DeepKOA: a deep-learning model for predicting progression in knee osteoarthritis using multimodal magnetic resonance images from the osteoarthritis initiative
Introduction
Knee osteoarthritis (KOA) is one of the most common chronic illnesses worldwide. However, no disease-modifying drugs are licensed for the treatment of KOA (1), and thus represents a significant burden to both families and society (2). In an ideal scenario, it would be beneficial to select patients who show disease progression within the osteoarthritis (OA) drug development trial period due to their robust response to the drug, thereby reducing the sample size and shortening the follow-up period in clinical trials. Conversely, patients who show no progression should not be selected (3). Current studies are focused on identifying individuals at high risk of disease progression for drugs trials to accelerate the development of disease-modifying drugs. Additionally, the early identification of these individuals would prompt the initiation of physical therapy which can significantly improve their prognosis (4,5).
Regarding the prediction of KOA progression, the most common predictors are clinical variables, biomechanical features, laboratory biomarkers, and imaging assessments and outcomes (4-17). The accuracy of clinical characteristics such as personal history or sensory and motor function assessed by multiple scales may be hampered by the bias of patients’ memories and feelings. The acquisition of biomolecular or laboratory biomarkers derived from synovial fluid, peripheral blood, or urine requires complicated techniques and cannot indicate the relevant structures that may be responsible for disease progression. In contrast, magnetic resonance imaging (MRI) is capable of sensitively reflecting the subtle changes in the intricate architecture of the knee, such as the cartilage volume, cartilage defect, and meniscus lesions (18,19). To date, various methods have been proposed to extract predictive information underlying OA progression from MRI, including semiquantitative or quantitative analysis of OA-related structural features through traditional statistics or machine-learning algorithms (8,10,14,20-27). Prior to this, manual segmentation of specific structures or individual scoring was required, which was laborious and depended on experts’ experience, making them inconvenient for real-world clinical practice.
Deep learning is a subset of machine learning that has been used for various musculoskeletal imaging tasks, such as structure segmentation, image reconstruction, and lesion detection (17,28-30). In the field of KOA, deep-learning models have been employed to predict the onset of KOA and total knee replacement via MRI and to predict OA progression through radiographs or clinical risk factors (4,5,9,17,31-34), The former method, referring to radiography, has long been the main approach for detecting structural changes in OA, but there is a limitation related to its low sensitivity to disease progression and the inability to image pathologies in nonosseous structures, such as the cartilage, meniscus, and synovium (35). In the latter method, there may be limitations in identifying the responsible structures, and few studies have examined the use of deep-learning methods and MR images to predict KOA progression. Panfilov et al. (36) fed MR images into a predictive model to predict radiographic KOA progression [defined according Kellgren-Lawrence grade (KLG) change] within the next 8 years (96 months). However, joint space narrowing remains the current gold standard for measuring clinical efficacy and the imaging endpoint for clinical trials in disease-modifying OA drugs (DMOADs) (37). Schiratti et al. (9) applied a deep-learning model and 2-dimensional (2D) MRI on a relatively large population, but this model was found to not be particularly good at predicting the structural progression of the following year according to joint space width (JSW). Both studies used a structural outcome measure but did not include symptoms in as an outcome variable, resulting in an inaccurate assessment of OA. This is because the presence of radiographic OA may be discrepant with the presence of other structural findings and related symptoms (38). Current imaging-based deep-learning models often rely on manually segmented lesions as inputs. In accounting for OA, morphological changes in knee cartilage, meniscal lesions, synovitis and synovial fluid effusion, and bone marrow lesions should all be taken into account. However, performing voxel-level segmentation for all of these structures can be challenging and time-consuming (39). Additionally, if only some of these structures are considered, the interrelationship between them may be ignored, leading to a less comprehensive understanding of the disease. Therefore, in this study, we developed a deep-learning model (hereafter referred to as DeepKOA) to predict clinically relevant KOA progression (defined as both radiographic and pain progression) over 24–48 months using unlabeled 3-dimensional (3D) MRI of the whole knee at baseline, 12, and 24 months (40,41). We present this article in accordance with the TRIPOD reporting checklist (available at https://qims.amegroups.com/article/view/10.21037/qims-22-1251/rc).
Methods
Study population
The study was conducted in accordance with the Declaration of Helsinki (as revised in 2013). The Foundation for the National Institutes of Health (FNIH) OA Biomarkers Consortium undertook a nested case-control study (194 cases and 406 controls) within the osteoarthritis initiative (OAI), a unique longitudinal cohort with a publicly available repository of radiological images and clinical data obtained at annual clinic visits. Details of the study design have been described elsewhere (42). Briefly, eligible participants for this present study were those with at least 1 knee with a KLG of 1–3 at baseline and with available knee radiographs as well as knee MRI and clinical data at baseline, 12, 24, 36, and 48 months. One indexed knee was selected from each participant. Among the participants, 194 OA knees with radiographic and pain progression and 200 nonprogressive OA knees over 24–48 months compared with baseline were initially included as cases and controls, respectively, in this investigation. The exclusion criteria were incomplete MRI sequences and clinical data at any month (case group: n=12; control group: n=4). Moreover, the 2 groups were matched according to sex, age (±3 years), and body mass index (±3 kg/m2). As a result, 12 pairs of cases and controls were further excluded. Finally, 364 participants met the inclusion criteria, as shown in Figure 1.
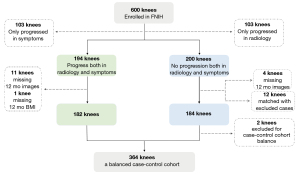
Definitions of radiographic and pain progression
Joint space narrowing, which could be measured by changes in JSW, has been proposed as the imaging endpoint for clinical trials in DMOADs and for assessing KOA structural progression (37). In this study, it was measured by automated software and defined as a loss in medial minimum JSW of ≥0.7 mm from baseline to 24, 36, or 48 months (43). This cutoff was based on the mean and standard deviation (SD) of changes during 1 year in 90 control knees who definitively did not have OA (KLG =0) and with the Western Ontario and McMaster Universities Osteoarthritis Index (WOMAC) pain scores of 0. Knee pain was assessed using the WOMAC pain subscale. Pain progression was defined as a persistent increase from baseline to 24, 36, or 48 months of ≥9 points on a 0–100 normalized score, an established minimum clinically important difference (MCID) for worsening pain (42).
