Model development and validation of noninvasive parameters based on coronary computed tomography angiography to predict culprit lesions in acute coronary syndromes within 3 years: value of plaque characteristics, hemodynamics and pericoronary adipose tissue
Introduction
Acute coronary syndrome (ACS) is a disease mainly caused by atherosclerosis (more than 90%), which is characterized by an acute onset and many complications. Therefore, it is necessary to focus on atherosclerosis for this disease (1). Most ACS is not due to obstructive coronary disease but rather rupture of vulnerable plaques (2), indicating that it is important to identify vulnerable plaques for patients. Coronary computed tomography angiography (CTA) provides plaque information in a noninvasive imaging modality (3,4), providing clinical predictive value through quantification and analysis of plaques to identify adverse plaques. In addition, the advent of coronary computed tomography-derived fractional flow reserve (FFRCT) enables coronary CTA to combine detailed anatomical and physiological information in a single standard examination (5,6), which can further reflect the presence of ischemic symptoms at the lesion site. However, plaque rupture is a complex clinical process, and the advent of a plaque to rupture and cause ACS is affected by many factors. Vascular inflammation is a key factor leading to the formation and rupture of atherosclerotic plaques, which can lead to the occurrence of ACS (7). A correlation between inflammation and pericoronary adipose tissue (PCAT) has been established; pro-inflammatory factors are released from the inflamed vessel wall and propagate in a paracrine manner toward PCAT, which inhibits local adipogenesis in PCAT, and this process is detectable in CTA by quantifying the fat attenuation index (FAI) (8-10).
Previous studies have shown that adding noninvasive hemodynamic parameters to anatomical and plaque characteristics improves the prediction of culprit lesions (11), and PCAT features have also been used to predict ACS (12). However, the predictive value of combining plaque characteristics, noninvasive hemodynamic parameters and PCAT for ACS has not been investigated. The use of machine learning (ML) on the basis of imaging can provide incremental value in cardiovascular disease diagnosis, facilitating faster and more accurate diagnosis and decision-making, thereby significantly reducing the burden of disease (13-15). Therefore, the present study used machine learning to develop and validate models of culprit lesions based on plaque characteristics, hemodynamic parameters and PCAT attenuation of CTA to identify culprit lesions that cause subsequent ACS in the next 3 months to 3 years. The present study is performed in accordance with the TRIPOD reporting checklist (available at https://qims.amegroups.com/article/view/10.21037/qims-22-1045/rc).
Methods
Study population
The study population was retrospectively recruited from the Fourth Affiliated Hospital of Harbin Medical University (HeiLongJiang Province, China) and included patients with well-documented ACS [unstable angina (UA), ST-elevation myocardial infarction (STEMI), and non-ST-elevation myocardial infarction (NSTEMI)] who underwent CTA at our hospital 3 months to 3 years ago due to symptoms, such as chest pain with suspected coronary artery disease (CAD). The diagnosis of ACS was based on the guidelines published by the 2020 ESC on the management of acute coronary syndromes (16). The patients included in this study had no clinically typical symptoms and definite evidence of ACS at the time of CTA examination. Invasive coronary angiography (ICA) was performed at the time of ACS, and culprit and nonculprit lesions were identified by interventional cardiologists who were unaware of the CTA evaluation results. Th exclusion criteria were as follows: (I) restenotic lesions after stenting at the time of ACS occurrence; (II) history of coronary artery bypass grafting (CABG) revascularization before ACS occurred; (III) implanted cardiac devices and abnormal coronary arteries confirmed by conventional CTA; and (IV) patients with poor-quality CTA images insufficient for hemodynamic and PCAT analysis. A total of 132 patients with 240 lesions were included in the present study. The lesions of patients from January to December 2020 (n=154) were included in the training set, and the lesions of patients from January to July 2021 (n=86) were included in the test set in chronological order. The flow chart of the study design is shown in Figure 1. The present study was conducted in accordance with the Declaration of Helsinki (as revised in 2013), and it was approved by the Medical Ethics Committee of The Fourth Affiliated Hospital, Harbin Medical University (No. 2022-SCILLSC-11). Individual consent for this retrospective analysis was waived.
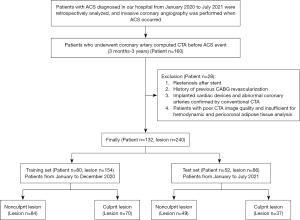
CTA acquisition
All scans were performed on a Toshiba 320-row computed tomography scanner (Aquilion ONE, Toshiba, Tokyo, Japan). A prospective ECG gating protocol was used for the CTA scan with the following scan parameters: tube voltage was 100 or 120 kV; tube current was determined by body mass index (300–500 mA); and time resolution was 275 ms. Prior to contrast injection, the control heart rate was <80 beats/min, and patients with high heart rates underwent CTA after heart rate stabilization or were considered for beta blockers at the discretion of the treating physician. Then, 60–70 mL of iodine contrast medium (350 mg/mL iodine-containing ethanol solution) was injected at a flow rate of 4.5–5 mL/s. The reconstructed image matrix was 512*512 with a layer thickness of 0.5 mm and increments of 0.25 mm.
