Harnessing dual-energy CT for glycogen quantification: a phantom analysis
Introduction
Glycogen storage disorders (GSD) are inherited metabolic disorders of glycogen metabolism linked to liver and muscle disorders (1). In GSD types 3 and 4, the degree of glycogen accumulation varies as the disease progresses, ultimately resulting in hepatosplenomegaly, liver cirrhosis, and liver failure (2). In this regard, the quantification of glycogen could provide essential biological insights. Correspondingly, the development of effective quantitative approaches is highly anticipated.
Previous studies have described non-invasive techniques for determining and assessing glycogen contents in vivo by using fluorescent glucosamine derivative probes. However, due to the limited tissue penetration ability of fluorescence, it could neither be used to directly annotate glycogen nor in clinical practice (3-6). In addition, positron emission tomography is costly, and the use of fluorescent glucosamine derivative probes requires the application of radioactive tracers, thus, restricting its applications beyond clinical research.
Computed tomography (CT) has evolved from a single-parameter imaging to a multi-parameter imaging modality with the advent of the energy spectrum technique in recent years. The attenuation of X-ray photons in diagnostic imaging is primarily caused by the photoelectric effect and Compton scattering. Photoelectric effect varies considerably based on the photon energy and the unique binding energy of the electrons in an atom. When low-energy X-rays interact with a material with a high effective atomic number, the photoelectric effect is primarily produced. In contrast, Compton scattering is proportional to the effective atomic number but is less frequently associated with photon energy (7-9). Through continuous energy spectrum acquisition by dual-energy computed tomography (DECT), this principle can be utilized to evaluate material-specific attenuation behavior, which is characterized by disparate attenuation at different energy levels, and to obtain material-specific images (10,11). Consequently, the DECT enables differentiation, classification, and quantification of the mass density of various materials (12-14). Accordingly, iodine-specific images can represent iodine content in the tissues and provide quantitative enhancement information, which is beneficial for making the transition from qualitative to quantitative evaluation of contrast-enhanced CT images (12). However, there have been relatively few attempts to apply this technique to the determination of glycogen. The objective of the present study was to evaluate the viability of quantifying glycogen content using DECT. On the basis of unique k-edge characteristics and differences in attenuation at different energy levels between iodine and glycogen, phantoms with inserts containing different proportions of a mixture of glycogen and iodine were scanned. In addition, the limit of detection (LOD) and the limit of quantification (LOQ) of glycogen quantification were investigated.
Methods
Phantom configuration
A cylindrical solid plastic phantom (diameter: 20 cm) with nine inserted removable cylindrical polyvinyl chloride plastic tubes was utilized (Figure 1A). Each tube had a diameter of 25 mm, a height of 96 mm, and a volume of 20 mL. Glycogen-iodine mixture samples were prepared as follows: 33 samples were prepared from three groups (Groups A, B, and C) of homogeneous glycogen (BR, 99% extracted from mussel, Source Leaf Creatures)-water mixed samples with a glycogen concentration gradient of 0, 10, 20, 30, 40, 50, 60, 70, 80, 90, and 100 mg/mL (corresponding glycogen weight-to-water weight ratio: 0%, 1%, 2%, 3%, 4%, 5%, 6%, 7%, 8%, 9%, 10%) (15). The glycogen concentration was configured based on the in vivo hepatic glycogen content, which could reach 10% under certain pathological conditions (16,17). Iopromide (Ultravist370, Bayer) was added to groups A, B, and C with volumes of 20, 30, and 40 µL, respectively, to simulate the in vivo iodine concentration during contrast-enhanced CT imaging, resulting in iodine concentrations of 0.37, 0.56, and 0.74 mg/mL, respectively (Table 1).
