T2*-corrected Q-Dixon and reduced-FOV diffusion kurtosis imaging (DKI) parameters: correlation with QCT-derived bone mineral density (BMD) and ability to identify abnormal BMD and osteoporosis in postmenopausal women
Introduction
Osteoporosis is a systemic metabolic disease characterized by reduced bone mass and degradation of bone microarchitecture, especially in postmenopausal women (1). Osteoporosis dramatically increases the risk of fragility fractures, and its complications seriously reduce the quality of life and can even lead to death (2). Therefore, improvements in the success of early screening for osteoporosis and the effective monitoring of its progression are undoubtedly crucial ways to prevent fragility fractures and their complications.
Measurements of bone mineral density (BMD) via dual-energy X-ray absorptiometry (DXA) or quantitative computed tomography (QCT) represent an essential reference standard for osteoporosis management (3,4). BMD values assessed by using DXA from a two-dimensional perspective have been reported to be susceptible to overlapping objects in the direction of X-rays (5). QCT avoids these interferences through three-dimensional imaging and is a reliable method for evaluating osteoporosis (6). However, the high cost and the effects of ionizing radiation limit the routine clinical practice of QCT (7). Furthermore, when exploring osteoporosis, changes in fat, water, microvasculature, and iron overload in the bone marrow microenvironment are closely associated with BMD, which cannot be assessed by using QCT methods (8).
The development of advanced magnetic resonance imaging (MRI) techniques offers the possibility to quantify the changes in the complex components of bone marrow. Due to the homology of osteoblasts and adipocytes, quantitative assessment targeting bone marrow adiposity has become one of the critical targets for academic research (9,10). The 6-echo water-lipid separation Dixon technique based on T2* correction has been reported to achieve more accuracy and flexibility than the conventional dual-echo Dixon technique for the accurate assessment of bone marrow adiposity (11) and to be in better agreement with the gold standard magnetic resonance spectroscopy technique (12,13). However, it should be noted that the Dixon technique only satisfies the quantification of bone marrow fat and does not allow for the assessment of complex alterations of vascularization and mineralization in the bone marrow microenvironment. In addition, it has not been reported as to whether there is a correlation between bone marrow fat distribution and heterogeneity of bone marrow structure, which is crucial to understanding the pathophysiological changes of the osteoporotic process.
The T2* value represents the spin relaxation time of adjacent protons in the tissue and reflects the anisotropy of the local magnetic field distribution (14). Changes in the microstructure of bone trabeculae and fat filling may lead to changes in magnetic field inhomogeneity, thus triggering differences in the reversible transverse relaxation time T2 due to phase deviations. Therefore, it is reasonable to assume that T2* values may identify osteoporosis through magnetic field differences triggered by changes in vertebral bone marrow and trabeculae. In a previous study by our team, we demonstrated that T2* values are valuable in identifying subjects with normal and abnormal bone masses (8). However, there was no significant difference in T2* values between osteopenia and osteoporosis subjects. Moreover, the diagnostic efficacy of T2* values in discriminating between osteopenia and osteoporosis was also unsatisfactory.
Diffusion-weighted imaging (DWI) can provide insight into changes in cell density, anisotropy, and perfusion due to pathophysiological changes in the bone marrow caused by osteoporosis (15). Although several diffusion techniques have been reported in bone marrow-related diseases, selections of pulse sequences and signal modeling remain essential considerations for optimizing diffusion techniques in bone marrow imaging (16). Recent studies have reported that parallel imaging and reduced-field-of-view (reduced-FOV) imaging through the use of external volume suppression pulses can enable single spin‒echo planar imaging (ssEPI) techniques to acquire higher-quality DWI images (17,18). In addition, conventional DWI involves isotropic imaging and does not truly reflect the true diffusive motion of water molecules in anisotropic tissues such as bone (19). Diffusion kurtosis imaging (DKI) is based on the non-Gaussian motion pattern of water molecules. By introducing the concept of kurtosis to quantify the extent to which the real water molecule diffusion shift deviates from the Gaussian distribution, it can more realistically reflect the degree of diffusion limitations and inhomogeneity of water molecules (20). The combination of small-field diffusion and DKI techniques makes it possible to noninvasively and accurately quantify the complex compositional changes and heterogeneity in the osteoporotic microenvironment.
Therefore, this study aimed to correlate Q-Dixon and DKI parameters with QCT-derived BMD values. On this basis, we strived to investigate the discriminatory capacity of Q-Dixon and DKI for normal bone density, osteopenia, and osteoporosis in postmenopausal women. We hypothesized that quantitative MRI detects alterations in structural bone marrow that may serve as a potential new imaging biomarker for abnormal BMD. We present this article in accordance with the STARD reporting checklist (available at https://qims.amegroups.com/article/view/10.21037/qims-22-1247/rc).
Methods
This prospective study was conducted in accordance with the Declaration of Helsinki (as revised in 2013). The study was approved by the Health Sciences Institutional Review Board (HIRB) of Huashan Hospital, Fudan University (No. KY2023-053). All of the subjects received a clear explanation of the study’s purpose, methods, potential risks, and benefits. Before the experimental procedures were initiated, written informed consent was obtained from all of the participants.
Study population
The individuals who were eligible for inclusion were postmenopausal women (over 50-year-old) with suspected osteoporosis based on low back pain. This mono-center study was conducted in tertiary care centers in China. All of the patients were recruited by using the consecutive sampling method. From March 2020 to February 2022, we recruited 200 subjects from the population who were undergoing health screening in our hospital. All of the subjects underwent QCT (µCT 780 64-layer scanner, United Imaging, Shanghai, China) and 3.0 T MRI (MAGNETOM Prisma MRI scanner; Siemens Healthcare, Erlangen, Germany) examinations. The inclusion criteria included postmenopausal women over 50 years of age. The exclusion criteria included spinal tumors, histories of spinal trauma and surgery, spinal dysplasia, spinal infectious diseases, previous hormone therapy, hematologic disorders, and intermediate-to-advanced malignancies. We investigated three possible increases in the risk of osteoporosis in postmenopausal women, including physical activity status, smoking, and alcohol consumption. The International Physical Activity Questionnaire—Short Form was used to assess the physical activity status of all of the subjects. Based on their physical activity level, patients were divided into sedentary, minimally active, and very active groups. Finally, 155 female subjects were enrolled in this study.
