Can spectral computed tomography (CT) replace perfusion CT to assess the histological classification of non-small cell lung cancer?
Introduction
Lung adenocarcinoma (LUAD) and lung squamous cell carcinoma (LUSC) are the two most common histological types of non-small cell lung cancer (NSCLC) (1,2). With the rapid development of precision medicine, the choice of NSCLC treatment is based on different pathological types and molecular phenotypes. For example, patients with LUAD and LUSC receive stereotactic external beam radiation simultaneously, with the former having a higher success rate than the latter (3). LUSC is less sensitive to pemetrexed than LUAD (4). Needle biopsy is a diagnostic method commonly used in clinical practice. However, the tumor tissue obtained by biopsy cannot fully reflect the biological characteristics of the lesion and may cause complications, such as pneumothorax, hemorrhage, and tumor metastasis (5). Therefore, it is necessary to explore a simple, noninvasive method to determine the pathological type of NSCLC before surgery.
Perfusion computed tomography (CT) can quantitatively evaluate tumor hemodynamics noninvasively (6) and has important clinical value in determining the pathological type of lung cancer and predicting the therapeutic efficacy and prognosis of lung cancer (7,8). However, perfusion CT has a high radiation dose owing to the need for multiple repeated acquisitions of lesions, which limits its wide application in clinical practice (9). Spectral CT is an imaging technique used to generate detailed images of the internal structures of the body using X-rays, which captures more extensive information about the composition of tissues and structures within the body by using multiple energy levels. The information can be visualized following the reconstruction process to obtain cross-sectional images of the internal structures of the body. One type of spectral CT is called dual-energy X-ray CT (DXCT) (10). Of note, early DXCT developments encountered issues with the basis material decomposition (BMD) concept, which required the mass attenuation coefficient of any material present in the body to be expressed as a linear combination of the mass attenuation coefficients of two basis materials (10). This resulted in energy-dependent systematic errors, particularly when radiographic contrast media was present (11). To address these issues, the basis material coefficients were numerically transformed into a more desirable set of basis materials, which is known as the BMD-DXCT method (12). This method has been shown to improve the accuracy of Single Photon Emission CT imaging by using DXCT images to provide object/energy-specific attenuation coefficient correction in SPECT imaging (13). Spectral CT has broad application prospects in the diagnosis and treatment of lung cancer by providing a variety of quantitative parameters to reflect the blood supply, functional and metabolic characteristics, and histopathological changes in tumors (14,15).
Some studies have shown that spectral and perfusion parameters are significantly correlated in liver, colorectal, and pancreatic cancers (16-18). However, no correlation study has confirmed their role in NSCLC and compared the accuracy of the two imaging modalities in identifying the histological type of NSCLC. We hypothesize that there is a correlation between spectral and perfusion parameters, with spectral CT exhibiting higher accuracy in identifying the histological type of NSCLC compared to perfusion CT, and that the clinical application of spectral CT in the histological classification of NSCLC will be more extensive than that of perfusion CT. Therefore, our study aimed to prospectively evaluate the relevance between spectral and perfusion parameters in NSCLC and to compare the differential diagnostic performance of the two CT modalities for the histological classification of NSCLC. We present this article in accordance with the STARD reporting checklist (available at https://qims.amegroups.com/article/view/10.21037/qims-22-1206/rc).
Methods
Patients
The study was conducted in accordance with the Declaration of Helsinki (as revised in 2013). The study was approved by the Medical Ethics Committee of Lanzhou University Second Hospital (No. 2021A-498) and informed consent was obtained from all the included patients. The study prospectively collected 221 patients with clinically suspected lung space-occupying lesions in our hospital who underwent “one-stop” spectral combined perfusion scan from September 2020 to December 2021 and with pathologically confirmed NSCLC. At the end, 62 patients were included (32 with LUAD and 30 with LUSC). The inclusion criteria were as follows: (I) CT showed solitary nodules or masses with lesions mearing ≥1 cm in diameter (n=194); (II) histopathologically confirmed LUAD or LUSC (n=153). The exclusion criteria were as follows: (I) treated with an anti-tumor or anti-inflammatory agent before undergoing CT (n=23); (II) incomplete clinical data (n=35); (III) period between the operation and spectral and perfusion CT scan ≥2 weeks (n=12); and (IV) poor quality of chest CT images (including respiratory motion artifacts or body surface metal artifact) (n=21). The inclusion and exclusion criteria are displayed in Figure 1.