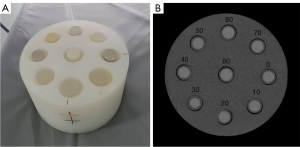
Table 1
NGC (mg/mL) | Glycogen weight-to-water weight ratio (%) | IC (mg/mL) | ||
---|---|---|---|---|
Group A (Nos. 1–11) | Group B (Nos. 12–22) | Group C (Nos. 23–33) | ||
0 | 0 | 0.37 | 0.56 | 0.74 |
10 | 1 | 0.37 | 0.56 | 0.74 |
20 | 2 | 0.37 | 0.56 | 0.74 |
30 | 3 | 0.37 | 0.56 | 0.74 |
40 | 4 | 0.37 | 0.56 | 0.74 |
50 | 5 | 0.37 | 0.56 | 0.74 |
60 | 6 | 0.37 | 0.56 | 0.74 |
70 | 7 | 0.37 | 0.56 | 0.74 |
80 | 8 | 0.37 | 0.56 | 0.74 |
90 | 9 | 0.37 | 0.56 | 0.74 |
100 | 10 | 0.37 | 0.56 | 0.74 |
Glycogen weight-to-water weight ratio: glycogen weight (g)/water weight (g)*100%. Groups A, B, and C refer to iodine concentrations of 0.37, 0.56, and 0.74 mg/mL, respectively. IC, iodine concentration; NGC, nominal glycogen concentration.
CT scanning protocols
The phantom was scanned on a 256-slice CT scanner (Revolution CT, GE Healthcare) using the helical spectral DECT imaging mode with fast-kilovolt-peak switching between 80 and 140 kVp. The scanning parameters were as follows: gantry rotation speed: 0.5 s; pitch: 0.984; display field of view: 250 mm; imaging matrix: 512×512. The X-ray tube currents were 200 mA, 335 mA, and 480 mA (18). To improve reproducibility, the scans were performed three times. Images were reconstructed with adaptive statistical iterative reconstruction -Veo at 50% strength (50% ASiR-V) and the standard reconstruction kernel (Stnd). The reconstruction layer thickness, as well as the layer spacing, was 1.25 mm.
Data analysis
All DECT data files were transferred to a local advantage workstation (AW4.7, Volume Share 5; GE Healthcare) for post-processing analysis using gemstone spectral imaging (GSI) software (version 2.20-05) and the material decomposition (MD) algorithm (GE Healthcare) (19). The glycogen concentration was determined by the MD algorithm using a two-material decomposition with glucose and iodine as the basis material pair. A circular region of interest (ROI) with a diameter of 10 mm was used to measure the virtual glycogen concentration (VGC) in mg/mL on the cross-sectional glucose-specific images across five slices (Figure 1B). The interval of each slice was 5 mm. In addition, the single-source spectral CT mode generates a series of monochromatic images with energies ranging from 40 to 140 keV. In GSI mode, the average energy of 120 kVp scanning for conventional polychromatic images was 70 keV (20). As a result, the 70 keV monochromatic images were selected to simulate conventional polychromatic images, and CT numbers were recorded in Hounsfield units (HU). To measure CT numbers, the GSI software automatically propagated ROIs to 70 keV monochromatic images. Subsequently, the VGC and CT numbers were averaged across 15 ROIs from three scans.
Statistics analysis
The statistical analyses were performed using SPSS 26.0 software. A P value less than 0.05 indicated statistical significance.
The correlation between the CT numbers on the 70 keV monochromatic images and the known nominal glycogen concentration (NGC) was evaluated using least-square linear regression. Similarly, the same methodology was used to construct the linear calibration curve and evaluate correlations between VGC and NGC. In addition, the coefficient of determination (R2) and residual root-mean-square error (RMSE) were calculated to evaluate the goodness of the linear fits. The intercept of the calibration curve (Yint) was set to be zero because the VGC was expected to be proportional to the NGC, and the inverse of the curve slope (Cslope) was determined as the conversion factor from VGC to NGC (21). Additionally, covariance analysis was used to evaluate the influence of iodine concentration and tube current on the Cslope.
