Deep learning radiomics for prediction of axillary lymph node metastasis in patients with clinical stage T1–2 breast cancer
Introduction
Breast cancer (BC) is the most frequently occurring cancer in women and a leading cause of cancer-related deaths (1). Surgeries for BC inevitably cause complications, such as postoperative scar tissue formation, limited range of motion of the upper limbs, and lymphatic circulation blockade-induced swelling in the upper limbs (2). Since BC metastasizes first to axillary lymph nodes (ALNs), axillary lymph node metastasis (ALNM) is the earliest detectable clinical manifestation of BC when distant metastases are present (3). Therefore, the accurate determination of the ALN status is vital for the clinical management of BC patients (4). Sentinel lymph nodes (SLNs) are the first draining lymph nodes for primary cancers. Therefore, the use of sentinel lymph node dissection (SLND) is clinically recommended to predict the ALN status in BC patients, particularly in patients with clinically negative lymph nodes (5). However, ALND is detrimental for patients due to its persistent side effects, including lymphedema and shoulder movement restriction (6). Accordingly, BC therapy is in transition to minimal axillary surgery, even in presence of SLN involvement (7). Of note, the Z0011 experiment demonstrated that the overall survival rate of clinical T1/T2 BC patients with two or fewer SLN metastases was not lower after SLND alone than after ALND (8). The results of several randomized trials have shown that the short- and long-term morbidity of ALND decreases in patients with negative ALNs, which improves the quality of life of patients (6,9). In addition, clinicians have observed that up to 70% of patients with early BC do not develop ALNM (10). Consequently, some types of axillary surgery can be considered, to some extent, a very significant overtreatment (5). As a result, the identification of the lymph node status should be the first step in BC management, not immediate surgical treatment (11).
Ultrasound has been widely used for the diagnosis of breast diseases. When breast malignancy is highly suspected, axillary ultrasound is preferred for examining the preoperative ALN status, which is an important prognostic factor for early BC (12). Axillary ultrasound can also identify the ALN status based on changes in morphology, cortical thickness, and internal echogenicity (13,14). As an essential screening method for breast lesions, ultrasound imaging vividly reflects the shape, growth direction, margins, and other features of tumors according to the Breast Imaging Reporting and Data System (15). In some studies, the ALN status was predicted based on the ultrasound image characteristics of lesions, such as quadrants, lesion size, boundary, and internal blood supply (2,7,13). Additionally, a prior study predicted the ALN status based on clinicopathological data, including lymph vascular infiltration, Ki-67 proliferation index, and hormone receptors (16). In several previous studies, models were constructed based on axillary ultrasound, ultrasound features of lesions, clinicopathological data, or a combination of these data for predicting the axillary lymphatic status and presented with the area under the receiver-operating characteristic curve (AUC) of 0.585–0.74, which was not ideal (2,16,17). In conclusion, understanding the preoperative ALN status is crucial for the selection of the suitable axillary treatment option (18).
Radiomics and deep learning radiomics have emerged as research hotspots, although there are many challenges in the deep learning of breast imaging and very few work has been landed to clinical trials (19). Radiomics can automatically extract numerous quantitative image features from medical images that are frequently imperceptible to the naked eye (20). In detail, this method uses specific advanced software to extract high-dimensional information, such as shape, intensity, and texture features, from medical images and then utilizes specific algorithms to label the characteristics of tumors (21). Of note, this study used deep learning radiomics to construct a model for predicting ALNM. Deep learning radiomics is a newly developed method that automatically extracts features in the hidden layer of a neural network from imaging data, thus obtaining quantitative and high-throughput features from medical images such as computed tomography, magnetic resonance imaging, and ultrasound through supervised learning (20-22). Different from the prediction model constructed with related clinical data, radiomics and deep learning radiomics combined with clinical parameters can integrate clinical information and network features to provide complementary information for image features. Prior research has unveiled that the model can be constructed with ultrasonic image features and clinical information, which improves the performance of the prediction model (23,24). Accordingly, it is reasonable to hypothesize that deep learning radiomics and radiomics can extract more quantitative characteristic information from ultrasound images of breast malignant lesions and can be combined with relevant clinical parameters to generate a combined prediction model for better prediction and stratification of ALNs.
Methods
Patients
The study was conducted in accordance with the Declaration of Helsinki (as revised in 2013). The study was approved by the Ethics Committee of the First Affiliated Hospital of Wannan Medical College (Yijishan Hospital of Wannan Medical College), and informed consent was obtained from patients for the collection of clinical data. In this study, we retrospectively collected and analyzed the data of female patients with solitary BC who underwent mastectomy and ALND in the First Affiliated Hospital of Wannan Medical College (Yijishan Hospital of Wannan Medical College) from January 2012 to June 2021. The inclusion criteria were as follows: (I) patients diagnosed with a malignant tumor of the breast based on pathological findings; (II) patients who had undergone ALND; (III) patients with complete and detailed measurements and images within 1 month before surgery; (IV) patients pathologically diagnosed with a single lesion that had a maximum transversal of less than or equal to 5 cm on ultrasound. The exclusion criteria were listed below: (I) patients pathologically diagnosed with multiple foci; (II) patients with a history of neoadjuvant chemotherapy; (III) patients without detailed ultrasound reports or complete image information, such as inability to confirm infiltration of the interstitial adipose tissue violation; (IV) patients with incomplete clinical data and immunohistochemistry and pathology results. Patients who were confirmed to have positive axillary nodes by ultrasound were included in the study. In addition, the patients in the training and test cohorts were stratified and matched according to clinical node status.
The clinical data, ultrasound images, and pathological findings of these patients were collected. Surgical specimens were obtained for the immunohistochemistry detection of estrogen receptor, progesterone receptor, human epidermal growth factor receptor-2, and Ki-67, as well as pathological analysis.
Ultrasound instruments and ultrasound image information acquisition
All patients underwent ultrasound examinations in our outpatient or inpatient ultrasound medicine department with an Esaote Mylab Twice (Esaote, Genova, Italy) color Doppler ultrasound instrument equipped with a 4–13 MHz linear array transducer, as well as a Siemens S2000 color Doppler ultrasound instrument (Siemens, Concord, CA, USA) equipped with a 4–9 MHz linear array transducer.
Breast Imaging Reporting and Data System-based feature extraction and axillary ultrasound findings
All ultrasound images were analyzed based on the Breast Imaging Reporting and Data System and with reference to the Adler blood flow classification. The image database included two-dimensional ultrasound images, color Doppler images of lesions, and internal blood flow grading of lesions. All images were reviewed by two senior radiologists (HF and TW, both with 12 years of experience in breast ultrasound) in a double-blind way. Images were imported into the database if both radiologists agreed. If there was disagreement between the two radiologists, a consensus was negotiated, and then images were imported into the database. If they still disagreed with the image analysis during evaluation, another radiologist (FJ, with 28 years of breast ultrasound experience) reviewed the image and entered it into a database. ALN ultrasound was conducted in this study. Lymph nodes were classified as positive ALNs in the presence of the following sonographic signs: a cortical thickness greater than 3 mm, a long/short diameter less than 2, a cortical/medullary thickness greater than 1, partial or complete loss of the lymphatic gate, complete or partial replacement of the lymph node, and microcalcifications of the lymph node. Before the start of the study, HF and TW attended centralized training in the standardized assessment of BC and ALN ultrasound images. All of the image information researchers in this study were blinded to the pathological findings of the patients. All relevant data were input into the database by the first author herself. Pathology labels were used to indicate the presence or absence of metastasis in the pathology after ALND. In cases of two classifications, a label of 0 indicated no metastasis, while a label of 1 indicated the presence of metastasis. In cases of multiple classifications, a label of 0 represented no transfer, a label of 1 marked low-load transfer, and a label of 2 suggested heavy-load transfer.
