Machine learning prediction model for the rupture status of middle cerebral artery aneurysm in patients with hypertension: a Chinese multicenter study
Introduction
Subarachnoid hemorrhage caused by ruptured aneurysm is a subtype of stroke. It has of high morbidity, high mortality, and a younger trend (1,2). Recently, with the development and popularization of noninvasive techniques such as computed tomography angiography (CTA) and magnetic resonance angiography (MRA), unruptured intracranial aneurysms are more likely to be detected. Unruptured intracranial cerebral aneurysms are most frequently found on the middle cerebral artery (MCA) (3,4). Approximately 80% of unruptured MCA aneurysms are located at the bifurcation of the MCA, surrounded by many perforating vessels and complicated hemodynamics (3). Previous studies have shown that most unruptured aneurysms may be stable throughout patient’s entire life (5,6). It is important to predict aneurysm rupture because the risks might be caused by prophylactic invasive treatment of unruptured aneurysms (7,8).
Hypertension is a common comorbidity in patients with unruptured intracranial aneurysms, and approximately half of unruptured intracranial aneurysms are comorbid with hypertension (9). A pooled analysis based on 6 prospective cohort studies showed a 1-point increase in the PHASES (Population, Hypertension, Age, Size, Earlier subarachnoid hemorrhage, and Site) risk score for unruptured aneurysms with hypertension (10). A recent Chinese Intracranial Aneurysm Research and Development Project study retrospectively analyzed the prospective data of 3,965 patients with saccular aneurysms from 20 medical centers and showed that 71.02% of hypertensive patients without regular blood pressure monitoring had ruptured intracranial aneurysms (11). Hypertension is thought to be closely related to the formation, development and rupture of aneurysms. The formation of a high hemodynamic pressure region resulting from hypertension is prone to increase the wall shear stress (WSS) of the bifurcation of the intracranial artery and induce destructive reconstruction of the arterial wall (12-14). Meanwhile, high wall tension of injured vasculature promotes the formation and development of aneurysms (15,16). In addition to the pure mechanical effects, the structural changes and dysfunctions of the arterial wall caused by hypertension, such as the imbalance of vasoactive substances and the expression of proinflammatory factors, play an important role in the occurrence and development of aneurysms and will destroy the normal structure of the arterial wall and hinder the repair of damaged blood vessels (17-19). However, to our knowledge, few studies have focused on the rupture of intracranial aneurysms in patients with hypertension, which leads to the lack of a prediction model for rupture risk. A stratified analysis of the rupture risk of intracranial aneurysms is necessary because of China’s huge population base. We previously reported a single-center study in which it was feasible to predict the rupture risk of MCA aneurysm in patients with hypertension based on a nomogram (20).
Recently, machine learning (ML) has been widely used and recognized as a promising tool in prediction. In theory, ML models have better flexibility and scalability than their traditional counterparts when dealing with high-dimensional data (21,22). In addition, ML can determine the hidden patterns of data and identify relevant variables (23). At present, ML has been widely used in the medical field, including in aneurysm rupture prediction. We performed a study to construct prediction models of aneurysm rupture using ML algorithms based on clinical and morphological features in MCA aneurysm patients with hypertension and externally validated the constructed models with multicenter datasets. We present this article in accordance with the TRIPOD reporting checklist (available at https://qims.amegroups.com/article/view/10.21037/qims-22-918/rc).
Methods
Patients and characteristics
The study was conducted in accordance with the Declaration of Helsinki (as revised in 2013) and was approved by the Medical Ethics Committees of the First Affiliated Hospital of Wenzhou Medical University, the Wenzhou Central Hospital, the Second Affiliated Hospital of Wenzhou Medical University, and the Zhejiang Hospital. As this was a retrospective study, the need for written informed consent was waived. From January 2009 to January 2021, 629 hospitalized patients from Hospital A with MCA aneurysm underwent CTA. The exclusion criteria were as follows: (I) patients with multiple, dissected, fusiform, and pseudoaneurysms, (II) aneurysms associated with arteriovenous malformations and Moyamoya disease, (III) poor quality of CTA images, and (IV) non-hypertensive patients. Finally, 277 MCA aneurysm patients with hypertension at Hospital A were screened as the training datasets for the study. The process of patient selection is shown in Figure 1. Clinical data were collected from the Electronic Medical Record System and included sex, age, smoking, and hypertension history. Hypertension was defined as a long-term history of hypertension with systolic blood pressures greater than 140 mmHg and/or diastolic blood pressures greater than 90 mmHg (24). Ruptured aneurysms were defined as those adjacent to the cisternal clots of subarachnoid hemorrhage and those that were not adjacent but were confirmed intraoperatively by digital subtraction angiography (DSA), while unruptured aneurysms were defined as those with neither subarachnoid hemorrhage nor positive symptoms (25).