Coronary plaque analysis
All patient images were retrospectively analyzed using semiautomatic plaque analysis software (Vitrea version 7.6; Vital Images). The software automatically extracted the coronary artery tree and automatically identified the inner and outer diameters of the lumen, and we performed manual correction when identifying errors (Figure 2A-2C). Plaque morphological features, including vessel location, diameter stenosis (DS), length, distance from the ostium, minimal lumen area (MLA) and spotty calcification, were measured (17). Low attenuation plaque (LAP) volume, fibrous plaque (FP) volume (medium attenuation plaque), calcified plaque (CP) volume and total plaque (TP) volume were also measured (17). Plaque burden (11), wall-to-lumen volume ratio, remodeling index and positive remodeling were quantitatively calculated. A total of 13 plaque parameters were analyzed. The detailed parameter definitions are provided in the Appendix 1.
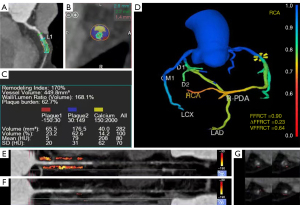
Analysis of hemodynamic parameters of coronary CTA
For the hemodynamic analysis, a software model based on computational fluid dynamics (Shukun Technology) was used. In each subject, the fractional flow reserve at the lesion site was calculated (expressed as FFRCT), and the vessel FFRCT (VFFRCT) was defined as the minimum value of the distal FFRCT of the vessel encompassing the entire coronary artery (18). ∆FFRCT was defined as the subtraction value of the proximal and distal FFRCT of the lesion (19) (Figure 2D).
PCAT attenuation analysis
We recorded the proximal 40 mm segments of the three major coronary arteries [left anterior descending artery (LAD), left circumflex artery (LCX) and right coronary artery (RCA)] containing lesions (RCA analyzed vessels 10–50 mm from the ostium), and we defined PCAT as the radial distance from the vessel wall equal to the vessel diameter for all voxels in the range of −190 to −30 HU (20). PCAT attenuation was calculated from the average attenuation of perivascular adipose tissue, and the fat attenuation index (FAI) was determined by quantifying weighted perivascular fat attenuation after adjustment for technical parameters. We used dedicated analysis software (Shukun Technology) to measure the proximal FAI of the vessel where the lesion was located (Figure 2E). In addition, we measured lesion-specific FAI (21), which was measured proximal and distal 5 mm from the center of the lesion as well as 10 mm in length and 2 mm from the outer diameter of the vessel (Figure 2F,2G).
Machine learning model construction, validation and performance
In the development cohort, logistic regression analysis was performed to identify factors associated with culprit lesions, and parameters with P<0.05 were included in the model construction (Appendix 1). Logistic regression (LR), random forest (RF) (22), Bayesian (23) and K-nearest neighbor (KNN)(22) algorithms were applied in the training set to build models. The area under the receiver operating characteristic (ROC) curve (AUC) was used as an indicator of the predictive performance of the model. Decision curve analysis (DCA) was used to elucidate the overall benefit of different predictive models. The nomogram of the combined model was established to predict the future incidence of ACS culprit lesions in patients with coronary artery disease.
Statistical analysis
Statistical analysis was performed using R software (version 4.1.0) and SPSS Statistics 25.0, and statistical significance was defined as two-sided P<0.05. Continuous variables with normal distribution are expressed as the mean ± standard deviation (SD), and nonnormal distributions are expressed as the median (25th and 75th). Categorical variables are expressed as number [n (%)]. T tests, Mann-Whitney U tests and chi-square tests were used to compare baseline characteristics of the cohorts.
Results
Baseline characteristics of patients
In this single-center retrospective study, a total of 160 patients with ACS who had both prior CTA images and concurrent ICA examinations were included according to previous inclusion criteria. The following patients were excluded: eight patients with restenosis after stenting, five patients who had undergone CABG surgery, four patients with implanted cardiac devices or containing abnormal coronary arteries, three patients with poor image quality and eight patients who could not be identified by software and measured by FFRCT or FAI. Ultimately, a total of 132 patients with 240 lesions (107 culprit lesions and 133 nonculprit lesions) were included in the present study. The training set included 154 lesions (70 culprit lesions and 84 nonculprit lesions), and the test set included 86 lesions (37 culprit lesions and 49 nonculprit lesions). The average interval between coronary CTA scan and ACS was 525.50 (329.00–758.75) days (Table 1). The baseline characteristics of the patients did not significantly differ between the training set and test set (all P>0.05).
