Morphological appearance of the B.1.1.7 mutation of the novel coronavirus 2 (SARS-CoV-2) in chest CT
Introduction
Since coronavirus disease 2019 (COVID-19) has been identified as an outbreak of severe acute respiratory syndrome in Wuhan, Hubei Province, China in the beginning of December 2019 caused by the novel severe acute respiratory syndrome coronavirus 2 (SARS-CoV-2), real-time reverse-transcription polymerase chain reaction (RT-PCR) as well as unenhanced chest computed tomography (CT) offers the potential for a fast and sensitive diagnostic approach (1-3). Besides the assessment of the severity of lung involvement, chest CT-imaging is considered crucial due to distinct imaging features of COVID-19 pneumonia (2). In particular, typical CT findings include bilateral pulmonary ground glass opacities (GGO), crazy-paving, and consolidating lung opacities, in peripheral lung distribution mostly with rounded morphology, depending on the individual stage (2,4).
In contrast, bacterial pneumonias show also a characteristic pattern in the CT image with a high proportion of consolidations, which tend to have a one-sided or lobar distribution pattern (5,6).
Whereas bacterial pneumonia mostly displays an asymmetric, often unilobular pattern, this is not the case in viral, and explicitly not in COVID-19 pneumonia (7-9). Other pulmonary infections caused by non-COVID-19 virus or other species, e.g., pneumocystis pneumonia, also show distinct distribution pattern and can be catalogued by predominantly centrally appearing GGO which in many cases differs from the specific distribution pattern of COVID-19 pneumonia (10-12).
The biological causes for the specific and unique distribution pattern of a COVID-19 pneumonia are unknown. However, since the CT morphologic appearance of pneumonia caused by SARS-CoV-2 is different from other viral non-COVID pneumonia, the underlying factor must be related to the molecular structure and consequently the distinct genome of the SARS-CoV-2 virus (13-17). The current knowledge of viral mutation rates suggests that viral genetic diversity is determined by several virus- and host-dependent processes; viral genetic features become established as a consequence of a high number of spontaneous mutations in response to a specific selection pressure (18,19). Several authors state that the SARS-CoV-2 virus has a high number of mutations, due to its rapid worldwide spread, resulting in a high frequency of different virus variants arising (20-22).
This genetic change became apparent by an abrupt increase in reported COVID-19 cases in the United Kingdom in late summer to early autumn 2020, attributed to the emergence of a new SARS-CoV-2 variant 501Y.V1 (B.1.1.7) (23,24). In contrast to the initial in the year 2019 emerging wild-type variant of SARS-CoV-2, this subtype had a mutation (N501Y) in the receptor-binding domain of the spike protein that contributed to an increased transmission (24,25).
Despite the genetic variation and higher infection rate, there is no evidence that the distinct COVID-19 CT-morphological appearance of the B.1.1.7-variant differs from the initial SARS-CoV-2 wild-type. On the other hand, we are not aware of any study comparing quantitative image parameters and CT morphological appearance of gender, age and duration of disease onset to CT imaging matched COVID-19 pneumonia caused by these different virus variants.
For this reason, the present study aims to quantitatively compare the CT morphological findings between wild-type COVID-19 and B.1.1.7. variants to determine potential differences in the specific distribution pattern or extent of lung involvement at the same time point after initial symptom onset. Furthermore a comparison of the groups with (I) bacterial pneumonia and (II) viral non-COVID-19 pneumonia was performed to demonstrate the feasibility of the method and to elaborate on the existing differences in lung involvement between the groups.
Methods
Ethical statement
The methodology used in this study involving human participants was conducted according to the guidelines of the Declaration of Helsinki (as revised in 2013), and approved by the Ethics Committee of Cologne University (No. 20-1676). The requirement for informed consent was waived, due to the study was a retrospective study without any contact with patients. All the imaging data were anonymized. The study process did not reveal the patients’ private information, increase the patients’ pain, or cause any damage. All imaging was performed due to a clinical indication.
Patient population
Screening the database at the University Hospital Cologne from March 2018 to September 2021, confirmed cases of COVID-19 and other pulmonary infectious diseases were selected. Baseline characteristics (sex, age, days since clinical onset of pneumonia) were collected from patient charts and medical reports, and assigned to the respective CT image that was generated according to the care protocols on admission to the emergency department or during hospitalization. Following this, all additional data that could lead to an identification were completely anonymized for the purpose of evaluating the image data as described below. The total number of included patients was chosen considering the retrospectively evaluable number of CT datasets of pneumonia caused by COVID-19 B.1.1.7. mutation (n=46) and a matched number of COVID-19 wild-type, non-COVID-19 pneumonias (bacterial and viral half each), as well as a half number unremarkable CT examinations. The estimated number of included CT examinations in the groups (see Figure 1) was found to have a sufficient level of confidence (Ppower=0.9) in a power analysis using the R package PWR for analysis of variance (ANOVA), even assuming a low to moderate effect size (f =0.2) at significant levels P=0.05.