Furthermore, the sensitivity of the measurement was represented by the LOD and LOQ. The LOD was calculated according to the Clinical and Laboratory Standards Institute (22,23). First, the limit of blank (LOB) signal was defined as the maximum expected signal level (measured in HU or mg/mL) of background (the glycogen-free solution) and calculated using Eq. [1]:
where, denotes the mean signal of the background, the represents the standard deviation of the measurements. The multiplicative factor of 1.645 is consistent with a Gaussian distribution with a 95% confidence interval (CI). The LOD signal was subsequently computed using Eq. [2]:
This algorithm required the standard deviation of a low-concentration (low conc) sample, which was set to 10 mg/mL because the signal was higher than that of the LOB under all conditions (22). Eq. [3] was then used to convert the signal to a concentration using the equation for the regression curve (22):
In addition, the LOQ was established as the lowest glycogen concentration at which the coefficient of variation was 20% or less (21).
The inter-class correlation coefficient (ICC) was used to assess the scan-repeat scan (scan-rescan) agreement of measured VGC. The ICC was interpreted as follows: poor agreement (<0.4), good agreement (0.4–0.75), and excellent agreement (≥0.75) (24). Finally, the scan-rescan variability was assessed using the coefficient of variation (CoV), which was determined as the proportion of the standard deviation of the mean (expressed as percentage). The CoV of each sample was calculated and then averaged to determine the mean CoV among samples, defined as the CoV of the scan-rescan.
Results
CT number on 70 keV monochromatic images was correlated to NGC (P<0.001). However, the linear curve fit was relatively poor (R2: 0.867–0.932; Figure 2). In contrast, excellent linear relationships were found between nominal and virtual glycogen concentrations (P<0.001, R2: 0.989–0.997; RMSE: 1.862–3.267 mg/mL), and linear calibration curves with an intercept of zero were obtained, confirming the proportionality between the two quantities (Figure 3). The fitted linear correlation equations are listed in Table 2. Additionally, the estimated conversion factor from VGC to NGC was 3.068–3.222 (Table 3). The covariance analysis revealed that the Cslope at various tube currents did not differ significantly (P=0.959, 0.458, and 0.821), and the regression lines were nearly parallel (Figure 4A-4C). Similarly, there were no significant differences in the Cslope at different iodine concentrations (P=0.997, 0.904, and 0.755), and the regression curves were nearly coincident (Figure 4D-4F). The findings revealed that the tube currents, as well as the iodine contents, did not impact the Cslope or the conversion factor. In addition, the LOD varied from 6.421 to 15.315 mg/mL, whereas the LOQ ranged from 10.95 to 16.46 mg/mL (Table 2). Furthermore, ICC (range: 0.977–0.991) and CoV (range: 0.1–0.2%) suggested an excellently reproducible measurement of VGC by means of DECT (Table 4).
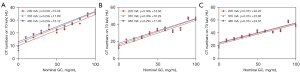
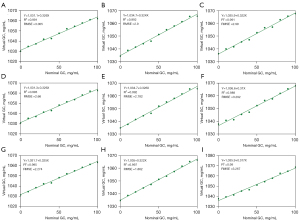
Table 2
Group | Tube current (mA) | Curve slope | Y-intercept (mg/mL) | R2 | RMSE (mg/mL) | P | LOD (mg/mL) | LOQ (mg/mL) |
---|---|---|---|---|---|---|---|---|
A | 200 | 0.326 | 1,031.1 | 0.991 | 3.005 | * | 8.265 | 15.025 |
335 | 0.324 | 1,034.7 | 0.992 | 2.9 | * | 6.421 | 14.502 | |
480 | 0.322 | 1,035.9 | 0.991 | 2.99 | * | 8.817 | 14.949 | |
B | 200 | 0.326 | 1,031.3 | 0.993 | 2.66 | * | 9.763 | 13.3 |
335 | 0.326 | 1,034.7 | 0.992 | 2.782 | * | 11.8 | 13.908 | |
480 | 0.31 | 1,036.6 | 0.989 | 3.292 | * | 12.613 | 16.46 | |
C | 200 | 0.325 | 1,031.7 | 0.995 | 2.274 | * | 15.315 | 15.315 |
335 | 0.322 | 1,035 | 0.997 | 1.862 | * | 10.95 | 10.95 | |
480 | 0.317 | 1,035.9 | 0.99 | 3.267 | * | 13.697 | 16.334 |
*, P<0.001. Groups A, B, and C refer to iodine concentrations of 0.37, 0.56, and 0.74 mg/mL, respectively. LOD, limit of detection; LOQ, limit of quantification; R2, coefficient of determination; RMSE, residual root-mean-square error.
