Native T1 mapping for non-invasive quantitative evaluation of renal function and renal fibrosis in patients with chronic kidney disease
Introduction
Chronic kidney disease (CKD) has a high prevalence, poor prognosis and incurs high medical costs. CKD is defined as abnormalities of the kidney structure or function that persist for at least three months. This condition has many health implications for individuals. CKD affects nearly 10% of the population worldwide and is often underrecognized by patients and clinicians (1,2). An accurate assessment of renal function is crucial for monitoring disease progression, treatment response and prognosis management for CKD patients. The most commonly used method to assess renal function is the evaluation of the estimated glomerular filtration rate (eGFR), which is calculated using the CKD epidemiology collaboration (CKD-EPI) equation (3). However, studies have shown that CKD-EPI-based eGFR measurement is not accurate for evaluating renal function in CKD patients mainly due to the large variability caused by the formula only based on serum creatinine (Scr) (4). Besides, the eGFR cannot accurately estimate split kidney function, which is often required for the evaluation of patients with CKD (5).
In addition, renal fibrosis (RF) inevitably occurs during the progression of CKD (6). RF, pathologically characterized by glomerulosclerosis and renal interstitial fibrosis, is an important factor leading to renal structural changes and loss of function. The degree of RF is strongly correlated with the CKD prognosis (7,8). Renal biopsy is the current gold standard for diagnosing RF. Unfortunately, invasive renal biopsy has a considerable risk of serious complications (e.g., gross hematuria, perirenal hematoma, arteriovenous fistula, etc.) (9). Hence, a non-invasive method of monitoring renal function and assessing RF would be a valuable tool to diagnose CKD and guide antifibrotic therapy.
Magnetic resonance imaging (MRI) is a non-invasive examination technology and has been widely used for the whole body because it has high contrast in soft tissues and provides radiation-free, multiplanar and multisequence imaging. However, conventional MRI sequences cannot be used to diagnose renal fibrotic diseases. Although gadolinium-based contrast-enhanced MRI sequences can provide fibrotic imaging of the heart, liver and other organs, gadolinium-based contrast agents are associated with RF and potentially nephrotoxicity, which increases the risk of renal insufficiency (10). In recent years, the application of functional MRI has made it possible to visualize complicated pathophysiological changes in the kidney without the use of contrast media. At present, renal functional MRI techniques mainly include diffusion-weighted imaging (DWI), magnetic resonance elastography (MRE), blood oxygenation level-dependent MRI (BOLD-MRI), and arterial spin labeling (ASL). These modalities can reveal characteristics of renal microvascular perfusion, oxygenation, interstitial diffusion and renal stiffness (11-15). Compared with these functional MRI techniques, relatively few studies on renal diseases have used MRI mapping techniques, which comprise the generation of a parametric map from a series of co-registered images with different T1, T2 or T2* relaxation times (16). Unlike normal T1-, T2-, and T2*-weighted images, parametric mapping allows for the quantification and visualization of focal or diffuse diseases. T1 mapping depicts the spin-lattice (longitudinal) relaxation in tissues and the T1 value (ms) of a voxel represents a time constant for recovery, which has been widely applied to quantitatively evaluate myocardial and articular cartilage lesions and iron overload (17). Furthermore, T1 mapping without the administration of a contrast agent, referred to as native T1 mapping, is highly reproducible and sensitive, especially for patients with renal insufficiency or who are allergic to contrast agents (18-20).
The aim of this study was to utilize native T1 mapping to non-invasively monitor renal function and quantitatively assess RF in patients with CKD. We present this article in accordance with the STARD reporting checklist (available at https://qims.amegroups.com/article/view/10.21037/qims-22-1304/rc).
Methods
Subjects
The study was conducted in accordance with the Declaration of Helsinki (as revised in 2013). This prospective, single-center study was approved by institutional ethics committee of our hospital (No. JD-LK-2022-060-01) and informed consent was taken from all the patients. The CKD group comprised 82 patients who were clinically diagnosed with CKD in the Nephrology Department of our tertiary care institution between October 2021 and September 2022 and were prepared for renal biopsy and agreed to undergo prebiopsy MRI examination. Forty healthy volunteers (HVs) with no history of kidney disease, hypertension, diabetes or vascular diseases and had an eGFR ≥90 mL/min/1.73 m2, a Scr and blood urea nitrogen (BUN) level within normal limits, and no proteinuria within 1 year were recruited as the control group. The inclusion criteria for CKD patients were as follows: conformity with a clinical diagnosis of CKD, including a history of proteinuria and/or glomerular hematuria over three months and/or decreased eGRF levels and abnormal kidney-related hematological and biochemical parameters. The MRI examination was performed within 3 days before renal biopsy. After biopsy, the sample underwent histopathological examination. There were no contraindications for MRI. There were no serious diseases of other organs or systems. Participants were excluded from this study for the following conditions: inability to complete the MRI examination due to claustrophobia (n=3), renal biopsy not finally performed (n=4), and no satisfactory MRI images were available for analysis (n=4). A total of 71 consecutive CKD patients were finally enrolled in this study. A flowchart with the exclusion criteria is shown in Figure 1.
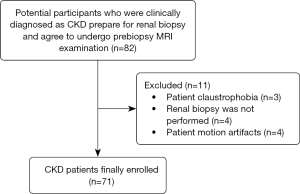
Clinical parameter measurement
Baseline characteristics, including age, gender, height, weight, body mass index (BMI), blood pressure, and blood glucose were collected for all participants. Hematological and biochemical parameters related to the kidney [Scr, BUN and 24-hour urinary protein (24h-UPRO)] were also collected. No specific 24h-UPRO value could be measured in the HV group due to the lack of proteinuria. The eGFR level was calculated according to the CKD-EPI formula. CKD stages were determined according to the Kidney Disease Outcomes Quality Initiative (K/DOQI) guidelines (1). The clinical and laboratory parameters in the two groups are shown in Table 1.