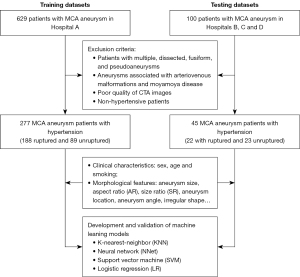
In addition, 45 patients from Hospital B (from May 2018 to June 2021), Hospital C (from January 2018 to May 2021), and Hospital D (from January 2019 to December 2019) were screened with the same criteria and were incorporated into one external testing datasets. All characteristics, such as sex, age, and smoking history, were collected through in-hospital electronic medical records. Each hospital in this study was a tertiary center with similar profiles and configurations.
Examination and reconstruction of CTA
All CTA images were obtained with the use of three computed tomography (CT) scanners, including a 320-detector row CT scanner (Aquilion ONE, Toshiba Medical Systems, Tochigi, Japan), a 64-channel multidetector CT scanner (LightSpeed VCT, General Electric Medical Systems, Milwaukee, WI, USA), and a 16-channel multidetector CT scanner (LightSpeed pro, General Electric Medical Systems, Milwaukee, WI, USA). The scanning parameters of CTA were reported in previous studies (26,27). The reconstructions of all CTA images were performed on a workstation (Version 4.6; General Electric Medical Systems, Milwaukee, Wisconsin, USA) to fully reveal the aneurysm and surrounding vasculature.
Morphological features of aneurysms
Aneurysm morphology features included aneurysm size, aneurysm neck, aneurysm height, perpendicular height, aspect ratio (AR), size ratio (SR), vessel size, aneurysm angle, vessel angle, flow angle, aneurysm location, aneurysm orientation, irregular shape, and daughter sac. Aneurysm location was classified according to whether it was at the main bifurcation of the MCA. Aneurysm orientation was divided into transverse orientation (anterior, posterior, and neutral) and coronal orientation (superior, inferior, and neutral). Vessel size referred to the mean cross-sectional diameter of all arteries related to the aneurysm. The specific definitions and measurements of the morphological features were consistent with the descriptions in previous studies and independently evaluated by an experienced neuroradiologist who was blinded to the rupture status of the aneurysm (26,28). The morphological parameters are described in Figure 2.
Prediction model construction
To predict rupture of an MCA aneurysm in patients with hypertension, we input all clinical and morphological features into k-nearest-neighbor (KNN), neural network (NNet), support vector machine (SVM) and logistic regression (LR) to construct the prediction models. The predictive importance of the features was evaluated and ranked in the KNN, NNet and SVM models. The training datasets were used to develop ML prediction models, and considering the possible overfitting effect from model training, the established models were 10-fold cross-validated. The performance of each model was evaluated using the area under the receiver operating characteristic (ROC) curve (AUC), sensitivity, specificity, and accuracy. The performance of the model was tested using training datasets, and the effectiveness was verified in independent external testing datasets. Model fitting was performed using the caret package (title: Classification and Regression Training; version 6.0-93; URL https://github.com/topepo/caret/) in R (version 3.6.3).
KNN, NNet and SVM are supervised ML algorithms. KNN classifies the input samples into the category of its nearest group based on the k-value, which is adjusted in the training datasets according to the distance computation for each unknown sample in the feature space (29). In this KNN model, the k parameter was tuned in the range of 3 to 20. NNet is a feed-forward NNet with a single hidden layer flowing left to right. The input sample information starts from the input layer, passes through the hidden layer, and is finally forwarded to the output layer (30). Default parameters were selected for NNet in this study, and the final values used for the model were size =1 and decay =0.1. SVM classifies data points by maximizing the distance between classes in a high-dimensional space (31). The SVM model was built using a radial basis function kernel, and the sigma hyperparameter was determined from the estimation based upon the 0.1 and 0.9 quantiles of the samples. For soft margins, the C parameter that achieved the best performance was in the range of 2−2 to 27. LR models the conditional probability using a logistic function, which is used to find the logistic distribution by measuring the relationship between the dependent and independent variables. A stepwise selection was applied using the likelihood ratio test with Akaike’s information criterion as the stopping rule.
Statistical analysis
Statistical analyses were conducted using IBM SPSS Statistics for Windows, version 25 (IBM Corp., Armonk, NY, USA). Continuous variables of training datasets, analyzed with Mann-Whitney U tests, are presented as median and interquartile range (IQR); categorical variables, analyzed with χ2 tests, are presented as frequencies. Multivariate LR analysis was performed for variables with P<0.1 in the univariate analysis. In addition, we performed a ROC curve analysis for AR, calculated the AUC value, and employed the highest Youden index to find cutoff values. Significance was set at a P value <0.05.