Table 3
Group | Tube current (200 mA) | Tube current (335 mA) | Tube current (480 mA) |
---|---|---|---|
A | 3.070 | 3.086 | 3.109 |
B | 3.068 | 3.072 | 3.222 |
C | 3.077 | 3.105 | 3.151 |
Groups A, B, and C refer to iodine concentrations of 0.37, 0.56, and 0.74 mg/mL, respectively.
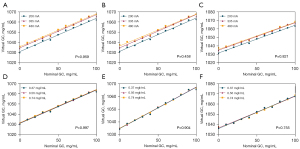
Table 4
Group | Tube current | ICC (95% CI) | CoV |
---|---|---|---|
A | 200 mA | 0.977 (0.964, 0.985)* | 0.20% |
335 mA | 0.987 (0.980, 0.992)* | 0.10% | |
480 mA | 0.980 (0.969, 0.988)* | 0.10% | |
B | 200 mA | 0.987 (0.979, 0.992)* | 0.10% |
335 mA | 0.990 (0.985, 0.994)* | 0.10% | |
480 mA | 0.988 (0.982, 0.993)* | 0.10% | |
C | 200 mA | 0.990 (0.984, 0.994)* | 0.10% |
335 mA | 0.990 (0.984, 0.994)* | 0.10% | |
480 mA | 0.991 (0.987, 0.995)* | 0.10% |
*, P<0.001. Groups A, B, and C refer to iodine concentrations of 0.37, 0.56, and 0.74 mg/mL, respectively. CoV, coefficient of variation; ICC, inter-class correlation coefficient; CI, confidence interval.
Discussion
Although it is difficult to achieve in vivo, the determination of glycogen is valuable and meaningful for providing biological information. In this phantom study, the feasibility and applicability of quantifying glycogen content using DECT and the MD algorithm were evaluated. Accordingly, linear relationships between nominal and virtual glycogen contents were discovered. In addition, the method demonstrated exceptional stability, repeatability, variability, and sensitivity in glycogen quantification.
Traditional hepatic CT images characterize GSD as a decrease in image density or CT values; however, there are substantial overlaps of CT values between different degrees of GSD. In this study, despite the fact that the CT numbers increased as the NGC increased, the curve fitting was found poor. In this regard, beam hardening artefacts, which are reduced by the pseudo-monochromatic images produced by GSI, could be a contributing factor (25). Notably, the current data demonstrated excellent linear relationships between VGC and NGC (R2: 0.989–0.997; RMSE in glycogen: 1.862–3.267 mg/mL). In addition, the scatter plot of the distribution of glycogen density in the test tube with a high glycogen concentration (10%) and a low glycogen concentration (0%) did not intersect at the same iodine concentration, indicating a significant differentiation in glycogen content (Figure 5). Moreover, the MD algorithm had the benefit of directly measuring glycogen content in mg/mL, which was an advantage over CT attenuation. With the introduction of glycogen-specific material density measurement, our findings may help overcome the ambiguity inherent to CT value alone and allow for a quantitative rather than qualitative diagnosis. Thus, this method is likely to be used to determine the extent of liver glycogen accumulation in GSD in future studies.
