Assessment of hepatic function employing hepatocyte specific contrast agent concentrations to multifactorially evaluate fibrotic remodeling
Introduction
Diffuse parenchymal liver diseases are contributing substantially to global morbidity and represent major causes of deaths worldwide (1). Subsumed disease entities include non-alcoholic fatty liver disease (NAFLD) with a current prevalence of about 20–30% in western countries (2), alcoholic fatty liver disease (3) as well as viral hepatitis (4). Each of these liver disease entities may cause chronic parenchymal inflammation with resulting fibrotic remodeling, portal hypertension, cirrhosis, and ultimately higher likelihoods to progress into hepatocellular carcinoma (HCC) (5). Fortunately, studies demonstrated that early stages of parenchymal remodeling and fibrosis may be reversible (6). Thus, early detection of liver disease seems to be of utmost importance to prevent disease progression with irreversible parenchymal damage and potentially development of malignancy.
Despite its inherent invasiveness, sampling heterogeneity and resultant sampling errors, liver biopsy still represents the reference standard for diagnosis and staging of hepatic remodeling and fibrosis as early hallmark of chronic liver disease (7). Current non-invasive quantitative imaging methods to assess liver fibrosis include ultrasound or magnetic resonance (MR) elastography as well as MR relaxometry. While for ultrasound-based assessments the diagnostic accuracy is highly operator-dependent (8,9), MR elastography (10) and non-contrast MR T1 relaxometry (11) imaging, on the other hand, showed promising results in detecting and characterizing early liver fibrosis. One advantage of T1 mapping over elastography in this regard is a shorter preparation time for the patient and that additional equipment is not necessary.
In addition to the histological stage [according to meta-analysis of histological data in viral hepatitis (METAVIR)] (12), the functional status of the liver is clinically relevant. Scores that address this are, for example, Child-Pugh or model of end-stage liver disease (MELD) (13,14). Methods such as elastography or T1 mapping are able to detect even early histologic stages of fibrotic remodeling. However, changes of the liver function cannot be evaluated on non-contrast MR imaging (MRI). Liver function is mostly assessed through determination of blood laboratory values such as total bilirubin, albumin, creatinine and platelet concentrations (15-19). The pharmacokinetics of hepatobiliary contrast agents through utilization of membrane transporters are depending on overall hepatic function. Hepatocyte-specific MR contrast agents may therefore allow for both global and segmental liver function estimation (15-17). Therefore, hepatic MRI is a valuable tool for quantitative evaluation and monitoring of advanced liver diseases, showing good results in cirrhotic screening. Moreover, it enables the quantitative determination of fibrosis, iron overload and steatosis.
The purpose of this study was to combine established quantitative MR non-contrast methodologies of relaxometry, specifically hepatic fat and iron quantitation with evaluation of intrahepatic distribution and relaxometry-based quantification of hepatocyte-specific contrast material as surrogate for liver function estimation, in order to improve detection of chronic liver diseases using machine learning differentiation algorithms. We present this article in accordance with the STARD reporting checklist (available at https://qims.amegroups.com/article/view/10.21037/qims-22-884/rc).
Methods
This retrospective study was approved by the Ethics Committee of Northwest and Central Switzerland (No. BASEC 2020-00943) and in accordance with the Declaration of Helsinki (as revised in 2013). The requirement for written informed consent was waived due to the retrospective nature of the study.
Study population
The local radiology information system (RIS) was retrospectively and consecutively queried for abdominal MRI examinations performed between 07/2018–12/2019 for the following indications: (I) patients with newly diagnosed liver lesions of unknown origin, or (II) patients with abnormally elevated hepatic or biliary parameters without preexistent diffuse liver disease or (III) patients with known liver fibrosis or cirrhosis undergoing screening for HCC as well as a recent liver parenchyma biopsy within one month of the imaging window. Exclusion criteria were: (I) non-contrast imaging protocols or protocols without hepatocyte-specific contrast agents, (II) known systemic diseases with involvement of the liver, e.g. autoimmune hepatitis, (III) known metastatic liver disease, (IV) patients undergoing chemotherapy or immune modulating therapy, (V) patients with mechanical obstruction of the hepatobiliary tree as well as (VI) patients with laboratory results of elevated transaminases and bilirubin levels older than one month within the imaging window (Figure 1).
The final study sample consisted of 202 patients in three disease groups: 72 patients (mean age: 53±16 years, 32 men) without known proven liver disease, 21 patients (mean age: 65±15 years, 14 men) with incidentally detected elevated liver enzymes [aspartate aminotransferase (ASAT) >34 U/L or alanine aminotransferase (ALAT) >41 U/L or gamma-glutamyltransferase (GGT) >40 U/L or bilirubin >24 µmol/L] (acute liver disease group) and 109 patients (mean age: 61±11 years, 80 men) with biopsy proven liver fibrosis/cirrhosis (chronic liver disease group); severity of fibrosis was classified by using the histopathologic METAVIR score. The functional classification was graded through calculation of the Child-Pugh score based on clinical information from the electronic patient record.