Table 1
Characteristics | CKD (n=71) | HVs (n=40) | Statistics | P |
---|---|---|---|---|
Age (years) | 46 [34, 53] | 41 [34, 49] | −0.990 | 0.322 |
Gender (male) | 33 (46.5) | 21 (52.5) | 0.371 | 0.542 |
Height (m) | 1.65±0.09 | 1.70 [1.63, 1.73] | −1.550 | 0.121 |
Weight (kg) | 65 [56, 77] | 66±12 | −0.240 | 0.811 |
BMI (kg/m2) | 24.18±3.52 | 22.65 [20.54, 24.57] | −1.849 | 0.064 |
Blood pressure (hypertensiona) | 42 (59.2) | 0 (0.0) | 38.065 | <0.001* |
Blood glucose (mmol/L) | 4.82 [4.55, 5.23] | 4.90 [4.38, 5.10] | −0.522 | 0.602 |
eGFR (mL/min/1.73 m2) | 87.61±30.47 | 108.70±9.97 | 5.345 | <0.001* |
Scr (µmol/L) | 76 [57, 109] | 66.5 [55, 79] | −2.411 | 0.016* |
BUN (mmol/L) | 5.5 [4.2, 6.95] | 4.54±0.97 | −3.128 | 0.002* |
24h-UPRO (g/24 h) | 2.12 [0.99, 4.01] | N.A. | N.A. | N.A. |
CKD stage | ||||
1 (eGFR ≥90) | 36 | N.A. | N.A. | N.A. |
2 (eGFR 60–89) | 19 | N.A. | N.A. | N.A. |
3 (eGFR 30–59) | 14 | N.A. | N.A. | N.A. |
4 (eGFR 15–29) | 2 | N.A. | N.A. | N.A. |
5 (eGFR <15) | 0 | N.A. | N.A. | N.A. |
RF stage | ||||
No (0%) | 9 | N.A. | N.A. | N.A. |
Mild (<25%) | 36 | N.A. | N.A. | N.A. |
Moderate (25–50%) | 17 | N.A. | N.A. | N.A. |
Severe (>50%) | 9 | N.A. | N.A. | N.A. |
Pathological type of CKD | ||||
IgA nephropathy | 24 | N.A. | N.A. | N.A. |
Membranous nephropathy | 24 | N.A. | N.A. | N.A. |
Minimal change nephropathy | 7 | N.A. | N.A. | N.A. |
Focal segmental glomerulosclerosis | 4 | N.A. | N.A. | N.A. |
Hypertensive nephropathy | 4 | N.A. | N.A. | N.A. |
Lupus nephritis | 3 | N.A. | N.A. | N.A. |
Diabetic nephropathy | 2 | N.A. | N.A. | N.A. |
Hepatitis B virus-related nephropathy | 1 | N.A. | N.A. | N.A. |
Amyloid Nephropathy | 1 | N.A. | N.A. | N.A. |
Glomerular podocytes | 1 | N.A. | N.A. | N.A. |
Data are presented as mean ± standard deviation, median [interquartile range] or number (%). *, statistically significant; a, hypertension was defined as systolic/diastolic blood pressure ≥140/90 mmHg. CKD, chronic kidney disease; HVs, healthy volunteers; BMI, body mass index; eGFR, estimated glomerular filtration rate; Scr, serum creatinine; BUN, blood urea nitrogen; 24h-UPRO, 24-hour urinary protein; N.A., not applicable.
MRI acquisition
MRI of both kidneys was performed with a Prisma 3.0 T scanner (Simens AG, Erlangen, Germany) using an 18-channel total imaging matrix body coil. An identical protocol was used for both the CKD patients and HVs. Native T1 mapping was performed using an end-expiratory, electrocardiographically (ECG)-gated modified Look-Locker inversion recovery (MOLLI) sequence, and breath-holds were used for respiratory motion compensation. MOLLI images of both kidneys were acquired in the coronal plane. The native T1 mapping MRI protocol parameters were as follows: repetition time (TR), 546.6 ms; echo time (TE), 1.1 ms; slice thickness, 5 mm; slice gap, 1 mm; number of slices, 5; field of view, 144 mm × 144 mm; matrix, 290 mm × 290 mm; flip angle, 35°; spatial resolution, 2×2×5 mm3; initial inversion time (TI), 284 ms; TI increment, 80 ms; 10-second breath holds per time. The total time of the sequence was approximately 2 min 47 s.
Image analysis
The reconstruction software (Syngo.via) in the Siemens postprocessing workstation automatically produced a coronary T1 parametric map with pixel-by-pixel computation of the T1 values. The region of interest (ROI) was delineated on the T1 mapping coronary pseudocolor images by two radiologists with similar experience in the urogenital system (reader 1 had 8 years of experience; reader 2 had 10 years of experience) using a double-blind method. For each radiologist, three ROIs were manually drawn at the same symmetrical location of the left and right kidneys, including the superior pole, middle and inferior pole of the cortex and medulla (21). The cortical ROI was set as an oval with an area of 0.14 cm2, while the medullary ROI was circular in shape with an area of 0.18 cm2. The average value of each kidney was taken as the T1 parameter value of that kidney for each radiologist. The final T1 value of each kidney was obtained by averaging the results of the two radiologists. The T1 parameters included the mean cortical T1 value (abbreviated as T1-C), the mean medullary T1 value (T1-M), the mean corticomedullary difference (ΔT1, T1-C − T1-M) and the mean corticomedullary ratio (T1%, T1-C/T1-M).
