Development and validation of a nomogram for difficult laryngoscopy at visual laryngoscopy: a prospective nested case-control study
Introduction
Unsuccessful airway management is associated with increased perioperative morbidity and mortality (1-3). Unforeseen difficult laryngoscopy is the main reason for unanticipated difficult airways, which may lead to severe hypoxia, hypoxic brain damage, and even intraoperative death (4-8). In clinical practice, difficult laryngoscopy is a challenge for anesthesiologists.
The incidence of airway management failure can be greatly reduced if difficult laryngoscopy is predicted with high specificity and sensitivity. However, predicting difficult laryngoscopy is not a simple task. Many anatomical structures and functional units are involved in the formation of difficult laryngoscopy (9). The diagnosis of a difficult laryngoscopy is a subjective process and highly dependent on the anesthesiologist’s experience. There is a pressing need to develop methods of predicting difficult laryngoscopy conveniently, quickly, and accurately in clinical practice (10).
Current guidelines provide several approaches to predict the risk of difficult laryngoscopy (11). There has been considerable heterogeneity among studies of bedside examination tests in predicting difficult laryngoscopy, with no single feature being identified as more predictive than another (12). Most of the commonly used bedside examination tests for predicting difficult laryngoscopy are based on body surface anatomical markers, and these tests cannot truly reflect the internal anatomy of the upper airway. A comprehensive score combining a patient’s history and bedside test results can improve the accuracy of predicting difficult airways, including the STOP-Bang questionnaire and Wilson score. However, both single examinations and comprehensive scores have only relatively low sensitivities and slightly higher specificities (13). These standard airway screening tests should be interpreted with caution because they seem not to be considered superior screening tests. Therefore, although several assessment strategies have been developed, including a single bedside examination or a comprehensive score, they still cannot completely meet the current clinical requirements (14-16).
Imaging techniques, including X-ray (17), computed tomography (CT) (18), magnetic resonance imaging (MRI) (19), and ultrasound (20), can visualize the anatomical features of the upper airways. Such techniques have been recommended for predicting the risk of difficult airways in guidelines and expert consensus (1,11,21). Studies have shown that the diagnostic performance and area under the curve (AUC) of ultrasound in difficult airways are similar to those of CT and X-ray (21).
Ultrasonography might facilitate a new direction in difficult airway research, as it may reflect the internal anatomical characteristics of a patient’s upper airway, and can be used as a priority diagnostic strategy for difficult laryngoscopy owing to its easy availability, low cost, and no radiation hazard (11,21). Previous clinical practice and observations have found that some ultrasound image features such as pretracheal soft tissue thickness and tongue thickness are associated with difficult laryngoscopy (22-26). However, most current studies have only analyzed the association between each predictor and difficult laryngoscopy independently. Furthermore, the results of most studies are based on direct laryngoscopy, which is not consistent with a medical environment in which visual laryngoscopy dominates. Therefore, this study was designed to develop a comprehensive model based on multivariate statistical analysis (including bedside examination tests and ultrasonography) for difficult laryngoscopy at visual laryngoscopy. We present this article in accordance with the TRIPOD reporting checklist (available at https://qims.amegroups.com/article/view/10.21037/qims-23-95/rc).
Methods
Participants
The study was conducted in accordance with the Declaration of Helsinki (as revised in 2013). The Ethics Committee of Shanghai Ninth People’s Hospital, Shanghai, China approved the research protocol (No. SH9H-2021-T356-2). All patients provided their written informed consent before enrolment in this prospective nested case-control study. This study was conducted from December 27, 2021, to September 16, 2022, at Shanghai Ninth People’s Hospital. The following inclusion criteria were applied: (I) adult patients (≥18 years of age); (II) scheduled for elective surgery with American Society of Anesthesiologists Physical Status (ASA-PS) 1-3; (III) required tracheal intubation at visual laryngoscopy. Patients were excluded based on the following criteria: (I) language communication disorders; (II) cervical spine fractures or cervical spine diseases; (III) head and neck injury; (IV) emergency surgery; (V) allergy to related drugs.
Airway assessments
All patients underwent an ultrasonographic examination (SII; SonoSite Corporation, Bothell, WA, USA). The ultrasonographic measurements were performed by 2 experienced researchers using 2 ultrasound probes (Figure 1). Before ultrasonographic measurement, each patient was placed in the supine position with neck hyperextension by lifting the shoulders using a hard pillow. The ultrasound measurements were selected based on previous studies and systematic reviews (22,27-29). The following ultrasonographic methods were used: (I) distance from the skin to the tongue root (DSTR; Figure 2A), (II) distance from the skin to the trachea at the jugular notch (DSTJ; Figure 2B), (III) distance from the skin to the thyroid isthmus (DSTI; Figure 2C), (IV) distance from the skin to the cricothyroid membrane (DSCM; Figure 2D), (V) distance from the skin to the anterior commissure of the vocal cord (DSAC; Figure 2E), (VI) distance from the skin to the hyoid bone (DSHB; Figure 2F), and (VII) distance from the skin to the epiglottis (DSE; Figure 2G). All ultrasound measurements conformed strictly to a standard operating procedure. The ultrasound system was adjusted to Steep Needle Profiling (SNP) mode before the measurement. The ultrasound images were saved after all measured targets were displayed completely and clearly. The depth was set to 3.3 cm for high-frequency probe and 9.2 cm for low-frequency probe.