MR parameters, acquisition and data assessment
All liver MR examinations were performed on one of two scanners systems: 133 liver imaging studies were acquired at 1.5 T (MAGNETOM Avanto FIT, Siemens Healthineers, Erlangen, Germany) and 69 studies at 3 T (MAGNETOM Skyra, Siemens Healthineers, Erlangen, Germany). Imaging protocols included the same sequences and were adapted for the different field strengths. Body phased-array coils of up to 48 channels were used for the examinations.
Prior to intravenous contrast administration, T1 maps were generated by using a single breath-hold axial Look-Locker approach [echo time (TE) =1.32 ms, repetition time (TR) =3 ms, acquisition time (TA) =0.7 s, 3 slices: slice thickness (ST) =8.5 mm, in plane resolution =1.15 mm × 1.15 mm, flip angle =8°, field of view 373×459 mm2]. Subsequently, parenchymal fat fraction and R2* values to detect hepatic iron overload were determined by using an axial whole-organ six-point gradient-echo Dixon T1 sequence (TE =1.26 ms, TR =9.26 ms, TA =17.4 s, 72 slices: ST =3 mm, in plane resolution =1.48 mm × 1.48 mm, flip angle =4°, field of view 308×380 mm2) (14). A fat-saturated T2-weighted sequence (TE =95 ms at 1.5 T, TE =152 ms at 3 T, TR =3,480 ms, TR =3,010 ms at 3 T, TA =88 s at 3 T, TA =134 s at 1.5 T; 35 slices: ST =5 mm, in plane resolution 1.4 mm × 1.4 mm, flip angle =160°, field of view 378×378 mm2) allowed assessment for presence or absence of ascites.
For intravenous contrast administration, a weight-adjusted bolus of hepatocyte-specific gadolinium ethoxybenzyl-diethylenetriaminepentaacetic acid (Gd-EOB-DTPA, 0.25 mmol/mL Primovist, Bayer AG, Leverkusen, Germany) was injected into the right antecubital vein. Patients weighing less than 50 kg received 7.5 mL of undiluted contrast media, patients weighing 50 to 100 kg received 10 mL of contrast media, and a fixed dose of 15 mL was administered if patient weight was above 100 kg. Analogously, whole-organ T1 maps were generated by using the same single breath-hold axial Look-Locker approach (TE =1.32 ms, TR =3 ms, 3 slices: ST =8.5 mm, in plane resolution =1.15 mm × 1.15 mm, flip angle =8°, field of view 373×459 mm2). Fifteen minutes post contrast administration.
Liver volume was evaluated via manual segmentation. Liver volume was calculated based on this segmentation. Data assessment was performed on a volume-of-interest (VOI) based segmentation of left and the right liver lobes, encompassing the entire lobar volume with exclusion of visible hepatic veins or arteries on unenhanced and delayed phase T1 maps, as well as fat fraction and R2* maps. Gd-concentrations in µmol/L were quantitated based on the calculated T1 maps and the relaxivity r1 at 1.5 T (6.9 L/µmol*s) and 3 T (6.2 L/µmol*s) (Figure 2) (18):
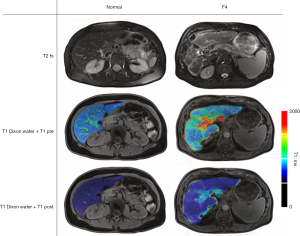
Fat fraction (in %) and R2* as a measure for hepatic iron overload (1/ms) were analogously extracted based on lobar segmentation VOIs. The same segmentations were used as for the T1 maps. Presence or absence of ascites was noted as dichotomous parameter. Fat fraction, R2* and T1 values were generated by using a commercial software (Siemens LiverLab) (19,20).
Statistical analyses
Statistical evaluation of the ability of hepatic function based on post-contrast relaxometry alone to predict presence and type of diffuse parenchymal disease used Wilcoxon signed-rank tests (α=0.05) for hypothesis testing and Spearman’s rho coefficient tests for correlation analyses with hepatobiliary phase hepatic gadolinium concentration (in µmol/L) as dependent variable and successively bilirubin levels (in µmol/L), METAVIR (chronic disease group only) and Child-Pugh scores (chronic disease group only) as independent variables following evaluation for normal distribution using Shapiro-Wilk-tests. Diagnostic accuracy was quantified through receiver operating characteristic (ROC)-curve analyses (21).