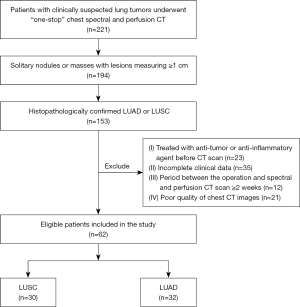
CT scanning protocol
All patients were scanned using a Revolution CT scanner (GE Healthcare, Milwaukee, WI, USA). The patients were first scanned unenhanced, followed by “one-stop” spectral-perfusion scanning protocol for all patients. The spectral scanning parameters: tube voltage, 80–140 kVp instantaneously (0.5 ms); tube current, automatically modulated; tube rotation time, 0.5 s; pitch, 0.984:1; collimation width, 128×0.625 mm; and Asir-V, 40%. The CT perfusion scanning parameters: tube current, 100 mA, and tube voltage 80–100 kVp. The other parameters were the same as those of the spectral CT. A nonionic iodine contrast agent (50–80 mL, 300 mgI/mL) was injected using a high-pressure syringe at an injection rate of 4–5.5 mL/s. The injection volume was 1.2 mL/kg, and then 30–40 mL normal saline was injected. The perfusion scan was started 2 s after the injection of the contrast agent, the arterial phase (AP) and venous phase (VP) spectral scan was performed at 30.2 and 52.6 s, respectively. Perfusion images were reconstructed using raw perfusion and spectral data in the AP and VP.
CT image analysis
All spectral and perfusion images were sent to the Advantage Workstation (AW) 4.7 post-processing workstation for analysis. Spectral and perfusion analysis was performed by two different radiologists (DLN and JJY with 5 and 8 years of experience in chest radiology, respectively). These radiologists were not aware of the study design and pathological results. Perfusion imaging analysis was performed first. The thoracic aorta and pulmonary artery at the same level as the lesion was defined as the input and output artery to put region of interest, and a pseudo-color map of each perfusion parameter was automatically generated. The layer with uniform lesion density and its adjacent higher and lower layers were selected to delineate three regions of interest (ROIs), and the final average was calculated. The perfusion parameters of the lesions included blood flow (BF), blood volume (BV), surface permeability (PS), and mean transit time (MTT). The spectral images used gemstone spectral imaging (GSI) Viewer to analyze. The measurement method was the same as that used for the perfusion. When measuring, we attempted to ensure that the size and position of the ROI in the perfusion and dual-phase spectra were consistent. The spectral parameters included iodine concentration (IC), effective atomic number (Zeff), CT40keV, and the formula used to calculate the slope was K70keV =(CT40keV – CT70keV)/(70 – 40).
Statistical analysis
The statistical analyses used SPSS 23.0 software (IBM, USA) and MedCalc (Version 19.2.3). The intraclass correlation coefficient (ICC) was used to analyze the consistency of the average spectral and perfusion parameters obtained by two radiologists. A value of P<0.05 was considered statistically significant. Categorical and continuous variables were expressed as frequencies or percentages and mean ± standard deviation or median and interquartile range, respectively. The Fisher’s exact test or chi-squared was used to assess categorical variables. The independent samples t-test or Mann-Whitney U test was used to compare the differences of continuous variables between the two groups. Correlations between the dual-phase spectral and perfusion parameters were assessed using Pearson or Spearman correlation analyses. A logistic regression model was constructed to evaluate different pathological types of NSCLC. The receiver operating characteristic (ROC) curve was drawn to assess the differential diagnostic efficiency of each parameter and model. The DeLong test was used to compare the diagnostic performance of different models.
Results
Clinical characteristics of the patients
There were 66.70% male and 34.40% female in the LUSC group, and 34.40% male and 65.60% female in LUAD group (P=0.01). Elevated SCCA values accounted for 46.70% and 18.80% in LUSC and LUAD (P=0.02), respectively. Elevated Cyfra21-1 levels accounted for 56.70% and 31.30% in LUSC and LUAD (P=0.04), respectively. However, there were no significant differences in age, smoking history, carcinoembryonic antigen, neuron-specific enolase, gastrin release peptide precursor levels, pathological grades, tumor size and tumor stage (P>0.05) (Table 1).