Sample size
As this is the first application of the DKI technique for osteoporosis assessment, an area under the curve (AUC) of no less than 0.7 was desired for this study. Power Analysis and Sample Size (PASS, 21.0.3, NCSS, USA) software was used to estimate the minimum sample size required for this study. To obtain more than 90% of the target statistical power, calculations were performed after setting α to 0.05 and selecting an AUC of 0.7. The results of the calculations showed that the actual statistical power was 90.42% for a minimum sample size of no less than 41 individuals per group.
QCT examination
BMD of the lumbar spine was obtained by using a United Imaging µCT 780 64-layer scanner (United Imaging). Routine calibration work was performed by using Mindways calibration body models (Mindways Software Inc., Austin). The following protocols were applied to the QCT scans: tube voltage, 120 kVp; tube current, 150 mA; acquisition field of view, 500; and matrix, 512×512. Musculoskeletal radiologists who were blinded to the clinical information of all of the subjects independently calculated the BMD values. Raw lumbar spine data were sent to the QCT Pro workstation (Mindways, Austin) for BMD calculations. After selecting the L1, L2, and L3 vertebrae as measurement targets and setting the positioning line at the center of the vertebrae, the QCT Pro software automatically identifies the L1, L2, and L3 vertebrae and generates a region of interest (ROI). Subsequently, the size of the ROI was manually adjusted to adequately cover the cancellous bone area, and the boundaries of the ROI were adjusted to avoid the cortical bone (Figure 1). The average of the L1, L2, and L3 vertebral body BMD values was regarded as the final result for each subject. According to the diagnostic thresholds for vertebral BMD recommended by the International Society for Clinical Densitometry, normal BMD, osteopenia, and osteoporosis were defined as BMD >120, 80–120, and <80 mg/cm3, respectively (21).
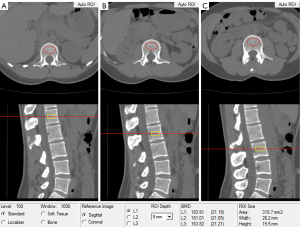
MRI examination
Spinal imaging was performed by using a 3.0T MRI scanner (MAGNETOM Prisma; Siemens Healthcare, Erlangen, Germany) equipped with a 32-channel spine matrix coil with the subject in a supine head advanced position. Conventional scanning protocols included sagittal turbo spin‒echo T1-weighted and T2-weighted scans. Sagittal Q-Dixon images were acquired by using a T2*-corrected 6-echo volumetric interpolation breath-hold examination sequence. A seven-peaked fat distribution model with multiple lipid components was used to accurately quantify the spatial distribution of bone marrow fat. A small flip angle (4°) was used to reduce the T1 relaxation effects. In addition, the system’s Maplt software automatically acquired fat fraction (FF) and T2* maps (Figure 2). Sagittal DKI images were acquired by using a reduced-FOV ssEPI sequence with 4 b values (0, 500, 1,000, and 2,000 s/mm2); moreover, each b value has three orthogonal directions. Both Q-Dixon and DKI used a 2-factor parallel imaging acceleration technique. The detailed imaging parameters are summarized in Table 1.
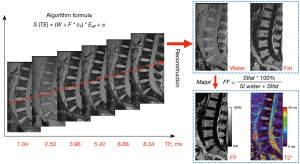
Table 1
MRI protocols | TSE T1-weighted | TSE T2-weighted | Q-Dixon | Reduced-FOV DKI |
---|---|---|---|---|
Time to repetition, ms | 460 | 2400 | 10.30 | 3,200 |
Time to echo, ms | 8.6 | 89 | 1.04, 2.50, 3.96, 5.42, 6.88, 8.34 | 67, 96 |
b values, s/mm2 | – | – | – | 0, 500, 1,000, 2,000 |
No. slices | 11 | 11 | 26 | 11 |
Slice thickness, mm | 4 | 4 | 4 | 4 |
Interslice gap, mm | 0.3 | 0.3 | 0.3 | 0.3 |
FOV, mm2 | 280×280 | 280×280 | 256×256 | 230×230 |
Acquisition matrix | 224×320 | 224×320 | 180×192 | 130×166 |
Voxel size, mm3 | 0.4×0.4×4.0 | 0.9×0.9×4.0 | 1.3×1.3×3.0 | 1.8×1.8×5.0 |
Phase encoding direction | H >> F | H >> F | A >> P | A >> P |
No. averages | 1 | 1 | 3 | 1, 1, 2, 2 |
Flip angle, degrees | 150 | 120 | 4 | 180 |
Bandwidth, Hz/Px | 252 | 252 | 900 | 1,085 |
Acquisition time, min:s | 00:56 | 00:58 | 01:35 | 04:26 |
MRI, magnetic resonance imaging; TSE, turbo spin-echo; Q-Dixon, quantitative Dixon; FOV, field of view; DKI, diffusion kurtosis imaging; H, head; F, feet; A, anterior; P, posterior. Hz, Hertz; Px, pixel.
MR image analysis
Two musculoskeletal radiologists who were blinded to the clinical information and QCT results independently outlined the ROIs and estimated the quantitative parameters of the Q-Dixon and DKI sequences.
T2*-corrected six-echo Q-Dixon analysis
The reconstructed FF and T2* maps were transferred to a Siemens Syngo workstation (B17; Siemens Healthineers) for data extraction. In this study, the ROI positioning procedure was similar to the manual approach that has been proposed in previous studies (9,22). To adequately assess the spatial distribution of bone marrow fat, rectangular ROIs were manually outlined on the L1, L2, and L3 vertebral bodies at the central level of the sagittal FF map and six evenly distributed levels adjacent to both sides. The ROI should have been as large as possible while also avoiding the bone cortex. The average of the L1, L2, and L3 vertebral body FF values was the final FF result for each subject. Subsequently, the ROIs were copied to the T2* maps to calculate the T2* values. FF values represent the percentage of bone marrow fat content. Furthermore, T2* values reflect changes in transverse relaxation time and magnetization rate within the bone marrow.
