Differentiating angiomatous meningioma from atypical meningioma using histogram analysis of apparent diffusion coefficient maps
Introduction
Meningioma, with an incidence of about 36.7%, is the most frequent primary central nervous system (CNS) tumor in adults and is classified into three grades and 15 subtypes according to the 2021 World Health Organization (WHO) CNS tumor classification criteria (1-3). As two different histological subtypes of meningioma, there are significant differences in the biological behavior and treatment modalities between angiomatous meningioma (AM) and atypical meningioma (ATM) (3-6). ATM tumor cells proliferate actively and tend to infiltrate surrounding tissues, which is often difficult to achieve complete resection of the tumor during the operation (7). Therefore, surgical resection combined with radiotherapy or chemotherapy is the most commonly used treatment modality to reduce the high recurrence rates (8). The boundary between AM and normal brain tissue is always easily distinguished during the procedure, and a better prognosis can be achieved by complete surgical resection (3). Notably, the histological characteristics of the vascular-rich structural components make AM a high risk of bleeding during the operation, while the occurrence risk of intraoperative complications can be effectively prevented or reduced by preoperative embolization (5). Therefore, an accurate and effective preoperative distinction of AM and ATM is of great clinical importance for treatment decision-making and improved prognosis.
Conventional magnetic resonance imaging (MRI) features based on structural imaging are of limited value in preoperative differentiation of AM and ATM, and cannot achieve quantitative assessment of tumor biological behavior and microstructural changes (4,9). Diffusion-weighted imaging (DWI) is one of the most commonly used advanced MRI techniques to assess the heterogeneity of meningiomas, which could be a quantitative analysis using apparent diffusion coefficient (ADC) (10-13). Histogram analysis is an advanced medical image processing tool, which could provide more detailed information about tumor pathophysiology heterogeneity by analyzing the diffusion distribution and variation of image grayscale pixel value (12,14). Nowadays, ADC histogram analysis is applied widely in the field of brain tumor assessment, including grading, identification, and prognostic evaluation of brain tumors (11-16). Previous studies have shown that ADC histogram analysis effectively graded, typed, and assessed the prognosis of meningioma patients (15,16). Ki-67 is a nuclear antigen that reflects cell proliferation activity, which can be quantitatively assessed by the Ki-67 proliferation index and is of great significance for evaluating the biological behavior of tumors. Still, it can only be obtained by invasive methods (10,12).
To the best of our knowledge, up to now, no studies have investigated the utility of ADC histogram analysis in differentiating AM from ATM or testing the correlations between these parameters and the Ki-67 proliferation index. Thus, the goal of our study was to evaluate the diagnostic usefulness of ADC histogram analysis in identifying the two types of tumors, and further assess the correlations between ADC histogram parameters and the Ki-67 proliferation index.
Methods
Patient selection
The study was conducted in accordance with the Declaration of Helsinki (as revised in 2013). This study was approved by the Ethics Committee of Lanzhou University Second Hospital, and individual consent for this retrospective analysis was waived. From January 2017 to October 2022, 36 patients with AM and 49 patients with ATM were searched by reviewing medical records in our hospital. The inclusion criteria include: (I) a definitive postoperative pathology; (II) available preoperative brain MRI Images. The exclusion criteria include: (I) any intervention performed on the lesion before the MRI scan; (II) MRI image quality is poor and not meeting the needs of analysis. Five patients with AM and 9 patients with ATM were excluded due to the unavailability of ADC maps. One patient with AM and 3 patients with ATM was excluded due to the poor-quality images. Two ATM patients previously treated were excluded. Finally, thirty patients with AM and 35 patients with ATM were included in this study.