Table 1
Patients | Total (N=132) | Training (N=80) | Test (N=52) | P |
---|---|---|---|---|
Age (years) | 63.70 (8.80) | 64.10 (8.74) | 63.10 (9.01) | 0.510 |
Male | 71 (53.8) | 38 (47.5) | 33 (63.5) | 0.106 |
BMI (kg/m2) | 25.30 (3.10) | 25.60 (3.03) | 24.80 (3.11) | 0.145 |
The average interval between CTA and ACS (days) | 525.50 [329.00, 758.75] | 520.00 [365.00, 736.00] | 557.00 [203.00, 802.00] | 0.234 |
Cardiovascular risk factors | ||||
Hypertension | 90 (68.2) | 59 (73.8) | 31 (59.6) | 0.130 |
Hyperlipidemia | 66 (50.0) | 44 (55.0) | 22 (42.3) | 0.212 |
Diabetes mellitus | 52 (49.4) | 32 (40.0) | 20 (38.5) | >0.999 |
Smoking | 46 (34.8) | 27 (33.8) | 19 (36.5) | 0.887 |
History of drinking | 22 (16.7) | 11 (13.8) | 11 (21.2) | 0.381 |
Clinical presentation | 0.542 | |||
UA | 100 (75.8) | 58 (72.5) | 42 (80.8) | |
NSTEMI | 17 (12.9) | 12 (15.0) | 5 (9.6) | |
STEMI | 15 (11.4) | 10 (12.5) | 5 (9.6) |
Table represents the baseline information for total patients, training set patients and test set patients. P values represent the difference between the training and test sets. Values are mean (SD), n (%), or median [25th, 75th]. BMI, body mass index; CTA, computed tomography angiography; ACS, acute coronary syndrome; UA, unstable angina; NSTEMI, non-ST-segment elevation myocardial infarction; STEMI, ST-segment elevation myocardial infarction.
Comparison of CTA parameters for culprit and nonculprit lesions
Based on the training set, we compared the differences in plaque characteristics, hemodynamic parameters and PCAT attenuation between culprit and nonculprit lesions (Table 2). Compared to nonculprit lesions, culprit lesions had a smaller MLA, longer lesion length and larger LAP, FP and TP volumes (all P<0.05). In terms of hemodynamic parameters, the culprit lesions had lower VFFRCT values and higher ΔFFRCT values (both P<0.01), while there was no significant difference in FFRCT values between culprit and nonculprit lesions (P=0.191). Regarding the comparison of PCAT attenuation, both proximal FAI and lesion-specific FAI in culprit lesions were higher than those in nonculprit lesions [−86.81±10.50 vs. −90.33±10.00 HU (P=0.036) and −77.67±11.8 vs. −85.80±10.10 HU (P<0.001), respectively]. The results of the comparison of CTA parameters for the test set are shown in Table 2.
Table 2
Characteristics | Training (N=154) | Test (N=86) | P | |||||
---|---|---|---|---|---|---|---|---|
Nonculprit (N=84) | Culprit (N=70) | P | Nonculprit (N=49) | Culprit (N=37) | P | |||
Vessel location | 0.348 | 0.737 | 0.811 | |||||
LAD | 30 (35.7) | 33 (47.1) | 17 (34.7) | 15 (40.5) | ||||
LCX | 25 (29.8) | 18 (25.7) | 17 (34.7) | 10 (27.0) | ||||
RCA | 29 (34.5) | 19 (27.1) | 15 (30.6) | 12 (32.4) | ||||
DS | 0.54 [0.38, 0.68] | 0.65 [0.50, 0.77] | 0.001 | 0.56 [0.45, 0.63] | 0.64 [0.56, 0.86] | 0.004 | 0.646 | |
Lesion length | 9.90 [7.40, 12.60] | 12.10 [8.68, 17.10] | 0.003 | 9.90 [7.50, 13.00] | 13.9 [9.50, 18.10] | 0.009 | 0.616 | |
Distance from ostium | 42.00 [32.10, 61.90] | 43.70 [32.60, 59.10] | 0.949 | 42.80 [36.90, 61.50] | 44.5 [32.40, 60.40] | 0.622 | 0.666 | |
MLA | 4.55 [3.10, 7.03] | 2.90 [1.42, 5.25] | 0.002 | 4.70 [3.10, 6.10] | 2.50 [0.90, 4.00] | 0.002 | 0.603 | |
Low attenuation plaque | 18.50 [12.70, 39.30] | 28.30 [15.60, 42.70] | 0.036 | 22.40 [13.60, 35.20] | 28.60 [18.20, 53.80] | 0.048 | 0.381 | |
Fibrous plaque | 46.40 [30.20, 72.40] | 66.90 [46.00, 104.00] | 0.003 | 51.80 [30.60, 85.40] | 74.00 [46.00, 146.00] | 0.009 | 0.346 | |
Calcified plaque | 35.60 [13.70, 62.50] | 36.60 [11.40, 72.50] | 0.795 | 25.90 [9.80, 48.40] | 40.00 [20.90, 49.50] | 0.186 | 0.282 | |
Total plaque volume | 104.00 [65.80, 159.00] | 129.00 [82.20, 230.00] | 0.039 | 107.00 [59.00, 148.00] | 151.00 [94.00, 232.00] | 0.015 | 0.845 | |