Related clinical data
The clinical data of patients were obtained, including basic clinical data (age, tumor size, location, and detailed ultrasound features) and pathological results (the pathological tissue types of the tumor and the main immunohistochemistry results). In addition, the histopathological findings of SLND and ALND were recorded, including the total number of resected ALNs and lymph node metastasis. Thereafter, a machine algorithm was used to select the optimal model where clinical data could predict ALNM. The characteristics including age and tumor size were introduced to explore the effects of clinical data on the prediction of ALNs. The specific statistical analysis is displayed in Table 1. Additionally, Pearson correlation among features was calculated, and the results showed that our clinical features were less correlated (Figure 1).
Table 1
Feature name | Test | Training | Validation | |||||||||||
---|---|---|---|---|---|---|---|---|---|---|---|---|---|---|
All | Label =0 | Label =1 | P | All | Label =0 | Label =1 | P | All | Label =0 | Label =1 | P | |||
Age, year | 52.7±10.0 | 52.8±9.8 | 52.3±10.9 | 0.782 | 52.60±10.16 | 53.19±10.64 | 51.87±9.51 | 0.108 | 52.86±9.38 | 52.65±9.64 | 54.32±7.17 | 0.406 | ||
Size, mm | 23.4±8.7 | 21.8±7.7 | 28.8±10.0 | <0.001 | 23.71±8.86 | 22.15±8.40 | 25.65±9.02 | <0.001 | 23.71±9.33 | 22.28±8.55 | 34.08±8.24 | <0.001 | ||
The axillary US | <0.001 | <0.001 | <0.001 | |||||||||||
Negative | 139 (0.67) | 125 (0.78) | 14 (0.30) | 386 (0.62) | 272 (0.80) | 114 (0.41) | 154 (0.74) | 146 (0.80) | 8 (0.32) | |||||
Positive | 69 (0.33) | 36 (0.23) | 33 (0.70) | 235 (0.38) | 72 (0.20) | 163 (0.59) | 53 (0.26) | 36 (0.20) | 17 (0.68) | |||||
ISAT | 0.003 | <0.001 | <0.001 | |||||||||||
Negative | 78 (0.38) | 69 (0.43) | 9 (0.19) | 231 (0.37) | 160 (0.47) | 71 (0.26) | 111 (0.54) | 107 (0.59) | 4 (0.16) | |||||
Positive | 130 (0.63) | 92 (0.57) | 38 (0.81) | 390 (0.63) | 184 (0.53) | 206 (0.74) | 96 (0.46) | 75 (0.41) | 21 (0.84) | |||||
IIAT | 0.003 | <0.001 | <0.001 | |||||||||||
Negative | 181 (0.87) | 146 (0.91) | 35 (0.74) | 506 (0.81) | 305 (0.89) | 201 (0.73) | 175 (0.85) | 161 (0.88) | 14 (0.56) | |||||
Positive | 27 (0.13) | 15 (0.09) | 12 (0.26) | 115 (0.19) | 39 (0.11) | 76 (0.27) | 32 (0.15) | 21 (0.12) | 11 (0.44) | |||||
Echo halo | <0.001 | <0.001 | <0.001 | |||||||||||
Negative | 116 (0.56) | 102 (0.63) | 14 (0.30) | 296 (0.48) | 203 (0.59) | 93 (0.34) | 115 (0.56) | 109 (0.60) | 6 (0.24) | |||||
Positive | 92 (0.44) | 59 (0.37) | 33 (0.70) | 325 (0.52) | 141 (0.41) | 184 (0.66) | 92 (0.44) | 73 (0.40) | 19 (0.76) | |||||
Laterality | 0.732 | 0.976 | 0.214 | |||||||||||
Left | 124 (0.60) | 97 (0.60) | 27 (0.57) | 321 (0.52) | 178 (0.52) | 143 (0.52) | 107 (0.52) | 97 (0.53) | 10 (0.40) | |||||
Right | 84 (0.40) | 64 (0.40) | 20 (0.43) | 300 (0.48) | 166 (0.48) | 134 (0.48) | 100 (0.48) | 85 (0.47) | 15 (0.60) | |||||
Quadrants | 0.288 | 0.002 | 0.337 | |||||||||||
Outer upper | 116 (0.56) | 90 (0.56) | 26 (0.55) | 330 (0.53) | 160 (0.47) | 170 (0.61) | 108 (0.52) | 91 (0.50) | 17 (0.68) | |||||
Outer lower | 27 (0.13) | 16 (0.10) | 11 (0.23) | 79 (0.13) | 50 (0.15) | 29 (0.10) | 27 (0.13) | 26 (0.14) | 1 (0.04) | |||||
Inner lower | 8 (0.04) | 6 (0.04) | 2 (0.04) | 34 (0.05) | 24 (0.07) | 10 (0.04) | 9 (0.04) | 9 (0.05) | Null | |||||
Inner upper | 51 (0.25) | 45 (0.28) | 6 (0.13) | 157 (0.25) | 97 (0.28) | 60 (0.22) | 54 (0.26) | 48 (0.26) | 6 (0.24) | |||||
Central | 6 (0.03) | 4 (0.02) | 2 (0.04) | 21 (0.03) | 13 (0.04) | 8 (0.03) | 9 (0.04) | 8 (0.04) | 1 (0.04) | |||||
Shape | 0.189 | 0.0150 | 0.091 | |||||||||||
Regular | 19 (0.09) | 17 (0.11) | 2 (0.04) | 47 (0.08) | 34 (0.10) | 13 (0.05) | 19 (0.09) | 19 (0.10) | Null | |||||
Irregular | 189 (0.91) | 144 (0.89) | 45 (0.96) | 574 (0.92) | 310 (0.90) | 264 (0.95) | 188 (0.91) | 163 (0.90) | 25 (1.00) | |||||
Orientation | 0.387 | 0.399 | 0.597 | |||||||||||
Horizontal | 135 (0.65) | 107 (0.67) | 28 (0.60) | 388 (0.62) | 220 (0.64) | 168 (0.61) | 148 (0.72) | 129 (0.71) | 19 (0.76) | |||||
Vertical | 73 (0.35) | 54 (0.34) | 19 (0.40) | 233 (0.38) | 124 (0.36) | 109 (0.39) | 59 (0.29) | 53 (0.29) | 6 (0.24) | |||||
Margin | 0.189 | 0.007 | 0.112 | |||||||||||
Circumscribed | 19 (0.09) | 17 (0.11) | 2 (0.04) | 55 (0.09) | 40 (0.12) | 15 (0.05) | 17 (0.08) | 17 (0.09) | Null | |||||
Not circumscribed | 189 (0.91) | 144 (0.89) | 45 (0.96) | 566 (0.91) | 304 (0.88) | 262 (0.95) | 190 (0.92) | 165 (0.90) | 25 (1.00) | |||||