MRI acquisition
All scans were conducted using a 3.0 T (Siemens Verio, Erlangen, Germany) equipped with a 32-channel phased array coil. T1-weighted imaging (T1WI), T2WI, DWI, and contrast-enhanced T1WI sequences were acquired. The parameters were as follows: for T1WI sequence, repetition time (TR) =550 ms, echo time (TE) =11 ms, slice thickness =5.0 mm, interslice gap =1.5 mm, field of view (FOV) =260 mm × 260 mm, and matrix size =256×256; for T2WI sequence, TR =2,200 ms, TE =96 ms, slice thickness =5.0 mm, interslice gap =1.5 mm, FOV =260 mm × 260 mm, and matrix size =256×256; for DWI sequence, TR =4,000 ms, TE =100 ms, slice thickness =5.0 mm, interslice gap =1.5 mm, FOV =260 mm × 260 mm, and matrix size =256×256 (b-values =0 and 1,000 s/mm2). The contrast-enhanced T1WI sequence was acquired after the injection of the contrast agent gadopentetate dimeglumine (Bayer Schering Pharma AG, Berlin, Germany), with 0.1 mmol/kg at a flow rate of 3.0 mL/s via an intravenously administered bolus injection.
Image analysis
Conventional MRI features analysis
Two experienced neuroradiologists (radiologists 1 and 2, with 14 and 7 years of experience in diagnostic neuroradiology, respectively), blinded to relevant clinical and pathological information, independently reviewed all magnetic resonance (MR) images. The conventional MRI features, including location, lobulation, necrosis/cystic changes, peritumoral edema, tumor brain interface, dural tail sign, adjacent skull changes, vascular flow signal, and enhancement pattern were assessed and recorded (4,17). Any disputes were resolved in the consensus during the analysis process, and the results are used in the final analysis.
ADC histogram analysis
ADC maps were automatically generated from the DWI images by the Siemens Medical Systems workstation. With reference to TIWI, T2WI, and contrast-enhanced T1WI, the tumor boundaries were determined by two experienced neuroradiologists, then a freehand region of interest (ROI) (including the whole tumor tissue of each slice) was sketched on the T2WI images, and the ROIs were then copied to the corresponding sections of ADC maps (18). An open-source MaZda software (version 4.7, The Technical University of Lodz, Institute of Electronics, http://www.eletel.p.lodz.pl/mazda/) was applied to perform histogram analysis. Finally, nine ADC histogram parameters, including mean, variance, skewness, and kurtosis, as well as the 1st (ADC1), 10th (ADC10), 50th (ADC50), 90th (ADC90), and 99th (ADC99) percentile of ADC were automatically extracted and selected (18,19).
Pathological analysis
Pathological analysis was conducted by a pathologist (with 8 years of clinical experience), who was blinded to ADC histogram parameter measurements. Surgical tissue specimens were stained with hematoxylin and eosin. The Ki-67 proliferation index was determined by the number of positive cells labeled with the total cell count based on the area with the strongest positive cell nucleus.
Statistical analysis
All statistical analyses were performed using MedCalc software (Version 19.1, Mariakerke, Belgium). Interobserver agreements of the ADC histogram parameters were evaluated using the intraclass correlation coefficient (ICC). Categorical variables were analyzed by the chi-square test. Continuous variables are tested for normal distribution and expressed as mean ± standard deviation (independent t-test) or median (interquartile range) (Mann-Whitney U test). The area under the curve (AUC), sensitivity, specificity, accuracy, positive predictive value (PPV), and negative predictive value (NPV) were calculated by receiver operating characteristic (ROC) curves to conduct diagnostic performance analysis of significant ADC histogram parameters. The relationship between ADC histogram parameters and the Ki-67 proliferation index was assessed by Spearman’s correlation coefficient. Differences between AUCs were compared using Delong’s test. A P value less than 0.05 was considered statistical significance.
Results
Detailed demographic data of thirty AM and 35 ATM patients with are summarised in Table 1. The AM group included 13 men and 17 women (mean age: 52.27±12.21 years). The ATM group included 14 men and 21 women (mean age: 53.78±11.67 years). However, no significant differences were observed between the two groups in age or sex (P=0.786, P=0.616, respectively).