Table 1
Variables | LUSC (n=30) | LUAD (n=32) | χ2/t | P |
---|---|---|---|---|
Sex, n (%) | 6.46 | 0.01 | ||
Male | 20 (66.70) | 11 (34.40) | ||
Female | 10 (33.30) | 21 (65.60) | ||
Age (years) | 61.60±10.44 | 61.09±9.76 | −1.97 | 0.84 |
Smoking history, n (%)* | 14 (46.70) | 12 (37.5) | 0.53 | 0.47 |
Tumor markers, n (%) | ||||
CEA (+) | 13 (43.30) | 18 (56.30) | 1.03 | 0.31 |
NSE (+) | 15 (50.00) | 17 (53.10) | 0.06 | 0.81 |
ProGRP (+) | 6 (20.00) | 4 (12.50) | 0.64 | 0.42 |
SCCA (+) | 14 (46.70) | 6 (18.80) | 5.52 | 0.02 |
Cyfra21-1 (+) | 17 (56.70) | 10 (31.30) | 4.07 | 0.04 |
Pathological grades, n (%) | 0.27a | 0.95 | ||
Poorly-differentiated | 6 (20.00) | 7 (21.88) | ||
Moderately-differentiated | 13 (43.33) | 15 (46.88) | ||
Well-differentiated | 11 (36.67) | 10 (31.25) | ||
Tumor size (cm) | ||||
Maximum diameter | 4.63±1.35 | 4.08±1.45 | 1.57 | 0.12 |
Minimum diameter | 3.77±1.15 | 3.21±1.39 | 1.71 | 0.09 |
Tumor stage, n (%) | ||||
I | 2 (6.67) | 2 (6.25) | 0.99a | 0.88 |
II | 8 (26.67) | 11 (34.38) | ||
III | 14 (46.66) | 15 (46.87) | ||
IV | 6 (20.00) | 4 (12.50) |
Age and tumor size are presented as mean ± standard deviation, other variables are presented as number (%). a, Fisher exact test; *, smoking history is defined as follows: Yes, former and current smokers; No, never smoked. LUSC, lung squamous cell carcinoma; LUAD, lung adenocarcinoma; CEA, carcinoembryonic antigen; NSE, neuron-specific enolase; ProGRP, pro-gastrin-releasing peptide; SCCA, squamous cell carcinoma antigen; Cyfra 21-1, cytokeratin 19 fragment.
Correlation between spectral and perfusion parameters
In NSCLC, BV and BF were strongly correlated with dual-phase CT40keV, IC, Zeff, and K70keV (0.6<r<0.8, P<0.001). MTT was moderately correlated with dual-phase CT40keV, IC, Zeff, and K70keV (0.4<r<0.6, P<0.001). PS was not significantly correlated with CT40keV, IC, Zeff, and K70keV in the AP (P>0.05), but it was weakly correlated with CT40keV, IC, Zeff, and K70keV in the VP (0.2<r<0.4, P<0.05) (Table 2).
Table 2
NSCLC (n=62) | BV | BF | PS | MTT | |||||||
---|---|---|---|---|---|---|---|---|---|---|---|
r | P | r | P | r | P | r | P | ||||
AP | |||||||||||
CT40keV | 0.67 | <0.001 | 0.71 | <0.001 | 0.16 | 0.22 | 0.43 | <0.001 | |||
K70keV | 0.69 | <0.001 | 0.65 | <0.001 | 0.17 | 0.18 | 0.44 | <0.001 | |||
IC | 0.72 | <0.001 | 0.65 | <0.001 | 0.17 | 0.18 | 0.44 | <0.001 | |||
Zeff | 0.69 | <0.001 | 0.63 | <0.001 | 0.18 | 0.16 | 0.44 | <0.001 | |||
VP | |||||||||||
CT40keV | 0.73 | <0.001 | 0.63 | <0.001 | 0.28 | 0.03 | 0.51 | <0.001 | |||
K70keV | 0.78 | <0.001 | 0.68 | <0.001 | 0.27 | 0.04 | 0.59 | <0.001 | |||
IC | 0.78 | <0.001 | 0.68 | <0.001 | 0.27 | 0.04 | 0.59 | <0.001 | |||
Zeff | 0.77 | <0.001 | 0.68 | <0.001 | 0.26 | 0.04 | 0.58 | <0.001 |
NSCLC, non-small cell lung cancer; AP, arterial phase; CT40keV, the CT value of 40 keV; K70keV, the slope of the spectral curve; IC, iodine concentration; Zeff, effective atomic number; VP, venous phase; BV, blood volume; BF, blood flow; PS, surface permeability; MTT, mean transit time.
Quantitative analyses with spectral and perfusion CT
The ICC values for spectral and perfusion CT parameters ranged from 0.71–0.89. The spectral parameters of LUAD and LUSC are displayed in Table 3. In the AP and VP, the CT40keV, IC, Zeff, and K70keV of LUAD were higher than those of LUSC (P<0.01). The perfusion parameters of LUAD and LUSC are shown in Table 4. The BV, BF, PS, and MTT of LUAD were significantly lower than those of LUSC (P<0.05). Figure 2 and Figure 3 show examples of the spectral and perfusion parameters of LUAD and LUSC.