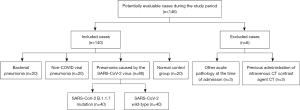
Inclusion criteria were
- Admission due to symptoms consistent with pulmonary infection, and
- CT on admission.
For classification to the subgroups we required either
- A positive RT-PCR SARS-CoV-2 test before virus variants spread pandemically (until May 2020), or
- A positive RT-PCR SARS-CoV-2 test for B.1.1.7 mutation, or
- Before January 2020 typical symptoms, exclusion of bacterial infections, or positive test for viral non-COVID-19 pneumonia, with corresponding infiltrates on CT scan. After January 2020 a positive test for viral non-COVID-19 pneumonia and negative test for COVID-19, as well as typical findings on CT.
- A microbiologically proven bacterial infection/characteristic response to antibiotic therapy and infiltrates on CT scan.
For a nonbiased comparison between B.1.1.7 mutation and wild-type, we matched for sex, age, and time between symptom onset and CT imaging. Hence, for each included patient with the COVID-19 B.1.1.7 variant, the time interval between symptom onset and CT scan was obtained and a sex- and age-matched patient from the wild-type group was matched, resulting in a balanced data set [within one standard deviation (SD) with respect to the interval scaled data]. For comparison, a test cohort of patients with unremarkable CT scans was included.
Exclusion criteria of COVID-19 B.1.1.7. group or potential matching patients of the other included groups were:
- Other acute pathology at the time of admission responsible for the respiratory symptoms and fulminant clinical course, such as pulmonary artery embolism (n=2), or acute coronary syndrome (n=1).
- Before (up to 6 days) performed contrast agent CT examination (n=3).
- Children and adolescents under 18 years.
- The CT scans of the excluded patients were not included in the image analysis and are not part of the analysis.
Imaging protocol
CT imaging was obtained at full inspiration in supine position using the institutional standard protocol. CT scans included 82% low-dose and 18% full-dose CTs with an average tube current of 83.6 mAs (±48.9), reference mAs for the low-dose examinations from 15–50 up to 140 mAs for the full-dose examinations. The tube voltage was set at 120 kV and a dose length product of 335.2 mGy*cm (±240.5), CDTIvol 3.7 mGy, 0.33 s rotation time and 0.76 pitch. Examinations were obtained on two CT-scanners (iCT 256, IQon Spectral CT, both Philips Healthcare, Amsterdam, The Netherland). Images were reconstructed in a 512×512 matrix containing pixels of 0.68×0.68 mm on hard lung kernel. Slice thickness was 2 mm with an overlap of 1 mm.
Automated segmentation of the lungs and lung pathologies
A group blinded volumetric analysis and visualization in 3D Slicer (http://www.slicer.org) on hard kernel chest CT image reconstructions using the open-source Lung CT Analyzer project tool (https://github.com/rbumm/SlicerLungCTAnalyzer/) was performed (26). The volumetric analysis proved robust for lung segmentation under the presence of severe pneumonia (see Figure 2A-2E) (26,27). The total 3D volume of each lung side was assessed (see Figure 2D) (26).
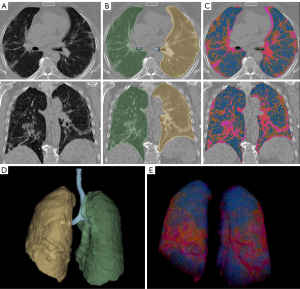
The segmentation of the pathologies was performed by application of threshold ranges to voxel-wise identify five volume classes: (I) bulla/emphysema, (II) inflated lung tissue, (III) GGO, (IV) consolidation, and (V) lung vessel. Segments are generated using 3D Slicer’s segment editor “Threshold” and “Grow from Seeds” function. The volume of each segment is calculated by using 3D Slicer’s “Segment statistics” function. The results are then superimposed to the CT 2D viewer and manually controlled for technical outliners in each segmentation (see Figure 2C).
To determine the percentage of pathologically altered lung portion (plp), the absolute extent of pathological altered (I) GGO, or (II) consolidation, or the (III) sum of both (abspath), was set in relation to the total lung volume {see Eq. [1]}, as already been done in other publications (28,29).
