Renal ectopic lipid deposition in rats with early-stage diabetic nephropathy evaluated by the MR mDixon-Quant technique: association with the expression of SREBP-1 and PPARα in renal tissue
Introduction
As one of the most common microvascular complications of diabetes mellitus (DM), diabetic nephropathy (DN) is the leading cause of end-stage renal failure (1,2). Previous studies have shown that lipid metabolism disorders, including hyperlipemia and renal ectopic lipid deposition (ELD), play a significant role in the occurrence and development of DN. Excess adipokines from renal ectopic lipid deposits activate various signaling pathways, including those that result in oxidative stress, inflammation, fibrosis, and apoptosis, leading to renal cell damage and dysfunction. However, the underlying mechanisms and regulatory network of renal ELD are incompletely understood (3-5). Recently, growing evidence has indicated that the occurrence of renal ELD may be related to the imbalance of fatty acid uptake and oxidation in the kidney, which are regulated by lipid metabolism genes, such as sterol regulatory element binding protein 1 (SREBP-1) and peroxisome proliferator-activated receptor alpha (PPARα) (6-8). SREBP-1 and PPARα are the major transcription factors that regulate fatty acid synthesis and oxidation. Both type 1 and type 2 diabetic models show increased fatty acid synthesis and decreased fatty acid oxidation, as evidenced by upregulated expression of SREBP-1 and downregulation of PPARα, respectively (8). Targeting genes involved in renal lipid metabolism may serve as an effective therapy for DN treatment. Thus, quantification of renal lipid content and the expression of SREBP-1 and PPARα in renal tissues may be critical to investigate the underlying mechanisms of renal ELD and to monitor the therapeutic efficacy of gene therapy or other treatments that may contribute to regulating renal lipid metabolism in DN.
Renal biopsy is the reference standard for the evaluation of renal lipid metabolism disorder; however, its clinical application is restricted by invasiveness, potential complications, and sampling error. Meanwhile, there is a lack of an available clinical biomarker. A reliable and noninvasive method is needed. In recent years, several magnetic resonance imaging (MRI) techniques have been used to quantify the tissue fat concentration in vivo, mainly including magnetic resonance spectroscopy (MRS) and the mDixon-Quant technique (9,10). MRS is generally considered the most accepted method. However, due to technical difficulties in both performance and evaluation, it has not achieved widespread use in routine clinical practice (11). The magnetic resonance (MR) mDixon-Quant technique, a multiecho water lipid separation technique, is a promising method that has been demonstrated to exhibit good correlation with both MRS and biopsy for quantifying the fat fraction (FF) in the liver and other tissues (12,13). Compared to MRS, it can be easily added to the clinical protocol, and the parameter FF is highly stable and accurate among different readers, field strengths and MRI platforms (14). Now, this technique has been widely used to detect, quantify, and monitor hepatic, pancreatic, and vertebral fat content (14-16), but it has rarely been used in the evaluation of renal ELD. Yokoo et al. (17) and Wang et al. (18) demonstrated that renal lipid content can be quantified noninvasively using Dixon-based MRI in patients with type 2 DM (T2DM) or early-stage DN. However, due to the limitations of clinical research, the investigators only compared renal lipid deposition in patients with and without T2DM or in patients with and without early DN. A pathological comparison between the lipid component measured by the MR sequence and the actual degree of renal lipid deposition was not described. Furthermore, a change in renal ELD in the progression of DN, as well as the correlations between FF values and the expression of lipid metabolism genes, are not yet clear. A rat model of DN induced by streptozotocin (STZ) is a valuable tool for identifying the molecular mechanisms of the disease and for the preclinical development of new therapeutic strategies (19).
Hence, this study aimed to evaluate renal ELD in a rat model of STZ-induced early-stage DN using the mDixon-Quant technique and to determine the correlations between FF and the expression of SREBP-1 and PPARα in renal tissue, which could help us to better understand and manage renal lipid metabolism disorder in DN. We present this article in accordance with the ARRIVE reporting checklist (available at https://qims.amegroups.com/article/view/10.21037/qims-22-1167/rc).
