Deep learning-based quantitative morphological study of anteroposterior digital radiographs of the lumbar spine
Introduction
Low back pain (LBP) is a serious global public health problem (1). The lifetime prevalence of LBP is up to 85%, and LBP causes significant personal, social, and economic burdens worldwide (2). Over the last decade, the number of people disabled by LBP has increased by 54% (3), with the greatest increase being in low- and middle-income countries. Moreover, the prevalence of LBP is increasing yearly, raising disability rates and healthcare costs. There are numerous causes of LBP, and although approximately 85% of LBP cases have no clear etiology, a link between LBP and intervertebral disc degeneration has been suggested (4), with discogenic LBP accounting for approximately 39% of the cases (5).
Anteroposterior X-ray of the lumbar spine is commonly used as the preferred examination method for patients with LBP. Such images are economical and convenient, and valuable in assessing lumbar spine diseases. Reducing intervertebral disc height (IDH) is a key point in the pathological process of intervertebral disc degeneration (6). Morphological changes in vertebral bodies are also an important part of intervertebral disc degeneration (6). Reduced vertebral body height (VBH) or IDH can cause lumbar foramen stenosis, facet joint dislocation, and spinal stenosis (7,8). In addition, alterations in VBH and IDH are risk factors for LBP-related diseases such as scoliosis (9), spondylolisthesis (10), and fragility fracture (11). Therefore, the measurement of VBH and IDH by observing the morphological changes of vertebral bodies from digital lumbar spine radiographs is important for diagnosing and treating LBP-related diseases.
Many studies have been performed to measure morphological parameters of the vertebral body and intervertebral disc from lateral digital radiographs of the lumbar spine, including the vertebral compression ratio, anterior, middle, and posterior heights of the vertebral body and intervertebral disc, the width and depth of the upper and lower endplates of the vertebral body, and the vertebral height index and intervertebral height index (12-15). Still, few studies have been performed to quantify the lumbar spine morphology from anteroposterior digital radiographs. The manual measurement of the lumbar morphological parameters represents a heavy workload with low efficiency and is time-consuming and laborious; in addition, there are differences among different observers and within the same observers, with low consistency and repeatability.
Furthermore, no previous studies used a deep learning algorithm to measure automatically and quantitatively VBH and IDH from anteroposterior digital radiographs of the lumbar spine, but some models are available for magnetic resonance imaging (MRI) (16,17), but access to MRI is limited, and the examination is more time-consuming and expensive than plain X-ray images. With the development of artificial intelligence, the application of the deep learning algorithm in diagnosing and treating lumbar spine diseases has become a hot research topic.
Therefore, this study aimed to create a deep learning-based model that uses a convolutional neural network for the quantitative morphological analysis of anteroposterior digital radiographs of the lumbar spine and to evaluate its performance and scalability. The results could provide a reference base and guide clinicians’ decision-making in treating lumbar spine diseases.
Methods
Materials
Data sets
The study was conducted in accordance with the Declaration of Helsinki (as revised in 2013). The Ethics Committee of Gansu Provincial Hospital of Traditional Chinese Medicine approved this retrospective study (No. 2020-112-01). The requirement for informed consent was waived because only retrospective imaging data were used.
From July 2020 to September 2021, the imaging data of 1,734 patients with LBP treated at the outpatient clinic were obtained from the hospital picture archiving and communication system (PACS). Patients ≥18 years of age who underwent anteroposterior digital radiography of the lumbar spine were included in this study. The age criterion was used to ensure that only patients who had achieved skeletal maturity were enrolled. The exclusion criteria were (I) a history of spinal surgery or severe spinal deformities; (II) metabolic bone disease, spinal tuberculosis, or tumors; (III) poor image quality with obscured landmarks; or (IV) severe bone hyperplasia or other factors affecting the measurement. Finally, 1,368 anteroposterior digital radiographs of the lumbar spine were included. They were randomly assigned to the training set, validation set, and test set according to the ratio of 65%, 20%, and 15%, respectively (Appendixes 1,2).
