A Bi-LSTM and multihead attention-based model incorporating radiomics signatures and radiological features for differentiating the main subtypes of lung adenocarcinoma
Introduction
Lung cancer is the leading cause of cancer-related death and is one of the most commonly diagnosed human malignancies (1). Adenocarcinoma is the dominant histological type of lung cancer and has shown an increasing incidence in recent decades (2,3). According to the World Health Organization (WHO) classification, lung adenocarcinoma is categorized into preinvasive lesions, such as atypical adenomatous hyperplasia (AAH) and adenocarcinoma in situ (AIS); minimally invasive adenocarcinoma (MIA); and invasive adenocarcinoma (IAC) (4,5). With the popularization and revitalization of computed tomography (CT), the ability to screen pulmonary nodules has developed rapidly. Adenocarcinoma often manifests on CT scans as pulmonary nodules, including pure ground-glass nodules (pGGNs), mixed ground-glass nodules (mGGNs), and solid nodules (SNs) (6). Radiologists identify the lesions by analyzing the changes in continuous layers of CT images and combining these changes with radiological features, which also provides an auxiliary diagnostic basis for clinical diagnosis. Imaging findings and pathological findings are not always equivalent, which affects the decision of diagnosis and treatment. The treatment schemes of AIS, MIA and IAC are quite different. Patients diagnosed with IAC of the lung typically undergo lobectomy (7-9), while those with AIS or MIA are managed with active surveillance or sublobar resection, respectively, due to differences in prognosis (10). If the results of image analysis are very similar to those of pathological analysis, this finding will play a positive guiding role in the choice of treatment or surgical plan. Low-dose computed tomography (LDCT) is one of the most popular lung cancer screening methods at present; it can realize the discovery of early cancer and has a positive effect on the reduction of lung cancer mortality. However, in recent years, many experts in this field have found that with the popularization of LDCT, excessive diagnosis and false-positive problems have inevitably arisen. For the first time, scholars have observed that the widespread use of LDCT screening could lead to overdiagnosis among young women who are nonsmokers and may lead to a large number of overdiagnoses and a falsely high 5-year survival rate (11-13). Computer analysis of CT image texture features can effectively assist doctors in determining subtypes, optimizing treatment plans and prognosis, and controlling overdiagnosis to a certain extent (14-17). The feature expression of CT images of lesions is a popular research topic at present, and disease recognition and diagnosis can be realized by analyzing these image features (18-20). Some studies analyzed a single CT image of a lesion and used algorithms such as convolutional neural network (CNN) to identify and diagnose the lesion by analyzing single-mode or multimode images (21,22). Research on neural network models based on 3D-CT images has also made many gratifying achievements in image calibration and diagnosis (23,24). Compared with the traditional analysis of imaging features and radiological features, the analysis of feature change trends and laws may be more accurate in identifying and diagnosing lesions. Thus, the purpose of this study was to develop a classification model based on Bi-LSTM and multihead attention using radiological features and radiomics signature sequences in continuous layers of CT images of lesions to assist radiologists in classifying MIA, AIS, and IAC. This study provides a new method for the auxiliary diagnosis of lung adenocarcinoma, which is closer to the diagnostic process of radiologists. The results of this study may also facilitate the mining and interpretability of diagnostic decision rules. We present this article in accordance with the TRIPOD reporting checklist (available at https://qims.amegroups.com/article/view/10.21037/qims-22-848/rc).
Methods
This study was conducted in accordance with the Declaration of Helsinki (as revised in 2013). The study was approved by the ethics committee of Shanghai University of Medicine & Health Sciences, and the written informed consent of patients was waived by the ethics committee because the study was a retrospective experiment and did not involve patient privacy.
Patients
A retrospective analysis was conducted of 421 patients (427 nodules) in three hospitals (Hospital 1, Shanghai Public Health Clinical Center; Hospital 2, Shanghai Ruijin Hospital; Hospital 3, Ningbo Beilun No. 2 Hospital) who were diagnosed with pulmonary adenocarcinomas based on pathologic analysis of surgical specimens. The inclusion criteria for the study were as follows: (I) patients had undergone routine CT examination within one month prior to surgery or diagnosis; and (II) the CT scans were acquired with a layer thickness of less than 2 mm. In cases where multiple nodules were detected, each nodule was analyzed separately for further analysis. The exclusion criteria were as follows: (I) significant artifacts in the CT image and (II) contrast agent used in the CT examination. Patient clinical information was also included in the study dataset. The flowchart of patient selection is listed in Figure 1.