Methods
Animal preparation
Experiments were performed under a project license (No. NSMC202199) granted by the institutional committee board of North Sichuan Medical College in compliance with our institutional guidelines for the care and use of animals. A total of 70 healthy Sprague-Dawley (SD) rats at 6 weeks of age (weighing between 160 and 200 g), which had not been subjected to other experiments before this research, were provided and maintained by our animal research institute under standard conditions with ad libitum access to food and water. The animals were housed individually, handled daily, and kept in a controlled environment with temperature of 20±2 ℃, humidity of 60%, and photoperiod of 12 h light-12 h dark (lights on from 07:00 to 19:00).
As a reasonable animal model of T2DM, a high-fat diet-fed, STZ-induced rat model was established in this study (20). Due to the approximate 70% incidence of T2DM and 40% mortality of uncontrolled T2DM in the experimental group of our preliminary experiment, the number of rats in the experimental group should be more than twice as many as that in the control group. Hence, after acclimatization for one week, the 70 rats were randomly divided into an experimental group (n=50) and a control group (n=20) in accordance with the random number table. To establish the T2DM model, the rats in the experimental group were fed a high-fat diet (containing 10% saccharose, 10% fat and 5% cholesterol) in the first 6 weeks. At the end of the 6th week, they were induced by an intraperitoneal injection of freshly constituted 1% STZ (Sigma-Aldrich, USA) dispersed in cold sodium citrate buffer (pH 4.5, Solarbio, China) at a dose of 35 mg/kg after fasting for 12 hours. Blood glucose was measured from tail vein samples at 72 hours after the injection of STZ. Rats with random blood glucose levels greater than 16.7 mmol/L after STZ administration were diagnosed with T2DM and included in the study. For the rats with random blood glucose levels less than 16.7 mmol/L, an additional intraperitoneal injection of STZ (Sigma-Aldrich) at a dose of 20 mg/kg was administered, and rats with random blood glucose levels greater than 16.7 mmol/L at 72 hours after the additional administration were also included in the study. Otherwise, the rats were excluded.
After the T2DM model was established, the included rats were fed for 4–16 weeks, and some of them developed DN models according to the natural course of this disease. The rats in the control group were provided with a standard diet and received an equal volume of sodium citrate buffer (pH 4.5, Solarbio) via intraperitoneal injection at the end of the 6th week. Those with random blood glucose levels less than 16.7 mmol/L at 72 hours after injection were included and continued to feed for 4–16 weeks.
A total of 32 rats in the experimental group were successfully induced by STZ, and 20 rats in the control group were eligible for this study. Subsequently, the 52 rats in the two groups were randomly stratified into four subgroups, with 13 rats (8 experimental and 5 control) in each subgroup. A subgroup was randomly chosen for MR scans at the end of the 4th, 8th, 12th, and 16th weeks after the T2DM model was established.
MR protocol
After a 6-h fasting period, the rats were anesthetized by an intraperitoneal injection of 2% sodium pentobarbital (Solarbio) at 2.3 mL/kg weight. Then, they were scanned using a 3.0 T MRI (uMR790, United Imaging Medical Technology Co., Ltd., China) with a rat coil. The rats were placed in a prone and head advanced position during imaging acquisition.
Renal coronal images of the mDixon-Quant technique were acquired. The following scanning parameters were used (21,22): repetition time (TR), 21 ms; echo time 1 (TE1), 1.45 ms, 6 echoes with delta TE 1.61 ms; section thickness, 1.3 mm; overlap, 1.0 mm; band width, 125 kHz; number of excitations, 4; flip angle, 4°; field of view (FOV), 90×146 mm; matrix, 102×208.
Fast recovery fast spin-echo fat suppressed coronal T2-weighted imaging (T2WI) was obtained for anatomical reference. The scanning parameters were TR/TE of 4,815.5/96 ms; band width of 61 kHz; section thickness of 2.0 mm; overlap of 1.0 mm; FOV of 120×120 mm; matrix of 325×384.
Image analysis
All acquired mDixon-Quant images were analyzed on a postprocessing workstation (United Imaging, China). No image was excluded due to suboptimal imaging or coverage. FF maps were acquired automatically to calculate the FF values of the right kidneys, which have the least bulk susceptibility-induced artifacts from bowel loops and breathing motions, compared to the left kidney, on the basis of our experience. Using the T2-weighted images for reference, circular regions of interest (ROIs) were placed on the upper, middle, and lower parts of the right renal parenchyma from the FF map at the level of the renal hilum while avoiding the renal pelvic structure and areas of artifacts (23) (Figure 1). The size of each ROI was 20–30 pixels. Each part was measured three times, and the average of the 9 measurements was considered the final value.
