0.86 (0.83–0.89) | 0.80 (0.68–0.88) | 0.81 (0.75 to 0.85) | ||
No | 6 | 0.97 (0.95–0.98) | 0.93 (0.87–0.97) | 0.91 (0.85 to 0.95) |
18F-FET, O-(2-18F-fluoroethyl)-L-tyrosine; AUC, area under curve; CI, confidence interval; HGG, high-grade glioma; LGG, low-grade glioma; MRI, magnetic resonance imaging; NA, not applicable; PET, positron emission tomography; WHO, World Health Organization.
Threshold effect evaluation
In the correlation analysis, the Spearman correlation coefficient was −0.059 between the logarithm of sensitivity and the logarithm of 1 − specificity (P=0.806). In other words, there was no threshold effect for the included studies, and the potential heterogeneity of the included studies was not caused by the threshold effect. This confirmed that the ensuing meta-analysis was feasible and valuable.
Diagnostic accuracy
Before pooling diagnostic values, we screened the PET parameters of each study according to the principle of data extraction. Among all included studies, the most effective parameter was the maximum tumor-to-brain ratio (TBRmax) in 10 studies (10,11,16,28,30,31,33,36,38,39), the mean tumor-to-brain ratio (TBRmean) in 3 studies (34,37,40), and the relative change of TBR in 2 studies (27,29). In the 5 remaining studies (9,12,26,32,35), the most effective parameter was the metabolic tumor volume (MTV), slope, standardized uptake values of the lesion divided by standardized uptake values of the normal (nSUVmax), radiomics signature, and combined parameter (Table 1), respectively. The summarized diagnostic performance of PET is shown in Table 2. The sensitivity and specificity of PET for identifying glioma PsP ranged from 0.00 to 1.00 and from 0.40 to 1.00, respectively, with a summary sensitivity of 0.86 (95% CI: 0.77–0.91) and a summary specificity of 0.84 (95% CI: 0.79–0.88), as shown in Figure 3. The corresponding PLR, NLR, and DOR were 5.47 (95% CI: 4.10–7.31), 0.17 (95% CI: 0.10–0.27), and 32.52 (95% CI: 16.89–62.64), respectively (Figures S1,S2). The SROC is shown in Figure 4A. As illustrated, the summary the AUCs of the included studies was 0.91 (95% CI: 0.88–0.93).
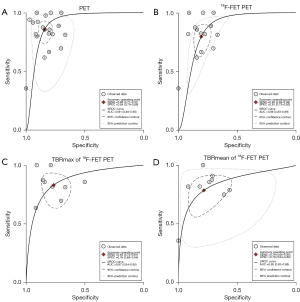
Regarding 18F-FET PET, the summary sensitivity, specificity, and AUC were 0.80 (95% CI: 0.68–0.88) and 0.81 (95% CI: 0.75–0.85), and 0.86 (95% CI: 0.83–0.89), respectively (Figure 4B, Table 2). In addition, the 2 most frequently used quantitative parameters for PsP identification were the TBRmax (cutoff value range: 1.9 to 3.44; median value: 2.3) and TBRmean (cutoff value range: 1.82 to 2.19; median value: 1.95); for these 2 quantitative parameters, the summary sensitivity was 0.83 (95% CI: 0.72–0.91) and 0.79 (95% CI: 0.65–0.88), respectively, while the specificity was 0.76 (95% CI: 0.68–0.84) and 0.78 (95% CI: 0.64–0.88), respectively; the SROCs with AUCs of 0.87 (95% CI: 0.84–0.90) and 0.85 (95% CI: 0.82–0.88), respectively, are shown in Figure 4C,4D.
Heterogeneity analysis
The I2 of the summary sensitivity and specificity of 63.39% and 53.51% (Figure 3), respectively, indicated obvious heterogeneity among the included studies. Therefore, meta-regression and subgroup analysis were applied to explore the sources of heterogeneity. The results demonstrated that radiotracer type (18F-FET vs. non-18F-FET) was the only factor for heterogeneity of summary sensitivity (P<0.05) (Figure 5). As for summary specificity, the sources of heterogeneity mainly included sample size, study design, WHO grade, gold standard, and radiotracer type (18F-FET vs. non-18F-FET) (P<0.05) (Figure 5). In the subgroup analysis, the diagnostic power of 18F-FET PET for identifying PsP was significantly lower than that of non-18F-FET, with summary AUCs, sensitivities, and specificities of 0.80 vs. 0.93, 0.81 vs. 0.91, and 0.86 vs. 0.97, respectively (Table 2). Furthermore, sensitivity analysis found that the main source of heterogeneity was the 2020 study of Kebir et al. (40). The heterogeneity of summary sensitivity and specificity decreased to 44.54% and 44.23% (Figure S3) when this study was removed.
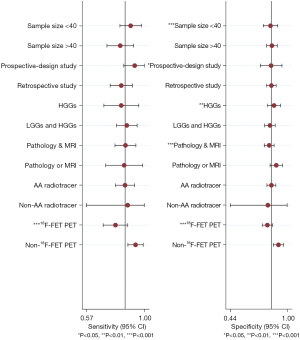
Publication bias
The Deeks’ funnel plot shows a symmetrical shape in Figure 6, suggesting no significant publication bias among the included studies (P=0.57).