In Hospital 1, 339 patients were randomly selected, with 341 pulmonary nodules (MIA: 206, 60.4%; AIS: 57, 16.7%; IAC: 78, 22.9%). In Hospitals 2 and 3, 82 patients with 86 pulmonary nodules were randomly selected (MIA: 54, 62.8%; AIS: 10, 11.6%; IAC: 22, 25.6%). Overall, there were 227 patients with 227 lesions in the training cohort, 112 patients with 114 lesions in the testing cohort, and 82 patients with 86 lesions in the verification cohort. The patient information is listed in Table 1.
Table 1
Characteristics | Training cohort (n=227) | Testing cohort (n=112) | Validation cohort (n=86) | ||||||||
---|---|---|---|---|---|---|---|---|---|---|---|
MIA/AIS (n=177) | IAC (n=50) | P value | MIA/AIS (n=86) | IAC (n=28) | P value | MIA/AIS (n=64) | IAC (n=22) | P value | |||
Age (years) | 50.74±11 | 54.60±11 | <0.05 | 51.43±11 | 53.28±11 | <0.05 | 54.02±14 | 62.74±11 | <0.05 | ||
Gender | 0.21 | 0.19 | 0.12 | ||||||||
Male | 53 | 18 | 35 | 10 | 25 | 8 | |||||
Female | 124 | 32 | 51 | 18 | 39 | 14 | |||||
Margin | 0.83 | 0.99 | 0.49 | ||||||||
Blurred | 154 | 46 | 77 | 24 | 33 | 16 | |||||
Clear | 23 | 4 | 9 | 4 | 9 | 6 | |||||
Lobulation | <0.05 | 0.18 | 0.12 | ||||||||
Present | 134 | 40 | 58 | 23 | 22 | 5 | |||||
Absent | 43 | 10 | 28 | 5 | 42 | 17 | |||||
Spiculation | 0.46 | 0.63 | 0.17 | ||||||||
Present | 127 | 33 | 61 | 23 | 58 | 20 | |||||
Absent | 50 | 17 | 25 | 5 | 6 | 2 | |||||
Pleural attachment | <0.05 | <0.05 | 0.3273 | ||||||||
Present | 28 | 23 | 19 | 15 | 28 | 12 | |||||
Absent | 149 | 27 | 67 | 13 | 36 | 10 | |||||
Air bronchogram | 0.09 | <0.05 | 0.14 | ||||||||
Present | 85 | 27 | 45 | 16 | 34 | 6 | |||||
Absent | 92 | 23 | 41 | 12 | 30 | 16 | |||||
Vessel change | 0.63 | 0.58 | <0.05 | ||||||||
Present | 121 | 37 | 69 | 25 | 52 | 19 | |||||
Absent | 56 | 13 | 17 | 3 | 12 | 3 | |||||
Bubble lucency | 0.19 | 0.18 | 0.09 | ||||||||
Present | 53 | 21 | 37 | 13 | 23 | 10 | |||||
Absent | 124 | 29 | 49 | 15 | 41 | 12 | |||||
Average major axis (mm) | 9.12±3.07 | 11.02±1.22 | <0.05 | 9.10±2.62 | 11.38±2.17 | <0.05 | 11.11±4.36 | 18.78±7.92 | <0.05 | ||
Average minor axis (mm) | 7.09±2.03 | 8.45±2.39 | <0.05 | 6.27±1.99 | 9.61±2.40 | <0.05 | 8.68±3.05 | 14.53±4.37 | <0.05 | ||
Lobe | 0.38 | 0.21 | 0.07 | ||||||||
Left | 74 | 23 | 40 | 12 | 20 | 13 | |||||
Right | 103 | 27 | 46 | 16 | 44 | 9 | |||||
Classification | <0.05 | <0.05 | <0.05 | ||||||||
pGGN | 135 | 21 | 67 | 9 | 55 | 7 | |||||
mGGN | 42 | 29 | 19 | 19 | 9 | 15 |
Numeric features are presented as the mean ± standard deviation. MIA, minimally invasive adenocarcinoma; AIS, adenocarcinoma in situ; IAC, invasive adenocarcinoma; pGGN, pure ground-glass nodule; mGGN, mixed ground-glass nodule.
CT image acquisition
An unenhanced chest CT exam was performed for an entire lung scan of each patient in all three hospitals. At Hospital 1, the CT images were obtained by a United-Imaging 760 CT device (tube voltage of 120 kVp, tube current modulation of 42–126 mA, and 1.0 mm reconstructed slice thickness) and a Siemens Emotion 16 CT device (tube voltage of 130 kVp, tube current modulation of 34–123 mA, and 1.0 mm reconstructed slice thickness) with a 512×512 resolution. At Hospitals 2 and 3, a Philips iCT 256 CT device (tube voltage of 120 kVp, tube current modulation of 161 mA, and 1.0 mm reconstructed slice thickness) and a Philips Brilliance 16 CT device (tube voltage of 120 kVp, tube current modulation of 219 mA, and 1.0 mm reconstructed slice thickness) were used to obtain CT images.