Table 3
Spectral parameters | LUSC (n=30) | LUAD (n=32) | t/Z | P |
---|---|---|---|---|
AP | ||||
CT40keV (HU) | 169.65±16.12 | 183.94±14.87 | −3.63 | <0.001 |
K70keV | 3.28±0.35 | 3.57±0.39 | −3.07 | 0.003 |
IC (100 µg/cm3) | 17.43±1.83 | 18.96±2.07 | −3.08 | 0.003 |
Zeff | 8.63±0.10 | 8.71±0.11 | −3.00 | 0.004 |
VP | ||||
CT40keV (HU) | 140.46±10.65 | 155.02±10.93 | −5.31 | <0.001 |
K70keV | 2.53±0.26 | 2.87±0.18 | −5.93 | <0.001 |
IC (100 µg/cm3) | 13.43±1.39 | 15.24±0.97 | −5.93 | <0.001 |
Zeff | 8.41±0.08 | 8.51±0.05 | −5.90 | <0.001 |
Data are presented as mean ± standard. LUSC, lung squamous cell carcinoma; LUAD, lung adenocarcinoma; AP, arterial phase; CT40keV, the CT value of 40 keV; HU, Hounsfiled Unit; K70keV, the slope of the spectral curve; IC, iodine concentration; Zeff, effective atomic number; VP, venous phase.
Table 4
Perfusion parameters | LUSC (n=30) | LUAD (n=32) | t/Z | P |
---|---|---|---|---|
BV (mL/100 g) | 5.33 (4.35, 6.89) | 7.32 (6.29, 9.21) | −3.31 | <0.001 |
BF [mL/(100 g·min)] | 69.63±13.51 | 79.46±13.93 | −2.82 | 0.007 |
PS [mL/(100 g·min)] | 18.74±3.80 | 22.43±4.84 | −3.33 | 0.002 |
MTT (s) | 10.97±3.86 | 13.20±3.44 | −2.41 | 0.02 |
BV is presented as median (interquartile range), other data are presented as mean ± standard. LUSC, lung squamous cell carcinoma; LUAD, Lung adenocarcinoma; BV, blood volume; BF, blood flow; PS, surface permeability; MTT, mean transit time.
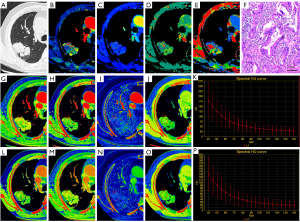
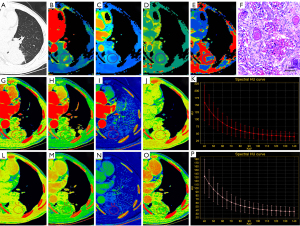
The differential diagnostic efficiency of spectral and perfusion CT for different pathological types of NSCLC are displayed in Table 5, Table 6 and Figure 4. The ROC curve analysis revealed that the AUC of the spectral parameter model was 0.93 (sensitivity =0.94, specificity =0.83), and the diagnostic efficiency was better than that of the perfusion parameter model (AUC =0.81, sensitivity =0.99, specificity =0.57). The DeLong test showed a significant difference between the AUC of the spectral and perfusion models (P<0.05).
Table 5
Models | AUC (95% CI) | Sensitivity | Specificity | P |
---|---|---|---|---|
Spectral CT | 0.93 (0.86–0.99) | 0.94 | 0.83 | 0.02 |
Perfusion CT | 0.81 (0.70–0.92) | 0.99 | 0.57 | 0.02 |
CT, computed tomography; AUC, area under curve; CI, confidence interval.
Table 6
Model | Pathological results | Total | |
---|---|---|---|
LUAD | LUSC | ||
Spectral CT | |||
LUAD | 30 | 6 | 36 |
LUSC | 2 | 24 | 26 |
Total | 32 | 30 | 62 |
Perfusion CT | |||
LUAD | 32 | 13 | 45 |
LUSC | 0 | 17 | 17 |
Total | 32 | 30 | 62 |
CT, computed tomography; NSCLC, non-small cell lung cancer; LUAD, lung adenocarcinoma; LUSC, lung squamous cell carcinoma.
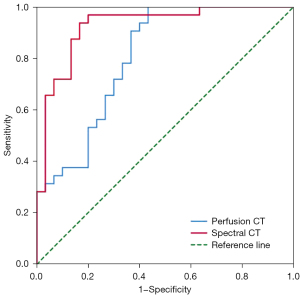
Discussion
Spectral CT has been considered an alternative to perfusion CT for hemodynamic analysis of lesions (17,19,20); however, no study has directly compared the two imaging modalities in patients with NSCLC. The study revealed that in patients with NSCLC, spectral CT parameters IC, Zeff, and K were significantly correlated with perfusion parameters BV, BF, and MTT. In addition, both spectral and perfusion CT have good diagnostic performance in differentiating different pathological types of NSCLC; however, the advantages of the former are more notable than those of the latter.
To the best of our knowledge, this study is the first to utilize “one-stop” spectral combined perfusion scanning to demonstrate the correlation between the two imaging modalities. Although previous studies have demonstrated significant correlations between energy spectrum and perfusion parameters in liver, pancreatic, and colorectal cancers (21-23), the advantage of the current study was in obtaining two quantitative parameters with only one contrast injection and one scan of the patient, which reduced the amount of contrast agent and shortened the examination time (24). In addition, we employed real-time iterative reconstruction Veo algorithm to reduce radiation dose while maintaining image quality through pre- and post-processing techniques (25). Importantly, our data had high consistency in both time and space, making our findings more accurate.