For statistical evaluation whether a multiparametric approach allows prediction of presence and type of diffuse parenchymal disease, a machine learning solution for data classification with multiparametric predictors was implemented in Matlab (R2019a, The MathWorks, Natick, United States). Data classification was performed with bootstrap aggregation to differentiate three classes: patients without known liver disease, patients with acute liver disease and patients with fibrosis or cirrhosis (22). A random undersampling boost tree ensemble classifier was used for differentiation of patients without known liver disease and those with METAVIR F1 fibrosis (23). Five-fold cross-validation was used for all models as a measure to evaluate the classification performance in small data sets. Field strength independent predicting factors used for the multiparametric approach were (I) patient age (in years); (II) hepatic fat fraction (in %); (III) presence of liver iron overload (R2* >39/ms at 1.5 T, R2* >69 ms at 3 T) (24); (IV) hepatobiliary phase parenchymal gadolinium concentrations in the left and the right liver lobe (in µmol/L) and (V) absence/presence of ascites. Analogously, diagnostic accuracy was quantified through ROC-curve analyses.
Statistical analyses were performed using commercially available software solutions (JMP v14, SAS Institute, North Carolina, United States); a P value of less than 0.05 was considered to be statistically significant.
Results
Disease spectrum in study population
Patients without liver disease were younger than patients with acute (P=0.007) or chronic (P=0.002) liver disease (Table 1).
Table 1
Characteristics | Disease group | ||
---|---|---|---|
No | Acute | Chronic | |
Gender (male/female) | 32/40 | 14/7 | 80/29 |
Age (years), mean ± SD | 53±16&,&& | 65±15& | 61±11&& |
[Gd] (µmol/L), mean ± SD | 0.52±0.19+,++ | 0.28±0.16+ | 0.31±0.12++ |
Fat fraction (%), mean ± SD | 7.1±5.3 | 6.8±6.7 | 7.9±6.0 |
Relaxation times R2* (ms−1), mean ± SD | 1.5 T (n=44): 37±13 | 1.5 T (n=12): 35±16 | 1.5 T (n=70): 37±14 |
3 T (n=28): 56±34 | 3 T (n=9): 43±23 | 3 T (n=39): 51±26 | |
[Bilirubin] (µmol/L), mean ± SD | 7.8±3.6#,## | 29.0±23.0# | 20.3±26.5## |
Pairwise significant differences are marked with: &, P=0.007 (no vs. acute); &&, P=0.002 (no vs. chronic); +, P<0.001 (no vs. acute); ++, P<0.001 (no vs. chronic); #, P<0.001 (no vs. acute); ##, P<0.001 (no vs. chronic). SD, standard deviation; [Gd], gadolinium concentration.
There were no differences for R2* between all three disease groups (P>0.05) (Table 1).
In contrast, the hepatic fat fraction of patients with mild liver fibrosis (METAVIR F1) was significantly higher compared to patients higher grades of liver fibrosis (METAVIR F2–F4) (Table 2).
Table 2
Characteristics | METAVIR | |||
---|---|---|---|---|
F1 | F2 | F3 | F4 | |
Gender (male/female) | 10/5 | 8/8 | 22/5 | 37/14 |
Age (years), mean ± SD | 63±5 | 63±15 | 57±13 | 62±10 |
[Gd] (µmol/L), mean ± SD | 0.39±0.14 | 0.33±0.12 | 0.30±0.15 | 0.28±0.10 |
Fat fraction (%), mean ± SD | 11.4±6.0& | 7.6±6.4& | 6.6±5.3& | 7.6±4.9& |
Relaxation times R2* (ms−1), mean ± SD | 1.5 T (n=9): 39±7 | 1.5 T (n=10): 44±14 | 1.5 T (n=18): 36±5 | 1.5 T (n=33): 35±17 |
3 T (n=6): 47±10 | 3 T (n=6): 39±13 | 3 T (n=9): 40±9 | 3 T (n=18): 57±31 | |
[Bilirubin] (µmol/L), mean ± SD | 11.0±3.1 | 17.2±14.0 | 18.7±11.0 | 19.7±15.2 |
Fat fraction was significant higher for patients with METAVIR F1 compared to F2–F4 (&, P<0.001). SD, standard deviation; [Gd], gadolinium concentration; METAVIR, meta-analysis of histological data in viral hepatitis.
Hepatic function based on post-contrast relaxometry alone predicting presence and type of diffuse parenchymal disease
Hepatobiliary phase gadolinium concentration was used as surrogate for hepatic function; mean delay of hepatobiliary phase MRI was 912±159 s.