Results
Clinical and morphological characteristics
The clinical and morphological characteristics of the training datasets are shown in Table 1, and the baseline characteristics of the testing datasets are shown in Table S1. Of all the 277 MCA aneurysm patients with hypertension, 188 ruptured and 89 unruptured. In the univariate analysis, the patients with ruptured MCA aneurysms were younger than those with unruptured MCA aneurysms (56.0 versus 65.0 years, P<0.05). There were no significant differences in sex or smoking between the two groups (P>0.05). For morphological characteristics, the aneurysm size, AR, SR, and vessel angle were higher in the aneurysm ruptured group (P<0.05), while the aneurysm angle was smaller (P<0.05). In the ruptured group, irregular shape (63.3% versus 23.6%, P<0.05) and daughter sac (39.4% versus 15.7%, P<0.05) were more frequent. Aneurysm location and transverse orientation were also associated with aneurysm rupture (P<0.05).
Table 1
Characteristics | Ruptured (n=188) | Unruptured (n=89) | P value |
---|---|---|---|
Clinical variables | |||
Sex (male/female) | 80/108 | 48/41 | 0.076 |
Age (years) | 56.0 (55.9, 58.9) | 65.0 (62.2, 66.3) | <0.001 |
Smoking (yes) | 50 (26.6) | 27 (30.3) | 0.516 |
Morphological characteristics | |||
Aneurysm size (mm) | 6.5 (6.6, 7.4) | 5.0 (5.2, 6.8) | <0.001 |
Aneurysm neck (mm) | 3.7 (3.9, 4.3) | 3.9 (4.0, 4.9) | 0.576 |
Vessel size (mm) | 2.3 (2.2, 2.4) | 2.5 (2.3, 2.5) | 0.056 |
AR | 1.0 (1.1, 1.2) | 0.6 (0.7, 0.9) | <0.001 |
SR | 2.1 (2.3, 2.7) | 1.3 (1.4, 2.1) | <0.001 |
Aneurysm angle (°) | 64.1 (60.2, 65.7) | 73.2 (67.7, 74.6) | 0.001 |
Vessel angle (°) | 61.1 (51.9, 59.1) | 53.2 (42.7, 53.9) | 0.035 |
Flow angle (°) | 142.9 (133.9, 142.0) | 137.7 (127.2, 139.3) | 0.167 |
Irregular shape | 119 (63.3) | 21 (23.6) | <0.001 |
Daughter sac | 74 (39.4) | 14 (15.7) | <0.001 |
Aneurysm location | <0.001 | ||
Main MCA bifurcation | 137 (72.9) | 43 (48.3) | |
Non-main MCA bifurcation | 51 (27.1) | 46 (51.7) | |
Transverse orientation | 0.002 | ||
Anterior | 104 (55.3) | 30 (33.7) | |
Posterior | 31 (16.5) | 18 (20.2) | |
Neutral | 53 (28.2) | 41 (46.1) | |
Coronal orientation | 0.247 | ||
Superior | 69 (36.7) | 26 (29.2) | |
Inferior | 52 (27.7) | 33 (37.1) | |
Neutral | 67 (35.6) | 30 (33.7) |
Data are presented ad median (interquartile range) or n (%). The P value level chosen to determine significance was established to be 0.05. AR, aspect ratio; MCA, middle cerebral artery; SR, size ratio.
The results of the multivariate LR analysis are shown in Table 2. For hypertensive patients, age [odds ratio (OR) =0.939, 95% confidence interval (CI): 0.909–0.969, P<0.001], aneurysm size (OR =0.867, 95% CI: 0.769–0.976, P=0.018), AR (OR =4.822, 95% CI: 2.029–11.459, P<0.001), aneurysm angle (OR =0.975, 95% CI: 0.957–0.995, P=0.013), irregular shape (OR =4.034, 95% CI: 1.951–8.342, P<0.001) and aneurysm location (OR =2.695, 95% CI: 1.422–5.105, P=0.002) were significantly associated with MCA aneurysm rupture.