Radiomics signatures and radiological feature extraction
Preliminary segmentation of CT images was performed using internal semiautomatic segmentation software (25). A radiologist with 6 years of experience manually examined the segmentation results. Subsequently, the diagnosis was confirmed by another radiologist with 20 years of experience. After confirming the segmentation results, the lesion area CT image was analyzed by open-source software (PyRadiomics 3.0.1) (26), and 952 radiological features (including tumor size, shape, first-order statistics of descriptor values, and high-order texture features) were extracted.
The radiographic features were evaluated independently by two experienced radiologists, as mentioned previously. Any discrepancies in interpretation between the observers were resolved through consensus to ensure agreement. The radiological features of each lesion were documented and analyzed, including (I) margin (clear, blurred); (II) lobulation (absent, present); (III) spiculation (absent, present); (IV) pleural attachment, including pleural tag and indentation (absent, present); (V) air bronchogram (absent, present); (VI) vessel change (absent, present); (VII) bubble lucency (absent, present); and (VIII) nodule location (left/right lobe, right upper lobe, right middle lobe, right lower lobe, left upper lobe, and left lower lobe). Finally, 963 features were extracted from CT images of the lesions, including 11 radiological features and 952 radiomics signatures.
Feature selection
First, we calculated the variance of all radiomics signatures and removed features with values less than 1 (using the “VarianceThreshold” method in the “sklearn.feature_selection” package in the Python 3.7 environment). Then, the correlation strength of the remaining radiological features was calculated, and the features with an absolute value greater than 0.9 were removed. In addition, the radiological features were assigned classification characteristics. The structure of each feature was considered too sparse if it was represented by a one-hot code. To merge the two types of features and reduce the impact of imaging features on the overall feature set, we used the ‘leaveoneoutencoder’ method (in the ‘category_encoders’ package) to convert classified data into numerical data. To further address the problem of collinearity between features, a subset of features that were more relevant to the target was obtained. The minimum redundancy maximum relevance (mRMR) method was employed to identify the 100 most relevant features from the dataset.
Dataset build
The dataset created based on the optimal 100 features was divided into a training cohort (80%) and a testing cohort (20%). We optimized the dataset to solve the problem of few data and increase the generalization ability of the trained model. Each sample in the training cohort was arranged in reverse order to double the amplification. Then, the data from each sample in the training cohort and testing set were supplemented to 25 rows (under the assumption that the CT image of each lesion had 25 layers), and the gap was filled with 0 s. Then, 30% of the columns of the samples in the training cohort were randomly selected and increased (multiplied by 1.3) or decreased (multiplied by 0.7). Next, the training cohort was doubled again. Finally, the optimized dataset was formed using radiomics signatures and radiological features.
Model structure
We proposed a Bi-LSTM and multihead attention-based model that was capable of classifying MIA, AIS, and IAC. Recurrent neural networks (RNNs) exhibit dynamic temporal behavior for a time sequence, as the connections between nodes form a directed graph along the sequence. The continuous CT images covering the lesion area are similar to the time-series data, where the time interval is represented by the slice thickness. Moreover, RNNs use an internal state to process input sequences, and they can connect previous information to the present task (27). Therefore, we hypothesized that features form “time series” in continuous CT layers. Compared with LSTM, Bi-LSTM performs well not only in long-distance information acquisition but also in near-position information acquisition. By training the model based on the Bi-LSTM algorithm to capture the long- and short-distance information rules in the sequence, it can better carry out an auxiliary diagnosis. Multihead attention mainly addresses three issues: (I) solving the problem that the model pays too much attention to the position of information itself; (II) enhancing the expression ability of the model; and (III) preventing overfitting. Both methods have open-source code and encapsulation packages. Multihead attention can use the “MultiHeadAttention” function in the “keras_multi_head” package. Bi-LSTM can use the combined “Bidirectional” and “LSTM” functions in the “tensorflow. keras.layers” package.
First, the model used the Bi-LSTM layer (units =32, activation = ‘sigmoid’) to extract the changing state of features in continuous CT layers as much as possible. Then, the multihead attention layer (head =8, name = ‘Multi-Head’ in the ‘keras_multi_head’ package) was used to enhance the output state sequence. This layer could enhance the generalization ability of the model and reduce the risk of focusing on specific feature changes. Then, the flattened layer was used to flatten the sequence. Finally, a series of FC layers and dropout layers was used to output the prediction of classification results (Figure S1). The workflow of this Bi-LSTM and multihead attention-based model is shown in Figure 2. In addition, we also studied the performance of datasets in CNN+LSTM and LSTM+multihead attention models. The overall process of the project is shown in Figure S2.