Discussion
The use of noninvasive methods for the timely and accurate differentiation of PsP from TPR in glioma remains challenging (17,41). The detection and comparison of metabolic differences between tumor and normal brain tissue with PET imaging has been considered a potential clinical means to differentiating PsP from TPR (9-12). Therefore, we conducted a meta-analysis of 1,018 patients with glioma in 20 related studies. The results demonstrated that the incidence of PsP was 30.9% in patients who were suspected of progression after treatment, which is close to the incidence of 30% to 39.5% reported in other studies (4,42,43). An aggregated high diagnostic potential of PET for identifying PsP was determined using SROC analysis, with the summary sensitivity, specificity, and AUC being 0.86, 0.84 and 0.91, respectively. However, there was obvious heterogeneity among the included studies, which compelled us to explore the possible sources of heterogeneity.
First, the results of Spearman correlation analysis indicated that the heterogeneity was not caused by the threshold effect. Subsequently, meta-regression and subgroup analysis revealed that sample size, study design, WHO grade, and gold standard were the main sources of summary specificity heterogeneity. The influence of studies with an adequate number of participants was slightly higher than that of the smaller-scale studies (0.85 vs. 0.83; P<0.05). Empirically, smaller samples cannot provide meaningful estimates of accuracy (18,44). Although we excluded the case reports and studies with sample sizes ≤10 patients, the heterogeneity of the summary specificity due to the higher risk of selection bias in the small-sample studies could not be avoided, as confirmed by the quality assessment of the included studies. In addition, compared with the studies which only included patients with high-grade gliomas (HGGs; WHO III–IV), the summary specificity of those studies which enrolled patients with LGGs (WHO I–II) and HGGs was lower (0.87 vs. 0.83; P<0.05). We believe this may be attributable to an increase in radiotracer uptake with increasing glioma grade (45-49). Usually, HGGs have a greater tumor cell density, microvascular density, and more efficient amino acid transport system, which can more quickly absorb amino acid radioactive tracers from blood pools (50,51). Moreover, HGGs tend to lead to more serious damage to the BBB. Although the disruption to the BBB is not a prerequisite for intratumoral accumulation (52), more serious damage to the BBB will lead to passive inflow of radiotracers and aggravate the original high uptake state in HGGs (49,53). Therefore, the difference of radiotracer metabolism between those with TPR and PsP is more obvious in HGGs than in LGGs, and static or multidynamic amino acid PET imaging can more accurately distinguish false progress from true progress in HGGs. Finally, the meta-regression showed that the summary sensitivity and specificity of non-18F-FET PET were significantly higher than those of 18F-FET PET (sensitivity: 0.93 vs. 0.80, P<0.001; specificity: 0.91 vs. 0.81, P<0.001). However, there were only six eligible studies with non-18F-FET radiotracer including one 18F-FDG study (9), three 11C-MET studies (11,29,32) and two 18F-FDOPA studies (12,16). Obviously, the present evidence is insufficiently robust to prove that the summary accuracy of imaging using other radiotracers for identification of glioma PsP is superior to that of 18F-FET imaging. Because the apparently superior diagnostic accuracy, this was based on a small amount of diagnostic data and should be interpreted with caution (45).
Interest into the value of each radiotracer in PET imaging for differentiating PsP from TPR has grown. 18F-FET is one of the first 18F-labeled amino acids that can be produced in large amounts for scientific investigation and clinical practice (54,55). 18F-FET is transported via a system of L-type amino acid transporters (LATs), particularly the subtypes LAT1 and LAT2; is not significantly incorporated into any metabolic pathway; and has no relevant participation in protein synthesis (37,56). It should be noted that overexpression of LAT1 is common in gliomas, which simultaneously facilitates the influx and causes the entrapment of 18F-FET in tumor cells to form a high uptake state (57). In contrast, the expression of LAT1 is normal or even downregulated in tissue affected by post therapeutic changes, and active transport of amino acid tracers into PsP tissue should be equal or less than of that into normal brain tissue. Increased 18F-FET uptake in PsP tissue, therefore, could only result from the passive influx of 18F-FET and has lower intensity compared with the 18F-FET uptake in TPR (58). Our meta-analysis, provides sufficient evidence to show that 18F-FET PET imaging displays a high accuracy for differentiating PsP from TPR, with a summary sensitivity and specificity of 0.80 and 0.81, respectively. Although our summary specificity is slightly lower than that reported in similar meta-analyses (19,20), this should not prevent clinicians from suggesting patients with glioma undergo 18F-FET examination when the progression pattern is unclear during follow-up. Of course, which parameter of 18F-FET PET should be selected to discriminate PsP from TPR in clinical practice remains a problem (33,39). A subgroup analysis based on nine 18F-FET PET studies (10,28,30,31,33,36-38,40) was performed, and the result being similar to that of previous studies (19), in that TBRmax and TBRmean were found to have a similar diagnostic efficiency, according to the summary sensitivities (0.83 vs. 0.79), specificities (0.76 vs. 0.78), and AUCs (0.87 vs. 0.85). Therefore, TBRmax and TBRmean should be equally considered when differentiating PsP from TPR using 18F-FET PET imaging.