Knee MRI acquisition
Examinations of the knees in the OAI were performed at 4 OAI clinical sites using 3.0 T MRI scanners (Trio, Siemens Healthineers, Erlangen, Germany). Two MRI sequences and two reconstruction positions were used, including sagittal intermediate-weighted turbo-spin echo sequences with fat-suppression (SAG-IW-TSE-FS), sagittal 3D dual-echo steady-state water excitation (SAG-3D-DESS-WE), axial multiplanar reformation 3D dual-echo steady-state water excitation (AXIAL-MPR-3D-DESS-WE), and coronal MPR-3D-DESS-WE (COR-MPR-3D-DESS-WE). SAG-IW-TSE-FS is one of the most strongly suggested MRI sequences for displaying all knee structures, except for osteophytes, especially for bone marrow lesions, articular cartilage, Hoffa synovitis, effusion synovitis, and ligaments. However, the bad display of osteophytes on SAG-IW-TSE-FS can be supplemented by axial, sagittal, and coronal 3D-DESS-WE (44). Additionally, it appears that 3D-DESS-WE can provide better cartilage discrimination than can SAG-IW-TSE-FS, especially on the AXIAL-MPR-3D-DESS-WE and COR-MPR-3D-DESS-WE. In this way, the tibial and central weight-bearing surface of the femoral cartilage (femoral condyle central region), as well as the trochlear (the anterior portion) and the patellar cartilage, could be optimally visualized. All the OAI pulse sequence protocols and parameters have been published in detail elsewhere (45). To eliminate the batch effect caused from the use of different scanners, we employed the max–min normalization for MRI input as follows:
where and are the minimal value and maximal value of input x, respectively.
Deep-learning model construction
DeepKOA was developed based on unlabeled knee MR images at baseline, 12, and 24 months using 3D DenseNet169 with 5-fold cross-validation (46,47). The patient-level progression status was identified as the ground truth. DenseNets have several compelling advantages, including the ability to alleviate the vanishing-gradient problem, strengthen feature propagation, encourage feature reuse, and substantially reduce the number of parameters. For each layer, the feature maps of all preceding layers are used as inputs, and their feature maps are used as inputs for all subsequent layers. Furthermore, each layer has direct access to the gradients from the loss function and the original input signal, leading to implicit deep supervision. Moreover, DenseNets require substantially fewer parameters and less computation to achieve state-of-the-art performances. The 3D DenseNets are compact versions requiring fewer parameters because of feature-map replication (48). The network architecture for 3D DenseNet is as follows: (I) the first layer is a convolution layer with an activation function and a pooling method; (II) after the first layer, there are 4 blocks for the convolution process, each block has 6, 12, 32, and 32 bottleneck layers, and each bottleneck layer consists of one 1×1×1 convolution layer and one 3×3×3 convolution layer; (III) there is a transition layer between each block; and (IV) the last layer is an average pool with a fully connected layer.
The output of the model was the progression risk estimate with a 1-dimension vector. Each separate MR sequence and the combination of MR sequences were considered as inputs. When using a single MRI sequence as input, we leveraged 1 network to extract the image features following a fully connected layer for binary progression prediction. For the combined MR sequences, we first used different networks with unshared weight to extract different modalities’ image features and then concatenated the extracted features together. There was a fully connected layer using the features for the progression prediction. Cross-entropy was chosen as our loss function to train the model. The processes of the networks and sequence combination are summarized in Figure 2A.
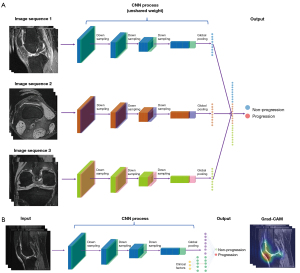
Model training was implemented in Python 3.7 and PyTorch 1.8.1 using a NVIDIA RTX 3090 24 GB graphics processing unit. We included several augmentation methods in the training process, including horizontal flip, vertical flip, and rotation. We evaluated the model performance via 5-fold cross-validation, and best model was selected based on the highest area under the curve (AUC) of the validation set. Adam optimizer was used with a learning rate of 1×10−4. The training batch size was 3 due to the limitation of computer resources. The training epoch was 50, with approximately 1 minute being required for 1 epoch training.
X-ray-based deep-learning method construction
In our study, we used ResNet200D as our X-ray-based model. The ResNet architecture comprises an input stem, 4 subsequent stages, and a final output layer. The input stem has a 7×7 convolution with 64 output channels and a stride of 2. This is followed by a 3×3 max pooling layer, which also has a stride of 2. The stem reduces the input width and height by 4 times while increasing its channel size to 64. Each stage following the stem begins with a downsampling block and then several residual blocks. The downsampling block consists of path A and path B. Path A has 3 convolutions with kernel sizes of 1×1, 3×3, and 1×1, respectively. The first convolution has a stride of 2 to halve the input width and height, whereas the last convolution's output channel is 4 times larger than the previous 2, which is known as a bottleneck structure. Path B uses a 1×1 convolution with a stride of 2 to transform the input shape into the output shape of path A, in which the output of both paths is summed to obtain the output of the downsampling block. A residual block is similar to a downsampling block but only uses convolutions with a stride of 1.
One can manipulate the number of residual blocks in each stage to achieve various ResNet models, such as ResNet-50 and ResNet-152. These models differ in the number of convolutional layers in the network. For our ResNet200D implementation, we introduced 2 modifications: (I) we switched the stride of the first convolution in path A with that of the second convolution. The first convolution then had a stride of 1, while the second convolution had a stride of 2. (II) In path B, we added a 2×2 average pooling layer with a stride of 2 before the convolution, whose stride was changed from 2 to 1.