Landmark annotation and parameter measurement
The naming of each landmark was standardized to calculate clinically relevant parameters. Taking the L1 vertebral body as an example: L1HR stands for the right margin point of the superior edge of a vertebral body, L1FR stands for the right margin point of the inferior edge of a vertebral body edge, L1HL stands for the left margin point of the superior edge of vertebral body edge, and L1FL stands for the left margin point of the inferior vertebral body edge. The distance between the margin point of a vertebral body’s superior edge and the margin point of the inferior edge of the vertebral body edge (on the same side) was the VBH. The distance between the margin point of the inferior edge of the vertebral body and the margin point of the superior edge of the lower vertebrae (on the same side) was the IDH. In the present study, VBH from L1 to L5 and IDH from L1–L2 to L4-L5 were measured, and the VBH and IDH parameters measured on the left and right sides were pooled and calculated as an average. All landmark annotations and each specific name (18,19) and parameter measurements are shown in Figure 1.
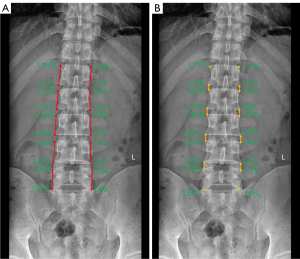
Three radiologists (R1, R2, and R3) with 3, 5, and 6 years of diagnostic experience in musculoskeletal imaging, respectively, independently labeled 27,360 landmarks from 1,368 anteroposterior digital radiographs of the lumbar spine in the form of pixel-level dots using the JPHV-specific software for training and validation of the model after uniform training. The radiologist R1 labeled the test set a second time after six weeks to assess intra-observer reliability. The mean of the three radiologists’ measurements was used as a reference standard when analyzing model performance.
Model construction
Data preprocessing
The original images were resized to a resolution of 640×640 pixels in the first stage. Each vertebral body was cropped according to the predicted vertebral key points and then scaled to 256×256 pixels in the second stage. In the model training phase, data augmentation methods such as random rotation (−30° to +30°), random scaling, and horizontal flipping were applied to increase the data diversity to improve model robustness. During the model inference phase, there were no data augmentation operations.
Study method
Inspired by the state-of-the-art pose methods (20,21) based on the heatmap coordinate representation, high-resolution network (HRNet) (21) was used as the backbone model and combined with the Distribution-Aware coordinate Representation of Key points (DARK) (20) to localize the vertebrae and identify the vertebral body key points. The whole pipeline design is summarized in Figure 2. According to the vertebrae to which each landmark belongs, the outer rectangle expanded by 60 pixels of every four landmarks, determining the region where a vertebra was located (Figure S1). The Cascaded DARK model construction process was divided into two stages to retain detailed information on lumbar spine images: lumbar spine vertebrae detection and landmark identification (22). This two-stage process was designed to reduce the resolution loss of the input images to the model. In the first stage, 20 key points of the spine were identified by the DARK model with HRNet-32 as the backbone, and vertebral bodies from L1 to L5 were obtained according to the key point locations of each vertebra. In the second stage, the five vertebral bodies detected in the first stage were cropped out from the original radiographs and fed into another DARK model similar to the first-stage structure to obtain the refined position of the key points of each vertebra. During the prediction process, the exact 20 landmark locations were obtained from the original radiographs with the trained Cascaded DARK model following the key point localization process (Figure S2). Based on the calculation method in parameter measurement, the relevant radiological parameters were then calculated from the coordinates of the predicted landmarks, thus enabling automatic measurement.
Model performance evaluation
The percentages within 1, 2, 3, 4, and 5 mm landmark-to-landmark distance thresholds were used to assess landmark annotation inter-observer and intra-observer reliability (23). The average value of the labeling results of the three radiologists was used as a reference standard. The model’s performance was evaluated in terms of two dimensions: accuracy of landmark localization and consistency of radiological parameters compared with the reference standard on the test set. Specifically, the percentage of correct key points (PCK) metric was used to evaluate the performance of all landmarks predicted by the model. The radiological parameters were calculated using the coordinates of the key points, as specified in the parameter measurement part. The intra-class correlation coefficient (ICC), the Pearson correlation coefficient (r), the mean absolute error (MAE), and the root mean square error (RMSE) were calculated between the model prediction and reference standard to evaluate the performance of the model (24). An ICC larger than 0.75 was considered to achieve high consistency between the reference standard and the model in evaluating the radiological parameters. |r|≥0.7 indicated a high correlation. Moreover, an inter-observer agreement was assessed between model estimated measurements and reference standard using the mean difference, standard deviation (SD), and 95% limit of agreement graphically represented by the Bland-Altman plots.