In this meta-analysis, we attempted to determine the diagnostic accuracy of other radiotracers for identification of PsP in addition to 18F-FET PET. Unfortunately, the true diagnostic performances of non-18F-FET PET are uncertain due to the insufficient amount of data. 18F-FDG is a classic radiotracer and used in tumor imaging, but it is not ideal for detecting gliomas due to the high physiologic uptake in normal brain tissue (59-61). Nevertheless, a retrospective small-sample study by Imani et al. (9) revealed the potential value of 18F-FDG PET imaging for identifying PsP using a semiquantitative parameter (nSUVmax), with the sensitivity, specificity, and accuracy being 0.86, 0.80, and 0.83 respectively. Additionally, 11C-MET is the most widely used radiotracer for amino acid PET (62), and 3 included studies showed that 11C-MET PET has excellent value for the identification of PsP: the diagnostic accuracy ranged from 0.89 to 0.92 and was markedly higher than that of 18F-FET PET (11,29,32). Finally, 18F-FDOPA, similarly to 18F-FET and 11C-MET, is transported intact through the BBB via LAT transporters (63). It has shown promising results in a small number of studies and could be comparable or perhaps superior to 18F-FET and 11C-MET in terms of identifying PsP (12,16).
Regarding the direction and prospect of future investigation, first, the diagnostic value of non-18F-FET radiotracers need to be proved in additional cohorts. Second, only a few preliminary studies have shown that the highest diagnostic accuracy would be achieved from the combination of advanced multiparameter MRI and PET imaging (9,12,34,36,64), and therefore future research should focus on the development and validation of such bimodal or multimodal diagnostic models (65). In addition, the kinetic parameters of dynamic PET imaging have demonstrated excellent performance in glioma grading (66) and prognosis evaluation (16,35,38,40). However, irregular dynamic PET scanning limits the clinical popularization of kinetic parameters (67,68). Therefore, researchers need to devote more energy to developing and verifying better dynamic PET scanning protocols. Finally, radiomics, as a novel imaging analysis technique, can not only extract thousands of quantitative features from routinely acquired images (69) but can also link them to the genotypic and phenotypic characteristics of the tissue at the genetic and molecular level (70,71). Two recent studies have already demonstrated that PET-based radiomics is a potential method for identifying PsP in glioma (26,31). In the future, researchers should perform radiomics analysis based on existing data to fully leverage the information from medical images while exploring better diagnostic models.
There are some limitations to our meta-analysis. First, most of the included studies were retrospective, but they did not specify the source of patients in detail, thus introducing selection bias as indicated in the QUADAS-2 evaluation. In addition, only a few studies mentioned the use of blinding when gold standard or quantitative parameters were used for measurement. Undoubtedly, this further increased the risk bias. Second, the applied bivariate regression method cannot be used for multivariate evaluation. Third, we set a stricter set of inclusion criteria than did previous studies, and thus many publications related to the differential diagnosis of therapy-related changes were not considered. There were only 6 studies on non-18F-FET PET, including studies on 18F-FDG, 11C-MET, and 18F-FDOPA PET. The diagnostic accuracy of these PET imaging modalities could be calculated with the random effects model and therefore must be verified in the future when data are sufficient. Fourth, most of the included studies failed to provide a detailed treatment course of each patient, so we could not conduct subgroup analysis to explore the potential heterogeneity caused by different treatments, such as radiotherapy, temozolomide chemotherapy, checkpoint inhibitor immunotherapy, or targeted therapy. Finally, the status of MGMT methylation is an independent predictor of glioma PsP, and a previous study reported a 3.5-fold increased probability of a patient developing PsP if the MGMT promoter is methylated in glioma (72). Therefore, future studies are needed to clarify how MGMT methylation status in PET imaging affects the diagnosis of PsP in glioma.
Conclusions
This meta-analysis demonstrated that PET imaging with the inclusion of 18F-FDG PET, 18F-FET PET, 11C-MET PET, and 18F-FDOPA PET has a generally high accuracy for differentiating between PsP and TPR. Considering the credibility of the meta-evidence and the practicability of radiotracers, 18F-FET PET holds the highest value for clinical implementation. In addition, TBRmax and TBRmean should be equally considered as reliable parameters when using 18F-FET PET to identify glioma PsP. Although PET with non-18F-FET radiotracers showed better accuracy in identifying PsP, this needs to be confirmed with further research.
Acknowledgments
Funding: This work was supported by the National Natural Science Foundation of China (No. 82060313 and No. 82160340).
Footnote
Reporting Checklist: The authors have completed the PRISMA-DTA reporting checklist. Available at https://qims.amegroups.com/article/view/10.21037/qims-22-1340/rc
Conflicts of Interest: All authors have completed the ICMJE uniform disclosure form (available at https://qims.amegroups.com/article/view/10.21037/qims-22-1340/coif). The authors have no conflicts of interest to declare.