Reduced-FOV DKI analysis
The DKI images were processed by using the MR body diffusion toolbox (Version 1.4.0; Siemens Healthineers) software. Traditional apparent diffusion coefficient (ADC) values were calculated from a single exponential model fitted with two b values (b =0 and 1,000 s/mm2) of signal intensity with the following equation:
where Sb represents the signal intensity when the b value is not 0, and S0 represents the signal intensity when the b value is 0 s/mm2.
We used signal intensity data from four b-values (0, 500, 1,000, and 2,000 s/mm2) to construct the DKI model (Figure 3). The DKI model is described by the following equation:
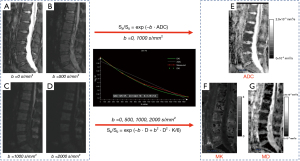
where D represents the non-Gaussian corrected ADC, and K is the diffusion kurtosis coefficient, which indicated the deviation of water motion from Gaussian diffusion.
ROIs were manually outlined on the ADC, K, and D maps. The ROI outlining principle was kept consistent with the Q-Dixon sequence to adequately assess the cancellous bone region of the vertebral body. Finally, the ADC, mean kurtosis (MK), and mean diffusivity (MD) values were obtained for each subject.
Statistical analysis
SPSS 26.0 (IBM Corp., Armonk, NY, USA) software was used for the statistical analysis. The interobserver agreement between the QCT and MRI measurements was analyzed by using the intraclass correlation coefficient (ICC). The Kolmogorov‒Smirnov test was used to determine the normality of all of the continuous variable data. Data conforming to a normal distribution are expressed as , whereas nonnormally distributed data are defined as the median (upper and lower quartiles). Due to the fact that BMD values were nonnormally distributed data, the correlations between BMD and quantitative MRI parameters were analyzed by using Spearman’s correlation coefficient instead of Pearson’s correlation coefficient. For normally distributed data, one-way analysis of variance was used for the multiple intergroup comparisons, and Holm’s method was used for post-hoc comparisons. For nonnormally distributed data, Kruskal‒Wallis tests for multiple intergroup comparisons and Wilcoxon tests for post-hoc comparisons were performed. In addition, receiver operating characteristic (ROC) curves were used to analyze the diagnostic efficacy of MRI parameters for screening abnormal BMD and osteoporosis. A single-parameter analysis was first performed to determine the optimal cutoff value by using the Youden index, followed by performing a combined multiparameter analysis between the Q-Dixon and DKI models. Multivariable logistic regression modeling was performed by using individual MRI parameters as predictors and abnormal BMD or osteoporosis as outcomes. The predicted probabilities that were generated by the logistic regression models were then used to construct ROC curves. The DeLong test was used to compare whether the differences between the models were statistically significant. P values less than 0.05 were considered to be statistically significant.
Results
Clinical baseline information and MRI characteristics
Figure 4 shows the flow chart of the participants who were included in this study. According to the measurement of BMD values, 52 participants were in the normal group, 51 were in the osteopenia group, and 52 were in the osteoporosis group. There were no significant differences in IPAQ results, as well as smoking and alcohol consumption findings, among the three groups (P=0.79, P=0.81, and P=0.89, respectively). No significant differences were observed between groups for age and BMI (P=0.87 and P=0.89, respectively). The clinical and quantitative MRI characteristics of all of the subjects are summarized in Table 2. Two observers with high reproducibility measured Q-Dixon and DKI parameters (Table 3). The ICC values ranged from 0.892–0.952. In addition, the percentage coefficient of variation (CV%) ranged from 3.3–4.8%. Figure 5 shows the Bland‒Altman plots of the MRI parameters that were calculated by the two observers. Table 4 demonstrates the results of the post-hoc test analysis of quantitative MRI parameters between the different BMD groups. There were significant differences observed in FF, T2*, and MK values between subjects with normal BMD, osteopenia, and osteoporosis (P<0.001, P<0.001, and P<0.001, respectively). Specifically, the highest FF, T2*, and MK values were observed in the osteoporotic group, and the lowest values were observed in the normal group. The MD values of the normal group were significantly higher than those of the osteopenia and osteoporosis groups (P=0.007 and P<0.001, respectively). However, there were no significant differences in MD values between the osteopenia and osteoporosis groups (P=0.54). No significant differences were observed in ADC values between the three groups of subjects (P=0.08). Figure 6 demonstrates three examples of typical subjects illustrating trends in bone marrow MRI parameters that differed between the normal BMD, osteopenia, and osteoporosis groups.
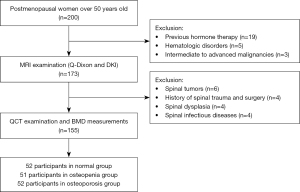
Table 2
Characteristics | Normal (n=52) | Osteopenia (n=51) | Osteoporosis (n=52) | P value |
---|---|---|---|---|
IPAD results† | 0.79 | |||
Sedentary | 8 (15.3) | 7 (13.7) | 12 (23.1) | |
Minimally active | 33 (63.5) | 30 (58.8) | 28 (53.8) | |
Very active | 11 (21.2) | 14 (27.5) | 12 (23.1) | |
Smoking† | 0.81 | |||
Yes | 12 (23.1) | 9 (17.6) | 15 (28.8) | |
No | 40 (76.9) | 42 (82.4) | 37 (71.2) | |
Alcohol consumption† | 0.89 | |||
Yes | 7 (13.5) | 9 (17.6) | 6 (11.5) | |
No | 45 (86.5) | 42 (82.4) | 46 (88.5) | |
Age (years old)§ | 64.1±5.2 | 67.4±4.8 | 66.3±6.5 | 0.87 |
BMI (kg/m2)§ | 23.8±2.8 | 24.6±3.3 | 24.2±3.3 | 0.89 |
BMD (mg/cm2)# | 146.2 (134.2, 161.1) | 101.5 (92.6, 110.6) | 70.1 (64.5, 74.1) | <0.001* |
FF (%)§ | 48.7±5.3 | 56.2±4.8 | 60.9±5.1 | <0.001* |
T2* (ms)# | 6.4 (6.1, 8.1) | 10.0 (8.9, 12.6) | 12.1 (10.3, 13.5) | <0.001* |
ADC (×10−3 mm2/s)# | 0.38 (0.33, 0.43) | 0.39 (0.34, 0.45) | 0.38 (0.33, 0.56) | 0.12 |
MK§ | 1.35±0.52 | 1.65±0.41 | 2.09±0.42 | <0.001* |
MD (×10−3 mm2/s)§ | 1.13±0.17 | 0.99±0.20 | 0.95±0.26 | 0.003* |
†, data are presented as No. (%); §, data are presented as the mean ± standard deviation; #, data are presented as medians, with upper and lower quartiles in parentheses; *, the result is statistically significant. MRI, magnetic resonance imaging; IPAQ, international physical activity questionnaire; BMI, body mass index; BMD, bone mineral density; FF, fat fraction; ADC, apparent diffusion coefficient; MK, mean kurtosis; MD, mean diffusivity.