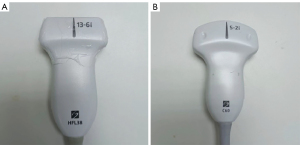
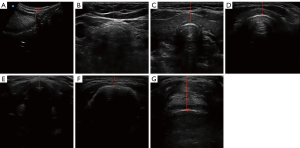
Before tracheal intubation, standard airway assessment was performed by a specific team comprising specially trained anesthesiologists and nurses. The results of baseline characteristics, medical history, and classic airway bedside examinations of each patient were recorded. The whole airway assessment process was mainly based on the 2022 American Society of Anesthesiologists Practice Guidelines for Management of the Difficult Airway (11). The definitions and details of baseline characteristics, medical histories, and classic airway bedside examinations are shown in Table S1.
Induction of general anesthesia
Patients were routinely monitored with electrocardiography, pulse oximetry, and non-invasive blood pressure measurement. They were administered with midazolam 2–3 mg, fentanyl 2–4 µg/kg, propofol 2–2.5 mg/kg, and rocuronium 0.6 mg/kg intravenously before tracheal intubation. After preoxygenation with mask-pressurized ventilation for approximately 3 minutes, tracheal intubation was performed using a visual laryngoscope (McGrath MAC; Aircraft Medical Co., Ltd., Edinburgh, UK), which is being increasingly utilized and gradually replacing direct laryngoscope.
During tracheal intubation, the Cormack–Lehane (CL) grade was evaluated (grade 1 represents a complete view of the glottis, grade 2 represents a partial view of the glottis or arytenoids, grade 3 represents only the visibility of the epiglottis, and grade 4 represents the absence of epiglottis visibility) (30). The CL grade was assessed by anesthesiologists with more than 3 years of experience who were blinded to the results of the ultrasonographic measurements. The classification was based on the CL grade, with grades 1–2 classified as “easy laryngoscopy” and grades 3–4 classified as “difficult laryngoscopy”. The airway assessments and CL evaluations were performed by different well-trained researchers, and they were both blinded to each other’s assessment results.
Sensitivity analysis
To examine the adequacy of the sample size, we performed a sensitivity analysis by performing a saturation analysis. Different sized datasets (from 10% to 100%) were used to evaluate the same model for the same outcome (difficult laryngoscopy). The relationship between dataset size and model performance can be summarized throughout the analysis (31).
Statistical analysis
Continuous variables were expressed as the median (quartile) or the mean ± standard deviation. Categorical variables were expressed as frequencies and percentages. Statistical analyses were conducted to assess group differences using different methods. Median values were analyzed using the Kruskal–Wallis H test, means were analyzed using Student’s t-test, and categorical variables were analyzed using chi-square analysis. The statistical analysis was performed using the software SPSS 25.0 (IBM Corp., Armonk, NY, USA) and R version 4.2.1 (The R Foundation for Statistical Computing, Vienna, Austria; https://www.r-project.org/).
A few missing values were filled using the missRanger algorithm in R. The data were randomly divided into a training set and a validation set (at a ratio of 7:3). Using the least absolute shrinkage and selection operator (LASSO) method (32), variables with a P-value of ≤0.1 in the univariate logistic regression analysis were considered, and the most significant risk factors were selected for prediction modeling. We performed 10-fold cross-validation to determine the optimal parameter configuration, and ‘typ.measure’ was set to ‘deviance’. The non-zero coefficient features were determined based on the λ value corresponding to a standard error of the minimum distance deviation. The development of the prediction model involved performing multivariable logistic regression analysis, and the optimal model was obtained by stepwise regression (33,34).
Following the establishment of the optimal model, the nomogram underwent bootstrapping validation using 1,000 bootstrap resamples. A calibration curve was plotted to evaluate the model’s calibration. Differential efficacy was evaluated by receiver operating characteristic (ROC) curve analysis, and clinical decision curve analysis (DCA) was performed to evaluate the clinical application value of the model. We then quantified the net income within the threshold probability range. Finally, the constructed model was verified in the validation set.
Results
Participant characteristics
A total of 516 patients were eligible for the study. Of these, 49 patients who underwent tracheal intubation without a visual laryngoscope were excluded, and another 11 patients were excluded because they could not follow instructions to perform standard actions. Finally, 456 patients aged between 18 and 82 years old, including 287 females and 169 males, were enrolled in the study (Figure 3). The incidence of difficult laryngoscopy (CL grade of 3–4) was 6.4% (29 patients). Some 8 patients were intubated using an alternative device, such as a fiberoptic bronchoscope. No complications occurred during tracheal intubation. The characteristics of patients in the training and validation sets are shown in Table S2 and Table S3, respectively.
Feature selection
Univariate binary logistic regression analysis was applied for all variables. A cutoff P value of 0.1 was utilized, resulting in the identification of 23 variables associated with difficult laryngoscopy, as listed in Table 1. Through the utilization of LASSO analysis and stepwise regression, a 4-variable [inter-incisor gap (IIG), thyromental distance (TMD), DSTR, and airway-related diseases] analysis was formulated as the optimal prediction model (Figure 4). The thresholds, AUCs and odds ratios (ORs) of the included variables are shown in Table 2.