Ethical Statement: The authors are accountable for all aspects of the work in ensuring that questions related to the accuracy or integrity of any part of the work are appropriately investigated and resolved.
Open Access Statement: This is an Open Access article distributed in accordance with the Creative Commons Attribution-NonCommercial-NoDerivs 4.0 International License (CC BY-NC-ND 4.0), which permits the non-commercial replication and distribution of the article with the strict proviso that no changes or edits are made and the original work is properly cited (including links to both the formal publication through the relevant DOI and the license). See: https://creativecommons.org/licenses/by-nc-nd/4.0/.
References
- Brandsma D, van den Bent MJ. Pseudoprogression and pseudoresponse in the treatment of gliomas. Curr Opin Neurol 2009;22:633-8. [Crossref] [PubMed]
- Wen PY, Macdonald DR, Reardon DA, Cloughesy TF, Sorensen AG, Galanis E, Degroot J, Wick W, Gilbert MR, Lassman AB, Tsien C, Mikkelsen T, Wong ET, Chamberlain MC, Stupp R, Lamborn KR, Vogelbaum MA, van den Bent MJ, Chang SM. Updated response assessment criteria for high-grade gliomas: response assessment in neuro-oncology working group. J Clin Oncol 2010;28:1963-72. [Crossref] [PubMed]
- van West SE, de Bruin HG, van de Langerijt B, Swaak-Kragten AT, van den Bent MJ, Taal W. Incidence of pseudoprogression in low-grade gliomas treated with radiotherapy. Neuro Oncol 2017;19:719-25. [PubMed]
- Lu VM, Welby JP, Laack NN, Mahajan A, Daniels DJ. Pseudoprogression after radiation therapies for low grade glioma in children and adults: A systematic review and meta-analysis. Radiother Oncol 2020;142:36-42. [Crossref] [PubMed]
- Galldiks N, Kocher M, Ceccon G, Werner JM, Brunn A, Deckert M, Pope WB, Soffietti R, Le Rhun E, Weller M, Tonn JC, Fink GR, Langen KJ. Imaging challenges of immunotherapy and targeted therapy in patients with brain metastases: response, progression, and pseudoprogression. Neuro Oncol 2020;22:17-30. [Crossref] [PubMed]
- Schwarzenberg J, Czernin J, Cloughesy TF, Ellingson BM, Pope WB, Grogan T, Elashoff D, Geist C, Silverman DH, Phelps ME, Chen W. Treatment response evaluation using 18F-FDOPA PET in patients with recurrent malignant glioma on bevacizumab therapy. Clin Cancer Res 2014;20:3550-9. [Crossref] [PubMed]
- Reardon DA, Weller M. Pseudoprogression: fact or wishful thinking in neuro-oncology? Lancet Oncol 2018;19:1561-3. [Crossref] [PubMed]
- la Fougère C, Suchorska B, Bartenstein P, Kreth FW, Tonn JC. Molecular imaging of gliomas with PET: opportunities and limitations. Neuro Oncol 2011;13:806-19. [Crossref] [PubMed]
- Imani F, Boada FE, Lieberman FS, Davis DK, Mountz JM. Molecular and metabolic pattern classification for detection of brain glioma progression. Eur J Radiol 2014;83:e100-5. [Crossref] [PubMed]
- Galldiks N, Dunkl V, Stoffels G, Hutterer M, Rapp M, Sabel M, Reifenberger G, Kebir S, Dorn F, Blau T, Herrlinger U, Hau P, Ruge MI, Kocher M, Goldbrunner R, Fink GR, Drzezga A, Schmidt M, Langen KJ. Diagnosis of Pseudoprogression in Patients with Glioblastoma Using O-(2-[18f]Fluoroethyl)-L-Tyrosine Pet. Eur J Nucl Med Mol Imaging 2015;42:685-95. [Crossref] [PubMed]
- Skvortsova TY, Brodskaya ZL, Gurchin AF. PET using 11C-methionine in recognition of pseudoprogression in cerebral glioma after combined treatment. Zh Vopr Neirokhir Im N N Burdenko 2014;78:50-8. [PubMed]
- Pellerin A, Khalifé M, Sanson M, Rozenblum-Beddok L, Bertaux M, Soret M, Galanaud D, Dormont D, Kas A, Pyatigorskaya N. Simultaneously acquired PET and ASL imaging biomarkers may be helpful in differentiating progression from pseudo-progression in treated gliomas. Eur Radiol 2021;31:7395-405. [Crossref] [PubMed]