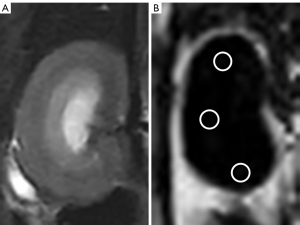
To test the interobserver concordance, two reviewers (the first and the fifth authors with 2 and 12 years of experience in abdominal radiology, respectively) who were blinded to the groups, the blood lipid parameters and the renal histopathological results of the rats performed the measurements. Furthermore, the first reviewer (the first author) repeated the measurements on the 180th day after the first measurement to test the intraobserver concordance.
Blood lipid parameters and renal histology
After the MR scan, the rats were kept in narcosis. Rat blood samples were collected from the central vein. Serum triglyceride (TG), total cholesterol (TC), low-density lipoprotein (LDL) and high-density lipoprotein (HDL) were assayed with corresponding reagent kits (Jisibiology, China).
Saline solution was then injected to perfuse the kidneys of each examined rat via the abdominal aorta until the kidneys were free of blood. The right kidney was obtained and cut into 3 equal pieces. One piece was fixed in 4% paraformaldehyde and embedded in paraffin. Multiple 3-µm-thick slices were obtained, and hematoxylin and eosin and periodic acid-Schiff staining were performed to observe the renal histopathological changes. One piece was processed as frozen sections, which were stained with oil red O to evaluate the renal fat distribution. The last piece of the kidney was immediately stored in the freezer at −80 degrees for Western blot assay to explore the expression of SREBP-1 and PPARα. After the collection of renal tissue samples, the rat was executed by air embolization.
For Western blot assays, renal tissues were lysed in RIPA lysis buffer (Sigma-Aldrich). Bicinchoninic acid assays (Sigma-Aldrich) were used to measure protein concentrations. Equal masses of protein were separated by sodium dodecyl sulfate-polyacrylamide gel electrophoresis and electrotransferred to polyvinylidene fluoride membranes (Beyotime Biotechnology, China). The membranes were sealed with Tris-buffered saline (Sangon Biotech, China) containing 5% nonfat milk for one hour, incubated overnight with primary antibodies against SREBP-1 (1:1,000 dilution, Affinity, USA), PPARα (1:1,000 dilution, Abcam, Britain), and glyceraldehyde-3-phosphate dehydrogenase (GAPDH) (1:1,000 dilution, HuaBio Biotechnology, China) at 4 ℃, and then incubated with horseradish peroxidase-labeled secondary antibodies (1:1,000, Beyotime Biotechnology, China) at room temperature for one hour. The band density was observed by a gel electrophoresis imaging system (Tanon-4200, Tanon, China). GAPDH was used as the internal control.
All renal tissue samples were analyzed in consensus by two reviewers skilled in the field of renal pathology (the first and fifth authors) with the Image-Pro Plus image analyzer version 6.0 for Windows (Media Cybernetics, Maryland, USA). The investigators were blinded to the group of rats, the results of MRI analysis, and the laboratory parameters.
Based on the histopathological changes in glomeruli and renal tubules, DN was diagnosed and stratified into four classes (24). DN with class I and II is defined as early-stage DN. According to the results of the renal histopathology, the enrolled rats were classified into two groups: an early-stage DN group and a nondiabetic control group.
Intracellular lipid deposition was observed by oil red O staining. The ratio of integrated optical density of the intracellular lipid droplets and the total area of all the cells (IOD/TAC) was calculated to assess the actual degree of renal lipid deposition. A larger ratio corresponds with more lipid accumulation in the kidney.
Statistical analyses
Statistical analyses were performed with the Statistical Package for Social Sciences version 26.0. A P value less than 0.05 was considered to indicate a statistically significant difference. All the data are presented as the mean ± standard deviation.