In spectral CT, lower keV values can increase tumor visibility due to the enhanced photoelectric effect (26,27). As the energy level approaches the iodine k-edge of 33 keV, the decay of iodine becomes more pronounced, and tissues with different atomic numbers can be distinguished by different spectral characteristics (28,29). Luo et al. (30) showed that the maximum AUC for identifying orbital lesions was obtained at 40 keV on the spectral attenuation curve, and the identification efficiency gradually decreased at higher energies. Kraus et al. (31) found that subjectively reconstruction under 40 keV was considered to be superior in terms of lesion significance, contour, focal sharpness and diagnostic reliability with good image quality. This is consistent with the results of a series of studies on head and neck, thyroid, liver, pancreas, kidney and intestinal lesions (32-37).
People might wonder whether spectral parameters at a point in time can replace dynamically acquired perfusion parameters. However, our results showed a significant correlation between perfusion (BV, BF, and MTT) and spectral parameters (CT40keV, IC, Zeff, and K70keV), whereas PS was not significantly correlated with spectral parameters in AP but was weakly correlated with CT40keV, IC, Zeff, and K70keV in the VP. This may be due to the use of the same scanning protocol for all the patients or the small sample size of our study. In some patients, the contrast agent did not completely fill the blood vessels and intercellular spaces because of the slow blood circulation during AP. Previous studies have shown that the spectra are significantly correlated with perfusion parameters in colorectal cancer (17), liver cancer (16), and pig lung model (38). Therefore, we can cautiously consider using that spectral CT to replace perfusion CT in assessing hemodynamic changes in NSCLC.
The results showed that spectral (IC, Zeff, and K70keV) and perfusion (BV, BF, MTT, and PS) parameters were significantly different between LUAD and LUSC, and that spectral CT showed better diagnostic performance than perfusion CT (AUC =0.93, sensitivity =0.94, specificity =0.83). Jia et al. compared the spectral parameters of LUAD and LUSC and found that the differences in IC, Zeff, and K between the two were statistically significant, and that the AUC of above three spectral parameters combined was 0.84 (39). Although some studies have shown that perfusion parameters have good differential diagnostic value in the histological classification of lung cancer, their diagnostic performance varies (40,41). This observation may be due to the software used by different vendors or differences in the study population. This study compared the diagnostic performance of two CT modalities based on the same study population using “one-stop” spectral combined perfusion scanning, and the results obtained were more objective and credible than those of previous studies.
Spectral CT has potential advantages in lung cancer, as it can simultaneously provide enhanced images and a variety of quantitative parameters, which can be used for both routine diagnosis and functional evaluation (16). Perfusion CT can evaluate hemodynamic changes in tumors quantitatively, especially in evaluating the efficacy of tumor anti-vascular therapy (42,43). Hemodynamic changes during treatment usually precede morphological changes. However, owing to the continuous dynamic scanning technique used in CT perfusion, limited scanning coverage and additional radiation doses are unavoidable. In addition, inaccurate perfusion parameters owing to respiratory motion artifacts limit their application in patients with suboptimal respiratory function (44). Therefore, because spectral CT can compensate for these deficiencies of perfusion CT, we propose it as an alternative to indirectly assess the tissue properties and hemodynamics of tumors in situations where low radiation is required and patients have suboptimal respiratory function.
The current had the following limitations: First, due to the low incidence of other pathological types of NSCLC, it was not included in this study, and other pathological types need to be collected and included in the future studied to infer broader and more accurate conclusions. Second, this study used the same scanning plan for all the patients and did not formulate a personalized scanning plan according to the patient’s heart and blood circulation status. Finally, because “one-stop” spectral combined perfusion scanning can only be achieved on higher-level CT, it cannot be widely implemented. However, with the rapid development of medical technology and the continuous exploration of scientific studies, this technology will certainly help in clinical diagnosis and treatment.
Conclusions
The results of our study showed a significant correlation between spectral and perfusion parameters in NSCLC, and spectral CT has a better diagnostic efficacy than perfusion CT in differentiating the histological classification of NSCLC. Therefore, in clinical practice, spectral CT may replace perfusion CT in assessing the histological properties and hemodynamics of tumors.
Acknowledgments
Funding: This work was supported by the National Natural Science Foundation of China (No. 82071872).
Footnote
Reporting Checklist: The authors have completed the STARD reporting checklist. Available at https://qims.amegroups.com/article/view/10.21037/qims-22-1206/rc
Conflicts of Interest: All authors have completed the ICMJE uniform disclosure form (available at https://qims.amegroups.com/article/view/10.21037/qims-22-1206/coif). The authors report that this work was supported by the National Natural Science Foundation of China (No. 82071872). The authors have no other conflicts of interest to declare.