Table 2
Variables | Univariate analysis | Multivariate analysis | |||||
---|---|---|---|---|---|---|---|
Odds ratio | 95% CI | P value | Odds ratio | 95% CI | P value | ||
Sex | 1.580 | 0.952–2.625 | 0.077 | – | – | – | |
Age | 0.935 | 0.911–0.961 | <0.001 | 0.939 | 0.909–0.969 | <0.001 | |
Aneurysm size (mm) | 1.103 | 1.013–1.202 | 0.024 | 0.867 | 0.769–0.976 | 0.018 | |
Vessel size (mm) | 0.589 | 0.351–0.987 | 0.045 | – | – | – | |
AR | 4.741 | 2.311–9.726 | <0.001 | 4.822 | 2.029–11.459 | <0.001 | |
SR | 1.699 | 1.311–2.202 | <0.001 | – | – | – | |
Aneurysm angle | 0.975 | 0.960–0.989 | 0.001 | 0.975 | 0.957–0.995 | 0.013 | |
Vessel angle | 1.011 | 1.001–1.021 | 0.032 | – | – | – | |
Irregular shape | 5.585 | 3.151–9.896 | <0.001 | 4.034 | 1.951–8.342 | <0.001 | |
Daughter sac | 3.477 | 1.831–6.603 | <0.001 | – | – | – | |
Aneurysm location | 2.874 | 1.699–4.861 | <0.001 | 2.695 | 1.422–5.105 | 0.002 | |
Transverse orientation | 0.003 | ||||||
Anterior | – | – | – | – | – | – | |
Posterior | 2.682 | 1.508–4.768 | 0.001 | – | – | – | |
Neutral | 1.332 | 0.655–2.709 | 0.428 | – | – | – |
The P value level chosen to determine significance was established to be 0.05. AR, aspect ratio; CI, confidence interval; MCA, middle cerebral artery; SR, size rat.
Performance of the ML models
The performance in prediction of each model is shown in Figure 3. The AUC values of the KNN model in the training and testing datasets were 0.83 (95% CI: 0.78–0.88) and 0.74 (95% CI: 0.59–0.89), respectively. The NNet model achieved an AUC value of 0.87 (95% CI: 0.82–0.92) in the training datasets, and an AUC value of 0.82 (95% CI: 0.69–0.94) in the testing datasets. The SVM model obtained an AUC value of 0.91 (95% CI: 0.88–0.95) in the training datasets, and of 0.73 (95% CI: 0.58–0.88) in the testing datasets. The LR model achieved AUC values of 0.83 (95% CI: 0.77–0.88) and 0.76 (95% CI: 0.61–0.90) in the training and testing datasets, respectively.
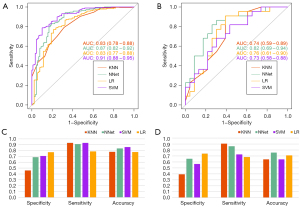
Variable importance of the ML models
All clinical and morphological characteristics were introduced into the KNN, NNet and SVM models, and the importance ranks of all features in the different models are shown in Figure 4. AR was ranked as the dominant predictor that contributed to the prediction of the KNN and SVM models, apart from NNet. In addition, AR also showed the highest OR in the multivariate LR analysis.
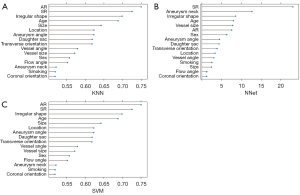
Further analysis with the ROC curve showed that the AUC of AR was 0.75 (95% CI: 0.69–0.82) in the training datasets, and the cutoff value was 0.80, with a sensitivity of 0.77, a specificity of 0.65, and an accuracy of 0.70. Meanwhile, in the testing datasets, the AUC of AR was 0.77 (95% CI: 0.63–0.91), with a sensitivity of 0.96, a specificity of 0.52, and an accuracy of 0.64.
Discussion
In this Chinese multicenter study, we developed and validated the ML prediction models based on clinical and CTA morphological features for the rupture status of MCA aneurysms in patients with hypertension, and we found that the ML prediction models showed reasonable performances. In addition, the results showed that AR was the leading predictor for rupture.
In recent years, many studies have constructed ML prediction models of intracranial aneurysm rupture risk based on parameters such as aneurysm morphology, hemodynamics and radiomics, with good prediction performance. Chen et al. combined clinical, morphological, and hemodynamic characteristics to construct random forest, multilayer perceptron, and SVM models to predict aneurysm rupture with AUCs of 0.851–0.871 (32). Liu et al. applied radiomics-extracted morphological features to construct general linear, ridge regression, and lasso regression models to predict the stability of aneurysms, and reached AUCs of 0.732–0.745 (33). To our knowledge, few studies have reported a prediction ML model of MCA aneurysm rupture risk: one study used morphological features to construct a generalized linear model to predict the rupture risk of MCA aneurysms with an AUC of 0.77 (27); the other study developed their MCA aneurysm rupture prediction model based on radiomics, clinical and morphological features, with the use of the SVM method and acquired an AUC of 0.856 (34). In this study, we focused on the rupture risk assessment of MCA aneurysms comorbid with hypertension. The performance of ML models is largely dependent on the datasets (35,36), and ML models based on different features perform differently in predicting aneurysm rupture. For the operability and convenience of clinical practice and promotion, clinical and aneurysm morphological features that are relatively easily available in clinical work were selected for model construction in this study. Similar to the ML models developed in previous studies, our ML models also have good prediction performance based on clinical and morphological characteristics. The results demonstrated that the achieved AUCs of our ML models ranged from 0.83–0.91 in the training datasets and 0.73–0.82 in the testing datasets.