Positive remodeling | 23 (27.4) | 31 (44.3) | 0.043 | 10 (20.4) | 12 (32.4) | 0.310 | 0.171 | |
Wall to lumen volume ratio | 1.55 [1.12, 2.50] | 1.80 [1.45, 2.30] | 0.088 | 1.66 [1.12, 2.42] | 2.12 [1.68, 3.08] | 0.005 | 0.352 | |
Plaque burden | 0.61 (0.13) | 0.65 (0.11) | 0.080 | 0.60 (0.13) | 0.68 (0.12) | 0.003 | 0.668 | |
Spotty calcification | 12 (14.3) | 16 (22.9) | 0.245 | 8 (16.3) | 4 (10.8) | 0.677 | 0.508 | |
FFRCT | 0.92 [0.84, 0.97] | 0.89 [0.82, 0.96] | 0.191 | 0.94 [0.90, 0.97] | 0.88 [0.73, 0.93] | 0.001 | 0.841 | |
VFFRCT | 0.82 [0.72, 0.89] | 0.71 [0.64, 0.85] | 0.004 | 0.83 [0.75, 0.89] | 0.69 [0.62, 0.79] | <0.001 | 0.784 | |
∆FFRCT | 0.05 [0.02, 0.11] | 0.08 [0.03, 0.20] | 0.009 | 0.06 [0.03, 0.09] | 0.10 [0.04, 0.18] | 0.047 | 0.971 | |
Proximal FAI | −90.33 (10.00) | −86.81 (10.50) | 0.036 | −87.53 (8.61) | −83.78 (8.37) | 0.046 | 0.026 | |
Lesion specific FAI | −85.80 (10.10) | −77.67 (11.80) | <0.001 | −79.43 (11.90) | −69.14 (12.40) | <0.001 | <0.001 |
Table shows the comparison of the differences in CTA parameters for the culprit lesions and nonculprit lesions in the training and test sets, and the p values in the last column represent the comparison of the differences between the training and test set data. Values are mean (SD), n (%), or median [25th, 75th]. CTA, computed tomography angiography; LAD, left anterior descending artery; LCX, left circumflex artery; RCA, right coronary artery; DS, diameter stenosis; MLA, minimal lumen area; FFRCT, computed tomography-derived fractional flow reserve; ΔFFRCT, delta FFRCT; VFFRCT, vessel FFRCT; FAI, fat attenuation index.
CTA lesion characteristics to estimate the risk of culprit lesions
In the training set, univariate logistic regression analyses were performed to identify plaque characteristics, hemodynamic parameters and PCAT attenuation from CTA lesions associated with culprit lesions (Table 3) as well as to calculate odds ratios (ORs) expressed with 95% confidence intervals (CIs). The following ten high-risk factors (P<0.05) were identified (Table 3): DS, lesion length, MLA, low-attenuation plaque, fibrous plaque, positive remodeling, VFFRCT, ∆FFRCT, proximal FAI and lesion-specific FAI. The above parameters were used to construct a predictive model for ACS culprit lesions.
Table 3
Characteristics | OR | 95% CI | P |
---|---|---|---|
Plaque characteristics | |||
Vessel location LAD | Reference | ||
Vessel location LCX | 0.65 | 0.30–1.43 | 0.29 |
Vessel location RCA | 0.60 | 0.28–1.27 | 0.18 |
DS | 20.13 | 3.33–121.76 | <0.001 |
Lesion length | 1.07 | 1.01–1.12 | 0.01 |
Distance from ostium | 1.00 | 0.99–1.01 | 0.61 |
MLA | 0.85 | 0.76–0.95 | <0.001 |
Low attenuation plaque | 1.02 | 1.00–1.03 | 0.03 |
Fibrous plaque | 1.01 | 1.00–1.02 | <0.001 |
Calcified plaque | 1.00 | 1.00–1.00 | 0.80 |
Total plaque volume | 1.00 | 1.00–1.00 | 0.11 |
Positive remodeling | 2.11 | 1.08–4.13 | 0.03 |
Wall to lumen volume ratio | 1.07 | 0.84–1.35 | 0.60 |
Plaque burden | 10.19 | 0.72–143.83 | 0.09 |
Spotty calcification | 1.78 | 0.78–4.07 | 0.17 |
Hemodynamic parameters | |||
FFRCT | 0.15 | 0.01–3.55 | 0.24 |
VFFRCT | 0.03 | 0.00–0.37 | 0.01 |
∆FFRCT | 250.99 | 5.12–12,295.67 | 0.01 |
PCAT attenuation | |||
Proximal FAI | 1.03 | 1.00–1.07 | 0.04 |
Lesion specific FAI | 1.07 | 1.04–1.11 | <0.001 |
OR, odds ratio; CI, confidence interval; PCAT, pericoronary adipose tissue; CTA, computed tomography angiography; LAD, left anterior descending artery; LCX, left circumflex artery; RCA, right coronary artery; DS, diameter stenosis; MLA, minimal lumen area; FFRCT, computed tomography-derived fractional flow reserve; ΔFFRCT, delta FFRCT; VFFRCT, vessel FFRCT; FAI, fat attenuation index.