Comparison of convolutional neural networks
The model performance in detecting all landmarks was compared to that of other convolutional neural networks (EfficientDet + Unet, EfficientDet + DarkPose, HRNet, Unet) by using PCK on the test sets. All convolutional neural networks received the same training data and strategies for a fair comparison.
Statistical analysis
All data analyses were performed using Python (Scipy, Statsmodels, and Pingouin). Data were shown as means ± SDs or medians (ranges) based on distribution determined using the D’Agostino and Pearson omnibus normality tests. General patient data, including sex and age distribution, were presented by statistical description. The difference between the reference standard and the model in terms of prediction was analyzed using the paired t-test. Differences were considered statistically significant with P<0.05. Further statistical analysis was based on MAE, PCK, ICC, regression analysis, and Bland-Altman plot.
Results
General data distributions
This study included 1,368 lumbar spine anteroposterior digital radiographs. The ratio of males to females was 606:762 (44.3%:55.7%). The training set consisted of 893 radiographs, the validation set consisted of 269 radiographs, and the test set consisted of 206 radiographs. Table 1 summarizes the overall data distribution.
Table 1
Characteristic | Training set | Validation set | Test set |
---|---|---|---|
Image number, n (%) | 893 (65.3) | 269 (19.6) | 206 (15.1) |
Male | 396 (44.3) | 122 (45.5) | 88 (42.8) |
Female | 497 (55.7) | 147 (54.5) | 118 (57.2) |
Age (years), median [95% CI] |
48 [46–49] | 47 [40–50] | 38 [35–44] |
Male | 39 [37–43] | 37 [32–48] | 37 [33–45] |
Female | 52 [50–54] | 51 [45–54] | 40 [35–47] |
CI, confidence interval.
Reliability of landmark annotation
The percentages of intra-observer landmark distances within the 3 mm threshold were 89%, and the percentages of inter-observer landmark distances were 86% (R1 and R2), 92% (R1 and R3), and 95% (R2 and R3), respectively (Table 2).
Table 2
Threshold (mm) | 1 | 2 | 3 | 4 | 5 |
---|---|---|---|---|---|
Intra-observer reliability | 42 | 79 | 89 | 93 | 96 |
Inter-observer reliability | |||||
R1 vs. R2 | 37 | 74 | 86 | 91 | 94 |
R1 vs. R3 | 63 | 86 | 92 | 96 | 98 |
R2 vs. R3 | 67 | 91 | 95 | 97 | 98 |
Landmark performance
The PCK ranged from 99.77% to 99.46% for the L1 to L4 vertebrae and 77.37% for the L5 vertebrae within the 3 mm distance threshold, as shown in Table 3. Figure S3 shows a typical illustration of the predictive landmark model.
Table 3
Threshold (mm) | L1 | L2 | L3 | L4 | L5 |
---|---|---|---|---|---|
1 | 69.42 | 66.89 | 72.71 | 62.54 | 21.64 |
2 | 98.02 | 98.24 | 98.40 | 95.80 | 59.86 |
3 | 99.77 | 99.70 | 99.70 | 99.46 | 77.37 |
4 | 99.92 | 100.00 | 100.00 | 99.84 | 85.63 |
5 | 100.00 | 100.00 | 100.00 | 100.00 | 90.67 |
PCK, percentage of correct key points.
Comparison of convolutional neural networks
For the test set, the PCK of our model within the 1.5 to 5 mm threshold was larger than for the EfficientDet + Unet, EfficientDet + DarkPose, HRNet, and Unet models. Especially within the 1.5 to 3 mm threshold, the highest PCK of the present model and the highest PCK of the other models were 96.41%, 94.20%, 93.54%, 35.80%, and 20.70%. In addition, the present model average PCK for the five lumbar vertebrae within the 3 mm threshold was >95%. Figure 3 shows the comparison of the landmark predictions of the five convolutional neural network models and the prediction ability of the present convolutional neural network for each vertebra.