AR is defined as the ratio of the perpendicular height to the neck size, and AR is closely related to aneurysm rupture. AR, as previously demonstrated, was positively associated with aneurysm rupture risk (37,38). Our study also demonstrated that AR was the strongest predictor in patients with MCA aneurysm complicated by hypertension. The AR of the ruptured aneurysms was significantly higher than that of the unruptured aneurysms (1.0 versus 0.6, P<0.01). Although many studies have proposed the importance of AR for aneurysm rupture, the cutoff value of AR in each study was not consistent with different thresholds that varied between 1.1 and 1.6 (39-41). We found that the AUC and accuracy were 0.75 and 0.70, respectively, for an AR cutoff value of 0.8 in MCA aneurysm patients with hypertension. The threshold of AR in this study was significantly lower than that in previous studies, which may be related to the presence of hypertension as a comorbidity in our study subjects.
Previous studies have demonstrated that hypertension is an independent risk factor for intracranial aneurysm rupture (42,43). High blood pressure can lead to a complex intravascular hemodynamic environment, especially at intracranial vascular bifurcations (13). Hypertension can induce a long-term low WSS environment in intra-aneurysms, which initiates endothelial dysfunction, triggers the proinflammatory signaling pathways and activates atherosclerosis, causing low resistibility of aneurysm walls and increasing the rupture risk of aneurysms (44-47). It has been proposed that the WSS of the intra-aneurysm cavity decreases significantly as AR exceeds the upper limit of a certain interval, which leads to a high risk of aneurysm rupture (40,48,49). Sun et al. conducted fluid-structure interaction analyses of intracranial aneurysms and found that the maximum wall stresses increased by 30–40% as the blood pressure increased from 120/80 to 160/100 mmHg, and the increase was higher in cases of aneurysms with large AR (45). In addition, they proposed that the decrease in the time-averaged WSS of the aneurysm models with high blood pressure would have a long-term impact on intracranial aneurysms, which would increase the risk of rupture (45). Therefore, we speculate that AR is a morphological feature reflecting the relationship between hypertension and the rupture of MCA aneurysms. However, this needs to be confirmed by further research.
This study has some limitations. First, a large multicenter study is needed to validate our study, as larger dimensional datasets could improve the quality of ML models. Second, we were unable to monitor the development of unruptured aneurysms or provide evidence of causality due to the nature of retrospective and cross-sectional analyses. Third, we were unable to determine whether daily blood pressure control in hypertensive patients would have an impact on the results of our study. In addition, the ML algorithms used in this study were common, and the more recent algorithms were not used to build the prediction models in this study. Finally, hemodynamic features were not included in this study.
Conclusions
The ML prediction model based on clinical and CTA morphological features showed reasonable performance for predicting the rupture status of MCA aneurysms in patients with hypertension. This study provides potential guidance for the stratified management of unruptured intracranial aneurysm patients with hypertension. Furthermore, AR was demonstrated as the leading predictor for the rupture of MCA aneurysm with hypertension.
Acknowledgments
We would like to thank AME Publishing Company for English language editing.
Funding: This work was supported by the Wenzhou Major Program of Science and Technology Innovation (No. ZY 2020012); the Health Foundation for Creative Talents in Zhejiang Province, China (No. 2016); the Project Foundation for the College Young and Middle-aged Academic Leader of Zhejiang Province, China (No. 2017); and the Science and Technology Planning Projects of Wenzhou (Nos. Y2020165 and Y2020164).
Footnote
Reporting Checklist: The authors have completed the TRIPOD reporting checklist. Available at https://qims.amegroups.com/article/view/10.21037/qims-22-918/rc
Conflicts of Interest: All authors have completed the ICMJE uniform disclosure form (available at https://qims.amegroups.com/article/view/10.21037/qims-22-918/coif). The authors have no conflicts of interest to declare.
Ethical Statement: The authors are accountable for all aspects of the work in ensuring that questions related to the accuracy or integrity of any part of the work are appropriately investigated and resolved. The study was conducted in accordance with the Declaration of Helsinki (as revised in 2013), and was approved by the Medical Ethics Committees of the First Affiliated Hospital of Wenzhou Medical University, the Wenzhou Central Hospital, the Second Affiliated Hospital of Wenzhou Medical University, and the Zhejiang Hospital. As this was a retrospective study, the need for written informed consent was waived.