Echo pattern | 0.978 | 0.277 | 0.258 | |||||||||||
Complex | 9 (0.04) | 7 (0.04) | 2 (0.04) | 16 (0.03) | 11 (0.03) | 5 (0.02) | 9 (0.04) | 9 (0.05) | Null | |||||
Hypoechoic | 199 (0.96) | 154 (0.96) | 45 (0.96) | 605 (0.97) | 333 (0.97) | 272 (0.98) | 198 (0.96) | 173 (0.95) | 25 (1.00) | |||||
Posterior acoustic feature | 0.43 | 0.08 | 0.01 | |||||||||||
No change | 121 (0.58) | 94 (0.58) | 27 (0.57) | 356 (0.57) | 204 (0.59) | 152 (0.55) | 137 (0.66) | 124 (0.68) | 13 (0.52) | |||||
Enhance | 41 (0.20) | 35 (0.22) | 6 (0.13) | 101 (0.16) | 61 (0.18) | 40 (0.14) | 30 (0.14) | 29 (0.16) | 1 (0.04) | |||||
Shadow | 46 (0.22) | 32 (0.20) | 14 (0.30) | 164 (0.26) | 79 (0.23) | 85 (0.31) | 40 (0.19) | 29 (0.16) | 11 (0.44) | |||||
Calcification | 0.013 | 0.002 | 0.272 | |||||||||||
No calcification | 97 (0.47) | 83 (0.52) | 14 (0.30) | 291 (0.47) | 179 (0.52) | 112 (0.40) | 102 (0.49) | 92 (0.51) | 10 (0.4000) | |||||
Macrocalcification | 4 (0.02) | 2 (0.01) | 2 (0.04) | 19 (0.03) | 13 (0.04) | 6 (0.02) | 4 (0.02) | 4 (0.02) | Null | |||||
Microcalcification | 107 (0.51) | 76 (0.47) | 31 (0.66) | 311 (0.50) | 152 (0.44) | 159 (0.57) | 101 (0.49) | 86 (0.47) | 15 (0.60) | |||||
Vascularity | <0.001 | <0.001 | 0.003 | |||||||||||
No flow | 48 (0.23) | 43 (0.27) | 5 (0.11) | 135 (0.22) | 93 (0.27) | 42 (0.15) | 58 (0.28) | 55 (0.30) | 3 (0.12) | |||||
Alder I | 58 (0.28) | 47 (0.30) | 11 (0.23) | 129 (0.21) | 82 (0.24) | 47 (0.17) | 35 (0.17) | 31 (0.17) | 4 (0.16) | |||||
Alder II | 57 (0.27) | 45 (0.28) | 12 (0.26) | 188 (0.30) | 116 (0.34) | 72 (0.26) | 73 (0.35) | 67 (0.37) | 6 (0.24) | |||||
Alder III | 45 (0.22) | 26 (0.16) | 19 (0.40) | 169 (0.27) | 53 (0.15) | 116 (0.42) | 41 (0.20) | 29 (0.16) | 12 (0.48) | |||||
ER | 0.469 | 0.820 | 0.480 | |||||||||||
− | 68 (0.33) | 54 (0.34) | 14 (0.30) | 186 (0.30) | 105 (0.31) | 81 (0.30) | 53 (0.26) | 47 (0.26) | 6 (0.24) | |||||
+ | 16 (0.08) | 15 (0.09) | 1 (0.02) | 44 (0.07) | 23 (0.07) | 21 (0.08) | 22 (0.11) | 20 (0.11) | 2 (0.08) | |||||
++ | 33 (0.16) | 22 (0.14) | 11 (0.23) | 81 (0.13) | 38 (0.11) | 43 (0.16) | 15 (0.07) | 15 (0.08) | Null | |||||
++++ | 91 (0.44) | 70 (0.43) | 21 (0.45) | 310 (0.50) | 178 (0.52) | 132 (0.48) | 117 (0.57) | 100 (0.55) | 17 (0.68) | |||||
PR | 0.138 | 0.591 | 0.133 | |||||||||||
− | 83 (0.40) | 68 (0.42) | 15 (0.32) | 241 (0.39) | 134 (0.39) | 107 (0.39) | 74 (0.36) | 67 (0.37) | 7 (0.28) | |||||
+ | 15 (0.07) | 12 (0.07) | 3 (0.06) | 64 (0.10) | 32 (0.09) | 32 (0.12) | 16 (0.08) | 15 (0.08) | 1 (0.04) | |||||
++ | 35 (0.17) | 27 (0.17) | 8 (0.17) | 91 (0.15) | 47 (0.14) | 44 (0.16) | 31 (0.15) | 29 (0.16) | 2 (0.08) | |||||
+++ | 75 (0.36) | 54 (0.34) | 21 (0.45) | 225 (0.36) | 131 (0.38) | 94 (0.34) | 86 (0.41) | 71 (0.39) | 15 (0.60) | |||||
HER-2 | 0.092 | 0.015 | 0.497 | |||||||||||
− | 30 (0.14) | 25 (0.16) | 5 (0.11) | 124 (0.20) | 79 (0.23) | 45 (0.16) | 52 (0.25) | 45 (0.25) | 7 (0.28) | |||||
+ | 71 (0.34) | 59 (0.37) | 12 (0.26) | 210 (0.34) | 120 (0.35) | 90 (0.32) | 62 (0.30) | 59 (0.32) | 3 (0.12) | |||||
++ | 53 (0.26) | 38 (0.24) | 15 (0.32) | 132 (0.21) | 67 (0.19) | 65 (0.23) | 47 (0.23) | 38 (0.21) | 9 (0.36) | |||||
+++ | 54 (0.26) | 39 (0.24) | 15 (0.32) | 155 (0.25) | 78 (0.23) | 77 (0.28) | 46 (0.22) | 40 (0.22) | 6 (0.24) | |||||
Ki-67 | 0.113 | 0.279 | 0.343 | |||||||||||
Negative | 49 (0.24) | 42 (0.26) | 7 (0.15) | 145 (0.23) | 86 (0.25) | 59 (0.21) | 58 (0.28) | 53 (0.29) | 5 (0.20) | |||||
Positive | 159 (0.76) | 119 (0.74) | 40 (0.85) | 476 (0.77) | 258 (0.75) | 218 (0.79) | 149 (0.72) | 129 (0.71) | 20 (0.80) | |||||
Pathology results | 0.049 | 0.010 | 0.077 | |||||||||||
Ductal carcinoma | 182 (0.88) | 137 (0.85) | 45 (0.96) | 547 (0.88) | 293 (0.85) | 254 (0.92) | 170 (0.82) | 147 (0.81) | 23 (0.92) | |||||
Lobular carcinoma | 8 (0.04) | 7 (0.04) | 1 (0.02) | 19 (0.03) | 12 (0.03) | 7 (0.03) | 10 (0.05) | 8 (0.04) | 2 (0.08) | |||||
Others | 18 (0.09) | 17 (0.11) | 1 (0.02) | 55 (0.09) | 39 (0.11) | 16 (0.06) | 27 (0.13) | 27 (0.15) | Null |
Label =0, lymph node dissection is negative, label =1: lymph node dissection is positive; data are presented as mean ± standard deviation or number (frequency). The staining intensity grading for pathological samples is as follows: ‘+’, ‘++’, ‘+++’. Ki-67: negative: <14%, positive: ≥14%. US, ultrasound; ISAT, infiltration of subcutaneous adipose tissue; IIAT, infiltration of the interstitial adipose tissue; ER, estrogen receptor; PR, progesterone receptor; HER-2, human epidermal growth factor receptor 2.