The inter- and intraobserver concordances in renal FF measurements were evaluated with the interclass correlation coefficient (ICC). If the concordance was good, the first measurements obtained by the first reviewer were used as the final values for the subsequent analysis. If not, the mean values of the three measurements were considered the final analysis values. Independent-samples t-tests were performed for comparisons between two samples, and analysis of variance (ANOVA) or Kruskal-Wallis tests were carried out for comparisons among three or more samples. Linear regression and correlation analyses were used to evaluate the associations between FF and blood lipid parameters, IOD/TAC, and the expression of SREBP-1 and PPARα.
Results
General characterization of the model
Some rats died of uncontrolled T2DM or natural causes during the 4–16 weeks after the T2DM model was established. The numbers of animal deaths in the subgroups are displayed in Table 1. After excluding them, 32 surviving rats were enrolled, which were composed of 15 rats in the control group and 17 in the experimental group. According to the renal histopathological appearances (Table 1), the rats in the experimental group were all diagnosed with early-stage DN with class I (n=3) and II (n=14), which were all included in the early-stage DN group, while for the rats in the control group, no lesions were found in the renal glomeruli and tubules. The differences in the serum TC, TG, HDL, and LDL levels between rats in the control and early-stage DN groups were significant (all P values <0.001) (Table 2). The differences in the serum TC, TG, HDL, and LDL levels among the early-stage DN subgroups were statistically significant (P=0.001, P=0.001, P=0.001, and P<0.001, respectively) (Table 3).
Table 1
Subgroups | Number of dead* (n) | Surviving number (n) | Renal histology findings of the surviving rats | ||||
---|---|---|---|---|---|---|---|
Normal (n) | Class I (n) | Class II (n) | Class III (n) | Class IV (n) | |||
Control subgroups | |||||||
4th week (n=5) | 0 | 5 | 5 | 0 | 0 | 0 | 0 |
8th week (n=5) | 2 | 3 | 3 | 0 | 0 | 0 | 0 |
12th week (n=5) | 1 | 4 | 4 | 0 | 0 | 0 | 0 |
16th week (n=5) | 2 | 3 | 3 | 0 | 0 | 0 | 0 |
Total (n=20) | 5 | 15 | 15 | 0 | 0 | 0 | 0 |
Experimental subgroups | |||||||
4th week (n=8) | 4 | 4 | 0 | 2 | 2 | 0 | 0 |
8th week (n=8) | 3 | 5 | 0 | 1 | 4 | 0 | 0 |
12th week (n=8) | 4 | 4 | 0 | 0 | 4 | 0 | 0 |
16th week (n=8) | 4 | 4 | 0 | 0 | 4 | 0 | 0 |
Total (n=32) | 15 | 17 | 0 | 3 | 14 | 0 | 0 |
*, number of dead indicates the number of rats which died from uncontrolled T2DM or natural causes. T2DM, type 2 diabetes mellitus; DN, diabetic nephropathy.
Table 2
Parameters | Control group (n=15) | Early-stage DN group (n=17) | P value |
---|---|---|---|
FF (%) | 3.33±0.23 | 4.45±0.70 | <0.001 |
IOD/TAC | 0.019±0.002 | 0.071±0.024 | <0.001 |
SREBP-1/GAPDH | 0.36±0.04 | 0.57±0.10 | <0.001 |
PPARα/GAPDH | 0.58±0.06 | 0.35±0.10 | <0.001 |
TC (mmol/L) | 1.87±0.08 | 2.53±0.53 | <0.001 |
TG (mmol/L) | 0.82±0.04 | 1.06±0.18 | <0.001 |
HDL (mmol/L) | 0.63±0.02 | 0.46±0.10 | <0.001 |
LDL (mmol/L) | 0.28±0.03 | 0.43±0.10 | <0.001 |
Data are presented as mean ± standard deviation. FF, fat fraction; DN, diabetic nephropathy; IOD/TAC, the ratio of integrated optical density of the intracellular lipid droplets and the total area of all the cells; SREBP-1, sterol regulatory element binding protein 1; GAPDH, glyceraldehyde-3-phosphate dehydrogenase; PPARɑ, peroxisome proliferator-activated receptor alpha; TC, total cholesterol; TG, triglyceride; HDL, high-density lipoprotein; LDL, low-density lipoprotein.