Open Access Statement: This is an Open Access article distributed in accordance with the Creative Commons Attribution-NonCommercial-NoDerivs 4.0 International License (CC BY-NC-ND 4.0), which permits the non-commercial replication and distribution of the article with the strict proviso that no changes or edits are made and the original work is properly cited (including links to both the formal publication through the relevant DOI and the license). See: https://creativecommons.org/licenses/by-nc-nd/4.0/.
References
- Tawk RG, Hasan TF, D'Souza CE, Peel JB, Freeman WD. Diagnosis and Treatment of Unruptured Intracranial Aneurysms and Aneurysmal Subarachnoid Hemorrhage. Mayo Clin Proc 2021;96:1970-2000. [Crossref] [PubMed]
- Vlak MH, Algra A, Brandenburg R, Rinkel GJ. Prevalence of unruptured intracranial aneurysms, with emphasis on sex, age, comorbidity, country, and time period: a systematic review and meta-analysis. Lancet Neurol 2011;10:626-36. [Crossref] [PubMed]
- Huttunen T. von und zu Fraunberg M, Frösen J, Lehecka M, Tromp G, Helin K, Koivisto T, Rinne J, Ronkainen A, Hernesniemi J, Jääskeläinen JE. Saccular intracranial aneurysm disease: distribution of site, size, and age suggests different etiologies for aneurysm formation and rupture in 316 familial and 1454 sporadic eastern Finnish patients. Neurosurgery 2010;66:631-8; discussion 638. [Crossref] [PubMed]
- Etminan N, Rinkel GJ. Unruptured intracranial aneurysms: development, rupture and preventive management. Nat Rev Neurol 2016;12:699-713. [Crossref] [PubMed]
- Juvela S, Porras M, Poussa K. Natural history of unruptured intracranial aneurysms: probability and risk factors for aneurysm rupture. Neurosurg Focus 2000;8:Preview 1.
- Juvela S, Poussa K, Porras M. Factors affecting formation and growth of intracranial aneurysms: a long-term follow-up study. Stroke 2001;32:485-91. [Crossref] [PubMed]
- Naggara ON, White PM, Guilbert F, Roy D, Weill A, Raymond J. Endovascular treatment of intracranial unruptured aneurysms: systematic review and meta-analysis of the literature on safety and efficacy. Radiology 2010;256:887-97. [Crossref] [PubMed]
- Darsaut TE, Findlay JM, Magro E, Kotowski M, Roy D, Weill A, et al. Surgical clipping or endovascular coiling for unruptured intracranial aneurysms: a pragmatic randomised trial. J Neurol Neurosurg Psychiatry 2017;88:663-8. [Crossref] [PubMed]
- Tominari S, Morita A, Ishibashi T, Yamazaki T, Takao H, Murayama Y, Sonobe M, Yonekura M, Saito N, Shiokawa Y, Date I, Tominaga T, Nozaki K, Houkin K, Miyamoto S, Kirino T, Hashi K, Nakayama T. Prediction model for 3-year rupture risk of unruptured cerebral aneurysms in Japanese patients. Ann Neurol 2015;77:1050-9. [Crossref] [PubMed]
- Greving JP, Wermer MJ, Brown RD Jr, Morita A, Juvela S, Yonekura M, Ishibashi T, Torner JC, Nakayama T, Rinkel GJ, Algra A. Development of the PHASES score for prediction of risk of rupture of intracranial aneurysms: a pooled analysis of six prospective cohort studies. Lancet Neurol 2014;13:59-66. [Crossref] [PubMed]
- Zhong P, Lu Z, Li T, Lan Q, Liu J, Wang Z, Chen S, Huang Q. Association Between Regular Blood Pressure Monitoring and the Risk of Intracranial Aneurysm Rupture: a Multicenter Retrospective Study with Propensity Score Matching. Transl Stroke Res 2022;13:983-94. [Crossref] [PubMed]
- Sforza DM, Putman CM, Cebral JR. Hemodynamics of Cerebral Aneurysms. Annu Rev Fluid Mech 2009;41:91-107. [Crossref] [PubMed]
- Nakatani H, Hashimoto N, Kang Y, Yamazoe N, Kikuchi H, Yamaguchi S, Niimi H. Cerebral blood flow patterns at major vessel bifurcations and aneurysms in rats. J Neurosurg 1991;74:258-62. [Crossref] [PubMed]
- Jamous MA, Nagahiro S, Kitazato KT, Satoh K, Satomi J. Vascular corrosion casts mirroring early morphological changes that lead to the formation of saccular cerebral aneurysm: an experimental study in rats. J Neurosurg 2005;102:532-5. [Crossref] [PubMed]
- Frösen J, Cebral J, Robertson AM, Aoki T. Flow-induced, inflammation-mediated arterial wall remodeling in the formation and progression of intracranial aneurysms. Neurosurg Focus 2019;47:E21. [Crossref] [PubMed]
- Sehgel NL, Sun Z, Hong Z, Hunter WC, Hill MA, Vatner DE, Vatner SF, Meininger GA. Augmented vascular smooth muscle cell stiffness and adhesion when hypertension is superimposed on aging. Hypertension 2015;65:370-7. [Crossref] [PubMed]
- Guzik TJ, Touyz RM. Oxidative Stress, Inflammation, and Vascular Aging in Hypertension. Hypertension 2017;70:660-7. [Crossref] [PubMed]
- Tada Y, Wada K, Shimada K, Makino H, Liang EI, Murakami S, Kudo M, Kitazato KT, Nagahiro S, Hashimoto T. Roles of hypertension in the rupture of intracranial aneurysms. Stroke 2014;45:579-86. [Crossref] [PubMed]
- Sprague AH, Khalil RA. Inflammatory cytokines in vascular dysfunction and vascular disease. Biochem Pharmacol 2009;78:539-52. [Crossref] [PubMed]
- Su N, Zhou J, Lin B, Zhu D, Yang Y, Chen Y. Prediction of rupture risk of middle cerebral artery aneurysms in hypertensive patients based on nomogram, Journal of Wenzhou Medical University. Journal of Wenzhou Medical University 2022;52:638-44.