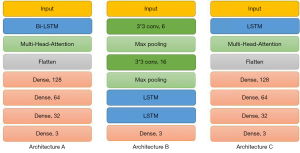
Identification evaluation and comparison with other algorithms
Several evaluation criteria were utilized to evaluate the model’s performance, including precision, recall, f1 score, and accuracy, as shown in Table 2 (28-30). These metrics were calculated based on the number of true positives (TP), false positives (FP), true negatives (TN), and false negatives (FN), following established methods. In addition, we conducted tenfold cross-validation experiments to further verify the performance of the model.
Table 2
Models | Precision | Recall | F1 | Accuracy |
---|---|---|---|---|
Bi-LSTM+ multihead attention in training cohort | 0.95 | 0.94 | 0.94 | 0.94 |
Bi-LSTM+ multihead attention in testing cohort | 0.92 | 0.92 | 0.92 | 0.92 |
Bi-LSTM+ multihead attention in validation cohort | 0.91 | 0.91 | 0.91 | 0.91 |
CNN+LSTM in testing cohort | 0.89 | 0.84 | 0.86 | 0.88 |
LSTM+ multihead attention in testing cohort | 0.88 | 0.87 | 0.86 | 0.87 |
Bi-LSTM, bidirectional long short-term memory; CNN, convolutional neural network.
We also evaluated the classification performance of the CNN+LSTM and LSTM+multihead attention methods after training on the same dataset. CNN+LSTM is a popular image feature analysis algorithm. In this experiment, we took the feature matrix of the lesion image group as an image and compared it with the change rules of lesion features to verify the model performance. The LSTM algorithm can also analyze the changes in lesion radiomics signatures. Here, we used it to test the performance of Bi-LSTM in this model. To test the performance difference between our model and the traditional method, we also extracted the 3D radiomic signatures of the lesions and built a nomogram based on the 3D radiomic signatures and radiological features (Figure S2). To further analyze the auxiliary diagnosis performance of the model, we compared its results to those of the manual diagnosis of radiologists in the same field. One study collected disease images similar to ours. The model built in the study and the results of artificial diagnosis by three radiologists were used as reference values (31). We also compared our model with another study that developed a combined radiographic–radiomics model for invasive prediction (32).
Results
Model training and performance
The categorical cross-entropy loss function was employed to calculate the loss during model training, with the Adam optimization algorithm utilized with a learning rate of 0.001. After considering the balance between accuracy and overfitting, we selected the model trained at the 400th epoch as the final model, as depicted in Figure 3. The ROC curve was plotted to assess the model’s performance, as shown in Figure 4. The model training demonstrated robust identification efficiency, as evident from the results obtained (training AUC =0.985; testing AUC =0.981; validation AUC =0.976). The four evaluation indicators were 0.95, 0.94, 0.94 and 0.94 in the training cohort; 0.92, 0.92, 0.92 and 0.92 in the testing cohort; and 0.91, 0.91, 0.91 and 0.91 in the validation cohort. The calibration plot of the model and the results of the tenfold cross-validation experiment are shown in the supplementary materials (Figures S3,S4, and Table S1).
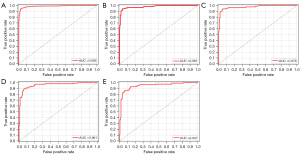
Performance of other models and artificial diagnosis
We trained the other two models and compared their performance in the testing cohort. The AUC of the CNN+LSTM model was 0.961, and its four evaluation indicators were 0.89, 0.84, 0.86 and 0.88. The AUC of the LSTM+multihead attention model was 0.947, and its four evaluation indicators were 0.88, 0.87, 0.86 and 0.87. The comparison results showed that the model developed in this study performed better than the other two in the same dataset (Table 2). The AUC of the nomogram model in the testing cohort was 0.864. The AUCs of the artificial diagnosis results of the three radiologists in another study were 0.692, 0.806 and 0.759, respectively (Figure S5). In this study, a nomograph model based on the GLCM was constructed. The AUC in the training cohort was 0.878, and that in the validation cohort was 0.923. The AUC of the combined radiographic–radiomics model was 0.80 in the training cohort, 0.77 in the validation cohort, and 0.82 in the testing cohort.