To obtain pathogen-specific distributions, on the one hand we obtained the lateralization index (li), which is calculated from the percentage ratio of the affected lung tissue between the two lungs {Eq. [2]}. Since the deviation from ‘1’ is a measure of lateralization, the Euclidean vector to ‘1’ is represented {see Eq. [2]}. On the other hand, the percentage ratio of GGO and consolidation was calculated to obtain the “pathological lung tissue quotient” (pq) in this way {Eq. [3]} (28).
The processing steps of automatic segmentation of the lungs and underlying pathologies were performed on a local computer equipped with Ryzen 5900X (AMD, Sunnyvale, CA, USA) Geforce 3090 (Nvidia, Santa Clara, CA, USA) and 64GB random-access memory (RAM).
Due to the few manual correction steps in our full lung segmentation approach, the segmentation was performed in a subset of 20 patients containing all included pathologies twice with one reader (JK) and once with another reader (PF) to demonstrate the intra-/inter-reader and retest reliability and consistency of the method (both readers have more than 4 years of experience in chest imaging).
Additional a visual-based analysis of the CO-RADS classification for each group assessing image appearance was performed. Regarding the categories I (no evidence of infection), II (typical of other infection but not COVID-19), III (appearances are compatible with COVID-19 but also with other diseases), IV (suspicious of COVID-19), V (typical of COVID-19), two radiological readers (PF and JK) had to score all the CT-images in the groups (30).
Statistics
Statistical data analysis was performed using R version 3.6.2 on Rstudio version 1.2.5033.24. Figures were plotted using the ggplot2 package 25 (31). Continuous variables were reported as mean and SD (32).
Due to the non-normally distributed data structure (Shapiro-Wilk test) of the volumes of pathologically affected lung tissue, a Kruskal-Wallis one-way analysis of variance (KS) for comparison of the individual groups defined by the pathogen was used (33,34). To locate differences between groups, we performed a pairwise Wilcoxon test adjusting for P value using the Bonferroni post-hoc test (35).
Intra- and inter-reader/retest reliability of the computed volume was tested using the intraclass correlation coefficient in two-way random-effects model (36). Statistical significance was defined as P<0.05.
Results
After application of the inclusion, exclusion, and matching criteria, 140 patients were finally enrolled in the investigation, divided into pneumonia caused by COVID-19 wild-type (n=40), COVID-19 B.1.1.7 variant (n=40), bacterial pneumonia (n=20), viral non-COVID-19 pneumonia (n=20) as well as a test group (n=20) including unremarkable CT-examinations of the chest (see Figure 1).
The mean age of patients in the data set was 58.5±20.7 years with 76 male and 66 female patients. The patient collectives of viral non-COVID-19 and bacterial infections turned out to be significantly older (P<0.05; t-test). No differences in gender distribution were found (chi2-test). In particular, the subgroups of the individual COVID-19 variants did not show any differences in patient demographics. Detailed patient characteristics within the groups are shown in Table 1.
Table 1
Infective agent | Sex | Age (years) | Symptom onset to CT (days) | N | |
---|---|---|---|---|---|
Male | Female | ||||
COVID-19wild-type | 23 | 17 | 46.9±20.7 [23–96] | 3.6±2.3 [0–8] | 40 |
COVID-19B.1.1.7 | 23 | 17 | 48.9±20.8 [20–95] | 3.8±2.7 [0–8] | 40 |
Viral non-COVID-19 | 12 | 8 | 64.2±19.6 [25–89] | 3.9±2.9 [0–12] | 20 |
Bacterial | 11 | 9 | 69.5±17.7 [26–91] | 6.7±5.7 [0–26] | 20 |
Normal control group | 11 | 9 | 44.3±16.8 [18–78] | 2.5±1.9 [0–6] | 20 |
Total | 74 | 66 | 58.5±20.7 [18–96] | 4.0±3.3 [0–26] | 140 |
The interval scaled data are presented as mean ± standard deviation , [range]. CT, computed tomography; COVID-19wild-type, initial variant of the coronavirus disease; COVID-19B.1.1.7, B1.1.7 mutation of the coronavirus disease; non-COVID-19 viral, viral caused non-COVID-19 pneumonia.