Clinical models and integrated models
Clinical models were developed using multilayer perceptron based on 8 clinical variables, including age, sex, body mass index (BMI), KLG, history of knee injury (a knee injured to a degree that it limited the ability to walk for at least 2 days), history of surgery (knee surgery or arthroscopy about 12 months prior to the last visit), and family history (blood relatives with knee replacement surgery for arthritis, in which all or part of the knee joint was replaced), and WOMAC pain score. We then the built integrated models by combining MR images with clinical features. We processed the concatenated features using a batch normalization layer. Details of the integrated model construction are shown in Figure 2B.
Interpretation of the deep-learning model
Grad-CAMs were used to highlight the class-discriminative region in the MR images for predicting the decision of interest. We created heatmaps generated from MR images, which displayed the key regions for the DeepKOA to classify the nonprogressive and progressive KOA. Specifically, the network architecture would output a set of feature maps after extracting features from the raw images through the convolutional and the pooling layers. A pixel in the feature map corresponded to a region in the raw images. The contribution of a pixel to the final prediction was shown as the intensity of the pixel value in the heatmap, with the region with a higher pixel value indicating a greater influence (49). To further eliminate the influence of noise on the results, the pixel value of the heatmap was sorted in descending order, and pixels with the top 10% value were selected, whereas the other pixel values were set to zero. A board-certified radiologist (with 12 years of experience in musculoskeletal diagnoses) read heatmaps of all the true-positive cases slice by the slice at the 3 time points and recorded the structures contained within the heatmaps.
Statistical analysis
Continuous variables are presented as the mean and SD, while categorical variables were presented as numbers and percentages, which were analyzed using the Mann-Whitney test (including age, BMI, KLG, JSW, WOMAC pain scores) or χ2 tests (including gender, racial, injured history, family history, surgery history). Receiver operating characteristic (ROC) curves were used to evaluate the models’ performance in predicting KOA progression with the input of different MR sequences and different modalities (including images or/and clinical data) at different time points. The AUCs between the clinical models, X-ray-based deep learning models, and DeepKOA at 3 time points were compared using Wilcoxon signed rank tests (50-52). A 2-step nonparametric statistical test (Friedman test and Nemenyi post hoc test) with P values was used to compare AUCs of different MR sequences at the same time point. All statistical analyses were performed using the SPSS version 23.0 software (IBM Corp., Armonk, NY, USA). A P value <0.05 was considered statistically significant.
Results
Study characteristics
A total of 364 participants (182 per group) were screened from the FNIH cohort. Table 1 illustrates the comparison of clinical characteristics between the nonprogression and progression groups, and the results showed no significant differences in age, gender, BMI, race, history of injury, family history, history of surgery, WOMAC pain score, or JSW (all P values >0.05); however, KLG was significantly different (P=0.040).
Table 1
Characteristics | Progression (n=182) | Nonprogression (n=182) | P value |
---|---|---|---|
Age, years | 62±9 | 62±9 | 0.552 |
Gender, female, n (%) | 105 (57.7) | 120 (65.9) | 0.106 |
BMI, kg/m2 | 30.7±4.7 | 30.5±4.7 | 0.845 |
Race, n (%) | 0.302 | ||
Non-White | 34 (18.7) | 42 (23.1) | |
White | 148 (81.3) | 140 (76.9) | |
Injury history†, yes, n (%) | 66 (36.3) | 61 (33.5) | 0.582 |
Family history‡, yes, n (%) | 32 (17.6) | 24 (13.2) | 0.245 |
Surgery history§, yes, n (%) | 33 (18.1) | 33 (18.1) | >0.99 |
Kellgren-Lawrence grade, n (%) | 0.040 | ||
1 | 23 (12.6) | 24 (13.2) | |
2 | 77 (42.3) | 99 (54.4) | |
3 | 82 (45.1) | 59 (32.4) | |
WOMAC pain score | 1.9±2.5 | 2.7±3.3 | 0.097 |
Joint space width, mm | 3.8±1.4 | 3.9±1.0 | 0.172 |
Continuous variables are presented as mean ± SD, and categorical variables are presented as number (%). †, an injury sufficiently severe to limit the ability to walk for at least 2 days; ‡, having a blood relative who had knee replacement surgery for arthritis, where all or part of the knee joint was replaced; §, either knee underwent surgery or arthroscopy since the last visit about 12 months prior. WOMAC, Western Ontario and McMaster Universities Osteoarthritis Index; BMI, body mass index; SD, standard deviation.
Classification performance of DeepKOA
The performance of DeepKOA based on single or combined MR sequences in predicting future KOA progression over 24–48 months is presented in Table 2 and Figure 3. At baseline and 24 months, no significant AUC differences were observed between the single and combined MRI-based models (P=0.130 and P=0.050, respectively). At 12 months, the AUCs of DeepKOA built with single and combined MR sequences were significantly different (P=0.021). However, further pairwise comparisons indicated that the AUC of SAG-IW-TSE-FS was similar to that of the combined sequences including SAG-IW-TSE-FS (P>0.05) but higher than that of COR-MPR-3D-DESS-WE (P=0.011) and SAG-3D-DESS-WE (P=0.028). There were no significant AUC differences between other single and combined MRI sequences.
Table 2
MRI sequences | Baseline | 12 months | 24 months |
---|---|---|---|
SAG-IW-TSE-FS | 0.664 (0.585–0.743) | 0.739 (0.703–0.775) | 0.775 (0.686–0.865) |
COR-MPR-3D-DESS-WE | 0.632 (0.582–0.682) | 0.687 (0.632–0.742) | 0.737 (0.667–0.807) |
AXIAL-MPR-3D-DESS-WE | 0.669 (0.634–0.703) | 0.715 (0.648–0.782) | 0.792 (0.711–0.873) |
SAG-3D-DESS-WE | 0.622 (0.573–0.670) | 0.694 (0.645–0.742) | 0.746 (0.691–0.802) |
Combination 1 | 0.669 (0.595–0.743) | 0.731 (0.707–0.754) | 0.781 (0.691–0.872) |
Combination 2 | 0.677 (0.601–0.754) | 0.741 (0.679–0.803) | 0.787 (0.721–0.854) |
Combination 1 indicates the integration of SAG-IW-TSE-FS and SAG-3D-DESS sequences. Combination 2 indicates the integration of SAG-IW-TSE-FS, AXIAL-MPR-SAG-3D-DESS-WE, and COR-MPR-SAG-3D-DESS-WE sequences. Numbers in parentheses are the 95% CI. MR, magnetic resonance; MRI, magnetic resonance imaging; SAG-IW-TSE-FS, sagittal intermediate-weighted turbo-spin echo sequences with fat suppression; SAG-3D-DESS-WE, sagittal 3D dual-echo steady-state water excitation; AXIAL-MPR-3D-DESS-WE, axial multiplanar reformation 3D dual-echo steady-state water excitation; COR-MPR-3D-DESS-WE, coronal multiplanar reformation 3D dual-echo steady-state water excitation; 3D, 3-dimensional.