Table 3
MRI parameters | ICC (95% CI) | CVs (95% CI) |
---|---|---|
ADC | 0.891 (0.842–0.932) | 4.8 (2.6–7.9) |
FF | 0.927 (0.871–0.967) | 3.8 (2.0–6.7) |
T2* | 0.942 (0.911–0.967) | 3.3 (1.7–6.3) |
MK | 0.908 (0.851–0.943) | 4.4 (2.4–7.6) |
MD | 0.921 (0.846–0.963) | 4.1 (2.2–7.2) |
MRI, magnetic resonance imaging; ICC, intraclass correlation coefficient; CI, confidence interval; CVs, coefficients of variation; ADC, apparent diffusion coefficient; FF, fat fraction; MK, mean kurtosis; MD, mean diffusivity.
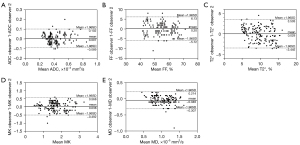
Table 4
MRI parameters | Normal vs. osteopenia | Normal vs. osteoporosis | Osteopenia vs. osteoporosis |
---|---|---|---|
FF (%) | <0.001* | <0.001* | <0.001* |
T2* (ms) | <0.001* | <0.001* | 0.003* |
ADC (×10−3 mm2/s) | 0.81 | 0.10 | 0.21 |
MK | 0.003* | <0.001* | <0.001* |
MD (×10−3 mm2/s) | 0.007 | <0.001* | 0.51 |
*, the result is statistically significant. MRI, magnetic resonance imaging; BMD, bone mineral density; FF, fat fraction; ADC, apparent diffusion coefficient; MK, mean kurtosis; MD, mean diffusivity.
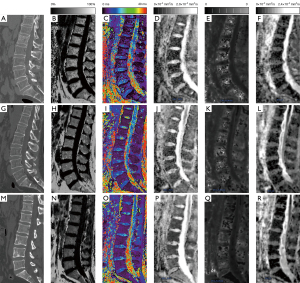
Correlation between BMD values and MRI parameters
Figure 7 illustrates the scatter plot between BMD values and quantitative MRI parameters. A strong inverse correlation was observed between FF and BMD values (r=−0.739, P<0.001). T2* values were strongly negatively correlated with BMD values (r=−0.676, P<0.001). Moreover, there was also a significant negative correlation between MK and BMD values (r=−0.626, P<0.001). Conversely, MD values showed a weak positive correlation with BMD values (r=0.304, P<0.001). Specifically, as the BMD values decreased, the FF, T2*, and MK values significantly increased, whereas the MD values decreased. However, no significant correlation was observed between ADC and BMD values (r=−0.027, P=0.74).
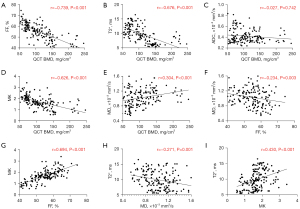
Correlation between Q-Dixon and DKI parameters
As shown in Figure 7, MK values exhibited a significant positive correlation with FF values (r=0.694, P<0.001), whereas there was a weak negative correlation between MD and FF values (r=−0.234, P=0.003). However, ADC values exhibited no significant correlation with FF values (r=0.005, P=0.95).
A moderate positive correlation was observed between MK and T2* values (r=0.430, P<0.001). A weak negative correlation existed between MD and T2* values (r=−0.271, P=0.001). Furthermore, no correlation was observed between ADC and T2* values (r=0.048, P=0.55).
Diagnostic performance of Q-Dixon and DKI parameters for predicting the normal BMD group and abnormal BMD (osteopenia and osteoporosis) group
In the current diagnostic model, osteopenia and osteoporosis are classified in the abnormal BMD group and compared to the normal BMD group. The single-parameter and combined multiparameter ROC curve results that were used to distinguish normal from abnormal BMD are shown in Table 5 and Figure 8A-8C. Both FF and T2* values predicted abnormal BMD, with AUCs above 0.90. The T2* value was observed to have the best AUC (0.939) and positive predictive value (PPV, 95.45%), whereas the FF value exhibited the best accuracy (86.45%) and negative predictive value (NPV, 75.41%). MK and MD values had slightly lower diagnostic efficacy; however, MK values demonstrated the best sensitivity (90.29%), and MD values displayed the best specificity (96.15%), for screening abnormal BMD. The combined multiparameter model improved the predictive power of abnormal BMD. According to the DeLong test, excellent performance was observed in the Q-Dixon differentiation between normal and abnormal BMD subjects and was significantly better than the DKI model (AUC: 0.976 vs. 0.812, respectively; P<0.001). The best diagnostic efficacy was obtained with the combined four-parameter diagnostic model.