Mean hepatobiliary phase hepatic parenchymal gadolinium concentration was significantly higher in patients without known liver disease (mean: 0.52±0.19 µmol/L) compared to patients with acute liver disease (mean: 0.28±0.16 µmol/L) or liver fibrosis/cirrhosis (mean: 0.31±0.12 µmol/L; P<0.001), while no significant difference in gadolinium concentration was seen between patients with acute liver disease and with fibrosis/cirrhosis (P=0.29) (Table 1, Figure 3). Specifically, mean hepatobiliary phase gadolinium concentration was higher in patients without known liver disease (mean: 0.52±0.19 µmol/L) than in subjects with METAVIR F2 (mean: 0.33±0.12 µmol/L, P=0.016), MEATVIR F3 (mean: 0.30±0.15 µmol/L, P=0.003) and METAVIR F4 liver fibrosis (mean: 0.28±0.10 µmol/L, P<0.001) (Table 2, Figure 3). Resultant moderate negative correlations between mean hepatobiliary phase parenchymal gadolinium concentration and the METAVIR stage of liver fibrosis (r=−0.44, P<0.001) were observed.
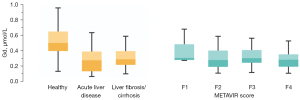
The highest diagnostic accuracy to differentiate patients without known liver disease from patients with liver fibrosis/cirrhosis was reached using a hepatobiliary phase hepatic gadolinium parenchymal concentration threshold of 0.42 µmol/L with an area under the curve (AUC)Linear regression of 0.73 (accuracy =0.74, sensitivity =0.74, specificity =0.63) in a monoparametric model.
Moderate negative correlations between mean hepatobiliary phase parenchymal gadolinium concentrations and the Child-Pugh stage of liver cirrhosis (r=−0.35, P<0.001) were observed; strong negative correlation between hepatobiliary phase hepatic parenchymal gadolinium concentrations and serum bilirubin levels were seen overall (r=−0.61, P<0.001), as well as within each subgroup (no proven liver disease: r=−0.38, P=0.009; acute liver disease: r=−0.51, P=0.02; fibrosis/cirrhosis: r=−0.38, P=0.002) (Figure 4). An overview of the values in the individual Child-Pugh stages is provided in Table 3.
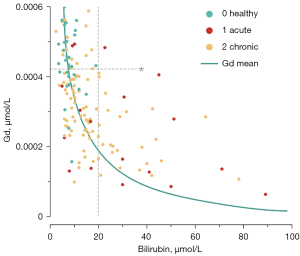
Table 3
Characteristics | Child-Pugh | ||
---|---|---|---|
A | B | C | |
Gender (male/female) | 45/22 | 23/11 | 5/3 |
Age (years), mean ± SD | 63±10 | 61±11 | 53±11 |
[Gd] (µmol/L), mean ± SD | 0.34±0.12 | 0.25±0.12 | 0.24±0.12 |
Fat fraction (%), mean ± SD | 8.3±6.3 | 7.0±4.8 | 10.0±9.3 |
Relaxation times R2* (1/ms), mean ± SD | 1.5 T (n=47): 36±6 | 1.5 T (n=18): 38±20 | 1.5 T (n=5): 34±6 |
3 T (n=20): 53±24 | 3 T (n=16): 52±24 | 3 T (n=3): 40±11 | |
[Bilirubin] (µmol/L), mean ± SD | 14.8±18* | 22.0±13 | 59.0±77* |
Bilirubin concentration was significant lower in patients with Child A compared to patients with Child C (*, P<0.001). SD, standard deviation; [Gd], gadolinium concentration.
Multiparametric approach predicting presence and type of diffuse parenchymal disease
Predictors incorporated into the multiparametric approach were hepatic fat fraction, patient age, presence of hepatic iron overload, parenchymal hepatobiliary phase hepatic gadolinium concentrations in left and right hepatic lobes as well as the occurrence of ascites.
Utilization of the multiparametric approach to differentiate patients without known liver disease from patients with known fibrosis/cirrhosis showed a better diagnostic performance than single-factor (hepatic function based on post-contrast relaxometry alone) linear regression when using the same hepatobiliary phase parenchymal gadolinium concentration threshold of 0.42 µmol/L (AUCMultiparametric =0.82 vs. AUCLinear regression =0.73; accuracyMultiparametric =0.76 vs. accuracyLinear regression =0.74, Table 4).
Table 4
Disease groups | Accuracy | AUC | Sensitivity | Specificity |
---|---|---|---|---|
No vs. acute vs. chronic | 0.65 | 0.79 | 0.77 | 0.69 |
No vs. chronic | 0.76 | 0.82 | 0.86 | 0.61 |
No vs. F1 | 0.74 | 0.84 | 0.75 | 0.70 |
Predicting factors used for the multiparametric approach were: (I) patient age; (II) hepatic fat fraction; (III) presence of liver iron overload quantification; (IV) hepatobiliary phase parenchymal gadolinium [concentration] and (V) presence of ascites. AUC, area under the curve.