Renal histopathology
Ultrasound-guided renal biopsy was performed within 3 days after the MRI examination. Renal biopsies were performed by an experienced pathologist and an experienced nephrologist, who were blinded to the MRI examination results. The patients were kept in the prone position, and a hard sandbag was placed under the abdomen to reduce kidney movement during kidney puncture. In most of the cases, the bottom of the right kidney was the puncture point, 16-gauge needles were used in this study (22). The renal biopsy tissue samples underwent standard histopathological processing. RF was quantitatively evaluated from the kidney biopsy tissue sections by staining them with Masson’s trichrome-stain. The evaluation involved assessing the degrees of glomerular injury, tubular interstitial injury and renal atrophy. In addition, according to the proportion of interstitial fibrosis, these patients were classified as no RF (0%), mild RF (<25%), moderate RF (25–50%) and severe RF (>50%) (23).
Statistical analysis
Statistical analyses were performed using SPSS version 22.0 (IBM Corp., Armork, NY, USA) and MedCalc version 15.2.2 (MedCalc Software Ltd., Ostend, Belgium). Graphs were generated using Prism 5 (GraphPad Software Inc., CA, USA). The Kolmogorov-Smirnov test or Shapiro-Wilk test was used for normal distributions. Continuous variables with normal distributions are expressed as mean ± standard deviation (SD). Paired or independent samples t-tests and one-way analysis of variance (ANOVA) with Bonferroni correction were used to compare measurements. Continuous variables with a non-normal distribution were analyzed by the Wilcoxon signed-rank test, Mann-Whitney U test or Kruskal-Wallis test and are presented as medians [interquartile range (IQR)]. The interobserver correlation coefficient (ICC) was used to evaluate the variability between the two radiologists. The ICC value ranges from 0 to 1 and was greater than 0.75, indicating good reliability. Correlation analysis between the T1 parameters and clinical and histopathological values was performed by calculating a Pearson (r) or Spearman (rs) correlation coefficient (normality test dependent). The correlation coefficient measures the strength of the relationship between two variables (<0.3, weak correlation; 0.3–0.7, moderate correlation; >0.7, strong correlation) (24). Univariate and multivariate logistic regression models were used to predict RF, and the diagnostic performance of RF was evaluated using a receiver operating characteristic (ROC) curve. Two-tailed P<0.05 indicated statistical significance.
Results
Baseline characteristics of participants
The baseline characteristics of the 71 CKD patients and 40 HVs enrolled in this study are shown in Table 1. All 71 patients underwent renal biopsy and were further classified according to CKD stage (CKD 1: 36 cases; CKD 2: 19 cases; CKD 3: 14 cases; CKD 4: 2 cases) and RF stage (no RF: 9 cases; mild RF: 36 cases; moderate RF: 17 cases; severe RF: 9 cases). There were no significant differences in age, gender, height, weight, BMI, or blood glucose between the CKD and HV groups (P>0.05), but there were significant differences in blood pressure, eGFR, Scr and BUN between the two groups (P<0.05). The major primary causes of CKD in these patients were IgA nephropathy and membranous nephropathy, which together accounted for nearly 68% (48/71) of all cases. The interobserver variability results are shown in Table 2. The two radiologists had good reliability for all T1 parameter values for both kidneys, with ICCs greater than 0.75 and P values less than 0.001.
Table 2
Parameters | ICC | P |
---|---|---|
Left kidney | ||
T1-C | 0.904 | <0.001* |
T1-M | 0.874 | <0.001* |
ΔT1 | 0.764 | <0.001* |
T1% | 0.770 | <0.001* |
Right kidney | ||
T1-C | 0.854 | <0.001* |
T1-M | 0.821 | <0.001* |
ΔT1 | 0.792 | <0.001* |
T1% | 0.797 | <0.001* |
*, statistically significant. T1-C, mean cortical T1 value; T1-M, mean medullary T1 value; ΔT1, mean corticomedullary difference; T1%, mean corticomedullary ratio; ICC, interobserver correlation coefficient.
Comparison of native T1 mapping parameters in the CKD and HV groups
In the CKD group, there were no significant differences among any of the native T1 mapping parameters (T1-C, T1-M, ΔT1 and T1%) between the left and right kidneys, and similar results were found in the HV group (all P>0.05, Figure 2). Because there were no significant differences in the T1 parameters between the kidneys, the MRI values of the right (biopsied) kidney were used in this study. As shown in Table 3, the T1-C (P=0.003) and T1% (P<0.001) values were significantly longer in the CKD group than in the HV group (right kidney), while no significant difference was evident in T1-M (P=0.283). An increased mean cortical T1 resulted in a significantly reduced ΔT1 in the CKD group compared with the HV group (P<0.001).