Table 5
MRI parameters | AUC (95% CI) | Cutoff value | Specificity, % | Sensitivity, % | Accuracy, % | PLR | NLR | PPV, % | NPV, % |
---|---|---|---|---|---|---|---|---|---|
FF | 0.902 (0.848–0.956) | 53.65% | 88.46 | 85.44 | 86.45 | 7.40 | 0.16 | 93.62 | 75.41 |
T2* | 0.939 (0.903–0.975) | 9.35 ms | 92.31 | 80.58 | 84.52 | 10.48 | 0.21 | 95.45 | 70.59 |
MK | 0.774 (0.693–0.854) | 1.44 | 53.85 | 90.29 | 78.06 | 1.96 | 0.18 | 79.49 | 73.68 |
MD | 0.703 (0.623–0.783) | 0.88×10−3 mm2/s | 96.15 | 40.78 | 59.35 | 10.60 | 0.62 | 95.40 | 45.05 |
Q-Dixon | 0.976 (0.955–0.997) | – | 96.15 | 89.32 | 91.61 | 23.22 | 0.11 | 97.87 | 81.97 |
DKI | 0.812 (0.741–0.882) | – | 88.46 | 61.17 | 70.32 | 5.30 | 0.44 | 91.30 | 53.49 |
Q-Dixon+DKI | 0.978 (0.959–0.997) | – | 96.15 | 91.26 | 92.90 | 23.73 | 0.09 | 97.92 | 84.75 |
MRI, magnetic resonance imaging; ROC, receiver operating characteristic; BMD, bone mineral density; AUC, area under curve; CI, confidence interval; FF, fat fraction; MK, mean kurtosis; MD, mean diffusivity; Q-Dixon, quantitative Dixon; DKI, diffusion kurtosis imaging; PLR, positive likelihood ratio; NLR, negative likelihood ratio; PPV, positive predictive value; NPV, negative predictive value.
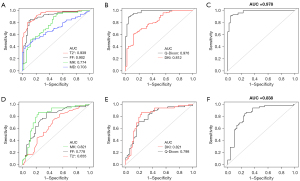
Diagnostic performance of Q-Dixon and DKI parameters for predicting osteopenia and osteoporosis
Single-parameter ROC analysis showed that MK values predicted osteoporosis with the best AUC (0.821), sensitivity (86.54%), specificity (76.47%), accuracy (81.55%), PPV (78.95%), and NPV (84.78%). The DeLong test demonstrated no significant difference in AUC between the MK and FF models (0.821 vs. 0.778, respectively; P=0.39), whereas the AUC of the MK value model was significantly better than that of T2* (0.821 vs. 0.655, respectively; P=0.01). Although the AUC of the DKI model to identify osteoporosis was slightly higher than that of the Q-Dixon model, the DeLong test showed that the difference between the two models was not statistically significant (AUC: DKI, 0.825 vs. Q-Dixon, 0.798; P=0.57). The final combined model showed the best diagnostic effect in differentiating between subjects with osteopenia and osteoporosis (Table 6, Figure 8D-8F).
Table 6
MRI parameters | AUC (95% CI) | Cutoff value | Specificity, % | Sensitivity, % | Accuracy, % | PLR | NLR | PPV, % | NPV, % |
---|---|---|---|---|---|---|---|---|---|
FF | 0.778 (0.687–0.870) | 56.75% | 64.71 | 86.54 | 75.73 | 2.45 | 0.21 | 71.43 | 82.50 |
T2* | 0.655 (0.548–0.762) | 10.45 ms | 54.90 | 73.08 | 64.08 | 1.62 | 0.49 | 62.30 | 66.67 |
MK | 0.821 (0.734–0.908) | 1.75 | 76.47 | 86.54 | 81.55 | 3.68 | 0.18 | 78.95 | 84.78 |
MD | 0.571 (0.458–0.684) | 0.81×10−3 mm2/s | 86.27 | 38.46 | 62.14 | 2.80 | 0.71 | 74.07 | 57.89 |
Q-Dixon | 0.798 (0.710–0.886) | – | 80.39 | 71.15 | 75.73 | 3.63 | 0.36 | 78.72 | 73.21 |
DKI | 0.825 (0.739–0.910) | – | 76.47 | 82.69 | 79.61 | 3.51 | 0.23 | 78.18 | 81.25 |
Q-Dixon+DKI | 0.838 (0.758–0.919) | – | 74.51 | 84.62 | 79.61 | 3.32 | 0.21 | 77.19 | 82.61 |
MRI, magnetic resonance imaging; ROC, receiver operating characteristic; AUC, area under curve; CI, confidence interval; FF, fat fraction; MK, mean kurtosis; MD, mean diffusivity; Q-Dixon, quantitative Dixon; DKI, diffusion kurtosis imaging; PLR, positive likelihood ratio; NLR, negative likelihood ratio; PPV, positive predictive value; NPV, negative predictive value.
Discussion
In this study, we evaluated the feasibility of using multiparametric functional MRI to assess the composition of the bone marrow microenvironment in postmenopausal women and explored the ability of MRI parameters to identify abnormal BMD and osteoporosis. Q-Dixon’s FF and T2* values, as well as the MK and MD values of DKI, showed varying degrees of correlation with BMD values. Furthermore, diagnostic models have shown that the Q-Dixon model excelled in identifying the presence of abnormal BMD by quantifying bone marrow fat distribution. Moreover, the introduction of DKI significantly improved the prediction of osteopenia and osteoporosis.
The Dixon technique based on 6-echo and T2* correction has good spatial resolution and simple technical requirements and was first applied in evaluating liver degeneration (23). In recent years, the Dixon technique has been progressively used for spinal lesions, including for the assessment of osteoporosis, the differentiation of benign and malignant spinal lesions, and differential diagnosis of acute osteoporotic and malignant fractures (24-27). We observed a tendency for the bone marrow FF to increase as BMD decreased. The highest correlation between FF and BMD values was observed, which is consistent with that of previous studies (9,28,29). The results of FF values reflect the fact that there is competition between adipogenesis and osteogenesis in the bone marrow. Several studies have shown that FF values may become a potential alternative to BMD values in the detection of osteoporosis (8,30). T2* values reflect the magnetic anisotropy of the tissue. These values are closely related to the microscopic composition and number of bone trabeculae, which reflect the reversible transverse relaxation time T2 caused by phase deviations due to magnetic field inhomogeneities within the tissue (31). In the current study, T2* values increased with decreasing BMD, which is consistent with previous studies (8,32). The change in T2* values reflects the loss of bone trabeculae and a large amount of adipose tissue that reduces local magnetic field inhomogeneity during the progression of osteoporosis, thereby delaying T2* decay.