- Ngiam KY, Khor IW. Big data and machine learning algorithms for health-care delivery. Lancet Oncol 2019;20:e262-73. [Crossref] [PubMed]
- Deo RC. Machine Learning in Medicine. Circulation 2015;132:1920-30. [Crossref] [PubMed]
- Krittanawong C, Zhang H, Wang Z, Aydar M, Kitai T. Artificial Intelligence in Precision Cardiovascular Medicine. J Am Coll Cardiol 2017;69:2657-64. [Crossref] [PubMed]
- Unger T, Borghi C, Charchar F, Khan NA, Poulter NR, Prabhakaran D, Ramirez A, Schlaich M, Stergiou GS, Tomaszewski M, Wainford RD, Williams B, Schutte AE. 2020 International Society of Hypertension Global Hypertension Practice Guidelines. Hypertension 2020;75:1334-57. [Crossref] [PubMed]
- Shi Z, Chen GZ, Mao L, Li XL, Zhou CS, Xia S, Zhang YX, Zhang B, Hu B, Lu GM, Zhang LJ. Machine Learning-Based Prediction of Small Intracranial Aneurysm Rupture Status Using CTA-Derived Hemodynamics: A Multicenter Study. AJNR Am J Neuroradiol 2021;42:648-54. [Crossref] [PubMed]
- Chen Y, Lin B, Zhou J, Chen L, Yang Y, Zhao B. Morphological predictors of middle cerebral artery bifurcation aneurysm rupture. Clin Neurol Neurosurg 2020;192:105708. [Crossref] [PubMed]
- Liu J, Chen Y, Zhu D, Li Q, Chen Z, Zhou J, Lin B, Yang Y, Jia X. A nomogram to predict rupture risk of middle cerebral artery aneurysm. Neurol Sci 2021;42:5289-96. [Crossref] [PubMed]
- Can A, Ho AL, Dammers R, Dirven CM, Du R. Morphological parameters associated with middle cerebral artery aneurysms. Neurosurgery 2015;76:721-6; discussion 726-7. [Crossref] [PubMed]
- Cover T, Hart P. Nearest neighbor pattern classification. IEEE Trans Inform Theory 1967;13:21-7. [Crossref]
- LeCun Y, Bengio Y, Hinton G. Deep learning. Nature 2015;521:436-44. [Crossref] [PubMed]
- Orrù G, Pettersson-Yeo W, Marquand AF, Sartori G, Mechelli A. Using Support Vector Machine to identify imaging biomarkers of neurological and psychiatric disease: a critical review. Neurosci Biobehav Rev 2012;36:1140-52. [Crossref] [PubMed]
- Chen G, Lu M, Shi Z, Xia S, Ren Y, Liu Z, Liu X, Li Z, Mao L, Li XL, Zhang B, Zhang LJ, Lu GM. Development and validation of machine learning prediction model based on computed tomography angiography-derived hemodynamics for rupture status of intracranial aneurysms: a Chinese multicenter study. Eur Radiol 2020;30:5170-82. [Crossref] [PubMed]
- Liu Q, Jiang P, Jiang Y, Ge H, Li S, Jin H, Li Y. Prediction of Aneurysm Stability Using a Machine Learning Model Based on PyRadiomics-Derived Morphological Features. Stroke 2019;50:2314-21. [Crossref] [PubMed]
- Zhu D, Chen Y, Zheng K, Chen C, Li Q, Zhou J, Jia X, Xia N, Wang H, Lin B, Ni Y, Pang P, Yang Y. Classifying Ruptured Middle Cerebral Artery Aneurysms With a Machine Learning Based, Radiomics-Morphological Model: A Multicentral Study. Front Neurosci 2021;15:721268. [Crossref] [PubMed]
- Joo YB, Baek IW, Park YJ, Park KS, Kim KJ. Machine learning-based prediction of radiographic progression in patients with axial spondyloarthritis. Clin Rheumatol 2020;39:983-91. [Crossref] [PubMed]
- van der Ploeg T, Austin PC, Steyerberg EW. Modern modelling techniques are data hungry: a simulation study for predicting dichotomous endpoints. BMC Med Res Methodol 2014;14:137. [Crossref] [PubMed]