- Albert NL, Weller M, Suchorska B, Galldiks N, Soffietti R, Kim MM, la Fougère C, Pope W, Law I, Arbizu J, Chamberlain MC, Vogelbaum M, Ellingson BM, Tonn JC. Response Assessment in Neuro-Oncology working group and European Association for Neuro-Oncology recommendations for the clinical use of PET imaging in gliomas. Neuro Oncol 2016;18:1199-208. [Crossref] [PubMed]
- Law I, Albert NL, Arbizu J, Boellaard R, Drzezga A, Galldiks N, la Fougère C, Langen KJ, Lopci E, Lowe V, McConathy J, Quick HH, Sattler B, Schuster DM, Tonn JC, Weller M. Joint EANM/EANO/RANO practice guidelines/SNMMI procedure standards for imaging of gliomas using PET with radiolabelled amino acids and [18F]FDG: version 1.0. Eur J Nucl Med Mol Imaging 2019;46:540-57. [Crossref] [PubMed]
- Brandes AA, Tosoni A, Spagnolli F, Frezza G, Leonardi M, Calbucci F, Franceschi E. Disease progression or pseudoprogression after concomitant radiochemotherapy treatment: pitfalls in neurooncology. Neuro Oncol 2008;10:361-7. [Crossref] [PubMed]
- Zaragori T, Ginet M, Marie PY, Roch V, Grignon R, Gauchotte G, Rech F, Blonski M, Lamiral Z, Taillandier L, Imbert L, Verger A. Use of static and dynamic (18)F-F-DOPA PET parameters for detecting patients with glioma recurrence or progression. EJNMMI Res 2020;10:56. [Crossref] [PubMed]
- Galldiks N, Niyazi M, Grosu AL, Kocher M, Langen KJ, Law I, Minniti G, Kim MM, Tsien C, Dhermain F, Soffietti R, Mehta MP, Weller M, Tonn JC. Contribution of PET imaging to radiotherapy planning and monitoring in glioma patients - a report of the PET/RANO group. Neuro Oncol 2021;23:881-93. [Crossref] [PubMed]
- Nihashi T, Dahabreh IJ, Terasawa T. Diagnostic accuracy of PET for recurrent glioma diagnosis: a meta-analysis. AJNR Am J Neuroradiol 2013;34:944-50, S1-11.
- Cui M, Zorrilla-Veloz RI, Hu J, Guan B, Ma X. Diagnostic Accuracy of PET for Differentiating True Glioma Progression From Post Treatment-Related Changes: A Systematic Review and Meta-Analysis. Front Neurol 2021;12:671867. [Crossref] [PubMed]
- de Zwart PL, van Dijken BRJ, Holtman GA, Stormezand GN, Dierckx RAJO, Jan van Laar P, van der Hoorn A. Diagnostic Accuracy of PET Tracers for the Differentiation of Tumor Progression from Treatment-Related Changes in High-Grade Glioma: A Systematic Review and Metaanalysis. J Nucl Med 2020;61:498-504. [Crossref] [PubMed]
- Page MJ, McKenzie JE, Bossuyt PM, Boutron I, Hoffmann TC, Mulrow CD, et al. The PRISMA 2020 statement: an updated guideline for reporting systematic reviews. BMJ 2021;372: [PubMed]
- Whiting PF, Rutjes AW, Westwood ME, Mallett S, Deeks JJ, Reitsma JB, Leeflang MM, Sterne JA, Bossuyt PM. QUADAS-2: a revised tool for the quality assessment of diagnostic accuracy studies. Ann Intern Med 2011;155:529-36. [Crossref] [PubMed]
- Bouwmeester W, Zuithoff NP, Mallett S, Geerlings MI, Vergouwe Y, Steyerberg EW, Altman DG, Moons KG. Reporting and methods in clinical prediction research: a systematic review. PLoS Med 2012;9:1-12. [Crossref] [PubMed]
- Higgins JP, Thompson SG, Deeks JJ, Altman DG. Measuring inconsistency in meta-analyses. BMJ 2003;327:557-60. [Crossref] [PubMed]
- Wu G, Song X, Liu J, Li S, Gao W, Qiu M, Yang C, Ma Y, Chen Y. Expression of CD44 and the survival in glioma: a meta-analysis. Biosci Rep 2020; [Crossref] [PubMed]
- Müller M, Winz O, Gutsche R, Leijenaar RTH, Kocher M, Lerche C, Filss CP, Stoffels G, Steidl E, Hattingen E, Steinbach JP, Maurer GD, Heinzel A, Galldiks N, Mottaghy FM, Langen KJ, Lohmann P. Static FET PET radiomics for the differentiation of treatment-related changes from glioma progression. J Neurooncol 2022;159:519-29. [Crossref] [PubMed]
- Galldiks N, Stoffels G, Ruge MI, Rapp M, Sabel M, Reifenberger G, Erdem Z, Shah NJ, Fink GR, Coenen HH, Langen KJ. Role of O-(2-18F-fluoroethyl)-L-tyrosine PET as a diagnostic tool for detection of malignant progression in patients with low-grade glioma. J Nucl Med 2013;54:2046-54. [Crossref] [PubMed]