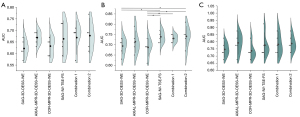
DeepKOA based on SAG-IW-TSE-FS achieved AUCs of 0.664 (95% CI: 0.585–0.743) at baseline, 0.739 (95% CI: 0.703–0.775) at 12 months, and 0.775 (95% CI: 0.686–0.865) at 24 months, with a statistical difference (P=0.015) in the AUCs between the time points. Furthermore, the AUCs of DeepKOA based on SAG-IW-TSE-FS between baseline and 24 months were significantly different (P=0.004). Moreover, the sensitivity (baseline: 58.8%, 95% CI: 53.1–64.5%; 12 months: 63.2%, 95% CI: 56.4–70.0%; 24 months: 66.5%, 95% CI: 57.2–75.9%) and specificity (baseline: 66.0%, 95% CI: 59.4–72.5%; 12 months: 70.9%, 95% CI: 62.5–79.2%; 24 months: 71.5%, 95% CI: 61.5–81.4%) of DeepKOA based on SAG-IW-TSE-FS increased with time, although there were no significant differences at the 3 timepoints (P=0.247 and P=0.104, respectively).
Comparison with the X-ray-based deep-learning method
The X-ray-based deep-learning method achieved AUCs of 0.573 (95% CI: 0.522–0.625) at baseline, 0.564 (95% CI: 0.531–0.597) at 12 months, and 0.613 (95% CI: 0.578–0.647) at 24 months, which were significantly inferior to those of DeepKOA (P=0.043 for all time points).
Clinical models and integrated models
The clinical models achieved AUCs of 0.561 (95% CI: 0.491–0.631), 0.605 (95% CI: 0.546–0.664), and 0.672 (95% CI: 0.631–0.713) at baseline, 12, and 24 months, respectively. After DeepKOA was combined with the clinical variables, the integrated models yielded AUCs of 0.639 (95% CI: 0.581–0.697), 0.709 (95% CI: 0.627–0.792), and 0.763 (95% CI: 0.668–0.858) at baseline, 12, and 24 months, respectively. Figure 4 shows the comparison between clinical models, DeepKOA, and the integrated models at baseline, 12, and 24 months. The addition of clinical variables could not improve the performance of DeepKOA at any time point (all P values >0.05).
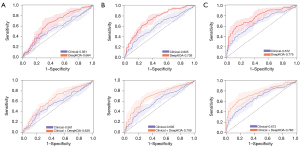
Interpretation of DeepKOA
Figure 5 displays the heatmaps of the correctly predicted and incorrectly predicted cases. We found that specific structural pathology, including cartilage damage, bone marrow lesions, meniscal tears, and hyperintensity in the infrapatellar fat pad and suprapatellar fat pad, as well as some regions with changes that could not be discriminated by the human eye, were highlighted on heatmaps of the correctly predicted cases. However, the hot spots in most incorrectly predicted cases were diffuse and could not be attributed to concrete structures. Figure 6 shows the proportions of the highlighted regions with the top 10% pixel values in true-positive samples at baseline, 12, and 24 months. We observed that the patellofemoral joint was highlighted with increasing frequency as over time, while decreasing frequency was found for the tibiofemoral joint. The meniscus, the infrapatellar fat pad, and muscles posterior to the knee were highlighted to varying degrees.
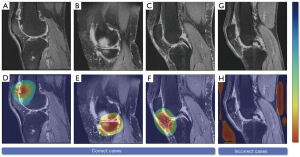
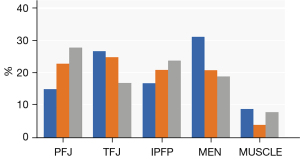
Discussion
In this study, the feasibility of multimodal MRI-based DeepKOA in forecasting the structural and symptomatic progression of KOA over 24–48 months was assessed. We first identified the SAG-IW-TSE-FS as the optimal sequence to develop a prediction model, the performance of which was very close to that of the combined MR sequences at any time point and superior to the deep-learning model built on X-ray. However, the accuracy of DeepKOA could not be further enhanced by the additional of clinical variables. The post hoc analysis using Grad-CAM revealed the tibiofemoral joint to be the main structure contributing to KOA progression at first but was later replaced by the patellofemoral joint. Moreover, the infrapatellar fat pad was consistently indicated as having an important role.
We did not aim to develop a superior KOA progression prediction model using MR images. Instead, the main goal of this study was to explore the predictive validity of MR images in combination with deep-learning analysis. Using 384 knees, the deep learning method was able to predict progression over a maximum time span of 48 months, demonstrating the potential value of using MR images to predict OA progression. Interestingly, the specificities were always superior to the sensitivities at each time point (baseline: 66.0% vs. 58.8%; 12 months: 70.9% vs. 63.2%; 24 months: 71.5% vs. 66.5%). This would be beneficial for differentiating patients who experience disease progression from those who would not and thus allow for the better selection of participants for novel disease-modifying drug trials.
The performance of DeepKOA was superior to that of the X-ray-based deep-learning method constructed on the same population. It has been shown that before the development of the first radiographic abnormalities, more than 10% of knee cartilage volume loss and over 40% of knee cartilage deficiency can be observed (19,53). Knee JSW loss, an indicator of radiographic progression, represents a variety of changes including abnormalities of cartilage, subchondral bone, and meniscus, all of which can be observed on MRI (20,54). Moreover, semiquantitative or quantitative measurement indices of these lesions detected on MRI have also been proven to be associated with KOA radiographic and pain progression (42,55,56). Therefore, as demonstrated in our investigation, there is promise for the possibility to predict future radiographic progression by detecting more sophisticated changes in the knee earlier on MRI before JSW narrowing can be detected on X-rays.