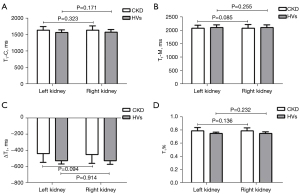
Table 3
Parameters | Left kidney | Right kidney | |||||||
---|---|---|---|---|---|---|---|---|---|
CKD | HVs | Statistics | P | CKD | HVs | Statistics | P | ||
T1-C (ms) | 1,636.56±112.23 | 1,567.89±81.49 | −3.372 | 0.001* | 1,639.96±121.80 | 1,575.31±78.70 | −3.017 | 0.003* | |
T1-M (ms) | 2,078.29±119.99 | 2,104.54±107.51 | 1.148 | 0.254 | 2,085.79±129.66 | 2,111.41±100.69 | 1.079 | 0.283 | |
ΔT1 (ms) | −441.73±105.78 | −527.47 [−545.64, −505.54] | −5.209 | <0.001* | −452.33 [−514.99, −405.47] | −531.33 [−549.73, −506.95] | −5.123 | <0.001* | |
T1% | 0.7847 [0.7578, 0.8098] | 0.7479 [0.7378, 0.7575] | −5.497 | <0.001* | 0.7870±0.0469 | 0.7499 [0.7357, 0.7568] | −5.374 | <0.001* |
Data are presented as mean ± standard deviation or median [interquartile range]. *, statistically significant. CKD, chronic kidney disease; HVs, healthy volunteers; T1-C, mean cortical T1 value; T1-M, mean medullary T1 value; ΔT1, mean corticomedullary difference; T1%, mean corticomedullary ratio.
The T1 parameters (T1-C, ΔT1 and T1%) with statistically significant differences between the CKD and HV group were used for the CKD subgroups analysis, and significant differences were found among the CKD subgroups (all P<0.05, Table 4). However, there were no significant differences in the T1-C value between CKD 1 and 2 (P>0.990), 2 and 3 (P=0.074), 3 and 4 (P=0.610). The ΔT1 and T1% values showed significant differences in all pairwise comparisons except for CKD 2 and 3 (P>0.05, Figure 3).
Table 4
Parameters | CKD 1 (n=36) | CKD 2 (n=19) | CKD 3 (n=14) | CKD 4 (n=2) | Statistics | P |
---|---|---|---|---|---|---|
T1-C (ms) | 1,613.68±102.69 | 1,612.81±119.22 | 1,713.83±116.17 | 1,853.61±177.48 | 5.546 | 0.002* |
ΔT1 (ms) | −502.29±89.79 | −413.99±73.92 | −381.10±78.38 | −185.18±76.47 | 15.739 | <0.001* |
T1% | 0.7631±0.0383 | 0.7960±0.0341 | 0.8186±0.0331 | 0.9108±0.0264 | 17.061 | <0.001* |
Data are presented as mean ± standard deviation. *, statistically significant. CKD, chronic kidney disease; T1-C, mean cortical T1 value; ΔT1, mean corticomedullary difference; T1%, mean corticomedullary ratio.
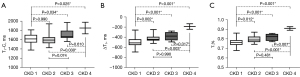
Correlation of T1 parameters with clinical and histopathological values
As shown in Figure 4, there were no correlations between T1-C, ΔT1, T1% and blood pressure (all P>0.05). The T1-C value was negatively correlated with eGFR (r=−0.319, P=0.007) and positively correlated with BUN (rs=0.235, P=0.049), but there was no correlation between T1-C and Scr or 24h-UPRO (P>0.05). The ΔT1 and T1% values were moderately correlated with eGFR (ΔT1: rs=−0.561; T1%: r=−0.602), Scr (ΔT1: rs=0.591; T1%: rs=0.563) and BUN (ΔT1: rs=0.433; T1%: rs=0.435; all P<0.001). Neither ΔT1 nor T1% were correlated with the 24h-UPRO (P>0.05). In addition, there were moderate positive correlations between the three T1 parameters and the histopathological scores (T1-C: rs=0.355, P=0.003; ΔT1: rs=0.630, P<0.001; T1%: rs=0.658, P<0.001).
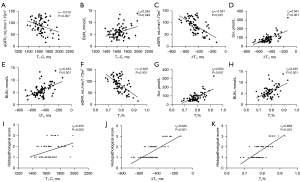
Univariate and multivariate logistic regression models for RF diagnosis
The native T1 mapping coronary raw images and pseudocolor images of both kidneys in each RF subgroup are shown in Figure 5. Univariate logistic regression of patient information, biochemical values and T1 parameters was used to identify significantly different indicators (age, eGFR, ΔT1, and T1%), which were then used for multivariate binary logistic regression (Table 5). Due to multicollinearity between ΔT1 and T1% (rs=0.945, P<0.001), the strong correlation made it inappropriate for these parameters to be included in the same model. Therefore, two models (Model 1: age + eGFR + ΔT1; Model 2: age + eGFR + T1%) were used to identify independent predictors for RF. In addition to age, the independent predictors to diagnose RF were ΔT1 (P=0.028) and T1% (P=0.037).