Table 1
Variable | Regression coefficient | P value |
---|---|---|
ASA-PS | ||
1 | ||
2 | 1.309 | 0.032 |
3 | −14.599 | 0.992 |
4 | −14.599 | 0.997 |
Age | 0.048 | 0.001 |
BMI | 0.135 | 0.03 |
Education | ||
1 | ||
2 | −1.569 | 0.025 |
3 | −1.303 | 0.016 |
HBP (%) | 1.339 | 0.051 |
Airway-related diseases (%) | 1.992 | 0.001 |
Smoking (%) | 1.019 | 0.052 |
Tumor (%) | 1.768 | 0.005 |
NC | 0.141 | 0.027 |
ULBT | ||
1 | ||
2 | 0.929 | 0.074 |
3 | 1.216 | 0.149 |
MMT | 1.225 | 0.002 |
IIG | −1.204 | <0.001 |
LT | −0.539 | 0.03 |
THD | −0.736 | 0.003 |
TMD | −1.013 | <0.001 |
SMD | −0.438 | 0.001 |
JD | −0.995 | 0.029 |
DSCM | 4.747 | <0.001 |
DSE | 1.214 | 0.054 |
DSTI | 2.168 | 0.017 |
DSHB | 2.737 | 0.001 |
DSAC | 2.69 | 0.018 |
DSTR | 2.397 | <0.001 |
LASSO, least absolute shrinkage and selection operator; ASA-PS, American Society of Anesthesiologists Physical Status; BMI, body mass index; HBP, hypertension; NC, neck circumference; ULBT, upper lip bite test; MMT, modified Mallampati test; IIG, inter-incisor gap; LT, length of tongue; THD, thyroid and hyoid distance; TMD, thyromental distance; SMD, sternomental distance; JD, jaw depth; DSCM, distance from skin to cricothyroid membrane; DSE, distance from skin to epiglottis; DSTI, distance from skin to thyroid isthmus; DSHB, distance from skin to the hyoid bone; DSAC, distance from skin to anterior commissure of the vocal cord; DSTR, distance from the skin to the tongue root.
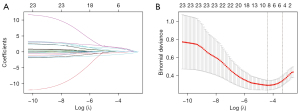
Table 2
Variable | Threshold | AUC (95% CI) | OR (95% CI) | P value |
---|---|---|---|---|
Airway-related diseases | 0.500 | 0.614 (0.507, 0.721) | 10.246 (2.133, 53.319) | 0.004 |
IIG | 4.150 | 0.798 (0.687, 0.909) | 0.276 (0.135, 0.488) | <0.001 |
TMD | 9.550 | 0.781 (0.694, 0.868) | 0.444 (0.205, 0.877) | 0.027 |
DSTR | 3.950 | 0.844 (0.736, 0.953) | 7.797 (2.379, 32.509) | 0.002 |
AUC, area under the curve; OR, odds ratio; CI, confidence interval; IIG, inter-incisor gap; TMD, thyromental distance; DSTR, distance from the skin to the tongue root.
Prediction model
An independent predictive model was developed and represented as a nomogram, incorporating relevant predictors (Figure 5). The predictive capability of the model for difficult laryngoscopy was assessed using ROC curve analysis. In the training set, the AUC value of the prediction model was 0.933 [95% confidence interval (CI): 0.770 to 0.935] with a sensitivity of 0.889 (95% CI: 0.744 to 1.000), a specificity of 0.860 (95% CI: 0.821 to 0.900), and an accuracy of 0.862 (95% CI: 0.861 to 0.863). Meanwhile, in the validation set, the AUC value of the prediction model was 0.956 (95% CI: 0.915 to 0.997), with a sensitivity of 1.000 (95% CI: 1.000 to 1.000), a specificity of 0.817 (95% CI: 0.750 to 0.885), and an accuracy of 0.832 (95% CI: 0.830 to 0.834) (Figure 6, Table 3).
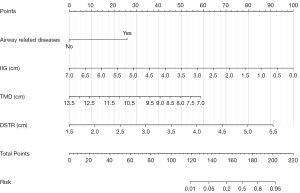
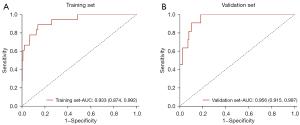
Table 3
Dataset | AUC (95% CI) | Specificity (95% CI) | Sensitivity (95% CI) | Accuracy (95% CI) |
---|---|---|---|---|
Training set | 0.933 (0.874, 0.992) | 0.889 (0.744, 1.000) | 0.860 (0.821, 0.900) | 0.862 (0.861, 0.863) |
Validation set | 0.956 (0.915, 0.997) | 1.000 (1.000, 1.000) | 0.817 (0.750, 0.885) | 0.832 (0.830, 0.834) |
AUC, area under the curve; CI, confidence interval.
The calibration plot revealed good predictive accuracy between the actual probability and predicted probability (Figure 7). The DCA showed that intervention (i.e., advanced difficult airway strategy) in patients based on the prediction model leads to greater benefits than the alternative strategies, except for a small range of low preferences (Figure 8).