Ethical Statement:
Open Access Statement: This is an Open Access article distributed in accordance with the Creative Commons Attribution-NonCommercial-NoDerivs 4.0 International License (CC BY-NC-ND 4.0), which permits the non-commercial replication and distribution of the article with the strict proviso that no changes or edits are made and the original work is properly cited (including links to both the formal publication through the relevant DOI and the license). See: https://creativecommons.org/licenses/by-nc-nd/4.0/.
References
- Meng F, Zhang L, Ren Y, Ma Q. The genomic alterations of lung adenocarcinoma and lung squamous cell carcinoma can explain the differences of their overall survival rates. J Cell Physiol 2019;234:10918-25. [Crossref] [PubMed]
- Smolle E, Taucher V, Lindenmann J, Pichler M, Smolle-Juettner FM. Liquid biopsy in non-small cell lung cancer-current status and future outlook-a narrative review. Transl Lung Cancer Res 2021;10:2237-51. [Crossref] [PubMed]
- Woody NM, Stephans KL, Andrews M, Zhuang T, Gopal P, Xia P, Farver CF, Raymond DP, Peacock CD, Cicenia J, Reddy CA, Videtic GM, Abazeed ME. A Histologic Basis for the Efficacy of SBRT to the lung. J Thorac Oncol 2017;12:510-9. [Crossref] [PubMed]
- Scagliotti G, Hanna N, Fossella F, Sugarman K, Blatter J, Peterson P, Simms L, Shepherd FA. The differential efficacy of pemetrexed according to NSCLC histology: a review of two Phase III studies. Oncologist 2009;14:253-63. [Crossref] [PubMed]
- Zhang Z, Zou H, Yuan A, Jiang F, Zhao B, Liu Y, Chen J, Zuo M, Gong L. A single enhanced dual-energy CT scan may distinguish lung squamous cell carcinoma from adenocarcinoma during the venous phase. Acad Radiol 2020;27:624-9. [Crossref] [PubMed]
- Kim JW, Jeong YY, Chang NK, Heo SH, Shin SS, Lee JH, Hur YH, Kang HK. Perfusion CT in colorectal cancer: comparison of perfusion parameters with tumor grade and microvessel density. Korean J Radiol 2012;13:S89-97. [Crossref] [PubMed]
- Morsbach F, Pfammatter T, Reiner CS, Fischer MA, Sah BR, Winklhofer S, Klotz E, Frauenfelder T, Knuth A, Seifert B, Schaefer N, Alkadhi H. Computed tomographic perfusion imaging for the prediction of response and survival to transarterial radioembolization of liver metastases. Invest Radiol 2013;48:787-94. [Crossref] [PubMed]
- Lind JS, Meijerink MR, Dingemans AM, van Kuijk C, Ollers MC, de Ruysscher D, Postmus PE, Smit EF. Dynamic contrast-enhanced CT in patients treated with sorafenib and erlotinib for non-small cell lung cancer: a new method of monitoring treatment? Eur Radiol 2010;20:2890-8. [Crossref] [PubMed]
- Kim SH, Kamaya A, Willmann JK. CT perfusion of the liver: principles and applications in oncology. Radiology 2014;272:322-44. [Crossref] [PubMed]
- Alvarez RE, Macovski A. Energy-selective reconstructions in X-ray computerized tomography. Phys Med Biol 1976;21:733-44. [Crossref] [PubMed]
- Goh KL, Liew SC. Energy-dependent systematic errors in dual-energy X-ray CT. IEEE T Nucl Sci 1997;44:212-7. [Crossref]
- Goh KL, Liew SC, Hasegawa BH. Correction of energy-dependent systematic errors in dual-energy X-ray CT using a basis material coefficients transformation method. IEEE T Nucl Sci 1997;44:2419-24. [Crossref]
- Goh KL, Liew SC. Dual-energy x-ray approach for object/energy-specific attenuation coefficient correction in single-photon emission computed tomography: effects of contrast agent. J Med Imaging (Bellingham) 2021;8:052106. [Crossref] [PubMed]
- Wen Q, Yue Y, Shang J, Lu X, Gao L, Hou Y. The application of dual-layer spectral detector computed tomography in solitary pulmonary nodule identification. Quant Imaging Med Surg 2021;11:521-32. [Crossref] [PubMed]
- Mu R, Meng Z, Guo Z, Qin X, Huang G, Yang X, Jin H, Yang P, Zhang X, Zhu X. Dual-layer spectral detector computed tomography parameters can improve diagnostic efficiency of lung adenocarcinoma grading. Quant Imaging Med Surg 2022;12:4601-11. [Crossref] [PubMed]