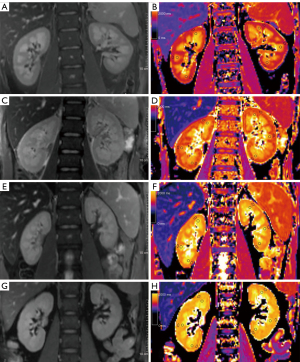
Table 5
Variables | Univariate logistic regression | Multivariate logistic regression (Model 1) | Multivariate logistic regression (Model 2) | |||||
---|---|---|---|---|---|---|---|---|
OR (95% CI) | P | OR (95% CI) | P | OR (95% CI) | P | |||
Age | 1.085 (1.013–1.163) | 0.020* | 1.107 (1.013–1.211) | 0.025* | 1.087 (1.005–1.175) | 0.037* | ||
Gender | 0.659 (0.161–2.690) | 0.561 | N.A. | N.A. | N.A. | N.A. | ||
BMI | 0.916 (0.750–1.119) | 0.388 | N.A. | N.A. | N.A. | N.A. | ||
Blood pressure | 1.731 (0.423–7.077) | 0.445 | N.A. | N.A. | N.A. | N.A. | ||
Blood glucose | 1.710 (0.639–4.577) | 0.286 | N.A. | N.A. | N.A. | N.A. | ||
eGFR | 0.973 (0.946–1.001) | 0.048* | 1.007 (0.969–1.046) | 0.715 | 1.000 (0.966–1.036) | 0.985 | ||
Scr | 1.010 (0.988–1.032) | 0.376 | N.A. | N.A. | N.A. | N.A. | ||
BUN | 1.079 (0.791–1.472) | 0.631 | N.A. | N.A. | N.A. | N.A. | ||
24h-UPRO | 0.834 (0.718–0.968) | 0.183 | N.A. | N.A. | N.A. | N.A. | ||
T1-C | 1.005 (0.998–1.012) | 0.142 | N.A. | N.A. | N.A. | N.A. | ||
T1-M | 0.998 (0.993–1.004) | 0.499 | N.A. | N.A. | N.A. | N.A. | ||
ΔT1 | 1.011 (1.002–1.020) | 0.016* | 1.013 (1.001–1.025) | 0.028* | N.A. | N.A. | ||
T1% | 1.742E+11 (142.937, 2.124E+20) | 0.015* | N.A. | N.A. | 8.306E+10 (4.585, 1.505E+21) | 0.037* |
Model 1: age + eGFR + ΔT1; Model 2: age + eGFR + T1%. *, statistically significant. BMI, body mass index; eGFR, estimated glomerular filtration rate; Scr, serum creatinine; BUN, blood urea nitrogen; 24h-UPRO, 24-hour urinary protein; T1-C, mean cortical T1 value; T1-M, mean medullary T1 value; ΔT1, mean corticomedullary difference; T1%, mean corticomedullary ratio; OR, odds ratio; CI, confidence interval; N.A., not applicable.
Diagnostic performance of T1 parameters for predicting RF
ROC curve analysis was used to evaluate the diagnostic value of ΔT1 and T1% for the prediction of RF. There were no significant differences in AUCs between ΔT1 and T1% among the no RF vs. RF, mild RF vs. moderate-severe RF and severe RF vs. mild-moderate RF comparisons (Figure 6, all P>0.05). As shown in Table 6, at the optimal threshold of −474.47 ms, the ΔT1 value had 66.1% (53.0–77.7%) sensitivity and 88.9% (51.8–99.7%) specificity when comparing no RF with RF. The optimal cutoff for diagnosing RF was set as 0.7829, and the sensitivity and specificity for the T1% value at this threshold were 51.6% (38.6–64.5%) and 100.0% (66.4–100.0%), respectively. When mild RF and moderate-severe RF were compared, the ΔT1 value was set as −410.07 ms [sensitivity, 61.5% (40.6–79.8%); specificity, 91.7% (77.5–98.2%)] and the T1% value was set as 0.8222 [sensitivity, 53.9% (33.4–73.4%); specificity, 97.2% (85.5–99.9%)]. When comparing severe RF and mild-moderate RF, the optimal cutoff for differentiating them was −343.81 ms for ΔT1 [sensitivity, 100.0% (66.4–100.0%); specificity, 90.6% (79.3–96.9%)] and 0.8359 for T1% [sensitivity, 100.0% (66.4–100.0%); specificity, 94.3% (84.3–98.8%)]. We also analyzed the different degrees of RF. Overall, there were significant differences among mild, moderate and severe RF (ΔT1: F=23.785; T1%: F=29.665, P<0.001). In addition, the ΔT1 and T1% values were significantly different in all pairwise comparisons (Figure 7, all P<0.05).
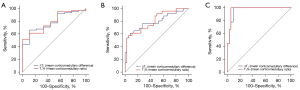
Table 6
Parameters | No RF & RF (n=9 & n=62) | Mild RF & moderate-severe RF (n=36 & n=26) | Mild-moderate RF& severe RF (n=53 & n=9) | |||||||||||
---|---|---|---|---|---|---|---|---|---|---|---|---|---|---|
AUC (95% CI) | Cutoff | Se (95% CI) | Sp (95% CI) | AUC (95% CI) | Cutoff | Se (95% CI) | Sp (95% CI) | AUC (95% CI) | Cutoff | Se (95% CI) | Sp (95% CI) | |||
ΔT1 (ms) | 0.781 (0.667–0.871) | −474.47 | 66.1 (53.0–77.7) | 88.9 (51.8–99.7) | 0.795 (0.673–0.887) | −410.07 | 61.5 (40.6–79.8) | 91.7 (77.5–98.2) | 0.964 (0.883–0.995) | −343.81 | 100.0 (66.4–100.0) | 90.6 (79.3–96.9) | ||
T1% | 0.789 (0.675–0.876) | 0.7829 | 51.6 (38.6–64.5) | 100.0 (66.4–100.0) | 0.806 (0.685–0.895) | 0.8222 | 53.9 (33.4–73.4) | 97.2 (85.5–99.9) | 0.973 (0.896–0.998) | 0.8359 | 100.0 (66.4–100.0) | 94.3 (84.3–98.8) |
RF, renal fibrosis; AUC, the area under the receiver operating characteristic curve; Se, sensitivity; Sp, specificity; CI, confidence interval; ΔT1, mean corticomedullary difference; T1%, mean corticomedullary ratio.