When targeting differentiation of all three disease groups (no liver disease vs. acute liver disease vs. fibrosis/cirrhosis), an AUCMultiparametric =0.79 was reached. The models used failed to differentiate between the three disease groups by using hepatobiliary phase hepatic gadolinium concentrations alone.
For subgroup differentiation of patients without known liver disease and with mild fibrotic changes of the liver parenchyma (METAVIR F1), a random undersampling boost tree ensemble classifier yielded a diagnostic accuracy of 0.74 (AUCMultiparametric =0.84), as the four METAVIR groups could not be differentiated using hepatobiliary phase hepatic gadolinium concentration alone, a further differentiation between patients without histologically-proven liver disease and only F1 fibrosis was again not possible.
Discussion
The aim of our study was to investigate the combination of established quantitative MR non-contrast methodologies of relaxometry, specifically hepatic fat and iron quantitation with relaxometry-based quantification of hepatocyte-specific contrast material as surrogate for liver function (24,25). A multifactorially approach was used to assess the ability to classify different stages of fibrotic remodeling. While in general non-contrast T1 mapping has been known to predict fibrotic changes (11,26), recent investigations showed the additional value of contrast enhanced T1 mapping as a useful parameter of hepatocyte transporter function (27,28). In contrast to previously established methods, which predominantly use either native T1 mapping or calculation of the reduction rate, an advantage of our method is that a parameter is calculated that can be used both for the diagnosis of liver fibrosis or cirrhosis and as a surrogate parameter for liver function (29).
Our results confirm this letter observation by showing significantly lower hepatobiliary phase hepatic gadolinium parenchymal concentration in patients with acute liver disease as well as patients with known liver fibrosis compared to patients without known liver disease. As there were no significant differences observed in hepatobiliary phase hepatic gadolinium parenchymal concentrations in patients with acute elevation of hepatic or biliary parameters and patients with known fibrosis or cirrhosis, at least two mechanisms individually or conjointly appear to be responsible: for acute hepatic disease with substantially elevated bilirubin levels a reduced excretion of gadolinium into the biliary radicals has been observed as bilirubin and Gd-EOB-DTPA compete for the same hepatocyte transporter molecules; for chronic liver disease remodeling and transformation of hepatocytes and associated biliary radicals into thickened fibrotic membranes lead to overall less processing capabilities in patients with hepatic fibrosis or cirrhosis.
Clinical scores assessing hepatic function use blood laboratory values such as total bilirubin with a normal cutoff value of >20 µmol/L. However, the majority of the patients with either acute or chronic liver disease in our study showed bilirubin levels of <20 µmol/L, and according to the clinical scores would characterize the hepatic function to be normal (30-32). Our study showed that a hepatobiliary phase hepatic gadolinium parenchymal concentration threshold of 0.42 µmol/L differentiates patients with temporarily pathologically altered liver enzymes from patients without histopathologic confirmed liver disease with an accuracy of 0.73.
Differentiation of patients with fibrosis/cirrhosis from patients with temporary elevation of liver enzymes was not possible either multi- or mono-parametrically. Hence, temporary elevation of liver enzymes may be considered a confounding factor for the evaluation of liver fibrosis based on T1 relaxometry alone.
The diagnostic performance of the multiparametric approach with an AUC of 0.82, a sensitivity of 0.84 and a specificity of 0.68 when evaluating parenchymal fibrotic changes in a patient group with a METAVIR score of F1–F4 is within the results of previous studies using MRI elastography, which is still seen as the reference standard for non-invasive classification of liver fibrosis: for instance meta-analysis based on 697 patients by of Singh et al. in 2015 showed a mean AUC of 0.84 for all stages of fibrosis (0.73 sensitivity, 0.79 specificity) (33). Recent studies calculating the T1 reduction rate show even better results in some cases: for example, Li et al. showed that it was possible to differentiate between patients with and without liver cirrhosis and between different Child-Pugh stages with an AUC >0.95 (34). However, the patients without liver cirrhosis also suffered from chronic liver disease. Diagnostic performance is similar compared with other more recent studies that calculated T1 reduction rates: For example, Obmann et al. achieved an AUC of 0.83 for distinguishing patients with and without cirrhosis (29). In contrast to this study, we did not evaluate diagnostic performance to differentiate between different Child-Pugh stages but examined histological subgroups. For a focused subgroup differentiation of patients without known liver disease and with mild fibrotic changes of the liver parenchyma (METAVIR F1), a diagnostic accuracy of 0.74 (AUCMultiparametric =0.84) was reached in our study. Identification of this subgroup may be considered of utmost importance as the likelihood of fibrosis reversibility is the highest.