- Maurer GD, Brucker DP, Stoffels G, Filipski K, Filss CP, Mottaghy FM, Galldiks N, Steinbach JP, Hattingen E, Langen KJ. (18)F-FET PET Imaging in Differentiating Glioma Progression from Treatment-Related Changes: A Single-Center Experience. J Nucl Med 2020;61:505-11. [Crossref] [PubMed]
- Ullrich RT, Kracht L, Brunn A, Herholz K, Frommolt P, Miletic H, Deckert M, Heiss WD, Jacobs AH. Methyl-L-11C-methionine PET as a diagnostic marker for malignant progression in patients with glioma. J Nucl Med 2009;50:1962-8. [Crossref] [PubMed]
- Skoblar Vidmar M, Doma A, Smrdel U, Zevnik K, Studen A. The Value of FET PET/CT in Recurrent Glioma with a Different IDH Mutation Status: The Relationship between Imaging and Molecular Biomarkers. Int J Mol Sci 2022;23:6787. [Crossref] [PubMed]
- Lohmann P, Elahmadawy MA, Gutsche R, Werner JM, Bauer EK, Ceccon G, Kocher M, Lerche CW, Rapp M, Fink GR, Shah NJ, Langen KJ, Galldiks N. FET PET Radiomics for Differentiating Pseudoprogression from Early Tumor Progression in Glioma Patients Post-Chemoradiation. Cancers (Basel) 2020;12:3835. [Crossref] [PubMed]
- Bag AK, Wing MN, Sabin ND, Hwang SN, Armstrong GT, Han Y, Li Y, Snyder SE, Robinson GW, Qaddoumi I, Broniscer A, Lucas JT, Shulkin BL. (11)C-Methionine PET for Identification of Pediatric High-Grade Glioma Recurrence. J Nucl Med 2022;63:664-71. [PubMed]
- Kertels O, Mihovilovic MI, Linsenmann T, Kessler AF, Tran-Gia J, Kircher M, Brumberg J, Monoranu CM, Samnick S, Ernestus RI, Löhr M, Meyer PT, Lapa C. Clinical Utility of Different Approaches for Detection of Late Pseudoprogression in Glioblastoma With O-(2-[18F]Fluoroethyl)-L-Tyrosine PET. Clin Nucl Med 2019;44:695-701. [Crossref] [PubMed]
- Paprottka KJ, Kleiner S, Preibisch C, Kofler F, Schmidt-Graf F, Delbridge C, Bernhardt D, Combs SE, Gempt J, Meyer B, Zimmer C, Menze BH, Yakushev I, Kirschke JS, Wiestler B. Fully automated analysis combining [18F]-FET-PET and multiparametric MRI including DSC perfusion and APTw imaging: a promising tool for objective evaluation of glioma progression. Eur J Nucl Med Mol Imaging 2021;48:4445-55. [Crossref] [PubMed]
- Steidl E, Langen KJ, Hmeidan SA, Polomac N, Filss CP, Galldiks N, Lohmann P, Keil F, Filipski K, Mottaghy FM, Shah NJ, Steinbach JP, Hattingen E, Maurer GD. Sequential Implementation of Dsc-Mr Perfusion and Dynamic [18f]Fet Pet Allows Efficient Differentiation of Glioma Progression from Treatment-Related Changes. Eur J Nucl Med Mol Imaging 2021;48:1956-65. [Crossref] [PubMed]
- Werner JM, Stoffels G, Lichtenstein T, Borggrefe J, Lohmann P, Ceccon G, Shah NJ, Fink GR, Langen KJ, Kabbasch C, Galldiks N. Differentiation of treatment-related changes from tumour progression: a direct comparison between dynamic FET PET and ADC values obtained from DWI MRI. Eur J Nucl Med Mol Imaging 2019;46:1889-901. [Crossref] [PubMed]
- Werner JM, Weller J, Ceccon G, Schaub C, Tscherpel C, Lohmann P, Bauer EK, Schäfer N, Stoffels G, Baues C, Celik E, Marnitz S, Kabbasch C, Gielen GH, Fink GR, Langen KJ, Herrlinger U, Galldiks N. Diagnosis of Pseudoprogression Following Lomustine-Temozolomide Chemoradiation in Newly Diagnosed Glioblastoma Patients Using FET-PET. Clin Cancer Res 2021;27:3704-13. [Crossref] [PubMed]
- Kebir S, Fimmers R, Galldiks N, Schäfer N, Mack F, Schaub C, Stuplich M, Niessen M, Tzaridis T, Simon M, Stoffels G, Langen KJ, Scheffler B, Glas M, Herrlinger U. Late Pseudoprogression in Glioblastoma: Diagnostic Value of Dynamic O-(2-[18f]Fluoroethyl)-L-Tyrosine Pet. Clin Cancer Res 2016;22:2190-6. [Crossref] [PubMed]
- Kebir S, Khurshid Z, Gaertner FC, Essler M, Hattingen E, Fimmers R, Scheffler B, Herrlinger U, Bundschuh RA, Glas M. Unsupervised Consensus Cluster Analysis of [18f]-Fluoroethyl-L-Tyrosine Positron Emission Tomography Identified Textural Features for the Diagnosis of Pseudoprogression in High-Grade Glioma. Oncotarget 2017;8:8294-304. [Crossref] [PubMed]