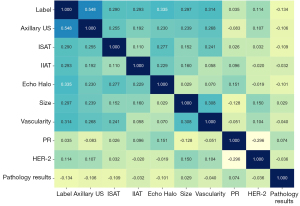
Intensity normalization
Our study was performed under variable imaging conditions of different machines. Therefore, the pixel value range of medical images varied considerably. In this context, all pixel values in each image were sorted and the intensity of each image was truncated to a range of 0.5 to 99.5 percentage points to minimize the side effects of pixel outliers. Subsequently, images were analyzed and features were extracted.
Hand-crafted features and feature selection
Hand-crafted features generally are categorized into three types, including geometric features (23 features, describing the third-order morphological characteristics), intensity features (340 features, representing the first-order statistical distribution of voxel intensity), and texture features (1,040 features, describing the pattern or second-order and higher-order spatial distribution of intensity). In our study, five texture features were extracted with software, including Gray Level Co-occurrence Matrix (GLCM), Gray Level Run Length Matrix (GLRLM), Gray Level Size Zone Matrix (GLSZM), Neighbouring Gray Tone Difference Matrix (NGTDM), and Gray Level Dependence Matrix (GLDM). A total of 1,403 hand-crafted features were extracted with Pyradiomics software (http://pyradiomics.readthedocs.io) for further statistical analysis.
For highly repetitive features, the correlation between features was analyzed with the Spearman’s rank correlation coefficient, and one of the two features with a correlation coefficient greater than 0.9 was retained. To retain the capability of depicting features to the greatest extent, features were filtered with a greedy recursive deletion strategy, that is, the feature with the largest redundancy was removed from the current set each time.
Radiomic feature selection
All feature lines were standardized with the z-score standardization method. Next, features with nonzero coefficients were selected from the training cohort with the least absolute shrinkage and selection operator (LASSO) logistic regression algorithm combined with penalty parameter tuning that was conducted through 10-fold cross-validation. The selected features were weighted by their respective coefficients and linearly combined to generate a radiomics signature.
Deep transfer learning model and feature compression
Resnet50, resnet101, inception_v3, and vgg19 were chosen as convolutional neural network models which were pre-trained in the ImageNet Large Scale Visual Recognition Challenge-2012 dataset. Images of the largest tumor were captured to represent each patient. Then, the grayscale values were normalized to the range [−1, 1] with min-max transformation. Next, each cropped subregion image was resized to 224×224, except for the inception model, which was set to 299×299 with the nearest interpolation.
The image of the largest cross-section of the lesion was used as the model input. Since the dimension of deep learning features was 2,048, their dimension was reduced by principal component analysis to maintain the balance among features. The dimension of deep learning was reduced to 128 to improve the generalization ability of the model and decrease the risk of overfitting.
Deep learning radiomic signature
A deep learning radiomic signature was constructed based on the selected clinical features, radiomic features, and 128 compressed deep transfer learning features. The same pipeline was followed up as the radiomics signature or deep transfer learning signature.
After features were screened with the LASSO analysis, a risk model was constructed by inputting the final features into the machine learning models to obtain the final deep learning radiomic signature. Support vector machine or linear regression was chosen during the construction of the final signature based on their performance in the test cohort. Figure 2 shows the workflow of deep transfer learning and radiomics combined with clinical parameters used in this study. Deep learning models were retrained, with 1,000 epochs for each model. Figure S1 of loss and accuracy curve in the supplementary material illustrate the training process.
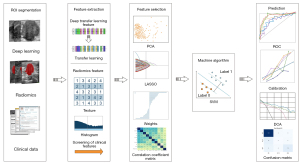
Statistical analysis
With ALND as the reference standard, the ALN status was classified into three groups, including no ALNM (N0), low-load ALNM [N + (1–2)], and heavy-load ALNM with ≥3 positive nodes [N + (≥3)]. The t-test or Mann-Whitney U test was used to compare the detailed basic clinical data and pathological data between the N0 and N+ (≥1) groups. The AUC of participants and their related characteristics, such as accuracy, sensitivity, specificity, positive predictive value, and negative predictive value, were utilized to evaluate the classification of axillary ultrasound, image-based radiomics, deep learning radiomics, clinical information, and combined diagnostic models with the model (Supplementary material—Appendix 1). The thresholds of sensitivity, specificity, positive predictive value, and negative predictive value were determined by the Youden index. Moreover, the AUC and these characteristics were compared with Delong’s test or other Hanley and McNeil methods. Decision Curve Analysis was also used to assess the predictive accuracy of the model. The fit of the model was evaluated with the calibration curves. In the clinical data, all cases were assigned into three groups, and P values less than 0.1 were used for further analysis. All statistics were two-sided, and a difference with P<0.05 was considered statistically significant. All statistical analyses were performed with Python 3.7.
Results
Baseline characteristics
A total of 5,321 women were enrolled in this study, among which 1,738 patients were diagnosed with BC after surgery. After careful screening according to our inclusion and exclusion criteria, 892 women were finally included, who were aged from 23 to 81 years (a mean age of 53 years), and 892 breast tumors were analyzed (their diameter ranged from 7 to 50 mm, with a mean diameter of 23.6 mm). Figure 3 exhibits the workflow for patient recruitment.
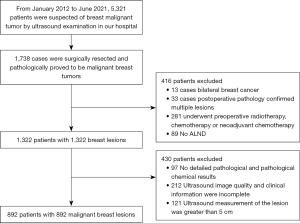
Selection of the deep learning radiomic model
The included patients were randomly classified into training and independent test cohorts at a ratio of 4:1, and the model parameters were optimized based on the training cohort. The training, validation, and test cohorts were matched in terms of clinicopathologic variables, including nodal stage. Specifically, 20% of training samples were selected for hyperparameter tuning. Finally, it was found that the optimal hyperparameters were as follows: stochastic gradient descent optimizer, an initial learning rate of 0.001, and a batch size of 32. The results revealed that inception_v3 outperformed other deep learning models (Table 2).
Table 2
Model | Cohort | Acc | AUC | 95% CI | Sensitivity | Specificity | PPV | NPV |
---|---|---|---|---|---|---|---|---|
Inception_v3 | Training | 0.730290 | 0.749599 | 0.6967–0.8024 | 0.707547 | 0.730667 | 0.428571 | 0.891720 |
Validation | 0.726708 | 0.731170 | 0.6327–0.8297 | 0.600000 | 0.769231 | 0.354167 | 0.884956 | |
Test | 0.725000 | 0.716721 | 0.6109–0.8226 | 0.714286 | 0.740458 | 0.357143 | 0.923077 | |
Resnet101 | Training | 0.726141 | 0.647468 | 0.5869–0.7081 | 0.452830 | 0.808511 | 0.393443 | 0.838889 |
Validation | 0.720497 | 0.641349 | 0.5311–0.7516 | 0.500000 | 0.801527 | 0.333333 | 0.870690 | |
Test | 0.768750 | 0.697781 | 0.5921–0.8035 | 0.571429 | 0.803030 | 0.378378 | 0.886179 | |
Resnet50 | Training | 0.757261 | 0.673763 | 0.6114–0.7361 | 0.481132 | 0.837766 | 0.451327 | 0.850949 |
Validation | 0.782609 | 0.735751 | 0.6342–0.8373 | 0.600000 | 0.838462 | 0.439024 | 0.900000 | |
Test | 0.725000 | 0.696158 | 0.5870–0.8053 | 0.714286 | 0.674242 | 0.333333 | 0.892857 | |
Vgg19 | Training | 0.759336 | 0.619606 | 0.5564–0.6828 | 0.471698 | 0.745989 | 0.307692 | 0.785088 |
Validation | 0.807453 | 0.609924 | 0.4880–0.7318 | 0.700000 | 0.584615 | 0.454545 | 0.833333 | |
Test | 0.837500 | 0.583063 | 0.4508–0.7153 | 0.714286 | 0.492424 | 0.625000 | 0.848684 | |
Wide_resnet50_v2 | Training | 0.757261 | 0.666964 | 0.6028–0.7311 | 0.528302 | 0.781915 | 0.447619 | 0.843501 |
Validation | 0.745342 | 0.678880 | 0.5660–0.7918 | 0.500000 | 0.846154 | 0.372093 | 0.881356 | |
Test | 0.837500 | 0.771916 | 0.6714–0.8725 | 0.642857 | 0.885496 | 0.529412 | 0.920635 |
Acc, accuracy; AUC, area under the curve; CI, confidence interval; PPV, positive predictive value; NPV, negative predictive value.