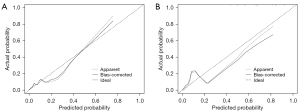
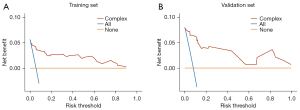
Sensitivity analysis
By performing saturation analysis, saturation level was reached at about 70% of our current sample size (Figure 9). In other words, a larger sample size would not produce any different result, and the current sample size is sufficient to represent the statistical stability of a larger sample size.
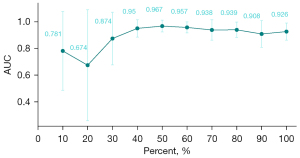
Discussion
Difficult laryngoscopy is the main reason for unanticipated difficult airways, and represents a challenge for anesthesiologists. Recently, a study developed a nomogram based on direct laryngoscope to predict difficult laryngoscopy and difficult intubation (35). However, as the utilization of visual laryngoscope increases, that of direct laryngoscope is gradually decreasing worldwide, diminishing the suitability of a direct laryngoscope-based nomogram for current clinical practice. This article reports the first time that most of the bedside examination tests and ultrasonographic measurements were combined to develop and validate a visual laryngoscope-based nomogram for clinical application. In this study, we evaluated the value of the nomogram for predicting difficult laryngoscopy at visual laryngoscopy and found a high correlation between CL grade and 4 clinical variables, including IIG, TMD, DSTR, and airway-related diseases. After multivariable logistic regression analysis, we developed a novel nomogram. The internal validation revealed good discrimination and correction ability. The high AUC value obtained from the interval validation demonstrated that the nomogram could be frequently and precisely used.
The utilization of video laryngoscope has steadily increased and is gradually replacing direct laryngoscope. However, no single device can solve all problems. It is notable that the utilization of awake intubation techniques has not declined significantly since the introduction of video laryngoscopy (36), indicating that visual laryngoscopy is still not a substitute for awake intubation techniques (37). We also noticed that most of the current studies on difficult airways still utilize direct laryngoscopy, which does not adhere to the clinical practice (24,38,39). Ultrasonography may facilitate new directions in difficult airway research when standard airway assessment provides inadequate predictive performance. Ultrasound has become an important tool in the operating room and intensive care unit to provide important information for diagnosis and prediction (40-42). In recent years, the application of ultrasound in airway management has gradually increased. It can be used to determine the correct position of the endotracheal tube (43,44), predict the suitable diameter of the endotracheal tube (45,46), and determine the position of the cricothyroid membrane for the front-of-neck airway (47). Several authors have used ultrasound to predict difficult intubation or difficult laryngoscopy (23,24,27,38,48); however, there is still no consensus regarding which ultrasound assessment is the best predictor, and high-quality evidence is also scarce.
We found that the DSTR was significant for predicting the classification of CL grade upon visual laryngoscopy. Most previous studies have measured the maximum thickness of the tongue (38,48,49), and their results showed that a thicker tongue (>6.1 cm, evaluated by ultrasonography) was an independent predictor for difficult tracheal intubation and difficult laryngoscopy, especially for direct laryngoscopy (24). However, most previous studies have used direct laryngoscope, rather than visual laryngoscope, during tracheal intubation (24,38,39). Visual laryngoscopes and direct laryngoscopes differ in their approach to laryngeal exposure. When using a direct laryngoscope, the anesthesiologist's view of the larynx is angled. At this moment, the largest factor interfering with laryngeal exposure is the midsection of the tongue, which is generally the maximum thickness of the tongue. When using a visual laryngoscope, the laryngoscope blade has crossed over the midsection of the tongue and the camera (the camera is usually placed at the front of the blade) is positioned relatively horizontal to the larynx. Thus, maximum tongue thickness is no longer an influencing factor for DL, whereas DSTR has the greatest effect on laryngeal exposure.
The maximum thickness of the tongue varied greatly when the patient was awake, and even when the patient had been instructed to maintain a uniform posture (keeping their mouth closed and placing the tongue tip to slightly touch the incisors, with the tongue relaxed) (24). There is a significantly greater variability between the maximum thickness of the tongue (3.5±2.5 vs. 1.2±3.4 mm, P=0.018) depending on whether the specified posture is performed or not (50). The tongue root is fixed by muscles, ligaments, lingual frenulum, and other structures. Therefore, the DSTR has less variation than the maximum thickness of the tongue, and the result based on the DSTR has lower heterogeneity and better prediction performance. Overall, the high correlation between DSTR and visual laryngoscopy and the low variability were 2 important reasons for utilizing this factor as a modeling variable.
Several studies have shown a correlation between difficult airways and the increased thickness of pretracheal soft tissue (51), which is not consistent with our present results. Studies have also indicated that a soft tissue thickness of the anterior neck at the thyrohyoid plane >2.8 cm is correlated with the difficulty of laryngoscope exposure, and the DSE of 2–2.5 cm had the highest accuracy in predicting difficult laryngoscopy (23,25,52,53). However, although the soft tissue thickness (DSTJ, DSTI, DSCM, DSAC, and DSHB) and DSE were also considered to have predictive value in our study, their predictive power was lower than that of the IIG and TMD. There are 2 possible reasons for such a difference. First, as mentioned earlier, most of the previous studies were based on direct laryngoscopy rather than visual laryngoscopy, and the visualization approach of these 2 laryngoscopes is different. Second, ultrasound results are strongly examiner-dependent, and each examiner varies in technique and experience.