Discussion
The analysis of imaging features has been widely used in the auxiliary diagnosis of lung cancer, and accurate auxiliary models can provide positive support for making decisions about treatment plans and saving medical resources (33-35). The analysis of feature change trends may be closer to the diagnostic process of radiologists and may provide a new method for the exploration of decision rules (36,37).
In this study, we developed a deep learning network architecture specifically designed for feature learning and implemented the model for the classification of MIA, AIS, and IAC lung adenocarcinoma subtypes. Based on Bi-LSTM, the change rules of selected radiomics signatures in the continuous layers of CT images of lesions were accurately learned. At the same time, multihead attention was used to strengthen the learning ability of the change rule sequence so that the model parameters had better generalization ability and overfitting was reduced. This approach is consistent with routine radiologist film reading and diagnostic procedures. It can effectively reduce the complexity of the model and increase the interpretability of the model results. The results show that the model based on multihead attention and Bi-LSTM achieved good performance in the classification of lung adenocarcinoma CT images. This indicates that the change rule of radiological features in CT images can well reflect the type of focus. Our model was superior to three comparison models in the same dataset and has certain advantages compared with other research models. In addition, comparison with the performance of three radiologists in another study shows that the model has good clinical auxiliary value.
Compared with nomograms, these methods obtain good results, and they can provide an interpretable scoring standard. However, they still lack the ability to identify disease subtypes. When radiologists observe medical images such as CT images, they look for diagnostic evidence by analyzing the image texture changes and feature changes in the focus area between layers to identify disease subtypes. Most of the current auxiliary diagnostic models for medical images train and learn on a single image or a whole 3D focus area. Most of these methods can obtain good results after training, but they lack the ability to interpret models and results, which is the focus of recent research.
In our research, we referred to the working methods of radiologists, analyzed and learned the texture changes between the focus image layers, and combined the imaging features to train the auxiliary diagnosis model. This is an innovative attempt, although there are still some defects in the interpretation ability. Compared with other models based on a single image or 3D region, we focused on the idea of image texture changes, which provides a feasible direction for later research on interpretable auxiliary diagnostic models. Analyzing the radiologic features and their changing rules and even mapping the signs of the lesions help to interpret the images and provide an interpretable auxiliary diagnostic basis.
We used semiautomatic segmentation software developed in house to extract the lesion area, calculate the radiologic features through the open source ‘PyRadiomics’ software package, and finally combine the features to create the model trained in the study. In this way, a feasible and robust workflow is constructed that can assist radiologists in their daily work and has higher advantages compared with the results of manual diagnosis. After locating the focus area, doctors can quickly obtain the segmented focus area and the auxiliary diagnosis evaluation provided by the model through this workflow.
Of the top 10 features (lobulation, original_firstorder_90Percentile, pleural attachment, spiculation, air bronchogram, margin, bubble lucency, square_glcm_ClusterProminence, square_glrlm_LongRunEmphasis, and wavelet-HL_firstorder_Energy), 60% were radiological features, and 40% were radiomics signatures. The “original_firstorder_90Percentile” feature refers to the calculated value representing the 90th percentile of the CT values within the lesion. The “square_glcm_ClusterProminence” feature is a measure of the skewness and asymmetry of the gray level cooccurrence matrix (GLCM) features. The “square_glrlm_LongRunEmphasis” feature is a measure of the distribution of long run lengths, with a greater value indicative of longer run lengths and more coarse structural textures. Although CT values were not used as a factor in the model, all these features provide a detailed description of the CT value distribution and its trends in the lesion area. This indicates that radiological features are still important criteria for the diagnosis of lung adenocarcinoma. At the same time, radiomics signatures supplement the basis of diagnosis results from more high-dimensional perspectives, such as morphology and grayscale. The comparison with the results of other models shows that, compared with a single feature, the feature change rules can better assist radiologists in diagnosis. This may provide a new method for radiologic features to participate in auxiliary diagnosis.
However, this study has some deficiencies. First, the dataset used in this study is small, which may affect the feature extraction and value setting during the transformation of radiological classification features. In addition, there are certain fluctuations in model training. However, this model classifies the lesions by analyzing the change rules of each feature, so the difference in these values will not have a great impact on the results. In addition, the study also has limitations. First, it is undeniable that the extraction of radiologic features is affected by many aspects, such as different computing software packages and inconsistent extracted lesion regions. These problems reduce the robustness of the model and affect the results of the auxiliary diagnosis. Second, although the study has preliminarily verified that the analysis of feature change trends can identify lesions well, there is still a large gap among the realization of the provision of a clinical auxiliary diagnosis basis, the discovery of decision rules and interpretability. Third, the research team has analyzed the image feature changes of the lesion image. Although it is convenient for the subsequent extraction of decision rules, these artificial intervention processes may cause the rules to deviate from the facts. In the future, we will expand the dataset and look for more radiomics signature calculation packages that can calculate more features to reflect the focus as comprehensively as possible. In addition, we will also analyze the relationship between features, especially the relationship between radiological features and radiomics signatures, and optimize the feature set to enhance the interpretability of the model and results.