Prediction of ALNs in the no ALNM (N0) and low-load ALNM [N + (1–2)] groups
With N0 as the negative reference standard, patients were randomly arranged into three groups, including training (535 lesions), validation (178 lesions), and test (179 lesions) cohorts, at a ratio of 6:2:2. The detailed characteristics of patients, including age, tumor size, lateral position, and echo aperture, were analyzed. The evaluation results of axillary ultrasound by experienced radiologists demonstrated that the AUC of axillary ultrasound was 0.783 (95% confidence interval: 0.748–0.817) in the training cohort and 0.790 (95% confidence interval: 0.718–0.861) in the test cohort.
In the training cohort, the AUC was the highest (0.948) for deep learning radiomics combined with clinical parameters and traditional radiomics and was 0.746, 0.786, and 0.893 for deep learning radiomics, traditional radiomics, and clinicopathological data, respectively. The AUC for the prediction of ALNM was slightly lower in the test cohort than in the training cohort. Specifically, the AUC of deep learning radiomics combined with clinical parameters and traditional radiomics was the highest (0.920) in the test cohort, while the AUC of deep learning radiomics, radiomics, and clinicopathological data were 0.717, 0.755, and 0.884, respectively. Detailed statistical results are summarized and the corresponding receiver-operating characteristic curves are shown in Figure 4. The prediction results of deep learning radiomics only based on ultrasound images were lower than those of the combined model of clinical parameters, deep learning radiomics, and radiomics, with a statistically significant difference (P=0.01). The prediction results of radiomics and clinical parameters were lower than those of the combined model, without a statistically significant difference (P=0.3644 and P=0.122) (Table 3).
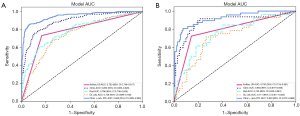
Table 3
Model name | Cohort | Accuracy | AUC | 95% CI | Sensitivity | Specificity | PPV | NPV |
---|---|---|---|---|---|---|---|---|
Rad | Training | 0.808896 | 0.785952 | 0.7357–0.8362 | 0.609091 | 0.853119 | 0.478571 | 0.907923 |
Test | 0.640523 | 0.755300a | 0.6496–0.8610 | 0.846154 | 0.598425 | 0.301370 | 0.950000 | |
DL-Image Only | Training | 0.730290 | 0.745599 | 0.6967–0.8024 | 0.707547 | 0.730667 | 0.428571 | 0.891720 |
Test | 0.725000 | 0.716721b | 0.6109–0.8226 | 0.714286 | 0.740458 | 0.357143 | 0.923077 | |
Clinical | Training | 0.852735 | 0.892740 | 0.8659–0.9196 | 0.762136 | 0.889546 | 0.737089 | 0.902000 |
Test | 0.821229 | 0.884268c | 0.8186–0.9499 | 0.914894 | 0.787879 | 0.605634 | 0.962963 | |
DLR | Training | 0.915849 | 0.947550 | 0.9289–0.9662 | 0.849515 | 0.942801 | 0.857843 | 0.939096 |
Test | 0.893855 | 0.920052 | 0.8722–0.9679 | 0.808511 | 0.924242 | 0.791667 | 0.931298 |
Different models predicted ALN state results [N0 vs. N+ (≥1)]. a, P=0.3644, Delong et al. compared radiomics with DLR in independent test cohorts; b, P=0.010, Delong et al. compared DLR in an independent test cohort with image-based deep learning; c, P=0.122, Delong et al. clinical parameters were compared with DLR in the independent test cohort. AUC, area under the curve; CI, confidence interval; PPV, positive predictive value; NPV, negative predictive value; DL, deep learning; DLR, deep learning radiomics; ALN, axillary lymph node.
Prediction of ALNS in the low-load ALNM [N + (1–2)] and heavy-load ALNM with ≥3 positive nodes [N + (≥3)] groups
In this experiment, with N + (1–2) as the negative reference standard, 206 lesions were randomly selected as the training cohort, and 47 lesions as the independent test cohort. In the training cohort, deep learning radiomics, image-based radiomics, and clinicopathological data showed the AUC of 0.766, 0.783, and 0.746, respectively, while clinical parameters combined with deep learning radiomics and radiomics had the AUC of 0.999. In the independent test cohort, the AUC of the combined model decreased but still reached 0.819, higher than that of deep learning radiomics (AUC: 0.718, P=0.1851), image-based radiomics (AUC: 0.744, P=0.3045), and clinicopathological data (AUC: 0.770, P=0.3869). The corresponding receiver-operating characteristic curves are listed in Figure 5.
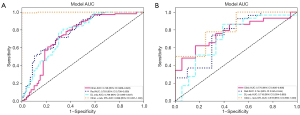
Prediction of ALNS in the no-ALNM, low-load ALNM, and heavy-load ALNM with ≥3 positive nodes groups
Patients were assigned into three groups [N0, N + (1–2), N + (≥3)] according to the ALN status. The prediction model was extended, and three groups of tasks were implemented to predict the lymph node status. Then, the number of lesions was 639 in the N0 group, 98 in the N + (1–2) group, and 155 in the N+ (≥3) group, of which 20% was selected as the test cohort, including 132 lesions in the N0 group, 18 lesions in the N + (1–2) group, and 29 lesions in the N+ (≥3) group. The training cohort included 376 lesions in the N0 group, 59 lesions in the N + (1–2) group, and 100 lesions in the N + (≥3) group. Deep learning radiomics and radiomics were generated based on conventional breast ultrasound images combined with clinicopathological data. The accuracy of the combined model was 0.798. The prediction model for the combined diagnosis performed well in the no ALNM group. The confusion matrix is exhibited in Figure 6.
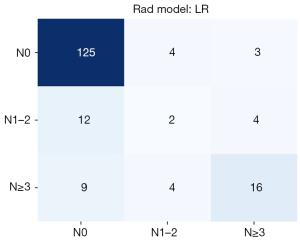
Explanatory nature of the deep learning radiomic model
Explainable artificial intelligence can be used to improve the visibility of deep learning models and better understand the underlying decision-making process (25). Thus, explainable artificial intelligence is important in model development for the goal of model visualization and inspection. To study the interpretability of deep learning radiomics, gradient-weighted class activation mapping was used in the present study to visualize the network, and the high coarse localization map was the import region of the classification target (Figure 7).