Table 3
Early-stage DN subgroups | TC (mmol/L) | TG (mmol/L) | HDL (mmol/L) | LDL (mmol/L) | IOD/TAC | SREBP-1/GAPDH | PPARα/GAPDH | FF (%) |
---|---|---|---|---|---|---|---|---|
4th week (n=4) | 1.90±0.12 | 0.87±0.07 | 0.58±0.09 | 0.32±0.07 | 0.037±0.002 | 0.50±0.02 | 0.40±0.01 | 3.77±0.14 |
8th week (n=5) | 2.25±0.30 | 0.95±0.07 | 0.52±0.05 | 0.38±0.03 | 0.060±0.007 | 0.54±0.07 | 0.39±0.08 | 4.01±0.24 |
12th week (n=4) | 2.89±0.34 | 1.13±0.11 | 0.39±0.02 | 0.41±0.04 | 0.078±0.007 | 0.55±0.06 | 0.39±0.01 | 4.62±0.67 |
16th week (n=4) | 3.14±0.31 | 1.28±0.16 | 0.32±0.07 | 0.57±0.06 | 0.105±0.005 | 0.71±0.10 | 0.17±0.03 | 5.42±0.43 |
P value | 0.001 | 0.001 | 0.001 | <0.001 | <0.001 | 0.009 | <0.001 | 0.002 |
Data are presented as mean ± standard deviation. FF, fat fraction; T2DM, type 2 diabetes mellitus; DN, diabetic nephropathy; TC, total cholesterol; TG, triglyceride; HDL, high-density lipoprotein; LDL, low-density lipoprotein; IOD/TAC, the ratio of integrated optical density of the intracellular lipid droplets and the total area of all the cells; SREBP-1, sterol regulatory element binding protein 1; GAPDH, glyceraldehyde-3-phosphate dehydrogenase; PPARα, peroxisome proliferator-activated receptor alpha.
Inter- and intraobserver agreement
In all the enrolled subjects, the mean renal FF values obtained by the two reviewers were 3.93%±0.23% and 3.99%±0.83%, and the repeated measurement by the first reviewer achieved a mean value of 3.84%±0.81%. The inter- and intraobserver concordances of the measurements were excellent [ICC values =0.942 (95% CI: 0.885–0.971) and 0.935 (95% CI: 0.869–0.968), respectively, with both P values <0.001], and the first measurements obtained by the first reviewer were used as the final values for further analysis.
Renal FF values in different groups
The renal FF value in the early-stage DN group was higher than that in the control group (P<0.001) (Table 2). Additionally, the difference in the FF values among the early-stage DN subgroups was statistically significant (P=0.002), and it gradually increased with the progression of disease [r=0.810 (95% CI: 0.675–0.928), P<0.001] (Table 3).
Renal fat distribution and the expression of SREBP-1 and PPARα
The results of oil red O staining showed that in the early-stage DN group, more lipids accumulated in renal cells, especially in renal tubular epithelial cells, than in the control group (P<0.001) (Table 2), and lipid accumulation increased gradually over time (P<0.001) (Table 3, Figure 2).
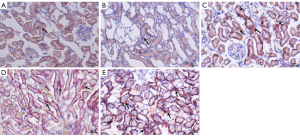
Western blot assays showed that the expression of SREBP-1 in renal tissue was significantly higher in the early-stage DN group than in the control group (P<0.001) and increased gradually over time (P=0.009), while the expression of PPARα was lower in the early-stage DN group than in the control subgroup (P<0.001) and decreased gradually over time (P<0.001) (Tables 2,3; Figure 3).
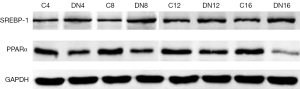
Correlations between renal FF and blood lipid parameters, IOD/TAC, and the expression of SREBP-1 and PPARα in renal tissue
The results of linear regression and correlation analysis showed that in all the included rats, there were positive correlations between FF and each of the following: TC, TG, LDL, IOD/TAC, and the expression of SREBP-1 [r=0.479 (95% CI: 0.353–0.640, P=0.012), 0.576 (95% CI: 0.283–0.842, P=0.002), 0.441 (95% CI: 0.305–0.606, P=0.021), 0.911 (95% CI: 0.809–0.964, P<0.001) and 0.800 (95% CI: 0.640–0.910, P<0.001), respectively]. Negative correlations were found between FF and each of the following: HDL and PPARα [r=−0.611 (95% CI: −0.809 to −0.469, P=0.001) and −0.748 (95% CI: −0.886 to −0.585, P<0.001), respectively]. The regression equations of FF and each of the following: IOD/TAC, SREBP-1 and PPARα were y = 0.400x − 0.096 (y = IOD/TAC, x = FF), y = 1.300x − 0.033 (y = SREBP/GAPDH, x = FF), and y = 0.987−1.300x (y = PPARα/GAPDH, x = FF), respectively.