Lastly, a major advantage of contrast-enhanced T1 mapping in comparison to non-contrast T1 mapping is the independence of underlying magnetic field strength for the calculation of hepatobiliary phase hepatic gadolinium parenchymal concentrations and thresholds as reported in previous studies (27,28).
Our study had several limitations which have to be addressed. Primarily, it was a single center retrospective analysis with a relatively small study sample and different etiologies of liver cirrhosis. Secondarily, the relaxivity r1, which was fundamental for the calculation of hepatic Gd-EOB-DTPA concentrations, was chosen from the literature for blood plasma at 37 ℃, neglecting significant differences depending on the tissue, warming effects due to the MRI examination as well as the indoor climate and inhomogeneity of the B0 field (18,35). Therefore, effects of different scanners, warming during examination and the relaxivity of Gd-EOB-DTPA in hepatic tissue need to be investigated for future studies. The measurement of the hepatobiliary phase intrahepatic contrast agent concentration was performed about 15 minutes after contrast injection, which is within the hepatocyte phase (15,24,36), though identical delays in our study with resulting inter-patient differences could not be achieved due to the retrospective character of the study design. Further studies are needed to determine a correction factor and investigate the influence of the measurement time point. In addition, a comparison between the reduction rate and intrahepatic gadolinium concentration would be interesting in terms of diagnostic performance. Thirdly, due to the different respiratory positions in the T1 maps before and after contrast administration, the calculation of gadolinium concentrations is performed ROI-based and not voxel-wise. This limits the local information and necessitates the acquisition of more slices if necessary using breath-triggered gating. Last, a multiparametric approach using machine learning is always highly dependent on the choice of parameters used. We ultimately decided to use only image-based quantitative and non-quantitative parameters with the exception of patient age. Ultimately, the selection is arbitrary and the additional use of, for example, clinical parameters as input variables could lead to an improvement of the results. However, a disadvantage of these multiparametric analyses is that it is difficult to find causality for the results. For example, it is unclear why a multiparametric approach leads to an improvement in diagnostics. In the best case, this is due to a balanced selection of input parameters; in the worst case, it is due to differences in individual variables across (sub-)groups. We have tried to exclude this as best as possible and think that our additionally chosen input parameters are plausible. For example, the presence of ascites is definitely a known surrogate parameter for the (functional) severity of liver fibrosis. Optimally, this needs to be verified in much larger collectives and preferably a multicenter setting.
Conclusions
In summary, quantification of hepatic Gd-EOB-DTPA concentrations might provide an additional surrogate parameter for the liver function and might improve detection of fibrotic changes in a multiparametric approach. In patients receiving hepatocyte-specific contrast agents, an improved detection of chronic liver diseases might be achieved.
Acknowledgments
Funding: None.
Footnote
Reporting Checklist: The authors have completed the STARD reporting checklist. Available at https://qims.amegroups.com/article/view/10.21037/qims-22-884/rc
Conflicts of Interest: All authors have completed the ICMJE uniform disclosure form (available at https://qims.amegroups.com/article/view/10.21037/qims-22-884/coif). The authors have no conflicts of interest to declare.
Ethical Statement: The authors are accountable for all aspects of the work in ensuring that questions related to the accuracy or integrity of any part of the work are appropriately investigated and resolved. This retrospective study was approved by the Ethics Committee of Northwest and Central Switzerland (No. BASEC 2020-00943) and in accordance with the Declaration of Helsinki (as revised in 2013). The requirement for written informed consent was waived due to the retrospective nature of the study.
Open Access Statement: This is an Open Access article distributed in accordance with the Creative Commons Attribution-NonCommercial-NoDerivs 4.0 International License (CC BY-NC-ND 4.0), which permits the non-commercial replication and distribution of the article with the strict proviso that no changes or edits are made and the original work is properly cited (including links to both the formal publication through the relevant DOI and the license). See: https://creativecommons.org/licenses/by-nc-nd/4.0/.