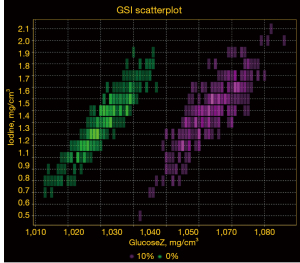
Using a small-sized phantom, fast-kilovolt-peak switching DECT imaging, and the MD algorithm, in vitro experimental study was conducted to examine the impact of fat content on iron quantification in the liver at tube currents of 200, 320, and 485 mA, respectively (18). Another previous report declared that the lower limit of iodine quantification is radiation-dose dependent (26). In addition, the clinical examination scan operator had access to varying doses and contrast dosages. In consideration of the aforementioned variables, the phantoms were prepared with differing amounts of iodine and scanned at various radiation dose levels. Consequently, the Cslopewas unaffected by tube currents and iodine concentrations. Accordingly, the stability of this approach was demonstrated, making it suitable for a variety of clinical applications.
In addition, LOD and LOQ are important measurement method parameters. In all conditions, our findings revealed that the LODs (0.6–1.5%) and LOQs (1.1–1.7%) were relatively stable. Moreover, the limits of quantification for glycogen obtained via DECT were sufficient for identifying pathological glycogen storage (3–10%). It indicated the potential of direct imaging of liver glycogen accumulation, particularly in GSD. It would be possible to image glycogen directly in tumors and bloods if this technology is further refined to achieve a lower limit of quantification. In addition, the evaluation of quantitative limitations could provide experimental references for future research.
Due to the chemical instability of glycogen, we chose glucose over glycogen as the basis material in this study (27). Notably, the MD algorithm could inspect the content directly in percentages. Theoretically, the use of glucose as base material has no effect on the measured VGC. Although the estimation of glycogen may be affected by its by-product, such as glucose, this effect is minimal in the liver. Moreover, this study introduced glycose and iodine as a material pair, exploring new avenues for exploiting the features of the material composition algorithm and expanding the clinical applications of this technique. However, the method for quantifying glycogen would be applied to enhanced images. Using the base materials in the body, such as iron, on non-enhanced images, additional experiments are required to determine the glycogen content.
Nevertheless, as demonstrated in Table 3, there are still minor variations in conversion factors under various conditions. Additionally, inter-scanner variation would likely amplify the uncertainty in the conversion factors and worsen the LOQ (21,22). In this regard, the use of a single scanner is a limitation of the present study. Accordingly, future research will require other scanners with distinct GSI modes and material quantification algorithms (28) in order to confirm the applicability and accuracy of the current analytical results. Therefore, to ensure reliable glycogen quantification, it is imperative to ensure accurate calibration of the conversion factor and LOQ. Always scanning a calibration phantom with the patient according to different conditions (such as patient size, iodine injection dose, tube current, GSI mode, and use of a material pair) would be one approach that could potentially meet these requirements.
In addition, the results demonstrated an excellent scan-rescan agreement and minimal variability of VGC, with coefficients of variation below 1% and ICC values close to 1. Overall, the method demonstrated excellent repeatability/reproducibility and stability.
The study also comprises several limitations. Initially, the study provided a relative estimation of glycogen concentration. However, to improve the technique for absolute measurement, additional research is required. Secondly, in this phantom study, the mixture to be analyzed consists of only glycogen, water, and iopromide, whereas the human organ is a more complex system. To test the accuracy of DECT for glycogen quantification, it is, therefore, necessary to compare theoretical results with pathological results, particularly in GSD patients. Moreover, the experimental design of glycogen concentration was based on an arithmetic principle. The current findings, therefore, must be confirmed in experiments employing geometric design with a lower glycogen concentration.
Conclusions
Our pilot phantom analysis demonstrated the feasibility and efficacy of detecting and quantifying glycogen using DECT and showed good quantitative performance with excellent stability and reproducibility/variability. Thus, in the future, DECT could be utilized as a convenient method for glycogen quantification to provide more reliable data for clinical decision-making.
Acknowledgments
The authors thank GE Healthcare for technical support.
Funding: This study received funding by the National Natural Science Foundation of China (Nos. 81971684 and 81771908).
Footnote
Conflicts of Interest: All authors have completed the ICMJE uniform disclosure form (available at https://qims.amegroups.com/article/view/10.21037/qims-22-1234/coif). The authors have no conflicts of interest to declare.