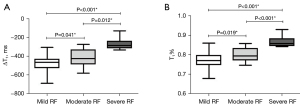
Discussion
Although native T1 mapping has been widely studied in myocardial and liver fibrosis, few studies have assessed T1 mapping in the context of RF. In our study, four T1 parameters, T1-C, T1-M, ΔT1 and T1%, were used to non-invasively evaluate renal function in CKD patients and to quantitatively assess RF. Similar investigations were previously reported by Berchtold et al. (25), Wu et al. (26) and Buchanan et al. (27). However, all of those studies had a smaller sample size of renal biopsy-proven native CKD patients, and none investigated the role of T1% in CKD and RF. In the present study, we found that the T1-C, ΔT1 and T1% values could be used to help diagnose CKD. Compared with HVs, the CKD group showed a significant increase in T1-C, a significant decrease in ΔT1 and no significant change in T1-M, which was in accordance with previous studies (27,28). Most studies have focused on the effect of the cortical T1 value and corticomedullary differences on CKD and RF, and no related studies on the corticomedullary ratio (T1%) have been reported thus far. We found that T1% was higher in the CKD group than in the HV group, mainly due to a significant increase in the cortical T1 value, which was caused by extracellular fluid due to interstitial edema, inflammation or fibrosis (27).
With the exception of T1-M, the other three T1 parameters (T1-C, ΔT1 and T1%) could be used for evaluating the CKD stage. We found that an increased T1-C and T1%, but a decreased ΔT1, were associated with a higher CKD stage, with a good correlation between these three parameters and renal function. However, there were no obvious differences in the T1-C value between CKD 1 and 2, 2 and 3, 3 and 4. Unlike T1-C, the ΔT1 and T1% values showed significant differences in the pairwise comparisons between each CKD subgroup, except for between CKD 2 and 3. These results indicated that ΔT1 and T1% were better than T1-C for assessing renal function in CKD patients. In the CKD group, T1-C, ΔT1 and T1% had mild-to-moderate correlations with eGFR, BUN and Scr. In addition, these three T1 parameters showed moderate positive correlations with the histopathological score. Similar results were reported by Wu et al. (26). They concluded that cortical T1 could be used for CKD staging and the cortical T1 value was positively correlated with the pathological score. Buchanan et al. also reported moderate negative correlations between cortical T1, ΔT1 and eGFR (27).
We further investigated the role of T1 parameters in the quantitative assessment of RF. All 71 CKD patients were pathologically confirmed by right kidney biopsy and stratified into four RF subgroups (no RF, mild RF, moderate RF and severe RF). The univariate and multivariate logistic regression analyses showed that ΔT1, but not T1-C, was an independent predictor for the diagnosis of RF in addition to patient age. Our results were similar to the study reported by Berchtold et al. (25), but inconsistent with Wu’s study (26). Although fibrosis usually affects the renal cortex, the T1-C value varied widely among individuals, ranging from 1,400 to 1,750 ms in our study. The ΔT1 value generated by comparing the cortex with the medulla could effectively reduce the difference. We also found that the ΔT1 value became significantly reduced with a higher degree of RF. Interestingly, the T1% value was an independent factor of RF prediction, which may be explained by the same reason as for ΔT1. These cortex-related values (like ΔT1 and T1%) weakened the difference among individuals, which helped to better observe RF-associated changes. The diagnostic performances of ΔT1 and T1% were separately analyzed for differentiating no RF (0%) from RF, mild RF (<25%) from moderate-severe RF, and mild-moderate RF from severe RF (>50%). We found that ΔT1 and T1% could distinguish the RF subgroups when appropriate thresholds were set. They could identify patients with >50% fibrosis with high sensitivity (ΔT1:100.0%; T1%: 100.0%) and specificity (ΔT1:90.6%; T1%: 94.3%). This ability would help to avoid biopsies that carry a risk of serious bleeding in the late stage of RF. In addition, the ΔT1 and T1% values were also able to identify patients with <25% fibrosis with good sensitivity (ΔT1: 61.5%; T1%: 53.9%) and high specificity (ΔT1: 91.7%; T1%: 97.2%), which would contribute to detecting early-stage RF and might help in developing effective treatments to slow RF progression. Our results demonstrated that ΔT1 and T1% generated by native T1 mapping could be used to non-invasively and quantitatively evaluate renal function and RF in CKD patients.
In our study, the commonly used MOLLI sequence was used to perform renal native T1 mapping without the application of contrast media, which could ensure accurate T1 measurements with high spatial resolution and within a single breath-hold. Renal native T1 mapping with the MOLLI sequence has previously shown satisfactory reproducibility in HVs and diabetic nephropathy patients (19). Recently, magnetic resonance fingerprinting (MRF) was established for relaxation mapping in the kidney. Compared with conventional MOLLI, similar accuracy and precision were achieved using an EPI-based MRF method to quantify the T1 time in the kidneys (29). However, additional studies are needed to explore the application of kidney MRF in routine clinical practice. In addition, renal imaging segmentation is an important step to derive the contours of the kidney, renal cortex and medulla. While manual delineation is currently still considered the gold standard for evaluating kidney segmentation, it is time-consuming, operator dependent and not sufficiently accurate. In our study, some ways may have mitigated the drawbacks of manual methods, the ROI delineation was independently performed by two radiologists, and the ROIs were plotted and averaged at multiple points for both the renal cortex and medulla. In addition, due to the subjective nature of manual methods, the effects of interobserver variability need to be quantified. Our results demonstrated good reliability for all T1 parameters for both kidneys, with ICCs all greater than 0.75 (ranging from 0.764 to 0.904).
There were several limitations to this study. First, this was a monocenter study. Despite the relatively large number of biopsy-proven native CKD patients included, there may have been patient selection bias. Multicenter studies with a larger sample size should be further conducted to validate our results. Second, we manually delineated the ROIs of the bilateral kidneys to obtain corresponding cortical and medullary T1 values. Although manual ROI selection of the renal cortex and medulla is considered to be an acceptable method for image analysis (30), automated ROIs selection is preferred over manual ROI selection. Furthermore, the automatic segmentation of renal cortical and medullary ROIs using artificial intelligence will be investigated our future study. Third, the primary etiology of CKD was not studied. In our study, IgA nephropathy and membranous nephropathy were the major primary causes of CKD. We will enlarge the sample size for an in-depth analysis in a future study.