In our study, the proposed SAG-IW-TSE-FS sequence was found to be optimal for developing a prediction model, yield very similar AUCs to those of other single and combined MR sequences at baseline and 24 months without significant differences. Meanwhile, the combination of the SAG-IW-TSE-FS, AXIAL-MPR-SAG-3D-DESS-WE, and COR-MPR-SAG-3D-DESS-WE sequences at baseline tended to perform better. We can infer that the pathological changes were at evident at such an early time point (baseline) but could only be apparent in combining all the dominant sequences of all lesions; for instance, all the lesions, except osteophytes, were displayed better on SAG-IW-TSE-FS, and could be easily detected on axial, sagittal, and coronal 3D-DESS-WE (44). Previous animal OA models indicated that the first change in the development of OA was subchondral bone marrow lesion, with the cartilage degeneration and meniscal pathology potentially being mediated by biomechanical changes through the subchondral bone (57). Hence, at 12 months, a relatively early period, the model built on SAG-IW-TSE-FS most sensitively demonstrated the bone marrow lesion performed significantly better than did COR-MPR-SAG-3D-DESS-WE and SAG-MPR-SAG-3D-DESS-WE. However, cartilage degeneration may not be so obvious at this time such that even combined with axial, sagittal, and coronal DESS, the models’ performance could not be improved as compared to that of only SAG-IW-TSE-FS. Moreover, we deduced that for the development of OA, the main structures that function for KOA may change from the tibiofemoral joints at an early time point to the patellofemoral joints at a later time point. This may account for the fact that the AXIAL-MPR-SAG-3D-DESS-WE sequence, on which the patellofemoral joint was detected excellently in a plane orthogonal to the cartilage plate (45), tended to perform better than did the other single and combined sequences at 24 months.
We also extracted Grad-CAM from the 3D DenseNet169 architecture to preliminarily clarify its functional mechanism. According to the heatmaps of different time points, the tibiofemoral joint played a larger role at the earlier time point in the disease progression that was later superceded by the patellofemoral joint. No studies on this mechanism have been conducted thus far. We concluded that weight bearing and knee joint malalignment figure prominently in the progression of primary KOA (58), and these may primarily damage the tibiofemoral joints. While damage to the patellofemoral joint, particularly during activities requiring a high degree of flexion, may influence disease progression, it is not obvious at an early stage. This phenomenon could also explain why the AXIAL-MPR-SAG-3D-DESS-WE sequence, on which the patellofemoral joint was detected excellently in a plane orthogonal to the cartilage plate, tended to perform better than did the other single and combined sequences at 24 months. Although the relevance of the infrapatellar fat pad in KOA progression remains unclear and has been investigated less thoroughly, it was also highlighted in the early to late periods by the deep-learning method. It is hypothesized that infrapatellar fat pads secrete cytokines and adipokines, causing local inflammation to enter a vicious circle that affects surrounding tissues, eventually leading to a chronic and perpetually inflammatory state (59). Therefore, it is reasonable to indicate that our model successfully perceived information about the whole knee and initially demonstrated the responsible structures.
Our experiments also showed that feeding clinical data did not enhance performance in a model in which only the image modality was applied. This might be due to the fact that the image-only modality itself already achieved a relatively moderate accuracy for differentiation and accounted for the main contribution of the proposed multimodality method and the demographic and that clinical features were beneficial for improving the diagnostic performance and robustness.
There are some limitations in this study. First, the image quality might have affected the performance of the deep-learning model despite the fact that various centers performed the same scanning standards. Second, this study was performed on a single database and lacked external validation, as it was difficult to obtain available nested case-control and longitudinal follow-up data for OA investigation. However, we derived and internally validated the accuracy of DeepKOA through 5-fold cross-validation. Once externally validated, our model has the potential to facilitate management decisions by providing sequential risk estimates that can be used in conjunction with clinical judgment to improve the care of patients at high risk of progression. Third, the sample size of this study was too small to explore the differentiation between different levels of disease. Therefore, a large-scale cohort is needed to improve the performance of DeepKOA to analyze it further. Fourth, we did not explore the role of the structural subregions, such as the bone, cartilage, and meniscus in the prediction of KOA progression, as it is challenging to segment these subregions. Finally, pain progression was assessed using questionnaire-derived WOMAC scores, which may be subjective but unavoidable.
Conclusions
In conclusion, this present study established a deep-learning system called DeepKOA for predicting KOA progression based on the SAG-IW-TSE-FS sequence at different time points. The DeepKOA performed better than did the clinical models and X-ray-based deep-learning models. By using the fully automated and advanced deep-learning technology, our study provides insights for future development of more clinically practical methods.
Acknowledgments
The authors would like to acknowledge the participants in the OAI study for providing this unique open-access database.
Funding: This work received support from the President Foundation of the Third Affiliated Hospital of Southern Medical University (No. YM2021012).
Footnote
Reporting Checklist: The authors have completed the TRIPOD reporting checklist. Available at https://qims.amegroups.com/article/view/10.21037/qims-22-1251/rc
Conflicts of Interest: All authors have completed the ICMJE uniform disclosure form (available at https://qims.amegroups.com/article/view/10.21037/qims-22-1251/coif). JH, QY, LZ, YC, and XZ report that this work was supported by the President Foundation of the Third Affiliated Hospital of Southern Medical University (No. YM2021012). The other authors have no conflicts of interest to declare.
Ethical Statement: The authors are accountable for all aspects of the work in ensuring that questions related to the accuracy or integrity of any part of the work are appropriately investigated and resolved. The study was conducted in accordance with the Declaration of Helsinki (as revised in 2013).