Discussion
As a fat quantitative technology, the MR mDixon-Quant technique adopts the multiecho water lipid separation technique, thus generating water and fat images, in-phase and opposed-phase images, and FF maps. Compared to other MR methods, it can provide higher resolution and faster imaging (13,14). However, this technique has rarely been used in the evaluation of renal lipid content. In this study, the mDixon-Quant technique was used to quantify renal lipid deposition in an early-stage DN rat model. The results showed that the renal FF values obtained from the MR mDixon-Quant technique were excellently correlated with the histological fat quantification. Therefore, it is proven that the FF values could accurately reflect the lipid content of the kidneys, and that the MR mDixon-Quant technique could serve as a useful tool to accurately and efficiently quantify the renal lipid content for noninvasively monitoring renal lipid deposition in early-stage DN.
Meanwhile, our results showed that compared to the control group, the renal FF value in the early-stage DN group was higher, and the renal FF values in the early-stage DN subgroups gradually increased with the progression of disease. These findings indicated that excessive lipids accumulate in the kidneys of rats with early-stage DN, which is in agreement with previous literature (18,24), and that the renal lipid content increases with the progression of the disease. Additionally, the renal FF values increased in accordance with rising blood lipid levels, which also demonstrated that elevated serum lipid profiles could cause renal ectopic lipid accumulation. However, only a poor positive correlation between the two factors was shown in this study. This is likely because renal ectopic lipid accumulation is not only influenced by hyperlipidemia but also regulated by renal lipid metabolism (3,25).
The homeostasis of renal lipid metabolism is associated with both fatty acid oxidation (or fatty acid utilization) and fatty acid synthesis, which are regulated by lipid metabolism genes, such as SREBP-1 and PPARα (3,5). In subjects with early-stage DN, high glucose conditions activate SREBP-1 to stimulate fatty acid synthesis and downregulate PPARα to decrease fatty acid oxidation in renal cells. Dysregulation of SREBP-1 and PPARα plays an important role in renal lipid deposition. Positive correlations between renal FF values and the expression of SREBP-1 and negative correlations between FF and the expression of PPARα were found in our study. The renal lipid content increased with enhanced expression of SREBP-1 and the decrease in the expression of PPARα in renal tissue. Recently, growing research has indicated that targeting genes involved in renal lipid metabolism may serve as an effective therapy for DN treatment (1,3). Hence, an assessment of the expression of SREBP-1 and PPARα in renal tissue is crucial to understanding the mechanisms of lipid metabolism and to monitoring the therapeutic efficacy of gene therapy or other treatments with respect to the regulation of renal lipid metabolism in early-stage DN. However, the expression of SREBP-1 and PPARα in renal tissue is a type of histologic diagnosis based on biopsy or postoperative microscopic examination of surgical specimens. Noninvasive evaluation of these molecular targets in vivo is challenging. The regression equations of renal FF values and the expression of SREBP-1 and PPARα in renal tissue obtained in this study may provide a promising potential method based on the MR mDixon-Quant technique to noninvasively estimate the expression of SREBP-1 and PPARα in vivo.
There were three limitations in the current study. First, we only assessed the changes in renal lipid deposition in rats with early-stage DN, and a further longitudinal study based on the same rat model should be designed to evaluate the progression of renal lipid deposition in various stages of DN. Second, due to the high mortality of uncontrolled T2DM in this study, the sample size in every experimental subgroup was small. In the future, we will enlarge the sample size to confirm the findings. Third, the ROIs were manually drawn in the kidneys to measure the renal FF values, which may induce measurement error. However, we measured each part three times to reduce the possible error.