- Kebir S, Schmidt T, Weber M, Lazaridis L, Galldiks N, Langen KJ, Kleinschnitz C, Hattingen E, Herrlinger U, Lohmann P, Glas M. A Preliminary Study on Machine Learning-Based Evaluation of Static and Dynamic FET-PET for the Detection of Pseudoprogression in Patients with IDH-Wildtype Glioblastoma. Cancers (Basel) 2020;12:3080. [Crossref] [PubMed]
- Bogsrud TV, Londalen A, Brandal P, Leske H, Panagopoulos I, Borghammer P, Bach-Gansmo T. 18F-Fluciclovine PET/CT in Suspected Residual or Recurrent High-Grade Glioma. Clin Nucl Med 2019;44:605-11. [Crossref] [PubMed]
- Abbasi AW, Westerlaan HE, Holtman GA, Aden KM, van Laar PJ, van der Hoorn A. Incidence of Tumour Progression and Pseudoprogression in High-Grade Gliomas: a Systematic Review and Meta-Analysis. Clin Neuroradiol 2018;28:401-11. [Crossref] [PubMed]
- Zhang J, Wang Y, Wang Y, Xiao H, Chen X, Lei Y, Feng Z, Ma X, Ma L. Perfusion magnetic resonance imaging in the differentiation between glioma recurrence and pseudoprogression: a systematic review, meta-analysis and meta-regression. Quant Imaging Med Surg 2022;12:4805-22. [Crossref] [PubMed]
- Evangelista L, Guttilla A, Zattoni F, Muzzio PC, Zattoni F. Utility of choline positron emission tomography/computed tomography for lymph node involvement identification in intermediate- to high-risk prostate cancer: a systematic literature review and meta-analysis. Eur Urol 2013;63:1040-8. [Crossref] [PubMed]
- Taylor C, Ekert JO, Sefcikova V, Fersht N, Samandouras G. Discriminators of pseudoprogression and true progression in high-grade gliomas: A systematic review and meta-analysis. Sci Rep 2022;12:13258. [Crossref] [PubMed]
- Singhal T, Narayanan TK, Jacobs MP, Bal C, Mantil JC. 11C-methionine PET for grading and prognostication in gliomas: a comparison study with 18F-FDG PET and contrast enhancement on MRI. J Nucl Med 2012;53:1709-15. [Crossref] [PubMed]
- Nioche C, Soret M, Gontier E, Lahutte M, Dutertre G, Dulou R, Capelle L, Guillevin R, Foehrenbach H, Buvat I. Evaluation of quantitative criteria for glioma grading with static and dynamic 18F-FDopa PET/CT. Clin Nucl Med 2013;38:81-7. [Crossref] [PubMed]
- Youland RS, Kitange GJ, Peterson TE, Pafundi DH, Ramiscal JA, Pokorny JL, Giannini C, Laack NN, Parney IF, Lowe VJ, Brinkmann DH, Sarkaria JN. The role of LAT1 in (18)F-DOPA uptake in malignant gliomas. J Neurooncol 2013;111:11-8. [Crossref] [PubMed]
- Falk Delgado A, Falk Delgado A. Discrimination between primary low-grade and high-grade glioma with (11)C-methionine PET: a bivariate diagnostic test accuracy meta-analysis. Br J Radiol 2018;91:20170426. [Crossref] [PubMed]
- Wang M, Tang J, Liu S, Yoshida D, Teramoto A. Expression of cathepsin B and microvascular density increases with higher grade of astrocytomas. J Neurooncol 2005;71:3-7. [Crossref] [PubMed]
- Arita H, Kinoshita M, Kagawa N, Fujimoto Y, Kishima H, Hashimoto N, Yoshimine T. 11C-methionine uptake and intraoperative 5-aminolevulinic acid-induced fluorescence as separate index markers of cell density in glioma: a stereotactic image-histological analysis. Cancer 2012;118:1619-27. [Crossref] [PubMed]
- Stegmayr C, Oliveira D, Niemietz N, Willuweit A, Lohmann P, Galldiks N, Shah NJ, Ermert J, Langen KJ. Influence of Bevacizumab on Blood-Brain Barrier Permeability and O-(2-(18)F-Fluoroethyl)-l-Tyrosine Uptake in Rat Gliomas. J Nucl Med 2017;58:700-5. [Crossref] [PubMed]
- Okita Y, Kinoshita M, Goto T, Kagawa N, Kishima H, Shimosegawa E, Hatazawa J, Hashimoto N, Yoshimine T. (11)C-methionine uptake correlates with tumor cell density rather than with microvessel density in glioma: A stereotactic image-histology comparison. Neuroimage 2010;49:2977-82. [Crossref] [PubMed]
- Hamacher K, Coenen HH. Efficient routine production of the 18F-labelled amino acid O-2-18F fluoroethyl-L-tyrosine. Appl Radiat Isot 2002;57:853-6. [Crossref] [PubMed]
- Rapp M, Floeth FW, Felsberg J, Steiger HJ, Sabel M, Langen KJ, Galldiks N. Clinical Value of O-(2-[(18)F]-Fluoroethyl)-L-Tyrosine Positron Emission Tomography in Patients with Low-Grade Glioma. Neurosurg Focus 2013;34:E3. [Crossref] [PubMed]