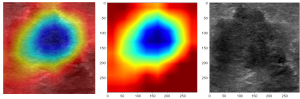
Evaluation of the model
Deep learning was used to construct and evaluate the ALNM model, and the Decision Curve Analysis was utilized to directly evaluate the benefit to patients. Decision Curve Analysis is an analytical method that integrates patient or decision-maker preferences into the analysis to evaluate a clinical prediction model and meet the actual needs of clinical decision-making, which is becoming increasingly popular in clinical analysis. Figure 8 shows that if the threshold probability is greater than 25%, the use of the deep learning radiomic prediction of lymph node metastasis will yield more net benefit in the current study. Meanwhile, the predictive ability and actual situation of various models were assessed with the calibration curve. The results illustrated that the combined application of clinical parameters, radiomics, and deep learning was the optimal way to evaluate ALNM (Figure 9).
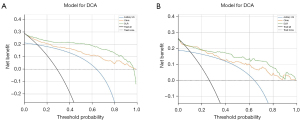
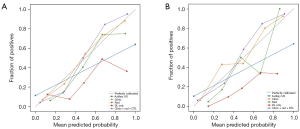
Discussion
BC has emerged as a serious threat to the health of women around the world (1). The high mortality of BC is attributable to the metastasis of vital organs other than the primary tumor (26). According to the ACOSOG Z0011 trial, SLND alone, but not ALND, did not decrease the survival rate of early BC patients with less than two metastatic SLNs (8). On the contrary, another study elucidated that SLN surgery alone without further ALND was an appropriate, safe, and effective treatment option for BC patients with clinically negative lymph nodes in the presence of negative SLNs (27). As reported, up to 70% of patients with early BC do not suffer from ALNM (10). Accordingly, certain types of axillary surgery can be viewed as a very significant overtreatment to some extent (5). Therefore, it is extremely critical for BC treatment to accurately predict the extent of ALNM in a non-invasive manner.
In this study, a combined model (clinical, radiomics, and deep learning radiomics) was developed and validated, for the first time, for evaluating the ALN status in patients with early BC. The diagnostic performance of this combined model was significantly superior to that of the single method in differentiating patients with N0 BC from patients with N + (≥1) BC, with the AUC of 0.920. Encouragingly, our model could favorably discriminate axillary diseases between patients with low-load ALNM [N + (1–2)] and patients with heavy-load ALNM of ≥3 positive node [N + (≥3)]. The combined prediction model constructed with the three methods may be developed as a non-invasive imaging approach with the potential to replace SLND for the treatment of early BC. Clinical parameters in combination with deep learning radiomics have been demonstrated to have the potential to help breast clinicians select the optimal axillary treatment: N0 patients do not require SLND or ALND, whereas N + (1–2) patients only require SLND and N + (≥3) patients require ALND (8,24).
At present, preoperative axillary ultrasound has a poor overall diagnostic effect. In this study, the AUC of axillary ultrasound was 0.790, consistent with the results of prior studies (28-30). Zhu et al. found that deep learning could be used to identify microinvasion of breast ductal carcinoma in situ from ultrasound images (31). Then, radiomics and deep learning radiomics based on conventional ultrasound images were combined, followed by combination with clinical parameters. Our results unraveled that the performance of the combination of these three methods (AUC: 0.920) was better than that (AUC: 0.790) of conventional axillary ultrasonography evaluated by two senior radiologists with 12 years of breast ultrasound experience (both) in predicting ALNM.
It has been reported that the ultrasound characteristics of some lesions, such as tumor size, internal blood supply, lesion boundary, infiltration of subcutaneous adipose tissues, and infiltration of the interstitial adipose tissue violation, can be used as independent risk factors for ALNM (2,7,32). Additionally, other studies have identified histopathological data, including lymphatic vascular invasion, estrogen receptor, and progesterone receptor, as potential risk factors for ALNM (2,33). Different from most previous studies, all histopathological data were used in this study and obtained before surgery. Meanwhile, detailed ultrasound features of lesions were collected before surgery. Accordingly, some preoperative clinical data were retained as candidate factors for constructing prediction models which could be used entirely as non-invasive prediction methods for assessing the ALN status.
In recent years, the development of radiomics and deep learning radiomics is an extremely important clinical research direction because these two methods are promising for applications (20,24). Qi et al. observed that radiomic features extracted from diagnostic Computed Tomography scans could enhance the predictive power of pathologic complete response when used in conjunction with clinical features (19). Lambin et al. noted that the proposed deep learning radiomics demonstrated excellent performance on the diagnosis of benign and malignant focal liver lesions when combined with contrast-enhanced ultrasound cines and clinical factors (34). Radiomics-based decision-support systems can be a powerful tool for precision diagnosis and treatment in modern medicine (35). Additionally, these methods also open up new research directions for predicting ALNM in BC. Usually, tens of thousands to hundreds of thousands of features can be extracted from medical images. Nevertheless, it is still in the initial stage of research on radiomics based on ultrasound images to predict ALNM. The efficiency of the research and development of prediction models has not yet reached the ideal state. As a result, it is necessary to improve and optimize the model to achieve the best state of clinical prediction (36). Feature selection is an important step in the feature construction process as it helps to identify the most relevant features and remove redundant and irrelevant features. Thus, different features and weightages may contribute to different conclusions. The selected features in the current study were weighted according to their respective coefficients and then linearly combined to generate a radiomic signature. Deep learning radiomics is a supervised method that can make use of the embedded information in images to learn high-throughput image features, which were different from hand-crafted and engineered features designed based on prior medical experiences. In our study, images were obtained under different imaging conditions of different machines, and their pixel value ranges varied substantially. To reduce the side effects of outlier pixels and avoid the inherent differences among different types of ultrasound machines, all pixel values in each image were ranked, and the intensity was truncated to the 0.5 to 99.5 percentage range. Meanwhile, data extraction and acquisition were standardized by two experienced radiologists.
It was previously demonstrated that radiological features obtained from ultrasound images of primary tumors could moderately predict the ALN status in BC, with the AUC ranging from 0.71 to 0.78 (36-38). Our results elaborated that radiological features extracted only from ultrasound images of primary tumors were effective in predicting ALNM. In addition, this method relied only on ultrasound which has been widely used for BC diagnosis, with images easily available. Therefore, this method can be utilized as a regular examination tool for BC. In this study, ALN status-related radiomic features collected from ultrasound images exerted a moderate predictive effect, with the AUC of 0.786 and 0.755 in the primary and test cohorts, respectively.
Compared with most previous studies, our study showed better diagnostic performance for the first time of the combined model by focusing on radiomics, deep learning radiomics, and clinical parameters, which could more effectively supplement image features by suppressing features extracted from images and render the model more reliable. To explore the most suitable basic model for the prediction of the ALN status, the model that best predicted the ALN status was selected between N0 and N + (≥1) patients for various models. When inception_v3 was selected as the basic model with the optimal performance, clinical parameters and the radiomics diagnostic model were further added. The prediction of the ALN status based on clinical data, radiomics, and deep learning radiomic composition was highly favorably effective, with the AUC of 0.948 and 0.920 in the main queue and validation cohorts, respectively. Furthermore, our experimental results exhibited that the combination of clinical parameters, radiomics, and deep learning radiomics could also effectively distinguish between patients with low-load [N + (1–2)] and heavy-load ALNM with ≥3 positive nodes [N+ (≥3)], which provide guidance to clinicians for axillary surgery. This study, which was performed completely based on conventional ultrasound images, was convenient, fast, and radiation-free and could realistically predict the ALN status under non-invasive conditions.