Develop predictive models for ACS culprit lesions using machine learning
Based on machine learning, a multiparameter combined model with 10 lesion features was constructed. The measured AUC values were used to compare the ability of the models constructed by the four algorithms to distinguish the culprit lesions (Figure 3A,3B). During the training process, the AUC values of the LR model, RF model, Bayesian model and KNN model were 0.809 (95% CI: 0.739–0.878), 0.990 (95% CI: 0.976–1.000), 0.693 (95% CI: 0.612–0.775), and 0.710 (95% CI: 0.628–0.793), respectively. In the test set, the LR model, RF model and Bayesian model had better predictive performance than the KNN model with AUC values of 0.819 [95% CI: 0.722–0.916), 0.754 (95% CI: 0.646–0.861), 0.754 (95% CI: 0.650–0.857) and 0.700 (95% CI: 0.586–0.815), respectively. It is worth noting that although the AUC value of the RF model was significantly higher than that of the LR model during training [AUC: 0.990 (95% CI: 0.976–1.000) vs. 0.809 (95% CI: 0.739–0.878)], the result was the opposite in the test set [AUC: 0.754 (95% CI: 0.646–0.861) vs. 0.819 (95% CI: 0.722–0.916)], which indicated that the RF model was overfitting during training.
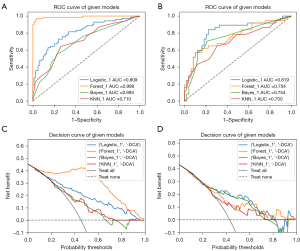
In the DCA curve (Figure 3C,3D), excluding the overfitting of the RF model in the training set, the LR model had the highest clinical application value and the best overall benefit of the model followed by the Bayesian model (Figure 3D). In the performance comparison of the four models (Table 4), the LR model had the best predictive performance with an accuracy, sensitivity, specificity, positive prediction and negative prediction 0.721, the 0.892, 0.592, 0.623 and 0.879, respectively. Combining the above factors, the logistic regression model had the best overall prediction performance, and the algorithm was selected for ML model construction.
Table 4
Model characteristics | Training | Test | |||||||
---|---|---|---|---|---|---|---|---|---|
LR | RF | Bayesian | KNN | LR | RF | Bayesian | KNN | ||
Accuracy | 0.740 | 0.955 | 0.675 | 0.662 | 0.721 | 0.640 | 0.733 | 0.674 | |
Sensitivity | 0.714 | 0.914 | 0.557 | 0.514 | 0.892 | 0.676 | 0.622 | 0.649 | |
Specificity | 0.762 | 0.988 | 0.774 | 0.786 | 0.592 | 0.612 | 0.816 | 0.694 | |
positive prediction | 0.714 | 0.985 | 0.672 | 0.667 | 0.623 | 0.568 | 0.719 | 0.615 | |
negative prediction | 0.762 | 0.933 | 0.677 | 0.660 | 0.879 | 0.714 | 0.741 | 0.723 | |
AUC (95% CI) | 0.809 (0.739–0.878) | 0.990 (0.976–1.000) | 0.693 (0.612–0.775) | 0.710 (0.628–0.793) | 0.819 (0.722–0.916) | 0.754 (0.646–0.861) | 0.754 (0.650–0.857) | 0.700 (0.586–0.815) |
LR, logistic regression; RF, random forest; KNN, K-nearest neighbor; AUC, area under the ROC curve; CI, confidence interval; ROC, receiver operating characteristic.
Evaluation and application of the ML model
We assessed the risk of ACS for each parameter in a combined prediction model containing ten parameters (Appendix 1). In this model, MLA was a protective factor for ACS (OR =0.81, 95% CI: 0.68–0.95, P=0.01). Of note, higher values indicated lower risk of the lesion becoming a culprit lesion. Positive remodeling and lesion-specific FAI were risk factors for culprit lesions (OR =3.06, 95% CI: 1.32–7.37, P=0.01; OR =1.10, 95% CI: 1.05–1.16, P<0.001, respectively) (Figure S1). In the nomogram (Figure 4), the visualization predicted the probability of future culprit lesions. The optimal cutoff value of the model was 0.408. When the Pr(label) value was greater than or equal to 0.408, the lesion prediction outcome was the culprit lesion, and when the Pr(label) value was less than 0.408, the lesion prediction outcome was the nonculprit lesion.
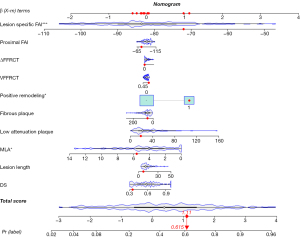
Comparison of the effects of different classification models in predicting ACS culprit lesions
In the test set, ten prediction parameters were formed into the following models according to categories to evaluate the plaque characteristics, hemodynamic parameters and PCAT attenuation for predicting the value of ACS: Model 1 was established for plaque characteristics; Model 2 was established for hemodynamic parameters; Model 3 was established for PCAT attenuation; Model 4 was established for plaque characteristics and hemodynamic parameters; and Model 5 was established for plaque characteristics, hemodynamic parameters and PCAT attenuation.
Among the three single-category models (Models 1–3), the ROC curve showed that the prediction effect of the plaque characteristics (Model 1) (AUC =0.793) was better than that of Model 2 (AUC =0.738) and Model 3 (AUC =0.715). Combining hemodynamic parameters and plaque features (Model 4) did not significantly improve prediction (AUC =0.783), but the combined model (Model 5) had the best prediction performance for culprit lesions (AUC =0.819) (Figure 5A). The DCA curve showed that Model 5 patients had the greatest net benefit followed by Model 4 patients (Figure 5B). The calibration curves for the different models are shown in Figure S2.