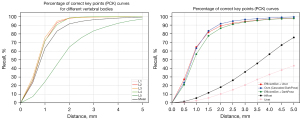
Model measurement performance
When the model’s parameters were compared to the three physicians’ mean values (gold standard), the results revealed that there were no significant differences between the two groups for VBH-L1, VBH-L2, VBH-L3, VBH-L4, IDH_L1–L2, and IDH_L2–L3 (all P>0.05). For VBH-L5, IDH_L3–L4, and IDH_L4–L5, the values measured by the model were 30.32±3.91, 12.04±1.54, and 8.08±2.34, respectively, compared to the reference standard values of 28.18±2.87, 11.71±1.30, and 9.85±1.72, respectively (all P<0.05) (Table 4).
Table 4
Parameters | Mean | Model | P | ICC (95% CI) | r | MAE | RMSE |
---|---|---|---|---|---|---|---|
VBH-L1 | 26.10±2.27 | 25.70±2.27 | 0.08 | 0.91 (0.85–0.95) | 0.93 | 0.66 | 0.95 |
VBH-L2 | 26.64±2.07 | 26.32±2.08 | 0.11 | 0.93 (0.88–0.96) | 0.93 | 0.59 | 0.79 |
VBH-L3 | 28.14±2.21 | 27.85±2.37 | 0.20 | 0.96 (0.92–0.98) | 0.97 | 0.50 | 0.66 |
VBH-L4 | 29.34±2.39 | 28.97±2.53 | 0.13 | 0.96 (0.9–0.98) | 0.97 | 0.58 | 0.74 |
VBH-L5 | 28.18±2.87 | 30.32±3.91 | 0.00* | 0.53 (0.19–0.72) | 0.67 | 2.53 | 3.63 |
IDH_L1–L2 | 9.40±1.58 | 9.67±1.61 | 0.09 | 0.84 (0.77–0.88) | 0.85 | 0.62 | 0.92 |
IDH_L2–L3 | 11.02±1.47 | 11.31±1.67 | 0.07 | 0.90 (0.84–0.94) | 0.93 | 0.51 | 0.69 |
IDH_L3–L4 | 11.71±1.30 | 12.04±1.54 | 0.02* | 0.88 (0.77–0.92) | 0.91 | 0.57 | 0.73 |
IDH_L4–L5 | 9.85±1.72 | 8.08±2.34 | 0.00* | 0.54 (0.04–0.79) | 0.78 | 1.88 | 2.30 |
Mean is the reference standard. Model is the parameter measured by the model. Data are expressed as the mean ± SD. *, P (paired t-test) <0.05 indicates the statistical significance between the model and the reference standard of the near parameters. VBH is the average height of the left and right vertebral body; IDH is the average height of the left and right intervertebral disc. ICC (95% CI), intra-class correlation coefficient (95% confidence interval); r, Pearson correlation coefficient; MAE, mean absolute error; RMSE, root mean square error; VBH, vertebral body height; IDH, intervertebral disc height.
Furthermore, the model’s overall performance was compared to the reference standard, with the results demonstrating that the model’s predicted values were consistent and reliable (ICC =0.84–0.96, r=0.85–0.97, MAE 0.5–0.66, RMSE 0.66–0.95) with the reference standard for all parameters except for two parameters: VBH-L5 (ICC 0.53, r 0.67, MAE 2.53, RMSE 3.63) and IDH_L4–L5 (ICC 0.54, r 0.78, MAE 1.88, RMSE 2.30) (Table 4). Bland Altman plots and regression analyses were performed to assess the differences and correlations between the model and the reference standard in measuring VBH and IDH (Figure 4); all parameters showed significant linear correlations except for two parameters: VBH-L5 and IDH_L4–L5.