Conclusions
In conclusion, our study verified that renal lipid deposition evaluated by the MR mDixon-Quant technique is associated with the blood lipid level, histological fat quantification, and the expression of SREBP-1 and PPARα in renal tissue. The renal FF value might serve as a biomarker for better understanding the mechanism of renal lipid metabolism and monitoring the efficacy of gene therapy or other treatments for the regulation of renal lipid metabolism in early-stage DN.
Acknowledgments
Funding: This work was supported by the National Natural Science Foundation of China (No. 81541127) and the Nanchong-University Cooperative Research Project (No. 20SXQT0039).
Footnote
Reporting Checklist: The authors have completed the ARRIVE reporting checklist. Available at https://qims.amegroups.com/article/view/10.21037/qims-22-1167/rc
Conflicts of Interest: All authors have completed the ICMJE uniform disclosure form (available at https://qims.amegroups.com/article/view/10.21037/qims-22-1167/coif). All authors report that this work was supported by the National Natural Science Foundation of China (grant No. 81541127) and the Nanchong-University Cooperative Research Project (grant No. 20SXQT0039). The authors have no other conflicts of interest to declare.
Ethical Statement: The authors are accountable for all aspects of the work in ensuring that questions related to the accuracy or integrity of any part of the work are appropriately investigated and resolved. Experiments were performed under a project license (No. NSMC202199) granted by the institutional committee board of North Sichuan Medical College in compliance with our institutional guidelines for the care and use of animals.
Open Access Statement: This is an Open Access article distributed in accordance with the Creative Commons Attribution-NonCommercial-NoDerivs 4.0 International License (CC BY-NC-ND 4.0), which permits the non-commercial replication and distribution of the article with the strict proviso that no changes or edits are made and the original work is properly cited (including links to both the formal publication through the relevant DOI and the license). See: https://creativecommons.org/licenses/by-nc-nd/4.0/.
References
- A/L B Vasanth Rao VR. Tan SH, Candasamy M, Bhattamisra SK. Diabetic nephropathy: An update on pathogenesis and drug development. Diabetes Metab Syndr 2019;13:754-62. [Crossref] [PubMed]
- Umanath K, Lewis JB. Update on Diabetic Nephropathy: Core Curriculum 2018. Am J Kidney Dis 2018;71:884-95. [Crossref] [PubMed]
- Thongnak L, Pongchaidecha A, Lungkaphin A. Renal Lipid Metabolism and Lipotoxicity in Diabetes. Am J Med Sci 2020;359:84-99. [Crossref] [PubMed]
- Narindrarangkura P, Bosl W, Rangsin R, Hatthachote P. Prevalence of dyslipidemia associated with complications in diabetic patients: a nationwide study in Thailand. Lipids Health Dis 2019;18:90. [Crossref] [PubMed]
- Gai Z, Wang T, Visentin M, Kullak-Ublick GA, Fu X, Wang Z. Lipid Accumulation and Chronic Kidney Disease. Nutrients 2019;11:722. [Crossref] [PubMed]
- Shimano H, Sato R. SREBP-regulated lipid metabolism: convergent physiology - divergent pathophysiology. Nat Rev Endocrinol 2017;13:710-30. [Crossref] [PubMed]
- Dubois V, Eeckhoute J, Lefebvre P, Staels B. Distinct but complementary contributions of PPAR isotypes to energy homeostasis. J Clin Invest 2017;127:1202-14. [Crossref] [PubMed]
- Li L, Wang C, Yang H, Liu S, Lu Y, Fu P, Liu J. Metabolomics reveal mitochondrial and fatty acid metabolism disorders that contribute to the development of DKD in T2DM patients. Mol Biosyst 2017;13:2392-400. [Crossref] [PubMed]