Open Access Statement: This is an Open Access article distributed in accordance with the Creative Commons Attribution-NonCommercial-NoDerivs 4.0 International License (CC BY-NC-ND 4.0), which permits the non-commercial replication and distribution of the article with the strict proviso that no changes or edits are made and the original work is properly cited (including links to both the formal publication through the relevant DOI and the license). See: https://creativecommons.org/licenses/by-nc-nd/4.0/.
References
- Roemer FW, Collins J, Kwoh CK, Hannon MJ, Neogi T, Felson DT, Hunter DJ, Lynch JA, Guermazi A. MRI-based screening for structural definition of eligibility in clinical DMOAD trials: Rapid OsteoArthritis MRI Eligibility Score (ROAMES). Osteoarthritis Cartilage 2020;28:71-81. [Crossref] [PubMed]
- Halilaj E, Le Y, Hicks JL, Hastie TJ, Delp SL. Modeling and predicting osteoarthritis progression: data from the osteoarthritis initiative. Osteoarthritis Cartilage 2018;26:1643-50. [Crossref] [PubMed]
- Widera P, Welsing PMJ, Ladel C, Loughlin J, Lafeber FPFJ, Petit Dop F, Larkin J, Weinans H, Mobasheri A, Bacardit J. Multi-classifier prediction of knee osteoarthritis progression from incomplete imbalanced longitudinal data. Sci Rep 2020;10:8427. [Crossref] [PubMed]
- Guan B, Liu F, Haj-Mirzaian A, Demehri S, Samsonov A, Neogi T, Guermazi A, Kijowski R. Deep learning risk assessment models for predicting progression of radiographic medial joint space loss over a 48-MONTH follow-up period. Osteoarthritis Cartilage 2020;28:428-37. [Crossref] [PubMed]
- Tiulpin A, Klein S, Bierma-Zeinstra SMA, Thevenot J, Rahtu E, Meurs JV, Oei EHG, Saarakkala S. Multimodal Machine Learning-based Knee Osteoarthritis Progression Prediction from Plain Radiographs and Clinical Data. Sci Rep 2019;9:20038. [Crossref] [PubMed]
- Collins JE, Losina E, Nevitt MC, Roemer FW, Guermazi A, Lynch JA, Katz JN, Kent Kwoh C, Kraus VB, Hunter DJ. Semiquantitative Imaging Biomarkers of Knee Osteoarthritis Progression: Data From the Foundation for the National Institutes of Health Osteoarthritis Biomarkers Consortium. Arthritis Rheumatol 2016;68:2422-31. [Crossref] [PubMed]
- Bonakdari H, Jamshidi A, Pelletier JP, Abram F, Tardif G, Martel-Pelletier J. A warning machine learning algorithm for early knee osteoarthritis structural progressor patient screening. Ther Adv Musculoskelet Dis 2021;13:1759720X21993254.
- Du Y, Almajalid R, Shan J, Zhang M. A Novel Method to Predict Knee Osteoarthritis Progression on MRI Using Machine Learning Methods. IEEE Trans Nanobioscience 2018;17:228-36. [Crossref] [PubMed]
- Schiratti JB, Dubois R, Herent P, Cahané D, Dachary J, Clozel T, Wainrib G, Keime-Guibert F, Lalande A, Pueyo M, Guillier R, Gabarroca C, Moingeon P. A deep learning method for predicting knee osteoarthritis radiographic progression from MRI. Arthritis Res Ther 2021;23:262. [Crossref] [PubMed]
- Hafezi-Nejad N, Guermazi A, Roemer FW, Hunter DJ, Dam EB, Zikria B, Kwoh CK, Demehri S. Prediction of medial tibiofemoral compartment joint space loss progression using volumetric cartilage measurements: Data from the FNIH OA biomarkers consortium. Eur Radiol 2017;27:464-73. [Crossref] [PubMed]
- Palmer JS, Jones LD, Monk AP, Nevitt M, Lynch J, Beard DJ, Javaid MK, Price AJ. Varus alignment of the proximal tibia is associated with structural progression in early to moderate varus osteoarthritis of the knee. Knee Surg Sports Traumatol Arthrosc 2020;28:3279-86. [Crossref] [PubMed]
- Sugiyama S, Itokazu M, Suzuki Y, Shimizu K. Procollagen II C propeptide level in the synovial fluid as a predictor of radiographic progression in early knee osteoarthritis. Ann Rheum Dis 2003;62:27-32. [Crossref] [PubMed]
- Kraus VB, Collins JE, Charles HC, Pieper CF, Whitley L, Losina E, Nevitt M, Hoffmann S, Roemer F, Guermazi A, Hunter DJ. Predictive Validity of Radiographic Trabecular Bone Texture in Knee Osteoarthritis: The Osteoarthritis Research Society International/Foundation for the National Institutes of Health Osteoarthritis Biomarkers Consortium. Arthritis Rheumatol 2018;70:80-7. [Crossref] [PubMed]
- Liu Y, Joseph GB, Foreman SC, Li X, Lane NE, Nevitt MC, McCulloch CE, Link TM. Determining a Threshold of Medial Meniscal Extrusion for Prediction of Knee Pain and Cartilage Damage Progression Over 4 Years: Data From the Osteoarthritis Initiative. AJR Am J Roentgenol 2021;216:1318-28. [Crossref] [PubMed]
- Loeser RF, Pathmasiri W, Sumner SJ, McRitchie S, Beavers D, Saxena P, Nicklas BJ, Jordan J, Guermazi A, Hunter DJ, Messier SP. Association of urinary metabolites with radiographic progression of knee osteoarthritis in overweight and obese adults: an exploratory study. Osteoarthritis Cartilage 2016;24:1479-86. [Crossref] [PubMed]