In conclusion, the promising technology of renal native T1 mapping can be used for the non-invasive diagnosis and quantitative evaluation of CKD and RF. The ΔT1 and T1% values generated by native T1 mapping were more important than the cortical T1 value when quantitatively evaluating renal function and RF in patients with CKD. ΔT1 and T1% could identify patients with >50% fibrosis and <25% fibrosis, which would contribute to avoiding biopsy in late-stage RF and could help guide physicians when making decision in early-stage RF in CKD patients.
Acknowledgments
We thank AJE Editing Service for editing this manuscript.
Funding: This work was financially supported by the Suzhou Science and Technology Bureau Development Plan (Grant No. SYS2020147), the National Natural Science Foundation of China (Grant No. 81801754) and the Project of State Key Laboratory of Radiation Medicine and Protection, Soochow University (Grant No. GZK1202023).
Footnote
Reporting Checklist: The authors have completed the STARD reporting checklist. Available at https://qims.amegroups.com/article/view/10.21037/qims-22-1304/rc
Conflicts of Interest: All authors have completed the ICMJE uniform disclosure form (available at https://qims.amegroups.com/article/view/10.21037/qims-22-1304/coif). The authors have no conflicts of interest to declare.
Ethical Statement: The authors are accountable for all aspects of the work in ensuring that questions related to the accuracy or integrity of any part of the work are appropriately investigated and resolved. The study was conducted in accordance with the Declaration of Helsinki (as revised in 2013). This prospective, single-center study was approved by institutional ethics committee of our hospital (No. JD-LK-2022-060-01) and informed consent was taken from all the patients.
Open Access Statement: This is an Open Access article distributed in accordance with the Creative Commons Attribution-NonCommercial-NoDerivs 4.0 International License (CC BY-NC-ND 4.0), which permits the non-commercial replication and distribution of the article with the strict proviso that no changes or edits are made and the original work is properly cited (including links to both the formal publication through the relevant DOI and the license). See: https://creativecommons.org/licenses/by-nc-nd/4.0/.
References
- Stevens PE, Levin A. Evaluation and management of chronic kidney disease: synopsis of the kidney disease: improving global outcomes 2012 clinical practice guideline. Ann Intern Med 2013;158:825-30. [Crossref] [PubMed]
- Global, regional, and national burden of chronic kidney disease, 1990-2017: a systematic analysis for the Global Burden of Disease Study 2017. Lancet 2020;395:709-33. [Crossref] [PubMed]
- Levey AS, Stevens LA, Schmid CH, Zhang YL, Castro AF 3rd, Feldman HI, Kusek JW, Eggers P, Van Lente F, Greene T, Coresh J. A new equation to estimate glomerular filtration rate. Ann Intern Med 2009;150:604-12. [Crossref] [PubMed]
- Porrini E, Ruggenenti P, Luis-Lima S, Carrara F, Jiménez A, de Vries APJ, Torres A, Gaspari F, Remuzzi G. Estimated GFR: time for a critical appraisal. Nat Rev Nephrol 2019;15:177-90. [Crossref] [PubMed]
- Shi W, Liang X, Wu N, Zhang H, Yuan X, Tan Y. Assessment of Split Renal Function Using a Combination of Contrast-Enhanced CT and Serum Creatinine Values for Glomerular Filtration Rate Estimation. AJR Am J Roentgenol 2020;215:142-7. [Crossref] [PubMed]
- Liu Y. Renal fibrosis: new insights into the pathogenesis and therapeutics. Kidney Int 2006;69:213-7. [Crossref] [PubMed]
- Berchtold L, Friedli I, Vallée JP, Moll S, Martin PY, de Seigneux S. Diagnosis and assessment of renal fibrosis: the state of the art. Swiss Med Wkly 2017;147:w14442. [PubMed]
- Liu Y. Cellular and molecular mechanisms of renal fibrosis. Nat Rev Nephrol 2011;7:684-96. [Crossref] [PubMed]
- Xu S, Ma L, Lin J, Zhang Z, Wang X, Yin J. Efficacy and safety of percutaneous renal biopsy performed using 18G needle versus 16G needle: a single-center retrospective study. Int Urol Nephrol 2022;54:3255-61. [Crossref] [PubMed]
- Rudnick MR, Wahba IM, Leonberg-Yoo AK, Miskulin D, Litt HI. Risks and Options With Gadolinium-Based Contrast Agents in Patients With CKD: A Review. Am J Kidney Dis 2021;77:517-28. [Crossref] [PubMed]
- Sigmund EE, Vivier PH, Sui D, Lamparello NA, Tantillo K, Mikheev A, Rusinek H, Babb JS, Storey P, Lee VS, Chandarana H. Intravoxel incoherent motion and diffusion-tensor imaging in renal tissue under hydration and furosemide flow challenges. Radiology 2012;263:758-69. [Crossref] [PubMed]
- Xu X, Fang W, Ling H, Chai W, Chen K. Diffusion-weighted MR imaging of kidneys in patients with chronic kidney disease: initial study. Eur Radiol 2010;20:978-83. [Crossref] [PubMed]