Table 1
Parameters | AM (n=30) | ATM (n=35) | P value |
---|---|---|---|
Age (years)a | 52.27±12.21 | 53.78±11.67 | 0.786 |
Sex (male/female), n | 13/17 | 14/21 | 0.616 |
Meanb | 124.07 (22.66) | 112.12 (16.04) | <0.001 |
Varianceb | 185.98 (132.01) | 229.65 (198.37) | 0.155 |
Skewnessa | 1.46±0.60 | 1.62±0.42 | 0.197 |
Kurtosisb | 3.76 (3.28) | 5.41 (4.02) | 0.067 |
ADC1b | 107.50 (17.00) | 82.00 (20.33) | <0.001 |
ADC10a | 115.80±12.09 | 96.86±9.86 | <0.001 |
ADC50b | 124.00 (21.13) | 109.00 (15.17) | <0.001 |
ADC90b | 134.08 (23.63) | 132.17 (17.20) | 0.099 |
ADC99b | 152.50 (27.75) | 155.00 (19.25) | 0.787 |
a, data are expressed as mean ± standard deviation and compared by independent t-test; b, data are expressed as median (interquartile range) and compared by Mann-Whitney U test. AM, angiomatous meningioma; ATM, atypical meningioma; ADC, apparent diffusion coefficient.
No significant differences were found between AM and ATM in terms of conventional MRI signatures (P=0.128–0.878), which were shown in Table 2.
Table 2
Parameters | AM (n=30) | ATM (n=35) | P |
---|---|---|---|
Location | 0.786 | ||
Falx or parasagittal | 11 | 12 | |
Convexity | 8 | 10 | |
Skull base | 7 | 5 | |
Posterior fossa | 3 | 5 | |
Others | 1 | 3 | |
Lobulation | 0.588 | ||
Yes | 16 | 21 | |
No | 14 | 14 | |
Necrosis/cystic changes | 0.128 | ||
Yes | 9 | 17 | |
No | 21 | 18 | |
Peritumoral edema | 0.878 | ||
Yes | 16 | 18 | |
No | 14 | 17 | |
Tumor brain interface | 0.229 | ||
Clear | 23 | 22 | |
Unclear | 7 | 13 | |
Dural tail sign | 0.780 | ||
Yes | 24 | 27 | |
No | 6 | 8 | |
Adjacent skull changes | 0.229 | ||
Yes | 7 | 13 | |
No | 23 | 22 | |
Vascular flow signal | 0.816 | ||
Yes | 18 | 20 | |
No | 12 | 15 | |
Enhancement pattern | 0.878 | ||
Inhomogeneous | 14 | 17 | |
Homogeneous | 16 | 18 |
AM, angiomatous meningioma; ATM, atypical meningioma; MRI, magnetic resonance imaging.
Excellent interobserver agreements were observed in the mean, variance, kurtosis, and all percentile ADC (ICC ranges, 0.767–0.985), while a moderate interobserver agreement was obtained from skewness (ICC, 0.740). Table 1 and Figure 1 demonstrate the comparative results of the ADC histogram parameters between the two groups of tumors. The AM group showed a significantly higher mean, ADC1, ADC10, and ADC50, compared to the ATM group (all P<0.05). Nevertheless, no differences were found in variance, skewness, kurtosis, ADC90, and ADC99 between AM and ATM (P=0.067–0.787). Figures 2,3 show the representative cases of AM and ATM, respectively.
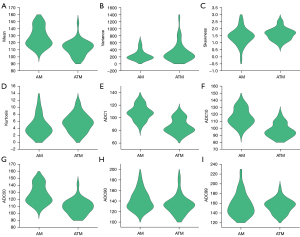
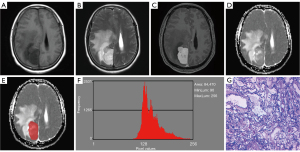
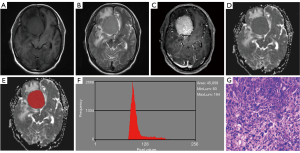
ROC analysis showed that the best diagnostic performance was achieved by ADC1, with the AUC, sensitivity, specificity, accuracy, PPV, and NPV were 0.900, 94.29%, 73.33%, 84.62%, 80.50%, and 91.70%, respectively, when using 103.00 as the optimal threshold (Table 3 and Figure 4). However, there were no differences in AUCs between significant ADC histogram parameters.