- Pokharel SS, Macura KJ, Kamel IR, Zaheer A. Current MR imaging lipid detection techniques for diagnosis of lesions in the abdomen and pelvis. Radiographics 2013;33:681-702. [Crossref] [PubMed]
- Runge JH, Bakker PJ, Gaemers IC, Verheij J, Hakvoort TB, Ottenhoff R, Nederveen AJ, Stoker J. Measuring liver triglyceride content in mice: non-invasive magnetic resonance methods as an alternative to histopathology. MAGMA 2014;27:317-27. [Crossref] [PubMed]
- Manias KA, Peet A. What is MR spectroscopy? Arch Dis Child Educ Pract Ed 2018;103:213-6. [Crossref] [PubMed]
- Zhang Y, Wang C, Duanmu Y, Zhang C, Zhao W, Wang L, Cheng X, Veronese N, Guglielmi G. Comparison of CT and magnetic resonance mDIXON-Quant sequence in the diagnosis of mild hepatic steatosis. Br J Radiol 2018;91:20170587. [Crossref] [PubMed]
- Kukuk GM, Hittatiya K, Sprinkart AM, Eggers H, Gieseke J, Block W, Moeller P, Willinek WA, Spengler U, Trebicka J, Fischer HP, Schild HH, Träber F. Comparison between modified Dixon MRI techniques, MR spectroscopic relaxometry, and different histologic quantification methods in the assessment of hepatic steatosis. Eur Radiol 2015;25:2869-79. [Crossref] [PubMed]
- Serai SD, Dillman JR, Trout AT. Proton Density Fat Fraction Measurements at 1.5- and 3-T Hepatic MR Imaging: Same-Day Agreement among Readers and across Two Imager Manufacturers. Radiology 2017;284:244-54. [Crossref] [PubMed]
- Zhang QH, Zhao Y, Tian SF, Xie LH, Chen LH, Chen AL, Wang N, Song QW, Zhang HN, Xie LZ, Shen ZW, Liu AL. Hepatic fat quantification of magnetic resonance imaging whole-liver segmentation for assessing the severity of nonalcoholic fatty liver disease: comparison with a region of interest sampling method. Quant Imaging Med Surg 2021;11:2933-42. [Crossref] [PubMed]
- Ji X, Huang W, Dong H, Shen Z, Zheng M, Zou D, Shen W, Xia S. Evaluation of bone marrow infiltration in multiple myeloma using whole-body diffusion-weighted imaging and T1-weighted water-fat separation Dixon. Quant Imaging Med Surg 2021;11:641-51. [Crossref] [PubMed]
- Yokoo T, Clark HR, Pedrosa I, Yuan Q, Dimitrov I, Zhang Y, Lingvay I, Beg MS, Bobulescu IA. Quantification of renal steatosis in type II diabetes mellitus using dixon-based MRI. J Magn Reson Imaging 2016;44:1312-9. [Crossref] [PubMed]
- Wang YC, Feng Y, Lu CQ, Ju S. Renal fat fraction and diffusion tensor imaging in patients with early-stage diabetic nephropathy. Eur Radiol 2018;28:3326-34. [Crossref] [PubMed]
- Islam MS. Animal models of diabetic neuropathy: progress since 1960s. J Diabetes Res 2013;2013:149452. [Crossref] [PubMed]
- Skovsø S. Modeling type 2 diabetes in rats using high fat diet and streptozotocin. J Diabetes Investig 2014;5:349-58. [Crossref] [PubMed]
- Eggers H, Börnert P. Chemical shift encoding-based water-fat separation methods. J Magn Reson Imaging 2014;40:251-68. [Crossref] [PubMed]
- Yu H, McKenzie CA, Shimakawa A, Vu AT, Brau AC, Beatty PJ, Pineda AR, Brittain JH, Reeder SB. Multiecho reconstruction for simultaneous water-fat decomposition and T2* estimation. J Magn Reson Imaging 2007;26:1153-61. [Crossref] [PubMed]
- Gjela M, Askeland A, Frøkjær JB, Mellergaard M, Handberg A. MRI-based quantification of renal fat in obese individuals using different image analysis approaches. Abdom Radiol (NY) 2022;47:3546-53. [Crossref] [PubMed]
- Tervaert TW, Mooyaart AL, Amann K, Cohen AH, Cook HT, Drachenberg CB, Ferrario F, Fogo AB, Haas M, de Heer E, Joh K, Noël LH, Radhakrishnan J, Seshan SV, Bajema IM, Bruijn JARenal Pathology Society. Pathologic classification of diabetic nephropathy. J Am Soc Nephrol 2010;21:556-63. [Crossref] [PubMed]
- Tsun JG, Yung S, Chau MK, Shiu SW, Chan TM, Tan KC. Cellular cholesterol transport proteins in diabetic nephropathy. PLoS One 2014;9:e105787. [Crossref] [PubMed]