- Mulé S, Pigneur F, Quelever R, Tenenhaus A, Baranes L, Richard P, Tacher V, Herin E, Pasquier H, Ronot M, Rahmouni A, Vilgrain V, Luciani A. Can dual-energy CT replace perfusion CT for the functional evaluation of advanced hepatocellular carcinoma? Eur Radiol 2018;28:1977-85. [Crossref] [PubMed]
- Kang HJ, Kim SH, Bae JS, Jeon SK, Han JK. Can quantitative iodine parameters on DECT replace perfusion CT parameters in colorectal cancers? Eur Radiol 2018;28:4775-82. [Crossref] [PubMed]
- Bao J, Liu A, Zhao C, Hao F, Su X, Bao L, Zhao L. Correlation Between Dual-Energy Computed Tomography Single Scan and Computed Tomography Perfusion for Pancreatic Cancer Patients: Initial Experience. J Comput Assist Tomogr 2019;43:599-604. [Crossref] [PubMed]
- Stiller W, Skornitzke S, Fritz F, Klauss M, Hansen J, Pahn G, Grenacher L, Kauczor HU. Correlation of quantitative dual-energy computed tomography iodine maps and abdominal computed tomography perfusion measurements: are single-acquisition dual-energy computed tomography iodine maps more than a reduced-dose surrogate of conventional computed tomography perfusion? Invest Radiol 2015;50:703-8. [Crossref] [PubMed]
- Gordic S, Puippe GD, Krauss B, Klotz E, Desbiolles L, Lesurtel M, Müllhaupt B, Pfammatter T, Alkadhi H. Correlation between Dual-Energy and Perfusion CT in Patients with Hepatocellular Carcinoma. Radiology 2016;280:78-87. [Crossref] [PubMed]
- Lin LY, Zhang Y, Suo ST, Zhang F, Cheng JJ, Wu HW. Correlation between dual-energy spectral CT imaging parameters and pathological grades of non-small cell lung cancer. Clin Radiol 2018;73:412.e1-7. [Crossref] [PubMed]
- Hong SR, Hur J, Moon YW, Han K, Chang S, Kim JY, Im DJ, Suh YJ, Hong YJ, Lee HJ, Kim YJ, Choi BW. Predictive factors for treatment response using dual-energy computed tomography in patients with advanced lung adenocarcinoma. Eur J Radiol 2018;101:118-23. [Crossref] [PubMed]
- Liu L, Zhi X, Liu B, Zhang Y. Utilizing gemstone spectral CT imaging to evaluate the therapeutic efficacy of radiofrequency ablation in lung cancer. Radiol Med 2016;121:261-7. [Crossref] [PubMed]
- Deng L, Zhang G, Lin X, Han T, Zhang B, Jing M, Zhou J. Comparison of Spectral and Perfusion Computed Tomography Imaging in the Differential Diagnosis of Peripheral Lung Cancer and Focal Organizing Pneumonia. Front Oncol 2021;11:690254. [Crossref] [PubMed]
- Tang H, Yu N, Jia Y, Yu Y, Duan H, Han D, Ma G, Ren C, He T. Assessment of noise reduction potential and image quality improvement of a new generation adaptive statistical iterative reconstruction (ASIR-V) in chest CT. Br J Radiol 2018;91:20170521. [Crossref] [PubMed]
- Forghani R, Kelly H, Yu E, Belair M, Létourneau-Guillon L, Le H, Proulx F, Ong T, Tan X, Curtin HD, Levental M. Low-Energy Virtual Monochromatic Dual-Energy Computed Tomography Images for the Evaluation of Head and Neck Squamous Cell Carcinoma: A Study of Tumor Visibility Compared With Single-Energy Computed Tomography and User Acceptance. J Comput Assist Tomogr 2017;41:565-71. [Crossref] [PubMed]
- Vogl TJ, Schulz B, Bauer RW, Stöver T, Sader R, Tawfik AM. Dual-energy CT applications in head and neck imaging. AJR Am J Roentgenol 2012;199:S34-9. [Crossref] [PubMed]
- Schueller-Weidekamm C, Schaefer-Prokop CM, Weber M, Herold CJ, Prokop M. CT angiography of pulmonary arteries to detect pulmonary embolism: improvement of vascular enhancement with low kilovoltage settings. Radiology 2006;241:899-907. [Crossref] [PubMed]
- Yuan R, Shuman WP, Earls JP, Hague CJ, Mumtaz HA, Scott-Moncrieff A, Ellis JD, Mayo JR, Leipsic JA. Reduced iodine load at CT pulmonary angiography with dual-energy monochromatic imaging: comparison with standard CT pulmonary angiography--a prospective randomized trial. Radiology 2012;262:290-7. [Crossref] [PubMed]