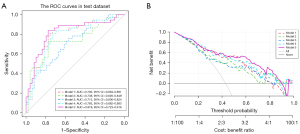
Discussion
The present study used ML to investigate the diagnostic performance of CTA-derived plaque characteristics, hemodynamics and PCAT attenuation in predicting culprit ACS lesions. In the present study, a simple random grouping was not used due to an insufficient sample size, but the patients were divided into training and test sets based on the year of enrollment, which more objectively reflected the generalization of the constructed model. The present results showed that there were significant differences in MLA, lesion length, LAP volume, FP volume, TP volume, VFFRCT, proximal FFRCT, proximal FAI and lesion-specific FAI between the culprit and nonculprit lesions (all P<0.05). Ten high-risk factors (all P<0.05) were screened from 18 CTA-derived parameters by logistic regression for ML model construction. The results showed that the ML model based on the LR algorithm was the best model. The comparison results of the combined models of different classifications showed that Model 5 had the best prediction effect (AUC =0.819).
Previous studies have suggested that ACS is associated with atherosclerotic plaque vulnerability (24). Identified high-risk plaque characteristics of CTA include LAP, spotty calcification, positive remodeling and napkin ring sign, and these characteristics are associated with the occurrence of ACS (25). Lee et al. (19) analyzed the predictive value of adverse plaque characteristics and hemodynamic parameters for ACS, and they reported that the relative importance of ∆FFRCT is higher than that of FFRCT, agreeing with the present results. In the present study, when adverse plaque and hemodynamic characteristics were added to the prediction model, the AUC continued to improve. The present study further investigated the predictive value of PCAT attenuation.
In addition to plaque characteristics and hemodynamic parameters, the discovery of PCAT attenuation provides more comprehensive inflammatory information for the lesion characteristics (9). Goeller et al. (26) reported that PCAT attenuation is increased around culprit lesions compared to nonculprit lesions, suggesting that FAI may help identify vulnerable plaques. Based on the level of the lesion, the present study measured the FAI of the proximal vessel where the lesion was located and the lesion-specific FAI to predict whether the lesion would become the culprit lesion for ACS. According to the logistic regression analysis, both proximal and lesion-specific FAI were risk factors for secondary ACS (proximal FAI: OR =1.03, P=0.04; lesion-specific FAI: OR =1.07, P<0.001), and the lesion-specific FAI had a higher value in predicting culprit lesions in the ML model (OR =1.1, P<0.001).
Plaque characteristics, hemodynamic parameters and PCAT attenuation all reflect the characteristics of lesions and provide corresponding clinical information for disease prediction. These parameters are all noninvasive parameters and do not require additional invasive examinations, thereby reducing harm to patients. In the present study, the plaque characteristics model (Model 1) outperformed Model 2 and Model 3, which may be due to the excessive number of parameters included in the plaque characteristics category. To the best of our knowledge, this study is the first to propose using ML combined with three-category CTA parameter characteristics to predict ACS culprit lesions based on lesion level. The present study investigated the direct correlation between the CTA parameter characteristics of each lesion and the culprit lesion to improve the identification of culprit lesions in patients prone to ACS. Early identification of culprit lesions will aid in timely preventive measures and interventions for treatment.
Limitations
The present study had some limitations. First, the present study was limited by retrospective study data, which may lead to inevitable bias. Further efforts to collect prospective data are necessary. Second, this was a single-center, single-vendor study. The prediction of ACS by different image acquisition parameters and different computed tomography scanners requires further study. Third, due to technical limitations, we measured the lesion-specific FAI in an area including 2 mm of vessel wall expansion, and it is necessary to exclude the 1 mm area around the vessel wall (which may include the effect of the eccentric plaque) for further validation. Fourth, the ACS patients in the present mainly had unstable angina pectoris, indicating that additional patients with culprit lesions of myocardial infarction need to be verified in the future. Fifth, the preset study analyzed ACS culprit lesions, and due to the limited number of patients, we were unable to analyze the CTA parameter characteristics for UA, NSTEMI, and STEMI subgroups to predict disease, indicating that further studies are needed. Sixth, the retrospective nature of the present study made it difficult to control the status of drug use from early CTA examination to ACS. However, changes in drug therapy during the clinical process were based on the actual patient situation, which is uncontrollable and unavoidable.
Conclusions
Using machine learning based on CTA parameters can predict ACS. Compared to other combined models, the model combining plaque characteristics, hemodynamic parameters and PCAT attenuation performed best in predicting culprit lesions of ACS.
Acknowledgments
Funding: This work was supported by the Beijing Cihua Medical Development Foundation Project (Research on CT-assisted diagnosis of coronary heart disease based on artificial intelligence), the Fourth Affiliated Hospital of Harbin Medical University (molecular imaging of myocardial oxidative stress response in obese states; No. HYDSYTB202228), and the Fourth Affiliated Hospital of Harbin Medical University (PD-L1 inhibitors in combination with targeted agents for kidney cancer treatment and molecular imaging; No. JD22C007).