Table 3
Parameters | AUC (95% CI) | Cutt-off value | Sensitivity (%) | Specificity (%) | Accuracy (%) | PPV (%) | NPV (%) |
---|---|---|---|---|---|---|---|
Mean | 0.814 (0.698, 0.900) | 119.65 | 80.00 | 70.00 | 75.38 | 75.70 | 75.00 |
ADC1 | 0.900 (0.801, 0.961) | 103.00 | 94.29 | 73.33 | 84.62 | 80.50 | 91.70 |
ADC10 | 0.896 (0.795, 0.958) | 99.00 | 71.43 | 96.67 | 83.08 | 96.20 | 74.40 |
ADC50 | 0.868 (0.761, 0.939) | 114.00 | 71.43 | 90.00 | 80.00 | 89.30 | 73.00 |
AM, angiomatous meningioma; ATM, atypical meningioma; ADC, apparent diffusion coefficient; AUC, area under the receiver operating characteristic curve; CI, confidence intervals; PPV, positive predictive value; NPV, negative predictive value.
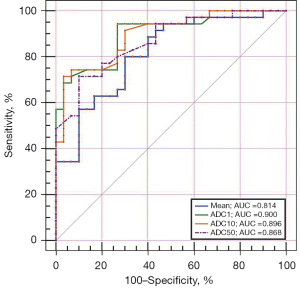
The AM group showed a significantly lower Ki-67 proliferation index than that in the ATM group (3.07%±2.31% vs. 10.51%±5.54%, P<0.05). Significant correlations were identified between the mean (r=−0.428, P<0.001), ADC1 (r=−0.549, P<0.001), ADC10 (r=−0.529, P<0.001), ADC50 (r=−0.483, P<0.001), and the Ki-67 proliferation index (Table 4).
Table 4
Parameters | Ki-67 proliferation index | |
---|---|---|
r | P | |
Mean | −0.428 | <0.001 |
ADC1 | −0.549 | <0.001 |
ADC10 | −0.529 | <0.001 |
ADC50 | −0.483 | <0.001 |
ADC, apparent diffusion coefficient; r, Spearman’s correlation coefficient.
Discussion
In this study, we compared the differences in ADC histogram parameters between AM and ATM, and further assess the correlations between these parameters and the Ki-67 proliferation index. The results suggest that some significant ADC histogram parameters could be useful to differentiate the two types of tumors before the operation, and a negative correlation could be observed between these parameters with the Ki-67 proliferation index, with ADC1 being identified as the most promising potential parameter. To date, this is the first study to evaluate the utility of ADC histogram analysis on the differentiation of AM and ATM.
Conventional MRI is an essential method in the evaluation of intracranial tumors. In this present study, no differences in conventional MRI features be detected between AM and ATM. Previous studies have shown that peritumor edema is an independent predictor to distinguish meningiomas of different pathological grades, and peritumor edema is more prone to appear in meningioma with higher aggression (4,20). In our study, no difference was observed in peritumor edema between the two types of tumor, which was probably caused by the unique histological characteristics of AM. Histologically, the histological features of the vascular-rich component can lead to overexpression of vascular endothelial growth factor (VEGF), which is closely related to the occurrence of peritumor brain edema, eventually resulting in AM exhibiting similar peritumoral edema to ATM (5,6). Moreover, the histological features of the vascular-rich components of AM may also contribute to the overlap of other conventional MRI features between the two types of tumors, which made it challenging to distinguish them (3,5,6). In addition, the number of patients included in this study was relatively small, which can also be another vital cause for the inability to distinguish between AM and ATM based on conventional MRI features.