In the normal control group, the average extent of GGO, consolidation, and total affected lung was 2.10% (±2.46%), 0.39% (±0.42%), and 2.49% (±2.63%), respectively. Small amounts of pathologically registered lung volume, due to pulsation artifacts, pleural irregularities, and respiratory artifacts. The COVID-19 wild type group had an average extent of GGO, consolidation, and total affected lung of 21.34% (±13.72%), 9.16% (±8.69%), and 30.06% (±21.01%), and the B.1.1.7 subgroup of 22.25% (±11.85%), 11.35% (±9.73%) and 33.65% (±20.66%). In the group containing bacterial pneumonia the average extent of GGO, consolidation, and total affected lung was 15.54% (±9.91%), 7.67% (±2.61%), and 23.21% (±13.05%). In the group including viral non-COVID-19 pneumonia the average extent of GGO, consolidation, and total affected lung was 19.83% (±14.18%), 5.21% (±6.61%) and 25.04 (±21.44%), see Tables 2,3 and Figure 3. Within a randomly selected subset of 20 cases, semiautomatic segmentation of lung pathologies showed excellent (>0.75) inter-reader reliability with an intraclass correlation coefficient of 0.78 (37). Also, a good interreader agreement of 0.76 was calculated for the determination of the above-mentioned COV-RADS score. There was no difference in the two COVID-19 groups (wild-type modal value: category IV, 37.5%; B.1.1.7. variant also category IV, 40%), modal value of the two non-COVID pneumonia groups category II for bacterial pathogens (60%), and category II for viral non-COVID-19 pathogens (55%). The normal control group CT examinations were assigned 100% to category I (30).
Table 2
Infective agent | GGO | Consolidation | Total affected lung tissue |
---|---|---|---|
COVID-19wild-type | 21.34%±13.72% | 9.16%±8.69% | 30.06%±21.01% |
COVID-19B.1.1.7 | 22.25%±11.85% | 11.35%±9.73% | 33.65%±20.66% |
Viral non-COVID-19 | 19.83%±14.18% | 5.21%±6.61% | 25.04%±21.44% |
Bacterial | 15.54%±9.91% | 7.67%±2.61% | 23.21%±13.05% |
Normal control group | 2.10%±2.46% | 0.39%±0.42% | 2.49%±2.63% |
Data are presented as mean ± standard deviation. GGO, ground glass opacities; COVID-19wild-type, initial variant of the coronavirus disease; COVID-19B.1.1.7, B1.1.7 mutation of the coronavirus disease; non-COVID-19 viral, viral caused non-COVID-19 pneumonia.
Table 3
KS-test | Comparison group | COVID-19B.1.1.7 | Bacterial | Normal control | Viral non-COVID-19 |
---|---|---|---|---|---|
GGO | Bacterial | – | – | – | – |
c2 =45.02 | Normal | <0.001 | <0.001 | – | – |
df =4 | Viral non-COVID-19 | – | – | <0.001 | – |
P<0.001 | COVID-19wild-type | – | – | <0.001 | – |
Consolidation | Bacterial | – | – | – | – |
c2 =55.94 | Normal | <0.001 | <0.001 | – | – |
df =4 | Viral non-COVID-19 | <0.05 | <0.05 | <0.001 | – |
P<0.001 | COVID-19wild-type | – | – | <0.001 | – |
Affected lung tissue | Bacterial | – | – | – | – |
c2 =41.93 | Normal | <0.001 | <0.001 | – | – |
df =4 | Viral non-COVID-19 | – | – | <0.001 | – |
P<0.001 | COVID-19wild-type | – | – | <0.001 | – |
Pathological lung tissue quotient | Bacterial | – | – | – | – |
c2 =46.28 | Normal | <0.001 | <0.001 | – | – |
df =4 | Viral non-COVID-19 | <0.001 | <0.001 | – | – |
P<0.001 | COVID-19wild-type | – | – | <0.001 | <0.001 |
Lateralization index | Bacterial | <0.05 | – | – | – |
c2 =57.20 | Normal | – | <0.05 | – | – |
df =4 | Viral non-COVID-19 | – | <0.05 | – | – |
P<0.001 | COVID-19Wild type | – | <0.05 | – | – |
KS-test, Kruskal-Wallis one-way analysis of variance; COVID-19B.1.1.7, B1.1.7 mutation of the coronavirus disease; non-COVID-19 viral, viral caused non-COVID-19 pneumonia; GGO, ground glass opacities; COVID-19wild-type, initial variant of the coronavirus disease.