Footnote
Reporting Checklist: The authors have completed the TRIPOD reporting checklist. Available at https://qims.amegroups.com/article/view/10.21037/qims-22-1045/rc
Conflicts of Interest: All authors have completed the ICMJE uniform disclosure form (available at https://qims.amegroups.com/article/view/10.21037/qims-22-1045/coif). MZ is a current employee of GE Healthcare. The other authors have no conflicts of interest to declare.
Ethical Statement: The authors are accountable for all aspects of the work in ensuring that questions related to the accuracy or integrity of any part of the work are appropriately investigated and resolved. The study was conducted in accordance with the Declaration of Helsinki (as revised in 2013). The study was approved by the Medical Ethics Committee of The Fourth Affiliated Hospital, Harbin Medical University (No. 2022-SCILLSC-11), and individual consent for this retrospective analysis was waived.
Open Access Statement: This is an Open Access article distributed in accordance with the Creative Commons Attribution-NonCommercial-NoDerivs 4.0 International License (CC BY-NC-ND 4.0), which permits the non-commercial replication and distribution of the article with the strict proviso that no changes or edits are made and the original work is properly cited (including links to both the formal publication through the relevant DOI and the license). See: https://creativecommons.org/licenses/by-nc-nd/4.0/.
References
- Tsao CW, Aday AW, Almarzooq ZI, Alonso A, Beaton AZ, Bittencourt MS, et al. Heart Disease and Stroke Statistics-2022 Update: A Report From the American Heart Association. Circulation 2022;145:e153-639. [Crossref] [PubMed]
- van Veelen A, van der Sangen NMR, Delewi R, Beijk MAM, Henriques JPS, Claessen BEPM. Detection of Vulnerable Coronary Plaques Using Invasive and Non-Invasive Imaging Modalities. J Clin Med 2022; [Crossref] [PubMed]
- Abdelrahman KM, Chen MY, Dey AK, Virmani R, Finn AV, Khamis RY, Choi AD, Min JK, Williams MC, Buckler AJ, Taylor CA, Rogers C, Samady H, Antoniades C, Shaw LJ, Budoff MJ, Hoffmann U, Blankstein R, Narula J, Mehta NN. Coronary Computed Tomography Angiography From Clinical Uses to Emerging Technologies: JACC State-of-the-Art Review. J Am Coll Cardiol 2020;76:1226-43. [Crossref] [PubMed]
- Moss AJ, Williams MC, Newby DE, Nicol ED. The Updated NICE Guidelines: Cardiac CT as the First-Line Test for Coronary Artery Disease. Curr Cardiovasc Imaging Rep 2017;10:15. [Crossref] [PubMed]
- Tesche C, De Cecco CN, Albrecht MH, Duguay TM, Bayer RR 2nd, Litwin SE, Steinberg DH, Schoepf UJ, Coronary CT. Angiography-derived Fractional Flow Reserve. Radiology 2017;285:17-33. [Crossref] [PubMed]
- Shi K, Yang FF, Si N, Zhu CT, Li N, Dong XL, Guo Y, Zhang T. Effect of 320-row CT reconstruction technology on fractional flow reserve derived from coronary CT angiography based on machine learning: single- versus multiple-cardiac periodic images. Quant Imaging Med Surg 2022;12:3092-103. [Crossref] [PubMed]
- Sugiyama T, Kanaji Y, Hoshino M, Yamaguchi M, Hada M, Ohya H, Sumino Y, Hirano H, Kanno Y, Horie T, Misawa T, Nogami K, Ueno H, Hamaya R, Usui E, Murai T, Lee T, Yonetsu T, Sasano T, Kakuta T. Determinants of Pericoronary Adipose Tissue Attenuation on Computed Tomography Angiography in Coronary Artery Disease. J Am Heart Assoc 2020;9:e016202. [Crossref] [PubMed]
- Margaritis M, Antonopoulos AS, Digby J, Lee R, Reilly S, Coutinho P, Shirodaria C, Sayeed R, Petrou M, De Silva R, Jalilzadeh S, Demosthenous M, Bakogiannis C, Tousoulis D, Stefanadis C, Choudhury RP, Casadei B, Channon KM, Antoniades C. Interactions between vascular wall and perivascular adipose tissue reveal novel roles for adiponectin in the regulation of endothelial nitric oxide synthase function in human vessels. Circulation 2013;127:2209-21. [Crossref] [PubMed]
- Oikonomou EK, Marwan M, Desai MY, Mancio J, Alashi A, Hutt Centeno E, et al. Non-invasive detection of coronary inflammation using computed tomography and prediction of residual cardiovascular risk (the CRISP CT study): a post-hoc analysis of prospective outcome data. Lancet 2018;392:929-39. [Crossref] [PubMed]
- Si N, Shi K, Li N, Dong X, Zhu C, Guo Y, Hu J, Cui J, Yang F, Zhang T. Identification of patients with acute myocardial infarction based on coronary CT angiography: the value of pericoronary adipose tissue radiomics. Eur Radiol 2022;32:6868-77. [Crossref] [PubMed]