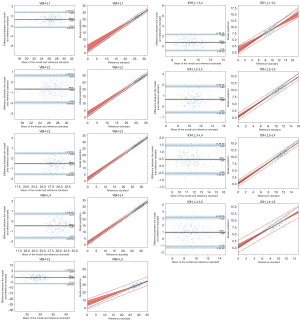
Discussion
The use of artificial intelligence for the automatic, quantitative, and efficient measurement of VBH and IDH is an urgent need for clinical diagnosis and treatment of lumbar spine diseases and is expected to provide clinicians with a basis for diagnosing and grading lumbar spine diseases, selecting treatment options, and assessing prognosis (19,25-27). IDH was the first imaging indicator used to evaluate intervertebral disc degeneration, and there is a relationship between morphological changes in the lumbar vertebral body and VBH and IDH, as well as between LBP and disc pathology (26,28). Karunanayake et al. (29) performed bivariate and logistic regression analyses of LBP with spinal space stenosis, vertebral osteophyte, and spondylolisthesis from posterior-anterior and lateral radiographs of the lumbosacral spine and showed that only spinal space stenosis was significantly associated with LBP. Machino et al. (14) performed a large-scale cross-sectional observational study using lateral and hyperextension-hyperflexion radiographs of the lumbar spine and found that age-related degenerative disc height reduction was most significant in the L4/L5 intervertebral space in the middle-aged and elderly population. Because the number of intervertebral disc surgeries is increasing, these findings will provide valuable information to clinicians before surgical interventions. Still, all the studies described above are based on manual measurements of relevant parameters. Two recent studies used deep learning but were based on MRI (16,17), which is less convenient than X-rays.
With the improvement of deep learning algorithms, many scholars used deep learning algorithms to measure anatomical parameters in medical images automatically, especially in bone and muscle images. Ye et al. (30) developed a model for measuring knee joint-related parameters based on lateral radiographs of both knees using the convolutional neural network algorithm. Compared with the gold standard, the model had high consistency in the measurement of left and right knee joint-related parameters (left knee ICC =0.91–0.95, r=0.84–0.91; right knee ICC =0.87–0.96, r=0.78–0.92). Li et al. (31) developed a model for automatically measuring the imaging parameters of hallux valgus in the weight-bearing dorsal plantar (DP) radiographs using deep learning. Under the 3-mm threshold, the PCK value of the predicted key point was 84–99%. Hao et al. (32) used high-level feature representation of deep convolutional neural networks to locate and identify automatically vertebrae in 3D CT volume. This study developed an automated parametric measurement model based on anteroposterior digital radiographs of the lumbar spine using the deep learning algorithm. The model could identify anatomical landmarks of the L1 to L4 vertebrae automatically and accurately. The model’s performance in measuring L1 to L4 vertebral body height and L1–L2 to L3–L4 IDH was near the radiologist’s level. Compared with the EfficientDet + Unet, EfficientDet + DarkPose HRNet, and Unet models, the present model performs better, especially within the 1.5–3 mm threshold. In order to locate vertebrae and identify vertebral key points in this study, a combination of HRNet and DARK was used. The results showed that the performance of the deep learning model developed here to identify the L1 to L4 vertebrae key points was good (PCK 99.77–99.46%), and the consistency between the measured parameters and the reference standard was close to or slightly better than the previous research results (ICC =0.84–0.96, r=0.85–0.97). The present study provides an accurate and efficient auxiliary tool for assisting clinicians in diagnosing and treating lumbar diseases in the future.
In the research, the mean of three radiologists’ measurements was used as a reference standard (33). Variability in traditional manual measurements is a common and unavoidable phenomenon where accuracy and reproducibility are primarily dependent on operator experience and judgment (34). However, three physicians with many years of experience in diagnostic musculoskeletal imaging underwent uniform training to ensure the accuracy of the data used for the model training set. In the present study, the percentage of inter-observer landmark distance within the 3-mm threshold was 86% to 95%, except for L5, while the PCK within the 3-mm distance threshold for landmarks predicted by the model ranged from 99.77% to 99.46%, indicating that the model predicted the L1 to L4 vertebral key points with better accuracy than the radiologists. The PCK of the L5 vertebral body within the 3-mm threshold was reduced compared with the other vertebral bodies, mainly due to the natural anterior convexity of the lumbar spine in the standing position of the human body and the anterior and posterior edges of the L5 vertebral body are not at the same level when performing anterior and posterior X-ray projection of the lumbar spine, and the incident X-rays cannot reach the tangential position for imaging, resulting in a limited view of the upper and lower edges of the L5 vertebral body (35) and reduced clarity. This situation is objective and unavoidable, and it is difficult for radiologists to accurately identify key points, independently from the deep learning algorithm.