- MacKay JW, Kapoor G, Driban JB, Lo GH, McAlindon TE, Toms AP, McCaskie AW, Gilbert FJ. Association of subchondral bone texture on magnetic resonance imaging with radiographic knee osteoarthritis progression: data from the Osteoarthritis Initiative Bone Ancillary Study. Eur Radiol 2018;28:4687-95. [Crossref] [PubMed]
- Guan B, Liu F, Mizaian AH, Demehri S, Samsonov A, Guermazi A, Kijowski R. Deep learning approach to predict pain progression in knee osteoarthritis. Skeletal Radiol 2022;51:363-73. [Crossref] [PubMed]
- Ding C, Zhang Y, Hunter D. Use of imaging techniques to predict progression in osteoarthritis. Curr Opin Rheumatol 2013;25:127-35. [Crossref] [PubMed]
- Ding C, Cicuttini F, Jones G. How important is MRI for detecting early osteoarthritis? Nat Clin Pract Rheumatol 2008;4:4-5. [Crossref] [PubMed]
- Madan-Sharma R, Kloppenburg M, Kornaat PR, Botha-Scheepers SA, Le Graverand MP, Bloem JL, Watt I. Do MRI features at baseline predict radiographic joint space narrowing in the medial compartment of the osteoarthritic knee 2 years later? Skeletal Radiol 2008;37:805-11. [Crossref] [PubMed]
- Harkey MS, Davis JE, Price LL, Ward RJ, MacKay JW, Eaton CB, Lo GH, Barbe MF, Zhang M, Pang J, Stout AC, Lu B, McAlindon TE, Driban JB. Composite quantitative knee structure metrics predict the development of accelerated knee osteoarthritis: data from the osteoarthritis initiative. BMC Musculoskelet Disord 2020;21:299. [Crossref] [PubMed]
- Edwards MH, Parsons C, Bruyère O, Petit Dop F, Chapurlat R, Roemer FW, Guermazi A, Zaim S, Genant H, Reginster JY, Dennison EM, Cooper C. High Kellgren-Lawrence Grade and Bone Marrow Lesions Predict Worsening Rates of Radiographic Joint Space Narrowing; The SEKOIA Study. J Rheumatol 2016;43:657-65. [Crossref] [PubMed]
- Kawahara T, Sasho T, Ohnishi T, Haneishi H. Stage-specific meniscal features predict progression of osteoarthritis of the knee: a retrospective cohort study using data from the osteoarthritis initiative. BMC Musculoskelet Disord 2019;20:33. [Crossref] [PubMed]
- Dam EB, Loog M, Christiansen C, Byrjalsen I, Folkesson J, Nielsen M, Qazi AA, Pettersen PC, Garnero P, Karsdal MA. Identification of progressors in osteoarthritis by combining biochemical and MRI-based markers. Arthritis Res Ther 2009;11:R115. [Crossref] [PubMed]
- Zhong H, Miller DJ, Urish KL. T2 map signal variation predicts symptomatic osteoarthritis progression: data from the Osteoarthritis Initiative. Skeletal Radiol 2016;45:909-13. [Crossref] [PubMed]
- Urish KL, Keffalas MG, Durkin JR, Miller DJ, Chu CR, Mosher TJ. T2 texture index of cartilage can predict early symptomatic OA progression: data from the osteoarthritis initiative. Osteoarthritis Cartilage 2013;21:1550-7. [Crossref] [PubMed]
- Attur M, Krasnokutsky S, Zhou H, Samuels J, Chang G, Bencardino J, Rosenthal P, Rybak L, Huebner JL, Kraus VB, Abramson SB. The combination of an inflammatory peripheral blood gene expression and imaging biomarkers enhance prediction of radiographic progression in knee osteoarthritis. Arthritis Res Ther 2020;22:208. [Crossref] [PubMed]
- Liu F. SUSAN: segment unannotated image structure using adversarial network. Magn Reson Med 2019;81:3330-45. [PubMed]
- Liu F, Samsonov A, Chen L, Kijowski R, Feng L. SANTIS: Sampling-Augmented Neural neTwork with Incoherent Structure for MR image reconstruction. Magn Reson Med 2019;82:1890-904. [Crossref] [PubMed]
- Liu F, Feng L, Kijowski R. MANTIS: Model-Augmented Neural neTwork with Incoherent k-space Sampling for efficient MR parameter mapping. Magn Reson Med 2019;82:174-88. [Crossref] [PubMed]
- Morales Martinez A, Caliva F, Flament I, Liu F, Lee J, Cao P, Shah R, Majumdar S, Pedoia V. Learning osteoarthritis imaging biomarkers from bone surface spherical encoding. Magn Reson Med 2020;84:2190-203. [Crossref] [PubMed]
- Tolpadi AA, Lee JJ, Pedoia V, Majumdar S. Deep Learning Predicts Total Knee Replacement from Magnetic Resonance Images. Sci Rep 2020;10:6371. [Crossref] [PubMed]
- Lee JJ, Namiri NK, Astuto B, Link TM, Majumdar S, Pedoia V. Personalized Risk Model and Leveraging of Magnetic Resonance Imaging-Based Structural Phenotypes and Clinical Factors to Predict Incidence of Radiographic Osteoarthritis. Arthritis Care Res (Hoboken) 2023;75:501-8. [Crossref] [PubMed]
- Cheung JC, Tam AY, Chan LC, Chan PK, Wen C. Superiority of Multiple-Joint Space Width over Minimum-Joint Space Width Approach in the Machine Learning for Radiographic Severity and Knee Osteoarthritis Progression. Biology (Basel) 2021;10:1107. [Crossref] [PubMed]
- Eckstein F, Wirth W, Nevitt MC. Recent advances in osteoarthritis imaging--the osteoarthritis initiative. Nat Rev Rheumatol 2012;8:622-30. [Crossref] [PubMed]
- Panfilov E, Saarakkala S, Nieminen MT, Tiulpin A. Predicting Knee Osteoarthritis Progression from Structural MRI Using Deep Learning. 2022 IEEE 19th International Symposium on Biomedical Imaging (ISBI); 2022:1-5.