- Park J, Lee JM, Koo BK, Choi G, Hwang D, Rhee TM, et al. Relevance of anatomical, plaque, and hemodynamic characteristics of non-obstructive coronary lesions in the prediction of risk for acute coronary syndrome. Eur Radiol 2019;29:6119-28. [Crossref] [PubMed]
- Shang J, Ma S, Guo Y, Yang L, Zhang Q, Xie F, Ma Y, Ma Q, Dang Y, Zhou K, Liu T, Yang J, Hou Y. Prediction of acute coronary syndrome within 3 years using radiomics signature of pericoronary adipose tissue based on coronary computed tomography angiography. Eur Radiol 2022;32:1256-66. [Crossref] [PubMed]
- Dey D, Slomka PJ, Leeson P, Comaniciu D, Shrestha S, Sengupta PP, Marwick TH. Artificial Intelligence in Cardiovascular Imaging: JACC State-of-the-Art Review. J Am Coll Cardiol 2019;73:1317-35. [Crossref] [PubMed]
- Lin A, Kolossváry M, Motwani M, Išgum I, Maurovich-Horvat P, Slomka PJ, Dey D. Artificial Intelligence in Cardiovascular Imaging for Risk Stratification in Coronary Artery Disease. Radiol Cardiothorac Imaging 2021;3:e200512. [Crossref] [PubMed]
- Lin A, Kolossváry M, Išgum I, Maurovich-Horvat P, Slomka PJ, Dey D. Artificial intelligence: improving the efficiency of cardiovascular imaging. Expert Rev Med Devices 2020;17:565-77. [Crossref] [PubMed]
- Collet JP, Thiele H, Barbato E, Barthélémy O, Bauersachs J, Bhatt DL, et al. 2020 ESC Guidelines for the management of acute coronary syndromes in patients presenting without persistent ST-segment elevation. Eur Heart J 2021;42:1289-367. [Crossref] [PubMed]
- Motoyama S, Sarai M, Harigaya H, Anno H, Inoue K, Hara T, Naruse H, Ishii J, Hishida H, Wong ND, Virmani R, Kondo T, Ozaki Y, Narula J. Computed tomographic angiography characteristics of atherosclerotic plaques subsequently resulting in acute coronary syndrome. J Am Coll Cardiol 2009;54:49-57. [Crossref] [PubMed]
- Doris MK, Otaki Y, Arnson Y, Tamarappoo B, Goeller M, Gransar H, Wang F, Hayes S, Friedman J, Thomson L, Slomka P, Dey D, Berman D. Non-invasive fractional flow reserve in vessels without severe obstructive stenosis is associated with coronary plaque burden. J Cardiovasc Comput Tomogr 2018;12:379-84. [Crossref] [PubMed]
- Lee JM, Choi G, Koo BK, Hwang D, Park J, Zhang J, et al. Identification of High-Risk Plaques Destined to Cause Acute Coronary Syndrome Using Coronary Computed Tomographic Angiography and Computational Fluid Dynamics. JACC Cardiovasc Imaging 2019;12:1032-43. [Crossref] [PubMed]
- Lin A, Nerlekar N, Yuvaraj J, Fernandes K, Jiang C, Nicholls SJ, Dey D, Wong DTL. Pericoronary adipose tissue computed tomography attenuation distinguishes different stages of coronary artery disease: a cross-sectional study. Eur Heart J Cardiovasc Imaging 2021;22:298-306. [Crossref] [PubMed]
- Ma R, van Assen M, Ties D, Pelgrim GJ, van Dijk R, Sidorenkov G, van Ooijen PMA, van der Harst P, Vliegenthart R. Focal pericoronary adipose tissue attenuation is related to plaque presence, plaque type, and stenosis severity in coronary CTA. Eur Radiol 2021;31:7251-61. [Crossref] [PubMed]
- Martin-Isla C, Campello VM, Izquierdo C, Raisi-Estabragh Z, Baeßler B, Petersen SE, Lekadir K. Image-Based Cardiac Diagnosis With Machine Learning: A Review. Front Cardiovasc Med 2020;7:1. [Crossref] [PubMed]
- Brophy JM. Bayesian Analyses of Cardiovascular Trials-Bringing Added Value to the Table. Can J Cardiol 2021;37:1415-27. [Crossref] [PubMed]
- Maurovich-Horvat P, Ferencik M, Voros S, Merkely B, Hoffmann U. Comprehensive plaque assessment by coronary CT angiography. Nat Rev Cardiol 2014;11:390-402. [Crossref] [PubMed]
- Motoyama S, Ito H, Sarai M, Kondo T, Kawai H, Nagahara Y, Harigaya H, Kan S, Anno H, Takahashi H, Naruse H, Ishii J, Hecht H, Shaw LJ, Ozaki Y, Narula J. Plaque Characterization by Coronary Computed Tomography Angiography and the Likelihood of Acute Coronary Events in Mid-Term Follow-Up. J Am Coll Cardiol 2015;66:337-46. [Crossref] [PubMed]
- Goeller M, Achenbach S, Cadet S, Kwan AC, Commandeur F, Slomka PJ, Gransar H, Albrecht MH, Tamarappoo BK, Berman DS, Marwan M, Dey D. Pericoronary Adipose Tissue Computed Tomography Attenuation and High-Risk Plaque Characteristics in Acute Coronary Syndrome Compared With Stable Coronary Artery Disease. JAMA Cardiol 2018;3:858-63. [Crossref] [PubMed]