There are some limitations in this study. First, the model struggled to identify and determine accurately the presence of transitional vertebrae, which impacted the accuracy of the results. We will train the model separately for the transition vertebrae in the future. Second, the dataset was not rich enough, and the prediction process was based only on 1,368 anteroposterior digital radiographic representations of the lumbar spine without clinical information such as age, sex, and weight, all factors that may be needed to link imaging findings to true clinical significance (36). In future studies, we intend to expand the lumbar anteroposterior digital radiograph dataset to include a broader range of cases and diseases to train the model better and investigate the relationship between age, sex, weight, diseases, and model performance. Finally, the performance of the measured parameters of the model is close to the level of the radiologist, which can reflect the diagnostic ability of the model from the side, but this study did not specifically explore how many patients were correctly diagnosed.
In conclusion, we developed a deep learning algorithm-based model for automatically measuring VBH and IDH from anteroposterior digital radiographs of the lumbar spine. The model has a good agreement in key-point identification and parameter measurement compared with radiologists, and its performance is comparable to or better than radiologists’. The model has yet to incorporate additional datasets and disease categories to train and improve the accuracy and stability of the model to assist clinicians in diagnosing and treating lumbar spine disorders from an efficient, accurate, and quantitative perspective.
Acknowledgments
Funding: This work was supported by
Footnote
Conflicts of Interest: All authors have completed the ICMJE uniform disclosure form (available at https://qims.amegroups.com/article/view/10.21037/qims-22-540/coif). G.C. is a consultant of Hangzhou Jianpei Technology Co., Ltd. L.H. is an employee of Hangzhou Jianpei Technology Co., Ltd. The other authors have no conflicts of interest to declare.
Ethical Statement: The authors are accountable for all aspects of the work in ensuring that questions related to the accuracy or integrity of any part of the work are appropriately investigated and resolved. The study was conducted in accordance with the Declaration of Helsinki (as revised in 2013). The study was approved by Gansu Provincial Hospital of Traditional Chinese Medicine approved this retrospective study (No. 2020-112-01). The requirement for informed consent was waived because retrospective imaging data were used.
Open Access Statement: This is an Open Access article distributed in accordance with the Creative Commons Attribution-NonCommercial-NoDerivs 4.0 International License (CC BY-NC-ND 4.0), which permits the non-commercial replication and distribution of the article with the strict proviso that no changes or edits are made and the original work is properly cited (including links to both the formal publication through the relevant DOI and the license). See: https://creativecommons.org/licenses/by-nc-nd/4.0/.
References
- Lamichhane B, Jayasekera D, Jakes R, Glasser MF, Zhang J, Yang C, Grimes D, Frank TL, Ray WZ, Leuthardt EC, Hawasli AH. Multi-modal biomarkers of low back pain: A machine learning approach. Neuroimage Clin 2021;29:102530. [Crossref] [PubMed]
- Hoy D, Brooks P, Blyth F, Buchbinder R. The Epidemiology of low back pain. Best Pract Res Clin Rheumatol 2010;24:769-81. [Crossref] [PubMed]
- Hartvigsen J, Hancock MJ, Kongsted A, Louw Q, Ferreira ML, Genevay S, Hoy D, Karppinen J, Pransky G, Sieper J, Smeets RJ, Underwood M. What low back pain is and why we need to pay attention. Lancet 2018;391:2356-67. [Crossref] [PubMed]