There are still some limitations in this study. First, our study was a single-center and retrospective study. Accordingly, image selection and information reading may result in certain biases. Second, patients with bilateral cancer or multifocal breast lesions were excluded from this study. Third, our study only included conventional two-dimensional grayscale images, and elastography and contrast-enhanced ultrasound images were not analyzed for the correlation with ALNM. Fourth, this study did not address whether BC genetic markers could be used to stratify patients according to disease risk (39). Finally, human factors in the development of the predicted model may cause bias. Models based on human feature selection is semi-automatic in nature, which can lead to bias in their performance due to the specific features selected or filtered by radiologists during the development phase. Previous studies have shown that such algorithms may not perform well in the real world.
To date, most of the current artificial intelligence tools are based on retrospective data, with very few artificial intelligence tools landed in clinical trials. However, as the deep learning tool is incorporated into clinical practice, supervision is needed to avoid algorithm deviation and solve the unique ethical, medicolegal, and quality-control problems of the deep learning algorithm (40). These ALNM-related issues are still unresolved, which warrants further investigation.
Conclusions
In this study, a combined model was constructed based on deep learning, which had the potential to predict preoperative ALN involvement in BC and was a minimally invasive, even non-invasive, and practical approach for BC diagnosis. This combined diagnosis model based on deep learning can evaluate the preoperative ALN status and help optimize axillary treatment for patients with early BC. Considering the fact that the receiver-operating characteristic curve analysis requires a large amount of data and relies on subjective selection of thresholds, further studies are needed to verify the current findings.
Acknowledgments
Funding: This work was supported by the Natural Science Research Project of the Higher Education in Anhui Province (No. KJ2020A0616 to XZ) and the Annual Scientific and Technological Projects of Wuhu City (No. 2020ms3-5 to XZ).
Footnote
Conflicts of Interest: All authors have completed the ICMJE uniform disclosure form (available at https://qims.amegroups.com/article/view/10.21037/qims-22-1257/coif). The authors have no conflicts of interest to declare.
Ethical Statement:
Open Access Statement: This is an Open Access article distributed in accordance with the Creative Commons Attribution-NonCommercial-NoDerivs 4.0 International License (CC BY-NC-ND 4.0), which permits the non-commercial replication and distribution of the article with the strict proviso that no changes or edits are made and the original work is properly cited (including links to both the formal publication through the relevant DOI and the license). See: https://creativecommons.org/licenses/by-nc-nd/4.0/.
References
- Bray F, Ferlay J, Soerjomataram I, Siegel RL, Torre LA, Jemal A. Global cancer statistics 2018: GLOBOCAN estimates of incidence and mortality worldwide for 36 cancers in 185 countries. CA Cancer J Clin 2018;68:394-424. [Crossref] [PubMed]
- Guo Q, Dong Z, Zhang L, Ning C, Li Z, Wang D, Liu C, Zhao M, Tian J. Ultrasound Features of Breast Cancer for Predicting Axillary Lymph Node Metastasis. J Ultrasound Med 2018;37:1354-3. [Crossref] [PubMed]
- Schwartz RS, Erban JK. Timing of Metastasis in Breast Cancer. N Engl J Med 2017;376:2486-8. [Crossref] [PubMed]
- Burkett BJ, Hanemann CW. A Review of Supplemental Screening Ultrasound for Breast Cancer: Certain Populations of Women with Dense Breast Tissue May Benefit. Acad Radiol 2016;23:1604-9. [Crossref] [PubMed]
- Lyman GH, Somerfield MR, Bosserman LD, Perkins CL, Weaver DL, Giuliano AE. Sentinel Lymph Node Biopsy for Patients With Early-Stage Breast Cancer: American Society of Clinical Oncology Clinical Practice Guideline Update. J Clin Oncol 2017;35:561-4. [Crossref] [PubMed]
- Land SR, Kopec JA, Julian TB, Brown AM, Anderson SJ, Krag DN, Christian NJ, Costantino JP, Wolmark N, Ganz PA. Patient-reported outcomes in sentinel node-negative adjuvant breast cancer patients receiving sentinel-node biopsy or axillary dissection: National Surgical Adjuvant Breast and Bowel Project phase III protocol B-32. J Clin Oncol 2010;28:3929-36. [Crossref] [PubMed]
- Zhang Y, Li J, Fan Y, Li X, Qiu J, Zhu M, Li H. Risk factors for axillary lymph node metastases in clinical stage T1-2N0M0 breast cancer patients. Medicine (Baltimore) 2019;98:e17481. [Crossref] [PubMed]
- Giuliano AE, Ballman KV, McCall L, Beitsch PD, Brennan MB, Kelemen PR, Ollila DW, Hansen NM, Whitworth PW, Blumencranz PW, Leitch AM, Saha S, Hunt KK, Morrow M. Effect of Axillary Dissection vs No Axillary Dissection on 10-Year Overall Survival Among Women With Invasive Breast Cancer and Sentinel Node Metastasis: The ACOSOG Z0011 (Alliance) Randomized Clinical Trial. JAMA 2017;318:918-26. [Crossref] [PubMed]
- Ashikaga T, Krag DN, Land SR, Julian TB, Anderson SJ, Brown AM, Skelly JM, Harlow SP, Weaver DL, Mamounas EP, Costantino JP, Wolmark N. Morbidity results from the NSABP B-32 trial comparing sentinel lymph node dissection versus axillary dissection. J Surg Oncol 2010;102:111-8. [Crossref] [PubMed]
- Bevilacqua JL, Kattan MW, Fey JV, Cody HS 3rd, Borgen PI, Van Zee KJ. Doctor, what are my chances of having a positive sentinel node? A validated nomogram for risk estimation. J Clin Oncol 2007;25:3670-9. [Crossref] [PubMed]
- Williams PA, Suggs J, Mangana SH. Axillary lymph node treatment in breast cancer: an update. J Miss State Med Assoc 2014;55:145-7. [PubMed]
- Cools-Lartigue J, Meterissian S. Accuracy of axillary ultrasound in the diagnosis of nodal metastasis in invasive breast cancer: a review. World J Surg 2012;36:46-54. [Crossref] [PubMed]
- Schwentner L, Helms G, Nekljudova V, Ataseven B, Bauerfeind I, Ditsch N, Fehm T, Fleige B, Hauschild M, Heil J, Kümmel S, Lebeau A, Schmatloch S, Schrenk P, Staebler A, Loibl S, Untch M, Von Minckwitz G, Liedtke C, Kühn T. Using ultrasound and palpation for predicting axillary lymph node status following neoadjuvant chemotherapy - Results from the multi-center SENTINA trial. Breast 2017;31:202-7. [Crossref] [PubMed]
- Van Berckelaer C, Huizing M, Van Goethem M, Vervaecke A, Papadimitriou K, Verslegers I, Trinh BX, Van Dam P, Altintas S, Van den Wyngaert T, Huyghe I, Siozopoulou V, Tjalma WA. Preoperative ultrasound staging of the axilla make's peroperative examination of the sentinel node redundant in breast cancer: saving tissue, time and money. Eur J Obstet Gynecol Reprod Biol 2016;206:164-71. [Crossref] [PubMed]
- Spak DA, Plaxco JS, Santiago L, Dryden MJ, Dogan BE. BI-RADS(®) fifth edition: A summary of changes. Diagn Interv Imaging 2017;98:179-90.