DWI provides information about the tumor microenvironments such as tumor cell density, proliferation activity, and distribution by detecting the movement of water molecules, which can be quantitatively analyzed by ADC values (4,10,21). It has been shown that ADC values are more valuable in the differential diagnosis of meningiomas compared to several other advanced imaging techniques (22). ADC histogram analysis is one of the widely used methods in the field of brain tumor research, which could provide a variety of quantitative histogram analysis parameters (15-18,23). In this study, AM exhibits significantly higher mean, ADC1, ADC10, and ADC50 when compared with ATM, and a negative correlation can be observed between these ADC histogram parameters and the Ki-67 proliferation index. Meanwhile, each of these parameters was effective in distinguishing between the two histological types of tumors. This may be caused by the different biological behaviors and histological structure differences between AM and ATM. ATM tumor cells proliferate actively, increasing the number and the density of cells, the dense arrangement of tumor cells restricts the movement of water molecules and ultimately leads to a decrease in ADC values (11,16,24,25). Moreover, the tumor cell arrangement becomes sparse due to the vascular-rich component inside the AM, which may further increase the difference between ADC values in the two histological types of tumors (3,5,26). Gihr et al. (15) used ADC histogram analysis to identify high-grade meningiomas from low-grade meningiomas, and the results showed that there were significant differences in ADC histogram parameters between high-grade and low-grade meningiomas, with negative correlation observed between lower-level percentile of ADC and the Ki-67 proliferation index, which was in accordance with the findings of the current study. Ki-67 proliferation index is a pathological marker for quantitative assessment of cell proliferation activity, and tumors with high cell proliferation activity tend to exhibit high expression levels of the Ki-67 proliferation index (7,19). ADC values are imaging markers that reflect the proliferative activity of tumor cells, and a decrease in ADC values represents an increase in the proliferative activity of tumor cells (24,27). A negative correlation was observed between them, further suggesting that ADC values are reliable imaging biomarkers for preoperative quantitative assessment of tumor heterogeneity. However, Ota et al. reported that the ADCmean could not provide an effective evaluation of grade 1 and grade 2 meningiomas, which is inconsistent with our study results (28). This might be related to the different research methods of the study. Histogram analysis based on the whole tumor provides a comprehensive assessment of tumor heterogeneity and the quantitative parameters obtained to assess tumor heterogeneity are more accurate and objective. Of note, the relatively small sample size of the study may be also an influential factor in the results.
Meanwhile, this study also found that ADC1 not only had the strongest correlation with the Ki-67 proliferation index, but also was the best ADC histogram parameter for differentiating AM and ATM, with AUC being 0.900. A possible explanation is that the lower-level percentile of ADC provides a more accurate and objective assessment of tumor heterogeneity since they represent the most active regions of cell proliferation in tumor tissue (29,30). In this study, no difference was observed between ADC90 and ADC99, which may further indicate that the lower-level percentile of ADC is more helpful in the differentiation between AM and ATM. Xue et al. (19) evaluated the relationship between ADC histogram parameters and the Ki-67 proliferation index of pituitary macroadenomas and found that ADC1 had the strongest correlation with the expression status of pituitary macroadenoma Ki-67 proliferation index. Another study also demonstrated that the low-level percentile ADC shows the best differential diagnostic performance in discriminating intracranial solitary fibrous tumor/hemangiopericytoma from ATM (30). Given these similar results, a lower-level percentile of ADC perhaps is more recommended for the evaluation of brain tumors when performing ADC histogram analysis.
Variance, skewness, and kurtosis are also significant indicators of the quantitative assessment of tumor heterogeneity and play a vital role in the comprehensive evaluation of brain tumors (31-33). Higher values for variance, skewness, and kurtosis represent greater tumor complexity and heterogeneity. Bohara et al. (31) found a significant difference in the variance between high-grade meningiomas and low-grade meningiomas, which can effectively differentiate between them. Another study reported that both skewness and kurtosis are effective imaging biomarkers for the assessment of early treatment response of glioblastoma (33). Numerically, the variance, skewness, and kurtosis of ATM are greater than those of AM in this study, but there was no statistical significance among these parameters, which may be related to the relatively small sample size. However, to some extent, the differences in the values of these parameters can also indicate that the heterogeneity of ATM is significantly higher.
There are a few limitations in the present study. First, this was a single-center retrospective study with a small patient cohort. Second, manually plotting the ROI for ADC histogram analysis is relatively time-consuming in daily clinical practice. Therefore, future research should be conducted by expanding the sample size and uniting multiple clinical centers. In parallel, the new image automatic segmentation method should also be adopted.
Conclusions
In conclusion, our study illustrated that conventional MRI features between AM and ATM overlap. ADC histogram analysis may be a reliable method for preoperative of the two types of tumor, with the ADC1 being the most promising quantitative imaging biomarker, which can contribute to the formulation of individual treatment plans for patients.