Moreover, our team has already explored the susceptibility of Q-Dixon to identify osteoporosis in a previous study (8). However, the previous study included a relatively small group of subjects with osteopenia (n=27) and a total sample size (n=105), which may have contributed to the inability of the T2* values to distinguish between osteopenia and osteoporosis. In this study, we expanded the study sample. The results showed that T2* values not only differed between the normal and abnormal BMD groups but also had the potential to differentiate between osteopenia and osteoporosis.
Diffusion techniques for the assessment of osteoporosis are controversial, which is often due to differences in pulse sequences and the lack of a uniform reference standard for b-values (33,34). As has been previously reported, isotropic DWI techniques assessed normal bone marrow ADC values of (0.2–0.6)×10−3 mm2/s (35). A previous study based on the IVIM model by our team also reported of a pure water molecule diffusion coefficient (Dslow) value of 0.34×10−3 mm2/s in normal bone marrow (8). Dslow values allowed for the identification of subjects with normal BMD, osteopenia, and osteoporosis. However, there are limitations in the diagnostic efficacy of Dfast values and f values for identifying osteopenia and osteoporosis. It should be noted that both DWI and IVIM models reflect Gaussian motion of water molecules, whereas bone tissue is significantly anisotropic (19). Water molecules in bone tissue are represented by non-Gaussian motion patterns. In the present study, we introduced a DKI model based on the non-Gaussian motion pattern of water molecules. DKI quantifies the extent to which the true diffusional displacement of water molecules deviates from the Gaussian distribution by introducing the concept of kurtosis. As has been previously reported, DKI may be more sensitive to reflect the complexity and heterogeneity within the tissue than the anisotropic DWI technique (36-38). We found similar findings in our study targeting trabecular structures and the bone marrow microenvironment. In the present study, there was no significant correlation between conventional monoexponential ADC values and FF values and no prediction of abnormal BMD or osteoporosis. With non-Gaussian correction, the MD of normal bone marrow was 1.13×10-3 mm2/s, which is higher than the upper limit reported in a previous study (35). MD values were positively correlated with BMD values. As BMD diminished, bone marrow water molecules exhibited significantly restricted motility. Indeed, the diffusion coefficient of yellow marrow was considerably lower than that of red marrow, which is the main pathophysiological factor for the diminished diffusion signal caused by fatty transformation in the progression of osteoporosis (39).
The performance of MK values in osteoporosis assessment is an exciting prospect. According to our observations, there was a significant negative correlation between MK and BMD values. Red‒yellow bone marrow conversion is a key pathological change in osteoporosis. Furthermore, iron overload, trabecular microfractures, and reduced perfusion will lead to increased heterogeneity of the bone marrow microenvironment. These pathological changes can lead to a diversity of bone marrow components and structural complexity, which may be the dominant factor causing the increase in MK values (31,40,41). Furthermore, MK values were shown to have a significant positive correlation with FF values, thus implying that bone marrow fat deposition may be an important factor in increasing bone marrow heterogeneity. To our knowledge, this is the first application of the DKI technique in the assessment of osteoporosis.
We also investigated the diagnostic efficacy of different models for the discrimination of abnormal BMD and osteoporosis. We found that the Q-Dixon model was significantly better than the DKI model in screening for abnormal BMD. However, the DKI model showed the best diagnostic efficacy in differentiating between osteopenia and osteoporosis. This result implies that fat deposition within the bone marrow is a sensitive measure for identifying abnormal BMD and that changes in structural heterogeneity of the bone marrow microenvironment measured by DKI may provide incremental information in detecting osteoporosis.
Several limitations should be considered when interpreting the results. First, this study was a single-center study with a small sample size. Second, this study focused only on postmenopausal women; however, osteoporosis can also affect older men. Future studies will need to expand on the sample size and study population to include patients with osteoporosis of different sexes. Finally, the optimal parameter selection for spinal DKI still needs to be further explored, which would be more beneficial for examining the overall microstructural changes in the bone marrow microenvironment.
Conclusions
In conclusion, the noninvasive assessment of lumbar spine osteoporosis via six-echo Q-Dixon and reduced FOV DKI techniques is feasible. The derived parameters of both methods may be used as imaging markers for the detection and quantification of bone marrow microenvironment alterations in postmenopausal women. Multimodal MRI is a potential alternative to QCT for identifying postmenopausal individuals with osteopenia and osteoporosis.
Acknowledgments
Funding: This work was supported by the National Natural Science Foundation of China (Nos. 82271968 and 82171911).
Footnote
Reporting Checklist: The authors have completed the STARD reporting checklist. Available at https://qims.amegroups.com/article/view/10.21037/qims-22-1247/rc
Conflicts of Interest: All authors have completed the ICMJE uniform disclosure form (available at https://qims.amegroups.com/article/view/10.21037/qims-22-1247/coif). All authors report that this work was supported by the National Natural Science Foundation of China (Nos. 82271968 and 82171911). QL and RG are employees of Siemens Healthcare. The authors have no other conflicts of interest to declare.
Ethical Statement: The authors are accountable for all aspects of the work in ensuring that questions related to the accuracy or integrity of any part of the work are appropriately investigated and resolved. The study was conducted in accordance with the Declaration of Helsinki (as revised in 2013). The study was approved by the Health Sciences Institutional Review Board (HIRB) of Huashan Hospital, Fudan University (No. KY2023-053), and informed consent was obtained from all the individual participants.
Open Access Statement: This is an Open Access article distributed in accordance with the Creative Commons Attribution-NonCommercial-NoDerivs 4.0 International License (CC BY-NC-ND 4.0), which permits the non-commercial replication and distribution of the article with the strict proviso that no changes or edits are made and the original work is properly cited (including links to both the formal publication through the relevant DOI and the license). See: https://creativecommons.org/licenses/by-nc-nd/4.0/.