References
- Lozano R, Naghavi M, Foreman K, Lim S, Shibuya K, Aboyans V, et al. Global and regional mortality from 235 causes of death for 20 age groups in 1990 and 2010: a systematic analysis for the Global Burden of Disease Study 2010. Lancet 2012;380:2095-128. [Crossref] [PubMed]
- Bellentani S, Scaglioni F, Marino M, Bedogni G. Epidemiology of non-alcoholic fatty liver disease. Dig Dis 2010;28:155-61. [Crossref] [PubMed]
- Rehm J, Samokhvalov AV, Shield KD. Global burden of alcoholic liver diseases. J Hepatol 2013;59:160-8. [Crossref] [PubMed]
- Stanaway JD, Flaxman AD, Naghavi M, Fitzmaurice C, Vos T, Abubakar I, et al. The global burden of viral hepatitis from 1990 to 2013: findings from the Global Burden of Disease Study 2013. Lancet 2016;388:1081-8. [Crossref] [PubMed]
- Bataller R, Brenner DA. Liver fibrosis. J Clin Invest 2005;115:209-18. [Crossref] [PubMed]
- Kumar M, Sarin SK. Is cirrhosis of the liver reversible? Indian J Pediatr 2007;74:393-9. [Crossref] [PubMed]
- Chalasani N, Younossi Z, Lavine JE, Diehl AM, Brunt EM, Cusi K, Charlton M, Sanyal AJ. The diagnosis and management of non-alcoholic fatty liver disease: practice Guideline by the American Association for the Study of Liver Diseases, American College of Gastroenterology, and the American Gastroenterological Association. Hepatology 2012;55:2005-23. [Crossref] [PubMed]
- Sandrin L, Fourquet B, Hasquenoph JM, Yon S, Fournier C, Mal F, Christidis C, Ziol M, Poulet B, Kazemi F, Beaugrand M, Palau R. Transient elastography: a new noninvasive method for assessment of hepatic fibrosis. Ultrasound Med Biol 2003;29:1705-13. [Crossref] [PubMed]
- Tang A, Cloutier G, Szeverenyi NM, Sirlin CB. Ultrasound Elastography and MR Elastography for Assessing Liver Fibrosis: Part 1, Principles and Techniques. AJR Am J Roentgenol 2015;205:22-32. [Crossref] [PubMed]
- Huwart L, Sempoux C, Vicaut E, Salameh N, Annet L, Danse E, Peeters F, ter Beek LC, Rahier J, Sinkus R, Horsmans Y, Van Beers BE. Magnetic resonance elastography for the noninvasive staging of liver fibrosis. Gastroenterology 2008;135:32-40. [Crossref] [PubMed]
- Heye T, Yang SR, Bock M, Brost S, Weigand K, Longerich T, Kauczor HU, Hosch W. MR relaxometry of the liver: significant elevation of T1 relaxation time in patients with liver cirrhosis. Eur Radiol 2012;22:1224-32. [Crossref] [PubMed]
- Bedossa P, Poynard T. An algorithm for the grading of activity in chronic hepatitis C. The METAVIR Cooperative Study Group. Hepatology 1996;24:289-93. [Crossref] [PubMed]
- Pugh RN, Murray-Lyon IM, Dawson JL, Pietroni MC, Williams R. Transection of the oesophagus for bleeding oesophageal varices. Br J Surg 1973;60:646-9. [Crossref] [PubMed]
- Trotter JF, Brimhall B, Arjal R, Phillips C. Specific laboratory methodologies achieve higher model for endstage liver disease (MELD) scores for patients listed for liver transplantation. Liver Transpl 2004;10:995-1000. [Crossref] [PubMed]
- Katsube T, Okada M, Kumano S, Hori M, Imaoka I, Ishii K, Kudo M, Kitagaki H, Murakami T. Estimation of liver function using T1 mapping on Gd-EOB-DTPA-enhanced magnetic resonance imaging. Invest Radiol 2011;46:277-83. [Crossref] [PubMed]
- Yamada A, Hara T, Li F, Fujinaga Y, Ueda K, Kadoya M, Doi K. Quantitative evaluation of liver function with use of gadoxetate disodium-enhanced MR imaging. Radiology 2011;260:727-33. [Crossref] [PubMed]
- Boll DT, Merkle EM. Diffuse liver disease: strategies for hepatic CT and MR imaging. Radiographics 2009;29:1591-614. [Crossref] [PubMed]
- Rohrer M, Bauer H, Mintorovitch J, Requardt M, Weinmann HJ. Comparison of magnetic properties of MRI contrast media solutions at different magnetic field strengths. Invest Radiol 2005;40:715-24. [Crossref] [PubMed]
- Hupfeld S, Pischel D, Jechorek D, Janicová A, Pech M, Fischbach F. MRI-based fat quantification of the liver: Is it time for commercially available products? Eur J Radiol 2021;144:109993. [Crossref] [PubMed]