- Jamaludin A, Lootus M, Kadir T, Zisserman A, Urban J, Battié MC, Fairbank J, McCall I. ISSLS PRIZE IN BIOENGINEERING SCIENCE 2017: Automation of reading of radiological features from magnetic resonance images (MRIs) of the lumbar spine without human intervention is comparable with an expert radiologist. Eur Spine J 2017;26:1374-83. [Crossref] [PubMed]
- Zhang YG, Guo TM, Guo X, Wu SX. Clinical diagnosis for discogenic low back pain. Int J Biol Sci 2009;5:647-58. [Crossref] [PubMed]
- Zhang F, Zhang K, Tian HJ, Wu AM, Cheng XF, Zhou TJ, Zhao J. Correlation between lumbar intervertebral disc height and lumbar spine sagittal alignment among asymptomatic Asian young adults. J Orthop Surg Res 2018;13:34. [Crossref] [PubMed]
- Jarman JP, Arpinar VE, Baruah D, Klein AP, Maiman DJ, Muftuler LT. Intervertebral disc height loss demonstrates the threshold of major pathological changes during degeneration. Eur Spine J 2015;24:1944-50. [Crossref] [PubMed]
- Abbas J, Peled N, Hershkovitz I, Hamoud K. The Role of Vertebral Morphometry in the Pathogenesis of Degenerative Lumbar Spinal Stenosis. Biomed Res Int 2021;2021:7093745. [Crossref] [PubMed]
- Ponrartana S, Fisher CL, Aggabao PC, Chavez TA, Broom AM, Wren TA, Skaggs DL, Gilsanz V. Small vertebral cross-sectional area and tall intervertebral disc in adolescent idiopathic scoliosis. Pediatr Radiol 2016;46:1424-9. [Crossref] [PubMed]
- Xuegang H, Yang F, Ren E, Yang L, Deng Y, Ma Z, Zhang G, Gao Y, Wang Y, Yang Y, Kang X. Trapezoidal Vertebral Body and Spine-Pelvis Sagittal Alignment in Patients with Lumber Spondylolisthesis. Med Sci Monit 2020;26:e927747. [Crossref] [PubMed]
- Agius R, Galea R, Fava S. Bone mineral density and intervertebral disc height in type 2 diabetes. J Diabetes Complications 2016;30:644-50. [Crossref] [PubMed]
- Roux JP, Belghali S, Wegrzyn J, Rendu ES, Chapurlat R. Vertebral body morphology is associated with incident lumbar vertebral fracture in postmenopausal women. The OFELY study. Osteoporos Int 2016;27:2507-13. [Crossref] [PubMed]
- Kim DH, Jeong JG, Kim YJ, Kim KG, Jeon JY. Automated Vertebral Segmentation and Measurement of Vertebral Compression Ratio Based on Deep Learning in X-Ray Images. J Digit Imaging 2021;34:853-61. [Crossref] [PubMed]
- Machino M, Nakashima H, Ito K, Katayama Y, Matsumoto T, Tsushima M, Ando K, Kobayashi K, Imagama S. Age-related degenerative changes and sex-specific differences in osseous anatomy and intervertebral disc height of the thoracolumbar spine. J Clin Neurosci 2021;90:317-24. [Crossref] [PubMed]
- Wáng JQ, Káplár Z, Deng M, Griffith JF, Leung JCS, Kwok AWL, Kwok T, Leung PC, Wáng YXJ. Thoracolumbar Intervertebral Disc Area Morphometry in Elderly Chinese Men and Women: Radiographic Quantifications at Baseline and Changes at Year-4 Follow-up. Spine (Phila Pa 1976) 2018;43:E607-14. [Crossref] [PubMed]
- Zheng HD, Sun YL, Kong DW, Yin MC, Chen J, Lin YP, Ma XF, Wang HS, Yuan GJ, Yao M, Cui XJ, Tian YZ, Wang YJ. Deep learning-based high-accuracy quantitation for lumbar intervertebral disc degeneration from MRI. Nat Commun 2022;13:841. [Crossref] [PubMed]
- Natalia F, Young JC, Afriliana N, Meidia H, Yunus RE, Sudirman S. Automated selection of mid-height intervertebral disc slice in traverse lumbar spine MRI using a combination of deep learning feature and machine learning classifier. PLoS One 2022;17:e0261659. [Crossref] [PubMed]
- Singh R, Yadav SK, Sood S, Yadav RK, Rohilla R. Spino-pelvic radiological parameters in normal Indian population. SICOT J 2018;4:14. [Crossref] [PubMed]
- Yao J, Dong B, Sun J, Liu JT, Liu F, Li XW, Yuan PW, Zhang JB. Accuracy and Reliability of Computer-aided Anatomical Measurements for Vertebral Body and Disc Based on Computed Tomography Scans. Orthop Surg 2020;12:1182-9. [Crossref] [PubMed]
- Zhang F, Zhu X, Dai H, Ye M, Zhu C, editors. Distribution-Aware Coordinate Representation for Human Pose Estimation. 2020 IEEE/CVF Conference on Computer Vision and Pattern Recognition (CVPR); 2020.