Ethical Statement: The authors are accountable for all aspects of the work in ensuring that questions related to the accuracy or integrity of any part of the work are appropriately investigated and resolved. This work did not involve any humans or animals, so ethical approval and informed consent were not required.
Open Access Statement: This is an Open Access article distributed in accordance with the Creative Commons Attribution-NonCommercial-NoDerivs 4.0 International License (CC BY-NC-ND 4.0), which permits the non-commercial replication and distribution of the article with the strict proviso that no changes or edits are made and the original work is properly cited (including links to both the formal publication through the relevant DOI and the license). See: https://creativecommons.org/licenses/by-nc-nd/4.0/.
References
- Ellingwood SS, Cheng A. Biochemical and clinical aspects of glycogen storage diseases. J Endocrinol 2018;238:R131-41. [Crossref] [PubMed]
- Kanungo S, Wells K, Tribett T, El-Gharbawy A. Glycogen metabolism and glycogen storage disorders. Ann Transl Med 2018;6:474. [Crossref] [PubMed]
- Louzao MC, Espiña B, Vieytes MR, Vega FV, Rubiolo JA, Baba O, Terashima T, Botana LM. "Fluorescent glycogen" formation with sensibility for in vivo and in vitro detection. Glycoconj J 2008;25:503-10. [Crossref] [PubMed]
- Zhao Q, Zhou J, Pan Y, Ju H, Zhu L, Liu Y, Zhang Y. The difference between steroid diabetes mellitus and type 2 diabetes mellitus: a whole-body (18)F-FDG PET/CT study. Acta Diabetol 2020;57:1383-93. [Crossref] [PubMed]
- Cole EL, Shao X, Sherman P, Quesada C, Fawaz MV, Desmond TJ, Scott PJ. Synthesis and evaluation of [(11)C]PyrATP-1, a novel radiotracer for PET imaging of glycogen synthase kinase-3beta (GSK-3beta). Nucl Med Biol 2014;41:507-12. [Crossref] [PubMed]
- Witney TH, Carroll L, Alam IS, Chandrashekran A, Nguyen QD, Sala R, Harris R, DeBerardinis RJ, Agarwal R, Aboagye EO. A novel radiotracer to image glycogen metabolism in tumors by positron emission tomography. Cancer Res 2014;74:1319-28. [Crossref] [PubMed]
- Kruger RA, Riederer SJ, Mistretta CA. Relative properties of tomography, K-edge imaging, and K-edge tomography. Med Phys 1977;4:244-9. [Crossref] [PubMed]
- Riederer SJ, Mistretta CA. Selective iodine imaging using K-edge energies in computerized x-ray tomography. Med Phys 1977;4:474-81. [Crossref] [PubMed]
- Rutherford RA, Pullan BR, Isherwood I. X-ray energies for effective atomic number determination. Neuroradiology 1976;11:23-8. [Crossref] [PubMed]
- Kalender WA, Perman WH, Vetter JR, Klotz E. Evaluation of a prototype dual-energy computed tomographic apparatus. I. Phantom studies. Med Phys 1986;13:334-9. [Crossref] [PubMed]
- Alvarez RE, Macovski A. Energy-selective reconstructions in X-ray computerized tomography. Phys Med Biol 1976;21:733-44. [Crossref] [PubMed]
- McCollough CH, Leng S, Yu L, Fletcher JG. Dual- and Multi-Energy CT: Principles, Technical Approaches, and Clinical Applications. Radiology 2015;276:637-53. [Crossref] [PubMed]
- Ye H, Li X, Yao N, Shi Y, Wang Y, Yu W. Effect of abdominal adipose content on spine phantom bone mineral density measured by rapid kilovoltage-switching dual-energy CT and quantitative CT. Quant Imaging Med Surg 2022;12:4914-23. [Crossref] [PubMed]