Our results indicated that the IIG and TMD had the best discrimination ability of the bedside examination tests. The institution that conducted this study is a medical institution that specializes in stomatology. Therefore, many of the patients included in this study had oral and maxillofacial diseases, such as temporomandibular joint disease, which presents with limited mouth opening. During the research process, 8 patients were intubated using an alternative device, among which 6 patients were intubated with a fiberoptic bronchoscope because their IIGs were very small (or even zero). The increased incidence of limited mouth opening is significant for IIG in predicting the classification of the CL grade. A short IIG refers to impaired mouth opening. When IIG is limited, it is difficult to insert the laryngoscope blade. TMD is another common bedside examination test; during the measurement, the anesthesiologist needs to measure the distance from the chin process to the notch of the thyroid cartilage with the patient's neck extended. A short TMD represents impaired neck mobility or a short neck, which lead to difficult airways. Previous studies have yielded similar results and conclusions regarding TMD (54-56).
Some diseases can lead to challenges in airway management. In our study, rheumatoid arthritis, ankylosing spondylitis, degenerative osteoarthritis, obstructive sleep apnea syndrome, Pierre–Robin syndrome, Klippel–Feil syndrome, Treacher–Collins syndrome, Down’s syndrome, and airway masses were considered airway-related diseases. This list of airway-related diseases is primarily based on the 2022 American Society of Anesthesiologists Practice Guidelines for Management of the Difficult Airway, which are currently the most authoritative list of recommendations worldwide (11). These diseases may result in upper airway obstruction, abnormal airway anatomy, and limited neck mobility in patients. After multivariable logistic regression analysis, airway-related diseases showed a high correlation with the AUC between the CL grade at visual laryngoscopy. Almost all consensuses of experts, guidelines, and systematic reviews with high quality and high recognition agree that a history of related diseases plays a critical role in difficult airways (1,2,11,12,57-59), and we reached the same conclusion. However, the airway anatomy is so complex that even the most authoritative guidelines currently do not cover all difficult airway-related diseases.
By dividing the dataset into the training and validation set and obtaining high AUC values on both datasets, the robustness of the model was validated. In addition, after performing saturation analysis, we found that the model sample size has similar predictive performance at about 70% of the samples with 100% of the samples, which further demonstrates the robustness of the model and the adequacy of the sample size (31). Compared with another similar study, our model has similar performance with lower model complexity (35), resulting in easier generalization and application. However, because this study is a single-center prospective study, it is almost impossible to collect information from other centers, and therefore no external validation could be performed to demonstrate its generalizability and transportability.
The model developed in our study included only 4 risk factors, but achieved a very high predictive performance, which is very helpful for clinical practice. However, it may still be unrealistic to perform ultrasound measurements for each patient. We suggest performing an additional DSTR measurement in a patient with suspected difficult laryngoscopy to further validate the risk of difficult airway in that patient. In actual clinical practice, the anesthesiologist's judgment of a difficult airway is often based on experience, especially if the bedside examination results are ambiguous. Generally, the anesthesiologist has 2 options. The first option is to attempt tracheal intubation and then perform airway rescue after a difficult airway has occurred (intervention for none in DCA). The second option is to immediately pre-prepare for difficult airway resuscitation or directly perform awake intubation techniques (intervention for all in DCA). Neither strategy is the best option, because the former is too conservative, and the latter is too aggressive. At this moment, it may be a good strategy to perform an ultrasound examination and assess the patient's airway risk based on the nomogram, as ultrasound is available and completely non-radioactive.
Our study has several limitations. Firstly, our study included a relatively small sample size. Larger patient populations are needed to explore further applications of the comprehensive model in patients with difficult laryngoscopy. Secondly, our study has the inherent limitations of a single-center study, including a restricted patient population and the unavoidable risk of bias, which may limit the generalizability of our results to the global population. In the future, we plan to expand the sample size and study population and introduce deep learning to develop artificial intelligence-based predictive models for further research.
Conclusions
Compared with a single predictor of difficult laryngoscopy, the comprehensive model and nomogram, especially through the integration of tongue root thickness, can predict the risk of difficult laryngoscopy more accurately and reliably, allowing for reasonable individualized regimen decision-making.
Acknowledgments
Funding: This study was supported by the Clinical Research Programs of 9th People’s Hospital, Shanghai Jiao Tong University School of Medicine (No. JYLJ202013), the Cross-disciplinary Programs of 9th People’s Hospital, Shanghai Jiao Tong University School of Medicine (No. JYJC202133), and Faculty Development Training Program of Shanghai Jiao Tong University School of Medicine (No. JFXM202109).
Footnote
Reporting Checklist: The authors have completed the TRIPOD reporting checklist. Available at https://qims.amegroups.com/article/view/10.21037/qims-23-95/rc
Conflicts of Interest: All authors have completed the ICMJE uniform disclosure form (available at https://qims.amegroups.com/article/view/10.21037/qims-23-95/coif). The authors have no conflicts of interest to declare.
Ethical Statement: The authors are accountable for all aspects of the work in ensuring that questions related to the accuracy or integrity of any part of the work are appropriately investigated and resolved. The study was conducted in accordance with the Declaration of Helsinki (as revised in 2013). The Ethics Committee of Shanghai Ninth People’s Hospital, Shanghai, China approved the research protocol (No. SH9H-2021-T356-2). Written informed consent forms were obtained from the patients before enrolment in this prospective nested case-control study.