- Wang J, Sun K, Cheng T, Jiang B, Deng C, Zhao Y, Liu D, Mu Y, Tan M, Wang X, Liu W, Xiao B. Deep High-Resolution Representation Learning for Visual Recognition. IEEE Trans Pattern Anal Mach Intell 2021;43:3349-64. [Crossref] [PubMed]
- Sun K, Xiao B, Liu D, Wang J. Deep High-Resolution Representation Learning for Human Pose Estimation. Institute of Electrical and Electronics Engineers 2019. doi:
10.1109/CVPR.2019.00584 . - Payer C, Štern D, Bischof H, Urschler M. Integrating spatial configuration into heatmap regression based CNNs for landmark localization. Med Image Anal 2019;54:207-19. [Crossref] [PubMed]
- Yang W, Ye Q, Ming S, Hu X, Jiang Z, Shen Q, He L, Gong X. Feasibility of automatic measurements of hip joints based on pelvic radiography and a deep learning algorithm. Eur J Radiol 2020;132:109303. [Crossref] [PubMed]
- Akeda K, Cheng K, Abarado E, Takegami N, Yamada J, Inoue N, Masuda K, Sudo A. Three-dimensional computed tomographic evaluation of lateral lumbar interbody fusion: morphometric change of intervertebral structure. Eur Spine J 2021;30:1355-64. [Crossref] [PubMed]
- Fyllos AH, Arvanitis DL, Karantanas AH, Varitimidis SE, Hantes M, Zibis AH. Magnetic resonance morphometry of the adult normal lumbar intervertebral space. Surg Radiol Anat 2018;40:1055-61. [Crossref] [PubMed]
- Qu B, Cao J, Qian C, Wu J, Lin J, Wang L, Ou-Yang L, Chen Y, Yan L, Hong Q, Zheng G, Qu X. Current development and prospects of deep learning in spine image analysis: a literature review. Quant Imaging Med Surg 2022;12:3454-79. [Crossref] [PubMed]
- Paholpak P, Wang Z, Sakakibara T, Kasai Y. An increase in height of spinous process is associated with decreased heights of intervertebral disc and vertebral body in the degenerative process of lumbar spine. Eur Spine J 2013;22:2030-4. [Crossref] [PubMed]
- Karunanayake AL, Pathmeswaran A, Wijayaratne LS. Chronic low back pain and its association with lumbar vertebrae and intervertebral disc changes in adults. A case control study. Int J Rheum Dis 2018;21:602-10. [Crossref] [PubMed]
- Ye Q, Shen Q, Yang W, Huang S, Jiang Z, He L, Gong X. Development of automatic measurement for patellar height based on deep learning and knee radiographs. Eur Radiol 2020;30:4974-84. [Crossref] [PubMed]
- Li T, Wang Y, Qu Y, Dong R, Kang M, Zhao J. Feasibility study of hallux valgus measurement with a deep convolutional neural network based on landmark detection. Skeletal Radiol 2022;51:1235-47. [Crossref] [PubMed]
- Hao C, Shen C, Jing Q, Dong N, Heng PAJSIP. Automatic Localization and Identification of Vertebrae in Spine CT via a Joint Learning Model with Deep Neural Networks. International Conference on Medical Image Computing and Computer-Assisted Intervention; 2015:515-22.
- Zhou S, Yao H, Ma C, Chen X, Wang W, Ji H, He L, Luo M, Guo Y. Artificial intelligence X-ray measurement technology of anatomical parameters related to lumbosacral stability. Eur J Radiol 2022;146:110071. [Crossref] [PubMed]
- Zhang J, Lou E, Shi X, Wang Y, Hill DL, Raso JV, Le LH, Lv L. A computer-aided Cobb angle measurement method and its reliability. J Spinal Disord Tech 2010;23:383-7. [Crossref] [PubMed]
- Guglielmi G, Diacinti D, van Kuijk C, Aparisi F, Krestan C, Adams JE, Link TM. Vertebral morphometry: current methods and recent advances. Eur Radiol 2008;18:1484-96. [Crossref] [PubMed]
- Azimi P, Yazdanian T, Benzel EC, Aghaei HN, Azhari S, Sadeghi S, Montazeri A. A Review on the Use of Artificial Intelligence in Spinal Diseases. Asian Spine J 2020;14:543-71. [Crossref] [PubMed]