Conclusions
In this study, a model based on Bi-LSTM and multihead attention was developed to classify CT images of lung adenocarcinoma by analyzing the change rules of radiological and radiomics features in CT images of lesions. The results showed that the analysis of the changes in radiomics signatures in the CT images of the lesions had a positive effect on the differentiation of lung adenocarcinoma subtypes. This method provides a new method for CT image classification of lesions and has a certain clinical value.
Acknowledgments
Funding: The study was supported by the Science and Technology Commission of Shanghai Municipality (No. 22S31904600).
Footnote
Reporting Checklist: The authors have completed the TRIPOD reporting checklist. Available at https://qims.amegroups.com/article/view/10.21037/qims-22-848/rc
Conflicts of Interest: All authors have completed the ICMJE uniform disclosure form (available at https://qims.amegroups.com/article/view/10.21037/qims-22-848/coif). The authors have no conflicts of interest to declare.
Ethical Statement: The authors are accountable for all aspects of the work in ensuring that questions related to the accuracy or integrity of any part of the work are appropriately investigated and resolved. This study was conducted in accordance with the Declaration of Helsinki (as revised in 2013). The study was approved by the ethics committee of Shanghai University of Medicine & Health Sciences, and the written informed consent of patients was waived by the ethics committee because the study was a retrospective experiment and did not involve patient privacy.
Open Access Statement: This is an Open Access article distributed in accordance with the Creative Commons Attribution-NonCommercial-NoDerivs 4.0 International License (CC BY-NC-ND 4.0), which permits the non-commercial replication and distribution of the article with the strict proviso that no changes or edits are made and the original work is properly cited (including links to both the formal publication through the relevant DOI and the license). See: https://creativecommons.org/licenses/by-nc-nd/4.0/.
References
- Hoy H, Lynch T, Beck M. Surgical Treatment of Lung Cancer. Crit Care Nurs Clin North Am 2019;31:303-13. [Crossref] [PubMed]
- Casal-Mouriño A, Valdés L, Barros-Dios JM, Ruano-Ravina A. Lung cancer survival among never smokers. Cancer Lett 2019;451:142-9. [Crossref] [PubMed]
- Kuhn E, Morbini P, Cancellieri A, Damiani S, Cavazza A, Comin CE. Adenocarcinoma classification: patterns and prognosis. Pathologica 2018;110:5-11. [PubMed]
- Li X, Zhang W, Yu Y, Zhang G, Zhou L, Wu Z, Liu B. CT features and quantitative analysis of subsolid nodule lung adenocarcinoma for pathological classification prediction. BMC Cancer 2020;20:60. [Crossref] [PubMed]
- Travis WD, Brambilla E, Nicholson AG, Yatabe Y, Austin JHM, Beasley MB, Chirieac LR, Dacic S, Duhig E, Flieder DB, Geisinger K, Hirsch FR, Ishikawa Y, Kerr KM, Noguchi M, Pelosi G, Powell CA, Tsao MS, Wistuba I. The 2015 World Health Organization Classification of Lung Tumors: Impact of Genetic, Clinical and Radiologic Advances Since the 2004 Classification. J Thorac Oncol 2015;10:1243-60. [Crossref] [PubMed]
- Zhang P, Li T, Tao X, Jin X, Zhao S. HRCT features between lepidic-predominant type and other pathological subtypes in early-stage invasive pulmonary adenocarcinoma appearing as a ground-glass nodule. BMC Cancer 2021;21:1124. [Crossref] [PubMed]
- Wen Z, Zhao Y, Fu F, Hu H, Sun Y, Zhang Y, Chen H. Comparison of outcomes following segmentectomy or lobectomy for patients with clinical N0 invasive lung adenocarcinoma of 2 cm or less in diameter. J Cancer Res Clin Oncol 2020;146:1603-13. [Crossref] [PubMed]