Acknowledgments
Funding: This work was supported by the National Natural Science Foundation of China (Nos. 82071872, 82260341 and 82260361), the 2021 SKY Imaging Research Fund of China International Medical Exchange Foundation (No. Z-2014-07-2101), the Science and Technology Program of Gansu Province (No. 21YF5FA123), the Special fund project for the doctoral training program of Lanzhou University Second Hospital (No. YJS-BD-33), and the Excellent doctoral program of Gansu Province (No. 22JR5RA946).
Footnote
Conflicts of Interest: All authors have completed the ICMJE uniform disclosure form (available at https://qims.amegroups.com/article/view/10.21037/qims-22-1224/coif). The authors have no conflicts of interest to declare.
Ethical Statement: The authors are accountable for all aspects of the work in ensuring that questions related to the accuracy or integrity of any part of the work are appropriately investigated and resolved. The study was conducted in accordance with the Declaration of Helsinki (as revised in 2013). This study was approved by the Ethics Committee of Lanzhou University Second Hospital, and individual consent for this retrospective analysis was waived.
Open Access Statement: This is an Open Access article distributed in accordance with the Creative Commons Attribution-NonCommercial-NoDerivs 4.0 International License (CC BY-NC-ND 4.0), which permits the non-commercial replication and distribution of the article with the strict proviso that no changes or edits are made and the original work is properly cited (including links to both the formal publication through the relevant DOI and the license). See: https://creativecommons.org/licenses/by-nc-nd/4.0/.
References
- Louis DN, Perry A, Wesseling P, Brat DJ, Cree IA, Figarella-Branger D, Hawkins C, Ng HK, Pfister SM, Reifenberger G, Soffietti R, von Deimling A, Ellison DW. The 2021 WHO Classification of Tumors of the Central Nervous System: a summary. Neuro Oncol 2021;23:1231-51. [Crossref] [PubMed]
- Zhang J, Yao K, Liu P, Liu Z, Han T, Zhao Z, Cao Y, Zhang G, Zhang J, Tian J, Zhou J. A radiomics model for preoperative prediction of brain invasion in meningioma non-invasively based on MRI: A multicentre study. EBioMedicine 2020;58:102933. [Crossref] [PubMed]
- Verma PK, Nangarwal B, Verma J, Dwivedi V, Mehrotra A, Das KK, Maurya VP, Bhaisora KS, Singh J, Sardhara J, Srivastava AK, Behari S, Jaiswal S, Jaiswal AK. A clinico-pathological and neuro-radiological study of angiomatous meningioma: Aggressive look with benign behaviour. J Clin Neurosci 2021;83:43-8. [Crossref] [PubMed]
- Liu X, Wang Y, Wei J, Li S, Xue C, Deng J, Liu H, Sun Q, Zhang X, Zhou J. Role of diffusion-weighted imaging in differentiating angiomatous meningioma from atypical meningioma. Clin Neurol Neurosurg 2022;221:107406. [Crossref] [PubMed]
- Ben Nsir A, Chabaane M, Krifa H, Jeme H, Hattab N. Intracranial angiomatous meningiomas: A 15-year, multicenter study. Clin Neurol Neurosurg 2016;149:111-7. [Crossref] [PubMed]
- Hua L, Luan S, Li H, Zhu H, Tang H, Liu H, Chen X, Bozinov O, Xie Q, Gong Y. Angiomatous Meningiomas Have a Very Benign Outcome Despite Frequent Peritumoral Edema at Onset. World Neurosurg 2017;108:465-73. [Crossref] [PubMed]
- Buerki RA, Horbinski CM, Kruser T, Horowitz PM, James CD, Lukas RV. An overview of meningiomas. Future Oncol 2018;14:2161-77. [Crossref] [PubMed]