- Duan Z, Li Y, Guan S, Ma C, Han Y, Ren X, Wei L, Li W, Lou J, Yang Z. Morphological parameters and anatomical locations associated with rupture status of small intracranial aneurysms. Sci Rep 2018;8:6440. [Crossref] [PubMed]
- Wang GX, Li W, Lei S, Ge XD, Yin JB, Zhang D. Relationships between aneurysmal wall enhancement and conventional risk factors in patients with intracranial aneurysm: A high-resolution MRI study. J Neuroradiol 2019;46:25-8. [Crossref] [PubMed]
- Jing L, Fan J, Wang Y, Li H, Wang S, Yang X, Zhang Y. Morphologic and Hemodynamic Analysis in the Patients with Multiple Intracranial Aneurysms: Ruptured versus Unruptured. PLoS One 2015;10:e0132494. [Crossref] [PubMed]
- Ujiie H, Tachibana H, Hiramatsu O, Hazel AL, Matsumoto T, Ogasawara Y, Nakajima H, Hori T, Takakura K, Kajiya F. Effects of size and shape (aspect ratio) on the hemodynamics of saccular aneurysms: a possible index for surgical treatment of intracranial aneurysms. Neurosurgery 1999;45:119-29; discussion 129-30. [PubMed]
- Lee UY, Kwak HS. Analysis of Morphological-Hemodynamic Risk Factors for Aneurysm Rupture Including a Newly Introduced Total Volume Ratio. J Pers Med 2021; [Crossref] [PubMed]
- Taylor CL, Yuan Z, Selman WR, Ratcheson RA, Rimm AA. Cerebral arterial aneurysm formation and rupture in 20,767 elderly patients: hypertension and other risk factors. J Neurosurg 1995;83:812-9. [Crossref] [PubMed]
- Nahed BV, DiLuna ML, Morgan T, Ocal E, Hawkins AA, Ozduman K, Kahle KT, Chamberlain A, Amar AP, Gunel M. Hypertension, age, and location predict rupture of small intracranial aneurysms. Neurosurgery 2005;57:676-83; discussion 676-83. [Crossref] [PubMed]
- Sho E, Nanjo H, Sho M, Kobayashi M, Komatsu M, Kawamura K, Xu C, Zarins CK, Masuda H. Arterial enlargement, tortuosity, and intimal thickening in response to sequential exposure to high and low wall shear stress. J Vasc Surg 2004;39:601-12. [Crossref] [PubMed]
- Sun HT, Sze KY, Tang AYS, Tsang ACO, Yu ACH, Chow KW. Effects of aspect ratio, wall thickness and hypertension in the patient-specific computational modeling of cerebral aneurysms using fluid-structure interaction analysis. Engineering Applications of Computational Fluid Mechanics 2019;13:229-44. [Crossref]
- Bertani F, Di Francesco D, Corrado MD, Talmon M, Fresu LG, Boccafoschi F. Paracrine Shear-Stress-Dependent Signaling from Endothelial Cells Affects Downstream Endothelial Function and Inflammation. Int J Mol Sci 2021; [Crossref] [PubMed]
- Nixon AM, Gunel M, Sumpio BE. The critical role of hemodynamics in the development of cerebral vascular disease. J Neurosurg 2010;112:1240-53. [Crossref] [PubMed]
- Yin JH, Su SX, Zhang X, Bi YM, Duan CZ, Huang WM, Wang XL. U-Shaped Association of Aspect Ratio and Single Intracranial Aneurysm Rupture in Chinese Patients: A Cross-Sectional Study. Front Neurol 2021;12:731129. [Crossref] [PubMed]
- Yamaguchi R, Ujiie H, Haida S, Nakazawa N, Hori T. Velocity profile and wall shear stress of saccular aneurysms at the anterior communicating artery. Heart Vessels 2008;23:60-6. [Crossref] [PubMed]