- Langen KJ, Stoffels G, Filss C, Heinzel A, Stegmayr C, Lohmann P, Willuweit A, Neumaier B, Mottaghy FM, Galldiks N. Imaging of Amino Acid Transport in Brain Tumours: Positron Emission Tomography with O-(2-[(18)F]Fluoroethyl)-L-Tyrosine (Fet). Methods 2017;130:124-34. [Crossref] [PubMed]
- Habermeier A, Graf J, Sandhöfer BF, Boissel JP, Roesch F, Closs EI. System L amino acid transporter LAT1 accumulates O-(2-fluoroethyl)-L-tyrosine (FET). Amino Acids 2015;47:335-44. [Crossref] [PubMed]
- Pöpperl G, Götz C, Rachinger W, Gildehaus FJ, Tonn JC, Tatsch K. Value of O-(2-[18f]Fluoroethyl)- L-Tyrosine Pet for the Diagnosis of Recurrent Glioma. Eur J Nucl Med Mol Imaging 2004;31:1464-70. [Crossref] [PubMed]
- Langleben DD, Segall GM. PET in differentiation of recurrent brain tumor from radiation injury. J Nucl Med 2000;41:1861-7. [PubMed]
- Mehrkens JH, Pöpperl G, Rachinger W, Herms J, Seelos K, Tatsch K, Tonn JC, Kreth FW. The Positive Predictive Value of O-(2-[18f]Fluoroethyl)-L-Tyrosine (Fet) Pet in the Diagnosis of a Glioma Recurrence after Multimodal Treatment. J Neurooncol 2008;88:27-35. [Crossref] [PubMed]
- Xiao J, Jin Y, Nie J, Chen F, Ma X. Diagnostic and grading accuracy of (18)F-FDOPA PET and PET/CT in patients with gliomas: a systematic review and meta-analysis. BMC Cancer 2019;19:767. [Crossref] [PubMed]
- Herholz K, Langen KJ, Schiepers C, Mountz JM. Brain tumors. Semin Nucl Med 2012;42:356-70. [Crossref] [PubMed]
- Bund C, Heimburger C, Imperiale A, Lhermitte B, Chenard MP, Lefebvre F, Kremer S, Proust F, Namer IJ. FDOPA PET-CT of Nonenhancing Brain Tumors. Clin Nucl Med 2017;42:250-7. [Crossref] [PubMed]
- Barajas RF, Ambady P, Link J, Krohn KA, Raslan A, Mallak N, Woltjer R, Muldoon L, Neuwelt EA. [18F]-fluoromisonidazole (FMISO) PET/MRI hypoxic fraction distinguishes neuroinflammatory pseudoprogression from recurrent glioblastoma in patients treated with pembrolizumab. Neurooncol Pract 2022;9:246-50. [Crossref] [PubMed]
- Dhermain FG, Hau P, Lanfermann H, Jacobs AH, van den Bent MJ. Advanced MRI and PET imaging for assessment of treatment response in patients with gliomas. Lancet Neurol 2010;9:906-20. [Crossref] [PubMed]
- Girard A, Le Reste PJ, Metais A, Chaboub N, Devillers A, Saint-Jalmes H, Jeune FL, Palard-Novello X. Additive Value of Dynamic FDOPA PET/CT for Glioma Grading. Front Med (Lausanne) 2021;8:705996. [Crossref] [PubMed]
- Girard A, Saint-Jalmes H, Chaboub N, Le Reste PJ, Metais A, Devillers A, Le Jeune F, Palard-Novello X. Optimization of time frame binning for FDOPA uptake quantification in glioma. PLoS One 2020;15:e0232141. [Crossref] [PubMed]
- Girard A, François M, Chaboub N, Le Reste PJ, Devillers A, Saint-Jalmes H, Le Jeune F, Palard-Novello X. Impact of point-spread function reconstruction on dynamic and static (18)F-DOPA PET/CT quantitative parameters in glioma. Quant Imaging Med Surg 2022;12:1397-404. [Crossref] [PubMed]
- Gillies RJ, Kinahan PE, Hricak H. Radiomics: Images Are More than Pictures, They Are Data. Radiology 2016;278:563-77. [Crossref] [PubMed]
- Aerts HJ, Velazquez ER, Leijenaar RT, Parmar C, Grossmann P, Carvalho S, Bussink J, Monshouwer R, Haibe-Kains B, Rietveld D, Hoebers F, Rietbergen MM, Leemans CR, Dekker A, Quackenbush J, Gillies RJ, Lambin P. Decoding tumour phenotype by noninvasive imaging using a quantitative radiomics approach. Nat Commun 2014;5:4006. [Crossref] [PubMed]
- Shaikh F, Dupont-Roettger D, Dehmeshki J, Awan O, Kubassova O, Bisdas S. The Role of Imaging Biomarkers Derived From Advanced Imaging and Radiomics in the Management of Brain Tumors. Front Oncol 2020;10:559946. [Crossref] [PubMed]
- Balaña C, Capellades J, Pineda E, Estival A, Puig J, Domenech S, et al. Pseudoprogression as an adverse event of glioblastoma therapy. Cancer Med 2017;6:2858-66. [Crossref] [PubMed]