- Chun SW, Kim KM, Kim MS, Kang H, Dho YS, Seo Y, Kim JW, Kim YH, Park CK. Adjuvant radiotherapy versus observation following gross total resection for atypical meningioma: a systematic review and meta-analysis. Radiat Oncol 2021;16:34. [Crossref] [PubMed]
- Schwingel R, Reis F, Zanardi VA, Queiroz LS, França MC Jr. Central nervous system lymphoma: magnetic resonance imaging features at presentation. Arq Neuropsiquiatr 2012;70:97-101. [Crossref] [PubMed]
- Xianwang L, Lei H, Hong L, Juan D, Shenglin L, Caiqiang X, Yan H, Junlin Z. Apparent Diffusion Coefficient to Evaluate Adult Intracranial Ependymomas: Relationship to Ki-67 Proliferation Index. J Neuroimaging 2021;31:132-6. [Crossref] [PubMed]
- Gihr GA, Horvath-Rizea D, Hekeler E, Ganslandt O, Henkes H, Hoffmann KT, Scherlach C, Schob S. Histogram Analysis of Diffusion Weighted Imaging in Low-Grade Gliomas: in vivo Characterization of Tumor Architecture and Corresponding Neuropathology. Front Oncol 2020;10:206. [Crossref] [PubMed]
- Yang H, Liu X, Jiang J, Zhou J. Apparent diffusion coefficient histogram analysis to preoperative evaluate intracranial solitary fibrous tumor: Relationship to Ki-67 proliferation index. Clin Neurol Neurosurg 2022;220:107364. [Crossref] [PubMed]
- Bruvo M, Mahmood F. Apparent diffusion coefficient measurement of the parotid gland parenchyma. Quant Imaging Med Surg 2021;11:3812-29. [Crossref] [PubMed]
- Zeng F, Chen L, Lin L, Hu H, Li J, He P, Wang C, Xue Y. Iodine map histogram metrics in early-stage breast cancer: prediction of axillary lymph node metastasis status. Quant Imaging Med Surg 2022;12:5358-70. [Crossref] [PubMed]
- Gihr GA, Horvath-Rizea D, Garnov N, Kohlhof-Meinecke P, Ganslandt O, Henkes H, Meyer HJ, Hoffmann KT, Surov A, Schob S. Diffusion Profiling via a Histogram Approach Distinguishes Low-grade from High-grade Meningiomas, Can Reflect the Respective Proliferative Potential and Progesterone Receptor Status. Mol Imaging Biol 2018;20:632-40. [Crossref] [PubMed]
- Surov A, Ginat DT, Lim T, Cabada T, Baskan O, Schob S, Meyer HJ, Gihr GA, Horvath-Rizea D, Hamerla G, Hoffmann KT, Wienke A. Histogram Analysis Parameters Apparent Diffusion Coefficient for Distinguishing High and Low-Grade Meningiomas: A Multicenter Study. Transl Oncol 2018;11:1074-9. [Crossref] [PubMed]
- He W, Xiao X, Li X, Guo Y, Guo L, Liu X, Xu Y, Zhou J, Wu Y. Whole-tumor histogram analysis of apparent diffusion coefficient in differentiating intracranial solitary fibrous tumor/hemangiopericytoma from angiomatous meningioma. Eur J Radiol 2019;112:186-91. [Crossref] [PubMed]
- Liu X, Huang X, Han T, Li S, Xue C, Deng J, Zhou Q, Sun Q, Zhou J. Discrimination between microcystic meningioma and atypical meningioma using whole-lesion apparent diffusion coefficient histogram analysis. Clin Radiol 2022;77:864-9. [Crossref] [PubMed]
- Xue C, Liu S, Deng J, Liu X, Li S, Zhang P, Zhou J. Apparent Diffusion Coefficient Histogram Analysis for the Preoperative Evaluation of Ki-67 Expression in Pituitary Macroadenoma. Clin Neuroradiol 2022;32:269-76. [Crossref] [PubMed]
- Han T, Zhang J, Liu X, Zhang B, Deng L, Lin X, Jing M, Zhou J. Differentiating atypical meningioma from anaplastic meningioma using diffusion weighted imaging. Clin Imaging 2022;82:237-43. [Crossref] [PubMed]
- Meyer HJ, Wienke A, Surov A. ADC values of benign and high grade meningiomas and associations with tumor cellularity and proliferation - A systematic review and meta-analysis. J Neurol Sci 2020;415:116975. [Crossref] [PubMed]