- Liu S, Wang R, Zhang Y, Li Y, Cheng C, Pan Y, Xiang J, Zhang Y, Chen H, Sun Y. Precise Diagnosis of Intraoperative Frozen Section Is an Effective Method to Guide Resection Strategy for Peripheral Small-Sized Lung Adenocarcinoma. J Clin Oncol 2016;34:307-13. [Crossref] [PubMed]
- Jiang B, Takashima S, Miyake C, Hakucho T, Takahashi Y, Morimoto D, Numasaki H, Nakanishi K, Tomita Y, Higashiyama M. Thin-section CT findings in peripheral lung cancer of 3 cm or smaller: are there any characteristic features for predicting tumor histology or do they depend only on tumor size? Acta Radiol 2014;55:302-8. [Crossref] [PubMed]
- Behera M, Owonikoko TK, Gal AA, Steuer CE, Kim S, Pillai RN, Khuri FR, Ramalingam SS, Sica GL. Lung Adenocarcinoma Staging Using the 2011 IASLC/ATS/ERS Classification: A Pooled Analysis of Adenocarcinoma In Situ and Minimally Invasive Adenocarcinoma. Clin Lung Cancer 2016;17:e57-64. [Crossref] [PubMed]
- Wu FZ, Huang YL, Wu YJ, Tang EK, Wu MT, Chen CS, Lin YP. Prognostic effect of implementation of the mass low-dose computed tomography lung cancer screening program: a hospital-based cohort study. Eur J Cancer Prev 2020;29:445-51. [Crossref] [PubMed]
- Wu FZ, Huang YL, Wu CC, Tang EK, Chen CS, Mar GY, Yen Y, Wu MT. Assessment of Selection Criteria for Low-Dose Lung Screening CT Among Asian Ethnic Groups in Taiwan: From Mass Screening to Specific Risk-Based Screening for Non-Smoker Lung Cancer. Clin Lung Cancer 2016;17:e45-56. [Crossref] [PubMed]
- Gao W, Wen CP, Wu A, Welch HG. Association of Computed Tomographic Screening Promotion With Lung Cancer Overdiagnosis Among Asian Women. JAMA Intern Med 2022;182:283-90. [Crossref] [PubMed]
- Shi L, Shi W, Peng X, Zhan Y, Zhou L, Wang Y, Feng M, Zhao J, Shan F, Liu L. Development and Validation a Nomogram Incorporating CT Radiomics Signatures and Radiological Features for Differentiating Invasive Adenocarcinoma From Adenocarcinoma In Situ and Minimally Invasive Adenocarcinoma Presenting as Ground-Glass Nodules Measuring 5-10mm in Diameter. Front Oncol 2021;11:618677. [Crossref] [PubMed]
- She Y, Zhang L, Zhu H, Dai C, Xie D, Xie H, Zhang W, Zhao L, Zou L, Fei K, Sun X, Chen C. The predictive value of CT-based radiomics in differentiating indolent from invasive lung adenocarcinoma in patients with pulmonary nodules. Eur Radiol 2018;28:5121-8. [Crossref] [PubMed]
- Zhang J, Liu M, Liu D, Li X, Lin M, Tan Y, Luo Y, Zeng X, Yu H, Shen H, Wang X, Liu L, Tan Y, Zhang J. Low-dose CT with tin filter combined with iterative metal artefact reduction for guiding lung biopsy. Quant Imaging Med Surg 2022;12:1359-71. [Crossref] [PubMed]
- Yang CC, Chen CY, Kuo YT, Ko CC, Wu WJ, Liang CH, Yun CH, Huang WM. Radiomics for the Prediction of Response to Antifibrotic Treatment in Patients with Idiopathic Pulmonary Fibrosis: A Pilot Study. Diagnostics (Basel) 2022;12:1002. [Crossref] [PubMed]
- Aslan MF, Unlersen MF, Sabanci K, Durdu A. CNN-based transfer learning-BiLSTM network: A novel approach for COVID-19 infection detection. Appl Soft Comput 2021;98:106912. [Crossref] [PubMed]
- Li Y, Wang G, Li M, Li J, Shi L, Li J. Application of CT images in the diagnosis of lung cancer based on finite mixed model. Saudi J Biol Sci 2020;27:1073-9. [Crossref] [PubMed]
- Wu YJ, Wu FZ, Yang SC, Tang EK, Liang CH. Radiomics in Early Lung Cancer Diagnosis: From Diagnosis to Clinical Decision Support and Education. Diagnostics (Basel) 2022;12:1064. [Crossref] [PubMed]
- Faruqui N, Yousuf MA, Whaiduzzaman M, Azad AKM, Barros A, Moni MA. LungNet: A hybrid deep-CNN model for lung cancer diagnosis using CT and wearable sensor-based medical IoT data. Comput Biol Med 2021;139:104961. [Crossref] [PubMed]