Open Access Statement: This is an Open Access article distributed in accordance with the Creative Commons Attribution-NonCommercial-NoDerivs 4.0 International License (CC BY-NC-ND 4.0), which permits the non-commercial replication and distribution of the article with the strict proviso that no changes or edits are made and the original work is properly cited (including links to both the formal publication through the relevant DOI and the license). See: https://creativecommons.org/licenses/by-nc-nd/4.0/.
References
- Frerk C, Mitchell VS, McNarry AF, Mendonca C, Bhagrath R, Patel A, O'Sullivan EP, Woodall NM, Ahmad I. Difficult Airway Society intubation guidelines working group. Difficult Airway Society 2015 guidelines for management of unanticipated difficult intubation in adults. Br J Anaesth 2015;115:827-48. [Crossref] [PubMed]
- Apfelbaum JL, Hagberg CA, Caplan RA, Blitt CD, Connis RT, Nickinovich DG, Hagberg CA, Caplan RA, Benumof JL, Berry FA, Blitt CD, Bode RH, Cheney FW, Connis RT, Guidry OF, Nickinovich DG, Ovassapian AAmerican Society of Anesthesiologists Task Force on Management of the Difficult Airway. Practice guidelines for management of the difficult airway: an updated report by the American Society of Anesthesiologists Task Force on Management of the Difficult Airway. Anesthesiology 2013;118:251-70. [Crossref] [PubMed]
- Sajayan A, Nair A, McNarry AF, Mir F, Ahmad I, El-Boghdadly K. Analysis of a national difficult airway database. Anaesthesia 2022;77:1081-8. [Crossref] [PubMed]
- Xu Z, Ma W, Hester DL, Jiang Y. Anticipated and unanticipated difficult airway management. Curr Opin Anaesthesiol 2018;31:96-103. [Crossref] [PubMed]
- Hove LD, Steinmetz J, Christoffersen JK, Møller A, Nielsen J, Schmidt H. Analysis of deaths related to anesthesia in the period 1996-2004 from closed claims registered by the Danish Patient Insurance Association. Anesthesiology 2007;106:675-80. [Crossref] [PubMed]
- Cook TM, Scott S, Mihai R. Litigation related to airway and respiratory complications of anaesthesia: an analysis of claims against the NHS in England 1995-2007. Anaesthesia 2010;65:556-63. [Crossref] [PubMed]
- Cook TM, MacDougall-Davis SR. Complications and failure of airway management. Br J Anaesth 2012;109:i68-85. [Crossref] [PubMed]
- Cumberworth A, Lewith H, Sud A, Jefferson H, Athanassoglou V, Pandit JJ. Major complications of airway management: a prospective multicentre observational study. Anaesthesia 2022;77:640-8. [Crossref] [PubMed]
- Myatra SN, Divatia JV, Brewster DJ. The physiologically difficult airway: an emerging concept. Curr Opin Anaesthesiol 2022;35:115-21. [Crossref] [PubMed]
- Langeron O, Cuvillon P, Ibanez-Esteve C, Lenfant F, Riou B, Le Manach Y. Prediction of difficult tracheal intubation: time for a paradigm change. Anesthesiology 2012;117:1223-33. [Crossref] [PubMed]
- Apfelbaum JL, Hagberg CA, Connis RT, Abdelmalak BB, Agarkar M, Dutton RP, Fiadjoe JE, Greif R, Klock PA, Mercier D, Myatra SN, O'Sullivan EP, Rosenblatt WH, Sorbello M, Tung A. 2022 American Society of Anesthesiologists Practice Guidelines for Management of the Difficult Airway. Anesthesiology 2022;136:31-81. [Crossref] [PubMed]
- Detsky ME, Jivraj N, Adhikari NK, Friedrich JO, Pinto R, Simel DL, Wijeysundera DN, Scales DC. Will This Patient Be Difficult to Intubate?: The Rational Clinical Examination Systematic Review. JAMA 2019;321:493-503. [Crossref] [PubMed]
- Roth D, Pace NL, Lee A, Hovhannisyan K, Warenits AM, Arrich J, Herkner H. Airway physical examination tests for detection of difficult airway management in apparently normal adult patients. Cochrane Database Syst Rev 2018;5:CD008874. [Crossref] [PubMed]
- Vannucci A, Cavallone LF. Bedside predictors of difficult intubation: a systematic review. Minerva Anestesiol 2016;82:69-83. [PubMed]
- Naguib M, Scamman FL, O'Sullivan C, Aker J, Ross AF, Kosmach S, Ensor JE. Predictive performance of three multivariate difficult tracheal intubation models: a double-blind, case-controlled study. Anesth Analg 2006;102:818-24. [Crossref] [PubMed]
- Lundstrøm LH, Vester-Andersen M, Møller AM, Charuluxananan S, L'hermite J, Wetterslev J. Danish Anaesthesia Database. Poor prognostic value of the modified Mallampati score: a meta-analysis involving 177 088 patients. Br J Anaesth 2011;107:659-67. [Crossref] [PubMed]