- Ota Y, Liao E, Zhao R, Capizzano AA, Baba A, Lobo R, Shah G, Srinivasan A. Utility of dynamic susceptibility contrast MRI for differentiation between paragangliomas and meningiomas in the cerebellopontine angle and jugular foramen region. Clin Imaging 2023;96:49-55. [Crossref] [PubMed]
- Lin X, Lee M, Buck O, Woo KM, Zhang Z, Hatzoglou V, Omuro A, Arevalo-Perez J, Thomas AA, Huse J, Peck K, Holodny AI, Young RJ. Diagnostic Accuracy of T1-Weighted Dynamic Contrast-Enhanced-MRI and DWI-ADC for Differentiation of Glioblastoma and Primary CNS Lymphoma. AJNR Am J Neuroradiol 2017;38:485-91. [Crossref] [PubMed]
- Surov A, Hamerla G, Meyer HJ, Winter K, Schob S, Fiedler E. Whole lesion histogram analysis of meningiomas derived from ADC values. Correlation with several cellularity parameters, proliferation index KI 67, nucleic content, and membrane permeability. Magn Reson Imaging 2018;51:158-62. [Crossref] [PubMed]
- Ren J, Yuan Y, Wu Y, Tao X. Differentiation of orbital lymphoma and idiopathic orbital inflammatory pseudotumor: combined diagnostic value of conventional MRI and histogram analysis of ADC maps. BMC Med Imaging 2018;18:6. [Crossref] [PubMed]
- Filippi CG, Edgar MA, Uluğ AM, Prowda JC, Heier LA, Zimmerman RD. Appearance of meningiomas on diffusion-weighted images: correlating diffusion constants with histopathologic findings. AJNR Am J Neuroradiol 2001;22:65-72. [PubMed]
- Liu L, Yin B, Geng DY, Li Y, Zhang BY, Peng WJ. Comparison of ADC values of intracranial hemangiopericytomas and angiomatous and anaplastic meningiomas. J Neuroradiol 2014;41:188-94. [Crossref] [PubMed]
- Ota Y, Liao E, Capizzano AA, Yokota H, Baba A, Kurokawa R, Kurokawa M, Moritani T, Yoshii K, Srinivasan A. MR diffusion and dynamic-contrast enhanced imaging to distinguish meningioma, paraganglioma, and schwannoma in the cerebellopontine angle and jugular foramen. J Neuroimaging 2022;32:502-10. [Crossref] [PubMed]
- Donati OF, Mazaheri Y, Afaq A, Vargas HA, Zheng J, Moskowitz CS, Hricak H, Akin O. Prostate cancer aggressiveness: assessment with whole-lesion histogram analysis of the apparent diffusion coefficient. Radiology 2014;271:143-52. [Crossref] [PubMed]
- Liu X, Deng J, Sun Q, Xue C, Li S, Zhou Q, Huang X, Liu H, Zhou J. Differentiation of intracranial solitary fibrous tumor/hemangiopericytoma from atypical meningioma using apparent diffusion coefficient histogram analysis. Neurosurg Rev 2022;45:2449-56. [Crossref] [PubMed]
- Bohara M, Nakajo M, Kamimura K, Yoneyama T, Fukukura Y, Kiyao Y, Yonezawa H, Higa N, Kirishima M, Yoshiura T. Histological Grade of Meningioma: Prediction by Intravoxel Incoherent Motion Histogram Parameters. Acad Radiol 2020;27:342-53. [Crossref] [PubMed]
- de Perrot T, Lenoir V, Domingo Ayllón M, Dulguerov N, Pusztaszeri M, Becker M. Apparent Diffusion Coefficient Histograms of Human Papillomavirus-Positive and Human Papillomavirus-Negative Head and Neck Squamous Cell Carcinoma: Assessment of Tumor Heterogeneity and Comparison with Histopathology. AJNR Am J Neuroradiol 2017;38:2153-60. [Crossref] [PubMed]
- Baek HJ, Kim HS, Kim N, Choi YJ, Kim YJ. Percent change of perfusion skewness and kurtosis: a potential imaging biomarker for early treatment response in patients with newly diagnosed glioblastomas. Radiology 2012;264:834-43. [Crossref] [PubMed]