- Oo WM, Little C, Duong V, Hunter DJ. The Development of Disease-Modifying Therapies for Osteoarthritis (DMOADs): The Evidence to Date. Drug Des Devel Ther 2021;15:2921-45. [Crossref] [PubMed]
- Roos EM, Arden NK. Strategies for the prevention of knee osteoarthritis. Nat Rev Rheumatol 2016;12:92-101. [Crossref] [PubMed]
- Li ZC, Yan J, Zhang S, Liang C, Lv X, Zou Y, Zhang H, Liang D, Zhang Z, Chen Y. Glioma survival prediction from whole-brain MRI without tumor segmentation using deep attention network: a multicenter study. Eur Radiol 2022;32:5719-29. [Crossref] [PubMed]
- Kraus VB, Collins JE, Hargrove D, Losina E, Nevitt M, Katz JN, Wang SX, Sandell LJ, Hoffmann SC, Hunter DJ. Predictive validity of biochemical biomarkers in knee osteoarthritis: data from the FNIH OA Biomarkers Consortium. Ann Rheum Dis 2017;76:186-95. [Crossref] [PubMed]
- Hunter D, Nevitt M, Lynch J, Kraus VB, Katz JN, Collins JE, Bowes M, Guermazi A, Roemer FW, Losina E. Longitudinal validation of periarticular bone area and 3D shape as biomarkers for knee OA progression? Data from the FNIH OA Biomarkers Consortium. Ann Rheum Dis 2016;75:1607-14. [Crossref] [PubMed]
- Eckstein F, Collins JE, Nevitt MC, Lynch JA, Kraus VB, Katz JN, Losina E, Wirth W, Guermazi A, Roemer FW, Hunter DJ. Brief Report: Cartilage Thickness Change as an Imaging Biomarker of Knee Osteoarthritis Progression: Data From the Foundation for the National Institutes of Health Osteoarthritis Biomarkers Consortium. Arthritis Rheumatol 2015;67:3184-9. [Crossref] [PubMed]
- Wirth W, Duryea J, Hellio Le Graverand MP, John MR, Nevitt M, Buck RJ, Eckstein F. Direct comparison of fixed flexion, radiography and MRI in knee osteoarthritis: responsiveness data from the Osteoarthritis Initiative. Osteoarthritis Cartilage 2013;21:117-25. [Crossref] [PubMed]
- Guermazi A, Roemer FW, Haugen IK, Crema MD, Hayashi D. MRI-based semiquantitative scoring of joint pathology in osteoarthritis. Nat Rev Rheumatol 2013;9:236-51. [Crossref] [PubMed]
- Peterfy CG, Schneider E, Nevitt M. The osteoarthritis initiative: report on the design rationale for the magnetic resonance imaging protocol for the knee. Osteoarthritis Cartilage 2008;16:1433-41. [Crossref] [PubMed]
- Huang G, Liu Z, Van Der Maaten L, Weinberger KQ. editors. Densely connected convolutional networks. Proceedings of the IEEE conference on computer vision and pattern recognition; 2017.
- He K, Zhang X, Ren S, Sun J. editors. Deep residual learning for image recognition. Proceedings of the IEEE conference on computer vision and pattern recognition; 2016.
- Polat Ç, Karaman O, Karaman C, Korkmaz G, Balcı MC, Kelek SE. COVID-19 diagnosis from chest X-ray images using transfer learning: Enhanced performance by debiasing dataloader. J Xray Sci Technol 2021;29:19-36. [Crossref] [PubMed]
- Yan Y, Zhou H, Huang L, Cheng X, Kuang S. A Novel Two-Stage Refine Filtering Method for EEG-Based Motor Imagery Classification. Front Neurosci 2021;15:657540. [Crossref] [PubMed]
- Choi KS, You SH, Han Y, Ye JC, Jeong B, Choi SH. Improving the Reliability of Pharmacokinetic Parameters at Dynamic Contrast-enhanced MRI in Astrocytomas: A Deep Learning Approach. Radiology 2020;297:178-88. [Crossref] [PubMed]
- Song Y, Zhang YD, Yan X, Liu H, Zhou M, Hu B, Yang G. Computer-aided diagnosis of prostate cancer using a deep convolutional neural network from multiparametric MRI. J Magn Reson Imaging 2018;48:1570-7. [Crossref] [PubMed]
- Hung TNK, Vy VPT, Tri NM, Hoang LN, Tuan LV, Ho QT, Le NQK, Kang JH. Automatic Detection of Meniscus Tears Using Backbone Convolutional Neural Networks on Knee MRI. J Magn Reson Imaging 2023;57:740-9. [Crossref] [PubMed]
- Ding C, Jones G, Wluka AE, Cicuttini F. What can we learn about osteoarthritis by studying a healthy person against a person with early onset of disease? Curr Opin Rheumatol 2010;22:520-7. [Crossref] [PubMed]
- Eckstein F, Wirth W, Hunter DJ, Guermazi A, Kwoh CK, Nelson DR, Benichou O. Magnitude and regional distribution of cartilage loss associated with grades of joint space narrowing in radiographic osteoarthritis--data from the Osteoarthritis Initiative (OAI). Osteoarthritis Cartilage 2010;18:760-8. [Crossref] [PubMed]
- Houck DA, Kraeutler MJ, Belk JW, Frank RM, McCarty EC, Bravman JT. Do Focal Chondral Defects of the Knee Increase the Risk for Progression to Osteoarthritis? A Review of the Literature. Orthop J Sports Med 2018;6:2325967118801931. [Crossref] [PubMed]
- Teichtahl AJ, Cicuttini FM, Abram F, Wang Y, Pelletier JP, Dodin P, Martel-Pelletier J. Meniscal extrusion and bone marrow lesions are associated with incident and progressive knee osteoarthritis. Osteoarthritis Cartilage 2017;25:1076-83. [Crossref] [PubMed]
- Aso K, Shahtaheri SM, McWilliams DF, Walsh DA. Association of subchondral bone marrow lesion localization with weight-bearing pain in people with knee osteoarthritis: data from the Osteoarthritis Initiative. Arthritis Res Ther 2021;23:35. [Crossref] [PubMed]
- Holzer LA, Kraiger M, Talakic E, Fritz GA, Avian A, Hofmeister A, Leithner A, Holzer G. Microstructural analysis of subchondral bone in knee osteoarthritis. Osteoporos Int 2020;31:2037-45. [Crossref] [PubMed]
- Paduszyński W, Jeśkiewicz M, Uchański P, Gackowski S, Radkowski M, Demkow U. Hoffa's Fat Pad Abnormality in the Development of Knee Osteoarthritis. Adv Exp Med Biol 2018;1039:95-102. [Crossref] [PubMed]