References
- Compston JE, McClung MR, Leslie WD. Osteoporosis. Lancet 2019;393:364-76. [Crossref] [PubMed]
- Bliuc D, Nguyen ND, Nguyen TV, Eisman JA, Center JR. Compound risk of high mortality following osteoporotic fracture and refracture in elderly women and men. J Bone Miner Res 2013;28:2317-24. [Crossref] [PubMed]
- Martel D, Monga A, Chang G. Osteoporosis Imaging. Radiol Clin North Am 2022;60:537-45. [Crossref] [PubMed]
- Wáng YXJ, Xiao BH. Estimations of bone mineral density defined osteoporosis prevalence and cutpoint T-score for defining osteoporosis among older Chinese population: a framework based on relative fragility fracture risks. Quant Imaging Med Surg 2022;12:4346-60. [Crossref] [PubMed]
- Damilakis J, Maris TG, Karantanas AH. An update on the assessment of osteoporosis using radiologic techniques. Eur Radiol 2007;17:1591-602. [Crossref] [PubMed]
- Cheng X, Zhao K, Zha X, Du X, Li Y, Chen S, et al. Opportunistic Screening Using Low-Dose CT and the Prevalence of Osteoporosis in China: A Nationwide, Multicenter Study. J Bone Miner Res 2021;36:427-35. [Crossref] [PubMed]
- Lewiecki EM. Imaging technologies for assessment of skeletal health in men. Curr Osteoporos Rep 2013;11:1-10. [Crossref] [PubMed]
- Li X, Lu R, Xie Y, Li Q, Tao H, Chen S. Identification of abnormal BMD and osteoporosis in postmenopausal women with T2*-corrected Q-Dixon and reduced-FOV IVIM: correlation with QCT. Eur Radiol 2022;32:4707-17. [Crossref] [PubMed]
- Zhao Y, Huang M, Ding J, Zhang X, Spuhler K, Hu S, Li M, Fan W, Chen L, Zhang X, Li S, Zhou Q, Huang C. Prediction of Abnormal Bone Density and Osteoporosis From Lumbar Spine MR Using Modified Dixon Quant in 257 Subjects With Quantitative Computed Tomography as Reference. J Magn Reson Imaging 2019;49:390-9. [Crossref] [PubMed]
- Li X, Xie Y, Lu R, Zhang Y, Li Q, Kober T, Hilbert T, Tao H, Chen S. Q-Dixon and GRAPPATINI T2 Mapping Parameters: A Whole Spinal Assessment of the Relationship Between Osteoporosis and Intervertebral Disc Degeneration. J Magn Reson Imaging 2022;55:1536-46. [Crossref] [PubMed]
- Grimm A, Meyer H, Nickel MD, Nittka M, Raithel E, Chaudry O, Friedberger A, Uder M, Kemmler W, Quick HH, Engelke K. Evaluation of 2-point, 3-point, and 6-point Dixon magnetic resonance imaging with flexible echo timing for muscle fat quantification. Eur J Radiol 2018;103:57-64. [Crossref] [PubMed]
- Lee SH, Yoo HJ, Yu SM, Hong SH, Choi JY, Chae HD. Fat Quantification in the Vertebral Body: Comparison of Modified Dixon Technique with Single-Voxel Magnetic Resonance Spectroscopy. Korean J Radiol 2019;20:126-33. [Crossref] [PubMed]
- Kim JW, Lee CH, Yang Z, Kim BH, Lee YS, Kim KA. The spectrum of magnetic resonance imaging proton density fat fraction (MRI-PDFF), magnetic resonance spectroscopy (MRS), and two different histopathologic methods (artificial intelligence vs. pathologist) in quantifying hepatic steatosis. Quant Imaging Med Surg 2022;12:5251-62. [Crossref] [PubMed]
- Aydıngöz Ü, Yıldız AE, Ergen FB. Zero Echo Time Musculoskeletal MRI: Technique, Optimization, Applications, and Pitfalls. Radiographics 2022;42:1398-414. [Crossref] [PubMed]
- Capuani S, Manenti G, Iundusi R, Tarantino U. Focus on diffusion MR investigations of musculoskeletal tissue to improve osteoporosis diagnosis: a brief practical review. Biomed Res Int 2015;2015:948610. [Crossref] [PubMed]
- Fathi Kazerooni A, Pozo JM, McCloskey EV, Saligheh Rad H, Frangi AF. Diffusion MRI for Assessment of Bone Quality; A Review of Findings in Healthy Aging and Osteoporosis. J Magn Reson Imaging 2020;51:975-92. [Crossref] [PubMed]
- Park EH, Lee YH, Jeong EK, Roh YH, Suh JS. Diffusion tensor imaging focusing on lower cervical spinal cord using 2D reduced FOV interleaved multislice single-shot diffusion-weighted echo-planar imaging: comparison with conventional single-shot diffusion-weighted echo-planar imaging. Magn Reson Imaging 2015;33:401-6. [Crossref] [PubMed]
- Kim H, Lee JM, Yoon JH, Jang JY, Kim SW, Ryu JK, Kannengiesser S, Han JK, Choi BI. Reduced Field-of-View Diffusion-Weighted Magnetic Resonance Imaging of the Pancreas: Comparison with Conventional Single-Shot Echo-Planar Imaging. Korean J Radiol 2015;16:1216-25. [Crossref] [PubMed]
- Capuani S, Rossi C, Alesiani M, Maraviglia B. Diffusion tensor imaging to study anisotropy in a particular porous system: the trabecular bone network. Solid State Nucl Magn Reson 2005;28:266-72. [Crossref] [PubMed]
- Jensen JH, Helpern JA, Ramani A, Lu H, Kaczynski K. Diffusional kurtosis imaging: the quantification of non-gaussian water diffusion by means of magnetic resonance imaging. Magn Reson Med 2005;53:1432-40. [Crossref] [PubMed]
- Engelke K, Adams JE, Armbrecht G, Augat P, Bogado CE, Bouxsein ML, Felsenberg D, Ito M, Prevrhal S, Hans DB, Lewiecki EM. Clinical use of quantitative computed tomography and peripheral quantitative computed tomography in the management of osteoporosis in adults: the 2007 ISCD Official Positions. J Clin Densitom 2008;11:123-62. [Crossref] [PubMed]