- Feng J, Jiang J. Deep Learning-Based Chest CT Image Features in Diagnosis of Lung Cancer. Comput Math Methods Med 2022;2022:4153211. [Crossref] [PubMed]
- Hu X, Yang J, Yang J. A CNN-Based Approach for Lung 3D-CT Registration. IEEE Access. 2020;8:192835-192843. [Crossref]
- Bayoudh K, Hamdaoui F, Mtibaa A. Hybrid-COVID: a novel hybrid 2D/3D CNN based on cross-domain adaptation approach for COVID-19 screening from chest X-ray images. Phys Eng Sci Med 2020;43:1415-31. [Crossref] [PubMed]
- Ren H, Zhou L, Liu G, Peng X, Shi W, Xu H, Shan F, Liu L. An unsupervised semi-automated pulmonary nodule segmentation method based on enhanced region growing. Quant Imaging Med Surg 2020;10:233-42. [Crossref] [PubMed]
- van Griethuysen JJM, Fedorov A, Parmar C, Hosny A, Aucoin N, Narayan V, Beets-Tan RGH, Fillion-Robin JC, Pieper S, Aerts HJWL. Computational Radiomics System to Decode the Radiographic Phenotype. Cancer Res 2017;77:e104-7. [Crossref] [PubMed]
- Marentakis P, Karaiskos P, Kouloulias V, Kelekis N, Argentos S, Oikonomopoulos N, Loukas C. Lung cancer histology classification from CT images based on radiomics and deep learning models. Med Biol Eng Comput 2021;59:215-26. [Crossref] [PubMed]
- Hung TNK, Le NQK, Le NH, Van Tuan L, Nguyen TP, Thi C, Kang JH. An AI-based Prediction Model for Drug-drug Interactions in Osteoporosis and Paget's Diseases from SMILES. Mol Inform 2022;41:e2100264. [Crossref] [PubMed]
- Le NQK, Ho QT, Nguyen VN, Chang JS. BERT-Promoter: An improved sequence-based predictor of DNA promoter using BERT pre-trained model and SHAP feature selection. Comput Biol Chem 2022;99:107732. [Crossref] [PubMed]
- Lam LHT, Do DT, Diep DTN, Nguyet DLN, Truong QD, Tri TT, Thanh HN, Le NQK. Molecular subtype classification of low-grade gliomas using magnetic resonance imaging-based radiomics and machine learning. NMR Biomed 2022;35:e4792. [Crossref] [PubMed]
- Wu YJ, Liu YC, Liao CY, Tang EK, Wu FZ. A comparative study to evaluate CT-based semantic and radiomic features in preoperative diagnosis of invasive pulmonary adenocarcinomas manifesting as subsolid nodules. Sci Rep 2021;11:66. [Crossref] [PubMed]
- Sun Y, Li C, Jin L, Gao P, Zhao W, Ma W, Tan M, Wu W, Duan S, Shan Y, Li M. Radiomics for lung adenocarcinoma manifesting as pure ground-glass nodules: invasive prediction. Eur Radiol 2020;30:3650-9. [Crossref] [PubMed]
- Wu FZ, Wu YJ, Tang EK. An integrated nomogram combined semantic-radiomic features to predict invasive pulmonary adenocarcinomas in subjects with persistent subsolid nodules. Quant Imaging Med Surg 2023;13:654-68. [Crossref] [PubMed]
- Li W, Wang X, Zhang Y, Li X, Li Q, Ye Z. Radiomic analysis of pulmonary ground-glass opacity nodules for distinction of preinvasive lesions, invasive pulmonary adenocarcinoma and minimally invasive adenocarcinoma based on quantitative texture analysis of CT. Chin J Cancer Res 2018;30:415-24. [Crossref] [PubMed]
- Liang L, Zhang H, Lei H, Zhou H, Wu Y, Shen J. Diagnosis of Benign and Malignant Pulmonary Ground-Glass Nodules Using Computed Tomography Radiomics Parameters. Technol Cancer Res Treat 2022;21:15330338221119748. [Crossref] [PubMed]
- Wan YL, Wu PW, Huang PC, Tsay PK, Pan KT, Trang NN, Chuang WY, Wu CY, Lo SB. The Use of Artificial Intelligence in the Differentiation of Malignant and Benign Lung Nodules on Computed Tomograms Proven by Surgical Pathology. Cancers (Basel) 2020;12:2211. [Crossref] [PubMed]
- Luo C, Li S, Zhao Q, Ou Q, Huang W, Ruan G, Liang S, Liu L, Zhang Y, Li H. RuleFit-Based Nomogram Using Inflammatory Indicators for Predicting Survival in Nasopharyngeal Carcinoma, a Bi-Center Study. J Inflamm Res 2022;15:4803-15. [Crossref] [PubMed]