- Lee HC, Kim MK, Kim YH, Park HP. Radiographic Predictors of Difficult Laryngoscopy in Acromegaly Patients. J Neurosurg Anesthesiol 2019;31:50-6. [Crossref] [PubMed]
- Grimes D, MacLeod I, Taylor T, O'Connor M, Sidebottom A. Computed tomography as an aid to planning intubation in the difficult airway. Br J Oral Maxillofac Surg 2016;54:80-2. [Crossref] [PubMed]
- Han Y, Fang J, Zhang H, Xu M, Guo X. Anterior neck soft tissue thickness for airway evaluation measured by MRI in patients with cervical spondylosis: prospective cohort study. BMJ Open 2019;9:e029987. [Crossref] [PubMed]
- Kristensen MS, Teoh WH, Graumann O, Laursen CB. Ultrasonography for clinical decision-making and intervention in airway management: from the mouth to the lungs and pleurae. Insights Imaging 2014;5:253-79. [Crossref] [PubMed]
- Ji C, Ni Q, Chen W. Diagnostic accuracy of radiology (CT, X-ray, US) for predicting difficult intubation in adults: A meta-analysis. J Clin Anesth 2018;45:79-87. [Crossref] [PubMed]
- Carsetti A, Sorbello M, Adrario E, Donati A, Falcetta S. Airway Ultrasound as Predictor of Difficult Direct Laryngoscopy: A Systematic Review and Meta-analysis. Anesth Analg 2022;134:740-50. [Crossref] [PubMed]
- Falcetta S, Cavallo S, Gabbanelli V, Pelaia P, Sorbello M, Zdravkovic I, Donati A. Evaluation of two neck ultrasound measurements as predictors of difficult direct laryngoscopy: A prospective observational study. Eur J Anaesthesiol 2018;35:605-12. [Crossref] [PubMed]
- Yao W, Wang B. Can tongue thickness measured by ultrasonography predict difficult tracheal intubation? Br J Anaesth 2017;118:601-9. [Crossref] [PubMed]
- Parameswari A, Govind M, Vakamudi M. Correlation between preoperative ultrasonographic airway assessment and laryngoscopic view in adult patients: A prospective study. J Anaesthesiol Clin Pharmacol 2017;33:353-8. [Crossref] [PubMed]
- Wang X, Wang Y, Zheng ZW, Liu YR, Ma WH. Ultrasound measurements for evaluation of changes in upper airway during anaesthesia induction and prediction difficult laryngoscopy: a prospective observational study. Sci Rep 2022;12:18564. [Crossref] [PubMed]
- Alessandri F, Antenucci G, Piervincenzi E, Buonopane C, Bellucci R, Andreoli C, Alunni Fegatelli D, Ranieri MV, Bilotta F. Ultrasound as a new tool in the assessment of airway difficulties: An observational study. Eur J Anaesthesiol 2019;36:509-15. [Crossref] [PubMed]
- Altun D, Kara H, Bozbora E, Ali A, Dinç T, Sonmez S, Buget M, Aydemir L, Basaran B, Tuğrul M, Çamci E. The Role of Indirect Laryngoscopy, Clinical and Ultrasonographic Assessment in Prediction of Difficult Airway. Laryngoscope 2021;131:E555-60. [Crossref] [PubMed]
- Pinto J, Cordeiro L, Pereira C, Gama R, Fernandes HL, Assunção J. Predicting difficult laryngoscopy using ultrasound measurement of distance from skin to epiglottis. J Crit Care 2016;33:26-31. [Crossref] [PubMed]
- Cormack RS. Cormack-Lehane classification revisited. Br J Anaesth 2010;105:867-8. [Crossref] [PubMed]
- Chen W, Qian W, Wu G, Chen W, Xian B, Chen X, Cao Y, Green CD, Zhao F, Tang K, Han JD. Three-dimensional human facial morphologies as robust aging markers. Cell Res 2015;25:574-87. [Crossref] [PubMed]
- Wang C, Li Q, Song X, Dong X. Bayesian adaptive lasso for additive hazard regression with current status data. Stat Med 2019;38:3703-18. [Crossref] [PubMed]
- van Smeden M, de Groot JA, Nikolakopoulos S, Bertens LC, Moons KG, Reitsma JB. A generic nomogram for multinomial prediction models: theory and guidance for construction. Diagn Progn Res 2017;1:8. [Crossref] [PubMed]
- Jalali A, Alvarez-Iglesias A, Roshan D, Newell J. Visualising statistical models using dynamic nomograms. PLoS One 2019;14:e0225253. [Crossref] [PubMed]
- Wang B, Yao W, Xue Q, Wang M, Xu J, Chen Y, Zhang Y. Nomograms for predicting difficult airway based on ultrasound assessment. BMC Anesthesiol 2022;22:23. [Crossref] [PubMed]
- Benumof JL. Awake intubations are alive and well. Can J Anaesth 2015;62:723-6. [Crossref] [PubMed]
- Meitzen SE, Benumof JL. Video Laryngoscopy: Positives, Negatives, and Defining the Difficult Intubation. Anesth Analg 2019;128:399-401. [Crossref] [PubMed]
- Agarwal R, Jain G, Agarwal A, Govil N. Effectiveness of four ultrasonographic parameters as predictors of difficult intubation in patients without anticipated difficult airway. Korean J Anesthesiol 2021;74:134-41. [Crossref] [PubMed]