- Guo Y, Chen Y, Zhang X, Mei Y, Yi P, Wang Y, Feng Q, Tegola L, Guglielmi G, Zhang X, Feng Y. Magnetic Susceptibility and Fat Content in the Lumbar Spine of Postmenopausal Women With Varying Bone Mineral Density. J Magn Reson Imaging 2019;49:1020-8. [Crossref] [PubMed]
- Hupfeld S, Pischel D, Jechorek D, Janicová A, Pech M, Fischbach F. MRI-based fat quantification of the liver: Is it time for commercially available products? Eur J Radiol 2021;144:109993. [Crossref] [PubMed]
- Chang R, Ma X, Jiang Y, Huang D, Chen X, Zhang M, Hao D. Percentage fat fraction in magnetic resonance imaging: upgrading the osteoporosis-detecting parameter. BMC Med Imaging 2020;20:30. [Crossref] [PubMed]
- Burian E, Subburaj K, Mookiah MRK, Rohrmeier A, Hedderich DM, Dieckmeyer M, Diefenbach MN, Ruschke S, Rummeny EJ, Zimmer C, Kirschke JS, Karampinos DC, Baum T. Texture analysis of vertebral bone marrow using chemical shift encoding-based water-fat MRI: a feasibility study. Osteoporos Int 2019;30:1265-74. [Crossref] [PubMed]
- Schmeel FC, Luetkens JA, Wagenhäuser PJ, Meier-Schroers M, Kuetting DL, Feißt A, Gieseke J, Schmeel LC, Träber F, Schild HH, Kukuk GM. Proton density fat fraction (PDFF) MRI for differentiation of benign and malignant vertebral lesions. Eur Radiol 2018;28:2397-405. [Crossref] [PubMed]
- Donners R, Obmann MM, Boll D, Gutzeit A, Harder D. Dixon or DWI - Comparing the utility of fat fraction and apparent diffusion coefficient to distinguish between malignant and acute osteoporotic vertebral fractures. Eur J Radiol 2020;132:109342. [Crossref] [PubMed]
- Cohen A, Shen W, Dempster DW, Zhou H, Recker RR, Lappe JM, Kepley A, Kamanda-Kosseh M, Bucovsky M, Stein EM, Nickolas TL, Shane E. Marrow adiposity assessed on transiliac crest biopsy samples correlates with noninvasive measurement of marrow adiposity by proton magnetic resonance spectroscopy ((1)H-MRS) at the spine but not the femur. Osteoporos Int 2015;26:2471-8. [Crossref] [PubMed]
- Tang R, Tang G, Hua T, Tu Y, Ji R, Zhu J. mDIXON-Quant technique diagnostic accuracy for assessing bone mineral density in male adult population. BMC Musculoskelet Disord 2023;24:125. [Crossref] [PubMed]
- Kim D, Kim SK, Lee SJ, Choo HJ, Park JW, Kim KY. Simultaneous Estimation of the Fat Fraction and R2(*) Via T2(*)-Corrected 6-Echo Dixon Volumetric Interpolated Breath-hold Examination Imaging for Osteopenia and Osteoporosis Detection: Correlations with Sex, Age, and Menopause. Korean J Radiol 2019;20:916-30. [Crossref] [PubMed]
- Emoto T, Lu J, Sivasubramaniyam T, Maan H, Khan AB, Abow AA, et al. Colony stimulating factor-1 producing endothelial cells and mesenchymal stromal cells maintain monocytes within a perivascular bone marrow niche. Immunity 2022;55:862-878.e8. [Crossref] [PubMed]
- Wu HZ, Zhang XF, Han SM, Cao L, Wen JX, Wu WJ, Gao BL. Correlation of bone mineral density with MRI T2* values in quantitative analysis of lumbar osteoporosis. Arch Osteoporos 2020;15:18. [Crossref] [PubMed]
- Griffith JF, Yeung DK, Antonio GE, Wong SY, Kwok TC, Woo J, Leung PC. Vertebral marrow fat content and diffusion and perfusion indexes in women with varying bone density: MR evaluation. Radiology 2006;241:831-8. [Crossref] [PubMed]
- He J, Fang H, Na Li X. Vertebral bone marrow diffusivity in normal adults with varying bone densities at 3T diffusion-weighted imaging. Acta Radiol 2018;59:89-96. [Crossref] [PubMed]
- Dietrich O, Geith T, Reiser MF, Baur-Melnyk A. Diffusion imaging of the vertebral bone marrow. NMR Biomed 2017; [Crossref] [PubMed]
- Xiao Z, Tang Z, Zhang J, Yang G, Zeng W, Luo J, Song Y, Zhang Z. Whole-tumor histogram analysis of monoexponential and advanced diffusion-weighted imaging for sinonasal malignant tumors: Correlations with histopathologic features. J Magn Reson Imaging 2020;51:273-85. [Crossref] [PubMed]
- Ding M, Liu B, Chen X, Ouyang Z, Peng D, Zhou Y. MiR-99b-5p suppressed proliferation of human osteoblasts by targeting FGFR3 in osteoporosis. Hum Cell 2021;34:1398-409. [Crossref] [PubMed]
- Xiao Z, Zhong Y, Tang Z, Qiang J, Qian W, Wang R, Wang J, Wu L, Tang W, Zhang Z. Standard diffusion-weighted, diffusion kurtosis and intravoxel incoherent motion MR imaging of sinonasal malignancies: correlations with Ki-67 proliferation status. Eur Radiol 2018;28:2923-33. [Crossref] [PubMed]
- Lavdas I, Rockall AG, Castelli F, Sandhu RS, Papadaki A, Honeyfield L, Waldman AD, Aboagye EO. Apparent Diffusion Coefficient of Normal Abdominal Organs and Bone Marrow From Whole-Body DWI at 1.5 T: The Effect of Sex and Age. AJR Am J Roentgenol 2015;205:242-50. [Crossref] [PubMed]
- Zhu J, Zhang L, Wu X, Xiong Z, Qiu Y, Hua T, Tang G. Reduction of Longitudinal Vertebral Blood Perfusion and Its Likely Causes: A Quantitative Dynamic Contrast-enhanced MR Imaging Study of a Rat Osteoporosis Model. Radiology 2017;282:369-80. [Crossref] [PubMed]
- Chen Y, Guo Y, Zhang X, Mei Y, Feng Y, Zhang X. Bone susceptibility mapping with MRI is an alternative and reliable biomarker of osteoporosis in postmenopausal women. Eur Radiol 2018;28:5027-34. [Crossref] [PubMed]