- Wang M, Wu Y, Zhou Y, Dong J, Hou P, Gao J. The new fast kilovoltage-switching dual-energy computed tomography for measuring bone mineral density. Quant Imaging Med Surg 2023;13:801-11. [Crossref] [PubMed]
- Li X, Chen XX, Xu Y, Xu XB, Wu WF, Zhao Q, Hu JN. Construction of Glycogen-Based Nanoparticles Loaded with Resveratrol for the Alleviation of High-Fat Diet-Induced Nonalcoholic Fatty Liver Disease. Biomacromolecules 2022;23:409-23. [Crossref] [PubMed]
- den Otter W, van Boxtel AB. Relation between the glycogen content of the liver and liver weight, and its meaning for enzymology. Experientia 1971;27:1271-2. [Crossref] [PubMed]
- Bonjorn VM, Latour MG, Bélanger P, Lavoie JM. Influence of prior exercise and liver glycogen content on the sensitivity of the liver to glucagon. J Appl Physiol (1985) 2002;92:188-94. [PubMed]
- Xie T, Li Y, He G, Zhang Z, Shi Q, Cheng G. The influence of liver fat deposition on the quantification of the liver-iron fraction using fast-kilovolt-peak switching dual-energy CT imaging and material decomposition technique: an in vitro experimental study. Quant Imaging Med Surg 2019;9:654-61. [Crossref] [PubMed]
- Brooks RA. A quantitative theory of the Hounsfield unit and its application to dual energy scanning. J Comput Assist Tomogr 1977;1:487-93. [Crossref] [PubMed]
- Matsumoto K, Jinzaki M, Tanami Y, Ueno A, Yamada M, Kuribayashi S. Virtual monochromatic spectral imaging with fast kilovoltage switching: improved image quality as compared with that obtained with conventional 120-kVp CT. Radiology 2011;259:257-62. [Crossref] [PubMed]
- Jiang X, Hintenlang DE, White RD. Lower limit of iron quantification using dual-energy CT - a phantom study. J Appl Clin Med Phys 2021;22:299-307. [Crossref] [PubMed]
- Jacobsen MC, Cressman ENK, Tamm EP, Baluya DL, Duan X, Cody DD, Schellingerhout D, Layman RR, Dual-Energy CT. Lower Limits of Iodine Detection and Quantification. Radiology 2019;292:414-9. [Crossref] [PubMed]
- Ceriotti F, Zakowski J, Sine H, Altaie S, Horowitz G, Pesce AJ, Boyd J, Horn P, Gard U, Horowitz G. Clinical and Laboratory Standards Institute (CLSI). 2012.
- Yiannakas MC, Schneider T, Yoneyama M, Aforlabi-Logoh I, Prados F, Ciccarelli O, Wheeler-Kingshott CAM. Magnetisation transfer ratio combined with magnetic resonance neurography is feasible in the proximal lumbar plexus using healthy volunteers at 3T. Sci Rep 2020;10:14568. [Crossref] [PubMed]
- Fang T, Deng W, Law MW, Luo L, Zheng L, Guo Y, Chen H, Huang B. Comparison of image quality and radiation exposure between conventional imaging and gemstone spectral imaging in abdominal CT examination. Br J Radiol 2018;91:20170448. [Crossref] [PubMed]
- Li B, Pomerleau M, Gupta A, Soto JA, Anderson SW. Accuracy of Dual-Energy CT Virtual Unenhanced and Material-Specific Images: A Phantom Study. AJR Am J Roentgenol 2020;215:1146-54. [Crossref] [PubMed]
- Meléndez R, Meléndez-Hevia E, Cascante M. How did glycogen structure evolve to satisfy the requirement for rapid mobilization of glucose? A problem of physical constraints in structure building. J Mol Evol 1997;45:446-55. [Crossref] [PubMed]
- Goodsitt MM, Shenoy A, Shen J, Howard D, Schipper MJ, Wilderman S, Christodoulou E, Chun SY, Dewaraja YK. Evaluation of dual energy quantitative CT for determining the spatial distributions of red marrow and bone for dosimetry in internal emitter radiation therapy. Med Phys 2014;41:051901. [Crossref] [PubMed]