- Tapia G, Ying V, Di Re A, Stellin A, Cai TY, Warrier S. Predicting non-sentinel lymph node metastasis in Australian breast cancer patients: are the nomograms still useful in the post-Z0011 era? ANZ J Surg 2019;89:712-7. [Crossref] [PubMed]
- Youk JH, Son EJ, Kim JA, Gweon HM. Pre-Operative Evaluation of Axillary Lymph Node Status in Patients with Suspected Breast Cancer Using Shear Wave Elastography. Ultrasound Med Biol 2017;43:1581-6. [Crossref] [PubMed]
- Boughey JC, Moriarty JP, Degnim AC, Gregg MS, Egginton JS, Long KH. Cost modeling of preoperative axillary ultrasound and fine-needle aspiration to guide surgery for invasive breast cancer. Ann Surg Oncol 2010;17:953-8. [Crossref] [PubMed]
- Qi TH, Hian OH, Kumaran AM, Tan TJ, Cong TRY, Su-Xin GL, et al. Multi-center evaluation of artificial intelligent imaging and clinical models for predicting neoadjuvant chemotherapy response in breast cancer. Breast Cancer Res Treat 2022;193:121-38. [Crossref] [PubMed]
- Wang K, Lu X, Zhou H, Gao Y, Zheng J, Tong M, Wu C, Liu C, Huang L, Jiang T, Meng F, Lu Y, Ai H, Xie XY, Yin LP, Liang P, Tian J, Zheng R. Deep learning Radiomics of shear wave elastography significantly improved diagnostic performance for assessing liver fibrosis in chronic hepatitis B: a prospective multicentre study. Gut 2019;68:729-41. [Crossref] [PubMed]
- Gillies RJ, Kinahan PE, Hricak H. Radiomics: Images Are More than Pictures, They Are Data. Radiology 2016;278:563-77. [Crossref] [PubMed]
- Shin HC, Roth HR, Gao M, Lu L, Xu Z, Nogues I, Yao J, Mollura D, Summers RM. Deep Convolutional Neural Networks for Computer-Aided Detection: CNN Architectures, Dataset Characteristics and Transfer Learning. IEEE Trans Med Imaging 2016;35:1285-98. [Crossref] [PubMed]
- Xie Y, Zhang J, Xia Y, Fulham M, Zhang Y. Fusing texture, shape and deep model-learned information at decision level for automated classification of lung nodules on chest CT. Information Fusion 2018;42:102-10. [Crossref]
- Zheng X, Yao Z, Huang Y, Yu Y, Wang Y, Liu Y, Mao R, Li F, Xiao Y, Wang Y, Hu Y, Yu J, Zhou J. Deep learning radiomics can predict axillary lymph node status in early-stage breast cancer. Nat Commun 2020;11:1236. [Crossref] [PubMed]
- Groen AM, Kraan R, Amirkhan SF, Daams JG, Maas M. A systematic review on the use of explainability in deep learning systems for computer aided diagnosis in radiology: Limited use of explainable AI? Eur J Radiol 2022;157:110592. [Crossref] [PubMed]
- Liang Y, Zhang H, Song X, Yang Q. Metastatic heterogeneity of breast cancer: Molecular mechanism and potential therapeutic targets. Semin Cancer Biol 2020;60:14-27. [Crossref] [PubMed]
- Krag DN, Anderson SJ, Julian TB, Brown AM, Harlow SP, Costantino JP, Ashikaga T, Weaver DL, Mamounas EP, Jalovec LM, Frazier TG, Noyes RD, Robidoux A, Scarth HM, Wolmark N. Sentinel-lymph-node resection compared with conventional axillary-lymph-node dissection in clinically node-negative patients with breast cancer: overall survival findings from the NSABP B-32 randomised phase 3 trial. Lancet Oncol 2010;11:927-33. [Crossref] [PubMed]
- Neal CH, Daly CP, Nees AV, Helvie MA. Can preoperative axillary US help exclude N2 and N3 metastatic breast cancer? Radiology 2010;257:335-41. [Crossref] [PubMed]
- Kim GR, Choi JS, Han BK, Lee JE, Nam SJ, Ko EY, Ko ES, Lee SK. Preoperative Axillary US in Early-Stage Breast Cancer: Potential to Prevent Unnecessary Axillary Lymph Node Dissection. Radiology 2018;288:55-63. [Crossref] [PubMed]
- Chen X, Li X, Fan Z, Li J, Xie Y, Wang T, Ouyang T. Ultrasound as a replacement for physical examination in clinical staging of axillary lymph nodes in breast cancer patients. Thorac Cancer 2020;11:48-54. [Crossref] [PubMed]
- Zhu M, Pi Y, Jiang Z, Wu Y, Bu H, Bao J, Chen Y, Zhao L, Peng Y. Application of deep learning to identify ductal carcinoma in situ and microinvasion of the breast using ultrasound imaging. Quant Imaging Med Surg 2022;12:4633-46. [Crossref] [PubMed]
- Luo Y, Zhao C, Gao Y, Xiao M, Li W, Zhang J, Ma L, Qin J, Jiang Y, Zhu Q. Predicting Axillary Lymph Node Status With a Nomogram Based on Breast Lesion Ultrasound Features: Performance in N1 Breast Cancer Patients. Front Oncol 2020;10:581321. [Crossref] [PubMed]
- Liu L, Tang C, Li L, Chen P, Tan Y, Hu X, Chen K, Shang Y, Liu D, Liu H, Liu H, Nie F, Tian J, Zhao M, He W, Guo Y. Deep learning radiomics for focal liver lesions diagnosis on long-range contrast-enhanced ultrasound and clinical factors. Quant Imaging Med Surg 2022;12:3213-26. [Crossref] [PubMed]
- Lambin P, Leijenaar RTH, Deist TM, Peerlings J, de Jong EEC, van Timmeren J, Sanduleanu S, Larue RTHM, Even AJG, Jochems A, van Wijk Y, Woodruff H, van Soest J, Lustberg T, Roelofs E, van Elmpt W, Dekker A, Mottaghy FM, Wildberger JE, Walsh S. Radiomics: the bridge between medical imaging and personalized medicine. Nat Rev Clin Oncol 2017;14:749-62. [Crossref] [PubMed]
- Yajima R, Fujii T, Yanagita Y, Fujisawa T, Miyamoto T, Hirakata T, Tsutsumi S, Iijima M, Kuwano H. Prognostic value of extracapsular invasion of axillary lymph nodes combined with peritumoral vascular invasion in patients with breast cancer. Ann Surg Oncol 2015;22:52-8. [Crossref] [PubMed]
- Qiu X, Jiang Y, Zhao Q, Yan C, Huang M, Jiang T. Could Ultrasound-Based Radiomics Noninvasively Predict Axillary Lymph Node Metastasis in Breast Cancer? J Ultrasound Med 2020;39:1897-905. [Crossref] [PubMed]
- Han L, Zhu Y, Liu Z, Yu T, He C, Jiang W, Kan Y, Dong D, Tian J, Luo Y. Radiomic nomogram for prediction of axillary lymph node metastasis in breast cancer. Eur Radiol 2019;29:3820-9. [Crossref] [PubMed]
- Yu FH, Wang JX, Ye XH, Deng J, Hang J, Yang B. Ultrasound-based radiomics nomogram: A potential biomarker to predict axillary lymph node metastasis in early-stage invasive breast cancer. Eur J Radiol 2019;119:108658. [Crossref] [PubMed]
- Pinker K, Chin J, Melsaether AN, Morris EA, Moy L. Precision Medicine and Radiogenomics in Breast Cancer: New Approaches toward Diagnosis and Treatment. Radiology 2018;287:732-47. [Crossref] [PubMed]
- Bhowmik A, Eskreis-Winkler S. Deep learning in breast imaging. BJR Open 2022;4:20210060. [PubMed]