- Kukuk GM, Hittatiya K, Sprinkart AM, Eggers H, Gieseke J, Block W, Moeller P, Willinek WA, Spengler U, Trebicka J, Fischer HP, Schild HH, Träber F. Comparison between modified Dixon MRI techniques, MR spectroscopic relaxometry, and different histologic quantification methods in the assessment of hepatic steatosis. Eur Radiol 2015;25:2869-79. [Crossref] [PubMed]
-
.Powers DM Evaluation: from precision, recall and F-measure to ROC, informedness, markedness and correlation. arXiv:201016061,2020 . - Breiman L. Bagging predictors. Machine Learning 1996;24:123-40. [Crossref]
- Seiffert C, Khoshgoftaar TM, Van Hulse J, Napolitano A. RUSBoost: A hybrid approach to alleviating class imbalance. IEEE Transactions on Systems, Man, and Cybernetics-Part A: Systems and Humans 2009;40:185-97. [Crossref]
- Bashir MR, Gupta RT, Davenport MS, Allen BC, Jaffe TA, Ho LM, Boll DT, Merkle EM. Hepatocellular carcinoma in a North American population: does hepatobiliary MR imaging with Gd-EOB-DTPA improve sensitivity and confidence for diagnosis? J Magn Reson Imaging 2013;37:398-406. [Crossref] [PubMed]
- Rauscher I, Eiber M, Ganter C, Martirosian P, Safi W, Umgelter A, Rummeny EJ, Holzapfel K. Evaluation of T1ρ as a potential MR biomarker for liver cirrhosis: comparison of healthy control subjects and patients with liver cirrhosis. Eur J Radiol 2014;83:900-4. [Crossref] [PubMed]
- Breit HC, Block KT, Winkel DJ, Gehweiler JE, Henkel MJ, Weikert T, Stieltjes B, Boll DT, Heye TJ. Evaluation of liver fibrosis and cirrhosis on the basis of quantitative T1 mapping: Are acute inflammation, age and liver volume confounding factors? Eur J Radiol 2021;141:109789. [Crossref] [PubMed]
- Sheng RF, Wang HQ, Yang L, Jin KP, Xie YH, Fu CX, Zeng MS. Assessment of liver fibrosis using T1 mapping on Gd-EOB-DTPA-enhanced magnetic resonance. Dig Liver Dis 2017;49:789-95. [Crossref] [PubMed]
- Ding Y, Rao SX, Zhu T, Chen CZ, Li RC, Zeng MS. Liver fibrosis staging using T1 mapping on gadoxetic acid-enhanced MRI compared with DW imaging. Clin Radiol 2015;70:1096-103. [Crossref] [PubMed]
- Obmann VC, Catucci D, Berzigotti A, Gräni C, Ebner L, Heverhagen JT, Christe A, Huber AT. T1 reduction rate with Gd-EOB-DTPA determines liver function on both 1.5 T and 3 T MRI. Sci Rep 2022;12:4716. [Crossref] [PubMed]
- Shapiro JM, Smith H, Schaffner F. Serum bilirubin: a prognostic factor in primary biliary cirrhosis. Gut 1979;20:137-40. [Crossref] [PubMed]
- Alessandria C, Ozdogan O, Guevara M, Restuccia T, Jiménez W, Arroyo V, Rodés J, Ginès P. MELD score and clinical type predict prognosis in hepatorenal syndrome: relevance to liver transplantation. Hepatology 2005;41:1282-9. [Crossref] [PubMed]
- Zoli M, Cordiani MR, Marchesini G, Iervese T, Labate AM, Bonazzi C, Bianchi G, Pisi E. Prognostic indicators in compensated cirrhosis. Am J Gastroenterol 1991;86:1508-13. [PubMed]
- Singh S, Venkatesh SK, Wang Z, Miller FH, Motosugi U, Low RN, Hassanein T, Asbach P, Godfrey EM, Yin M, Chen J, Keaveny AP, Bridges M, Bohte A, Murad MH, Lomas DJ, Talwalkar JA, Ehman RL. Diagnostic performance of magnetic resonance elastography in staging liver fibrosis: a systematic review and meta-analysis of individual participant data. Clin Gastroenterol Hepatol 2015;13:440-451.e6. [Crossref] [PubMed]
- Li J, Cao B, Bi X, Chen W, Wang L, Du Z, Zhang X, Yu X. Evaluation of liver function in patients with chronic hepatitis B using Gd-EOB-DTPA-enhanced T1 mapping at different acquisition time points: a feasibility study. Radiol Med 2021;126:1149-58. [Crossref] [PubMed]
- Shuter B, Tofts PS, Wang SC, Pope JM. The relaxivity of Gd-EOB-DTPA and Gd-DTPA in liver and kidney of the Wistar rat. Magn Reson Imaging 1996;14:243-53. [Crossref] [PubMed]
- Motosugi U, Ichikawa T, Sou H, Sano K, Tominaga L, Kitamura T, Araki T. Liver parenchymal enhancement of hepatocyte-phase images in Gd-EOB-DTPA-enhanced MR imaging: which biological markers of the liver function affect the enhancement? J Magn Reson Imaging 2009;30:1042-6. [Crossref] [PubMed]