- Li J, An C, Kang L, Mitch WE, Wang Y. Recent Advances in Magnetic Resonance Imaging Assessment of Renal Fibrosis. Adv Chronic Kidney Dis 2017;24:150-3. [Crossref] [PubMed]
- Li LP, Tan H, Thacker JM, Li W, Zhou Y, Kohn O, Sprague SM, Prasad PV. Evaluation of Renal Blood Flow in Chronic Kidney Disease Using Arterial Spin Labeling Perfusion Magnetic Resonance Imaging. Kidney Int Rep 2017;2:36-43. [Crossref] [PubMed]
- Morrell GR, Zhang JL, Lee VS. Magnetic Resonance Imaging of the Fibrotic Kidney. J Am Soc Nephrol 2017;28:2564-70. [Crossref] [PubMed]
- Wolf M, de Boer A, Sharma K, Boor P, Leiner T, Sunder-Plassmann G, Moser E, Caroli A, Jerome NP. Magnetic resonance imaging T1- and T2-mapping to assess renal structure and function: a systematic review and statement paper. Nephrol Dial Transplant 2018;33:ii41-50. [Crossref] [PubMed]
- Messroghli DR, Moon JC, Ferreira VM, Grosse-Wortmann L, He T, Kellman P, Mascherbauer J, Nezafat R, Salerno M, Schelbert EB, Taylor AJ, Thompson R, Ugander M, van Heeswijk RB, Friedrich MG. Clinical recommendations for cardiovascular magnetic resonance mapping of T1, T2, T2* and extracellular volume: A consensus statement by the Society for Cardiovascular Magnetic Resonance (SCMR) endorsed by the European Association for Cardiovascular Imaging (EACVI). J Cardiovasc Magn Reson 2017;19:75. [Crossref] [PubMed]
- Graham-Brown MP, Rutherford E, Levelt E, March DS, Churchward DR, Stensel DJ, McComb C, Mangion K, Cockburn S, Berry C, Moon JC, Mark PB, Burton JO, McCann GP. Native T1 mapping: inter-study, inter-observer and inter-center reproducibility in hemodialysis patients. J Cardiovasc Magn Reson 2017;19:21. [Crossref] [PubMed]
- Dekkers IA, Paiman EHM, de Vries APJ, Lamb HJ. Reproducibility of native T(1) mapping for renal tissue characterization at 3T. J Magn Reson Imaging 2019;49:588-96. [Crossref] [PubMed]
- Graham-Brown MP, Singh A, Wormleighton J, Brunskill NJ, McCann GP, Barratt J, Burton JO, Xu G. Association between native T1 mapping of the kidney and renal fibrosis in patients with IgA nephropathy. BMC Nephrol 2019;20:256. [Crossref] [PubMed]
- Zhao J, Wang ZJ, Liu M, Zhu J, Zhang X, Zhang T, Li S, Li Y. Assessment of renal fibrosis in chronic kidney disease using diffusion-weighted MRI. Clin Radiol 2014;69:1117-22. [Crossref] [PubMed]
- Xu J, Wu X, Xu Y, Ren H, Wang W, Chen W, Shen P, Li X, Shi H, Xie J, Chen X, Zhang W, Pan X. Acute Kidney Disease Increases the Risk of Post-Kidney Biopsy Bleeding Complications. Kidney Blood Press Res 2020;45:873-82. [Crossref] [PubMed]
- Srivastava A, Palsson R, Kaze AD, Chen ME, Palacios P, Sabbisetti V, Betensky RA, Steinman TI, Thadhani RI, McMahon GM, Stillman IE, Rennke HG, Waikar SS. The Prognostic Value of Histopathologic Lesions in Native Kidney Biopsy Specimens: Results from the Boston Kidney Biopsy Cohort Study. J Am Soc Nephrol 2018;29:2213-24. [Crossref] [PubMed]
- Ratner B. The correlation coefficient: its values range between +1/-1, or do they? Journal of Targeting Measurement and Analysis for Marketing 2009;17:139-42. [Crossref]
- Berchtold L, Friedli I, Crowe LA, Martinez C, Moll S, Hadaya K, de Perrot T, Combescure C, Martin PY, Vallée JP, de Seigneux S. Validation of the corticomedullary difference in magnetic resonance imaging-derived apparent diffusion coefficient for kidney fibrosis detection: a cross-sectional study. Nephrol Dial Transplant 2020;35:937-45. [Crossref] [PubMed]
- Wu J, Shi Z, Zhang Y, Yan J, Shang F, Wang Y, Lu H, Gu H, Dou W, Wang X, Yuan L. Native T1 Mapping in Assessing Kidney Fibrosis for Patients With Chronic Glomerulonephritis. Front Med (Lausanne) 2021;8:772326. [Crossref] [PubMed]
- Buchanan CE, Mahmoud H, Cox EF, McCulloch T, Prestwich BL, Taal MW, Selby NM, Francis ST. Quantitative assessment of renal structural and functional changes in chronic kidney disease using multi-parametric magnetic resonance imaging. Nephrol Dial Transplant 2020;35:955-64. [Crossref] [PubMed]
- Cox EF, Buchanan CE, Bradley CR, Prestwich B, Mahmoud H, Taal M, Selby NM, Francis ST. Multiparametric Renal Magnetic Resonance Imaging: Validation, Interventions, and Alterations in Chronic Kidney Disease. Front Physiol 2017;8:696. [Crossref] [PubMed]
- Hermann I, Chacon-Caldera J, Brumer I, Rieger B, Weingärtner S, Schad LR, Zöllner FG. Magnetic resonance fingerprinting for simultaneous renal T(1) and T2* mapping in a single breath-hold. Magn Reson Med 2020;83:1940-8. [Crossref] [PubMed]
- Dekkers IA, de Boer A, Sharma K, Cox EF, Lamb HJ, Buckley DL, et al. Consensus-based technical recommendations for clinical translation of renal T1 and T2 mapping MRI. MAGMA 2020;33:163-76. [Crossref] [PubMed]