- Yadav NK, Rudingwa P, Mishra SK, Pannerselvam S. Ultrasound measurement of anterior neck soft tissue and tongue thickness to predict difficult laryngoscopy - An observational analytical study. Indian J Anaesth 2019;63:629-34. [Crossref] [PubMed]
- Diaz-Tormo C, Rodriguez-Martinez E, Galarza L. Airway Ultrasound in Critically Ill Patients: A Narrative Review. J Ultrasound Med 2022;41:1317-27. [Crossref] [PubMed]
- Lazar AE, Gherghinescu MC. Airway Ultrasound for Anesthesia and in Intensive Care Patients-A Narrative Review of the Literature. J Clin Med 2022; [Crossref] [PubMed]
- Xu J, Wang B, Wang M, Yao W, Chen Y. The value of multiparameter combinations for predicting difficult airways by ultrasound. BMC Anesthesiol 2022;22:311. [Crossref] [PubMed]
- Abbasi S, Farsi D, Zare MA, Hajimohammadi M, Rezai M, Hafezimoghadam P. Direct ultrasound methods: a confirmatory technique for proper endotracheal intubation in the emergency department. Eur J Emerg Med 2015;22:10-6. [Crossref] [PubMed]
- Ahmad I, Wong DJN. Recognising oesophageal intubation. Anaesthesia 2022;77:1321-5. [Crossref] [PubMed]
- Shibasaki M, Nakajima Y, Ishii S, Shimizu F, Shime N, Sessler DI. Prediction of pediatric endotracheal tube size by ultrasonography. Anesthesiology 2010;113:819-24. [Crossref] [PubMed]
- Gupta K, Gupta PK, Rastogi B, Krishan A, Jain M, Garg G. Assessment of the subglottic region by ultrasonography for estimation of appropriate size endotracheal tube: A clinical prospective study. Anesth Essays Res 2012;6:157-60. [Crossref] [PubMed]
- Bowness J, Teoh WH, Kristensen MS, Dalton A, Saint-Grant AL, Taylor A, Crawley S, Chisholm F, Varsou O, McGuire B. A marking of the cricothyroid membrane with extended neck returns to correct position after neck manipulation and repositioning. Acta Anaesthesiol Scand 2020;64:1422-5. [Crossref] [PubMed]
- Andruszkiewicz P, Wojtczak J, Sobczyk D, Stach O, Kowalik I. Effectiveness and Validity of Sonographic Upper Airway Evaluation to Predict Difficult Laryngoscopy. J Ultrasound Med 2016;35:2243-52. [Crossref] [PubMed]
- Hall EA, Showaihi I, Shofer FS, Panebianco NL, Dean AJ. Ultrasound evaluation of the airway in the ED: a feasibility study. Crit Ultrasound J 2018;10:3. [Crossref] [PubMed]
- Chen JW, Chang CH, Wang SJ, Chang YT, Huang CC. Submental ultrasound measurement of dynamic tongue base thickness in patients with obstructive sleep apnea. Ultrasound Med Biol 2014;40:2590-8. [Crossref] [PubMed]
- Dong F, Wang Y, Wang X, Zhao H, Ma W. Changes in the upper airway following induction of anaesthesia: a prospective observational study protocol to determine the use of ultrasound in the assessment of a difficult airway in China. BMJ Open 2019;9:e029782. [Crossref] [PubMed]
- Koundal V, Rana S, Thakur R, Chauhan V, Ekke S, Kumar M. The usefulness of point of care ultrasound (POCUS) in preanaesthetic airway assessment. Indian J Anaesth 2019;63:1022-8. [Crossref] [PubMed]
- Martínez-García A, Guerrero-Orriach JL, Pino-Gálvez MA. Ultrasonography for predicting a difficult laryngoscopy. Getting closer. J Clin Monit Comput 2021;35:269-77. [Crossref] [PubMed]
- Chhina AK, Jain R, Gautam PL, Garg J, Singh N, Grewal A. Formulation of a multivariate predictive model for difficult intubation: A double blinded prospective study. J Anaesthesiol Clin Pharmacol 2018;34:62-7. [Crossref] [PubMed]
- Khan ZH, Mohammadi M, Rasouli MR, Farrokhnia F, Khan RH. The diagnostic value of the upper lip bite test combined with sternomental distance, thyromental distance, and interincisor distance for prediction of easy laryngoscopy and intubation: a prospective study. Anesth Analg 2009;109:822-4. [Crossref] [PubMed]
- Iohom G, Ronayne M, Cunningham AJ. Prediction of difficult tracheal intubation. Eur J Anaesthesiol 2003;20:31-6. [Crossref] [PubMed]
- Kornas RL, Owyang CG, Sakles JC, Foley LJ, Mosier JMSociety for Airway Management’s Special Projects Committeetab. Evaluation and Management of the Physiologically Difficult Airway: Consensus Recommendations From Society for Airway Management. Anesth Analg 2021;132:395-405. [Crossref] [PubMed]
- Popat M, Mitchell V, Dravid R, Patel A, Swampillai C, Higgs A. Difficult Airway Society Guidelines for the management of tracheal extubation. Anaesthesia 2012;67:318-40. [Crossref] [PubMed]
- Heidegger T. Management of the Difficult Airway. N Engl J Med 2021;384:1836-47. [Crossref] [PubMed]