- Luo S, Sha Y, Wu J, Lin N, Pan Y, Zhang F, Huang W. Differentiation of malignant from benign orbital tumours using dual-energy CT. Clin Radiol 2022;77:307-13. [Crossref] [PubMed]
- Kraus MS, Selo N, Kiefer LS, Esser M, Albtoush OM, Weiss J, Wichmann JL, Bamberg F, Othman AE. Advanced Virtual Monoenergetic Imaging: Improvement of Visualization and Differentiation of Intramuscular Lesions in Portal-Venous-phase Contrast-enhanced Dual-energy CT. Acad Radiol 2019;26:1457-65. [Crossref] [PubMed]
- Bunch PM, Pavlina AA, Lipford ME, Sachs JR. Dual-energy parathyroid 4D-CT: improved discrimination of parathyroid lesions from thyroid tissue using noncontrast 40-kev virtual monoenergetic images. AJNR Am J Neuroradiol 2021;42:2001-8. [Crossref] [PubMed]
- Lourenco PDM, Rawski R, Mohammed MF, Khosa F, Nicolaou S, McLaughlin P. Dual-energy CT iodine mapping and 40-keV monoenergetic applications in the diagnosis of acute bowel ischemia. AJR Am J Roentgenol 2018;211:564-70. [Crossref] [PubMed]
- Martin SS, Wichmann JL, Pfeifer S, Leithner D, Lenga L, Reynolds MA, D'Angelo T, Hammerstingl R, Gruber-Rouh T, Vogl TJ, Albrecht MH. Impact of noise-optimized virtual monoenergetic dual-energy computed tomography on image quality in patients with renal cell carcinoma. Eur J Radiol 2017;97:1-7. [Crossref] [PubMed]
- Noda Y, Tochigi T, Parakh A, Joseph E, Hahn PF, Kambadakone A. Low keV portal venous phase as a surrogate for pancreatic phase in a pancreatic protocol dual-energy CT: feasibility, image quality, and lesion conspicuity. Eur Radiol 2021;31:6898-908. [Crossref] [PubMed]
- Shen H, Yuan X, Liu D, Huang Y, Wang Y, Jiang S, Zhang J. Multiparametric dual-energy CT for distinguishing nasopharyngeal carcinoma from nasopharyngeal lymphoma. Eur J Radiol 2021;136:109532. [Crossref] [PubMed]
- Yoo J, Lee JM, Yoon JH, Joo I, Lee ES, Jeon SK, Jang S. Comparison of low kVp CT and dual-energy CT for the evaluation of hypervascular hepatocellular carcinoma. Abdom Radiol (NY) 2021;46:3217-26. [Crossref] [PubMed]
- Fuld MK, Halaweish AF, Haynes SE, Divekar AA, Guo J, Hoffman EA. Pulmonary perfused blood volume with dual-energy CT as surrogate for pulmonary perfusion assessed with dynamic multidetector CT. Radiology 2013;267:747-56. [Crossref] [PubMed]
- Jia Y, Xiao X, Sun Q, Jiang H. CT spectral parameters and serum tumour markers to differentiate histological types of cancer histology. Clin Radiol 2018;73:1033-40. [Crossref] [PubMed]
- Shi J, Schmid-Bindert G, Fink C, Sudarski S, Apfaltrer P, Pilz LR, Liu B, Haberland U, Klotz E, Zhou C, Schoenberg SO, Henzler T. Dynamic volume perfusion CT in patients with lung cancer: baseline perfusion characteristics of different histological subtypes. Eur J Radiol 2013;82:e894-900. [Crossref] [PubMed]
- Bevilacqua A, Gavelli G, Baiocco S, Barone D. CT perfusion in patients with lung cancer: squamous cell carcinoma and adenocarcinoma show a different blood flow. Biomed Res Int 2018;2018:6942131. [Crossref] [PubMed]
- Qiao PG, Huang Q, Zhou J, Wang XC, Li M, Ma JL, Tian N, Li GJ. Feasibility of quantitative parameters of dynamically enhanced patterns of spiral computed tomography scanning integrated into tumour progression before targeted treatment of non-small cell lung cancer. J Med Imaging Radiat Oncol 2015;59:216-20. [Crossref] [PubMed]
- Yabuuchi H, Kawanami S, Iwama E, Okamoto I, Kamitani T, Sagiyama K, Yamasaki Y, Honda H. Prediction of Therapeutic Effect of Chemotherapy for NSCLC Using Dual-Input Perfusion CT Analysis: Comparison among Bevacizumab Treatment, Two-Agent Platinum-based Therapy without Bevacizumab, and Other Non-Bevacizumab Treatment Groups. Radiology 2018;286:685-95. [Crossref] [PubMed]
- Bevilacqua A, Barone D, Malavasi S, Gavelli G. Quantitative assessment of effects of motion compensation for liver and lung tumors in CT perfusion. Acad Radiol 2014;21:1416-26. [Crossref] [PubMed]