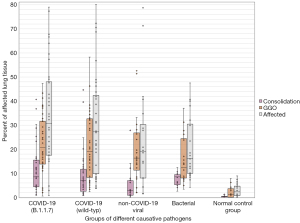
Regarding the time interval between anamnestically reported symptom onset and CT imaging, there was no significant difference between the groups with COVID-19 wild-type and B.1.1.7 variant, as expected based on the matching procedure. Further, there was no significant difference in time after symptom onset between the non-COVID-19 pneumonia groups.
Using the Kruskal-Wallis test for group comparisons, demonstrated differences between the groups in terms of (I) GGO, (II) consolidations as well as (III) total affected lung tissue. In the subsequent post-hoc tests with Bonferroni correction, the differences were determined as follows: regarding the percentage of GGO as well as the sum of affected lung tissue, the normal control group differs significantly from all pathogen groups (see Figure 2). No further group difference with respect to GGO was detected. Regarding the percentage of consolidation, the normal control group also differed significantly from all pathogenic groups. In addition, the group of pneumonia caused by non-COVID-19 viruses had a significantly lower percentage of consolidation than the bacterial pneumonia and the COVID-19 B.1.1.7 subgroup (P<0.05; see Table 3 and Figure 3). A similar result is obtained by considering the extent of the relationship between GGO and consolidations in the form of the above-mentioned “pathological lung tissue quotient”. Here we found that the group of bacterial as well as both COVID-19 subgroups stand out with proportionally more consolidation per GGO (P<0.001) than no-COVID-19 or the normal control group (see Table 3 and Figure 4). Considering the distribution ratio of affected lung tissue between the right and left lung in the form of the “lateralization index” a generalized significant distinction of the bacterial and all other groups was detected (P<0.05; see Table 3 and Figure 5).
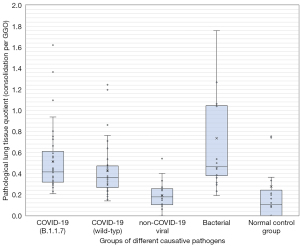
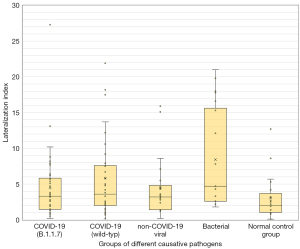
Discussion
The present study investigated whether altered molecular structure of the B.1.1.7 variant of the SARS-CoV-2 virus causes a quantitatively different involvement and distribution pattern from the distinct pulmonary changes caused by the wild type COVID-19 variant in a chest CT as described in multiple international publications (2-4). For this purpose, we used a semiautomatic 3D segmentation tool for quantification of the most common infectious-related lung tissue changes such as GGO and consolidations, as well as their total sum, ratio and distribution within each affected patient. Neither a significant difference between the total proportion of inflammatory affected lung tissue, nor the specific proportion of GGO or consolidations with respect to SARS-CoV-2 wild type and B.1.1.7 variant were detected in our PCR-confirmed study group. Regarding distribution ratio between the proportion of GGO per consolidation or distribution pattern between right and left lung in the sense of an asymmetry, no difference was detected between SARS-CoV-2 wild type and the B.1.1.7 variant. In the present study, both wild-type and B.1.1.7 variant showed symmetric distribution patterns of stage-dependent, inflammation-related GGO and consolidations in the matched time intervals (from early to late stages) (3). Given an equal distribution of the time course in the two COVID-19 groups, we were able to show that on a quantitative level there is no evidence that COVID-19 wild-type and B.1.1.7. mutation have a different stage course. In contrast, our method showed that the included non-COVID-19 virus pneumonias had significantly fewer consolidations than the bacterial, but also than the COVID-19 B.1.1.7 variant, a fact that supports findings of existing literature (6,7). At the same time, it was shown that these above-mentioned groups differed in terms of the quantitative ratio of GGO and consolidations. Furthermore, as expected, bacterial pneumonia showed a significantly more asymmetric pattern than all other groups, which is also consistent with previous investigations (6,8,9). In addition, it should be mentioned that another study also compared COVID-19 B.1.1.7. variants with non-B.1.1.7. variants and basically came to the same conclusion as the current investigation (38). A slightly different result, however, is that the B1.1.7. variant shows a greater lateralization. This slightly different result may be due to the fact that the duration of symptom onset for CT imaging is not matched between the groups, so that a homogeneous comparison is not possible (38). Because COVID-19 CT morphology is stage-specific and the features measured depend on the time elapsed since the onset of infection, we recommend strict matching between the variant and the comparison collective. In addition, the above study did not apply matching with respect to sex and age; in respect of age, there was even a significant difference between the two comparison groups (38).
However, our study reveals several limitations. Besides the retrospective, single center study design, the time-based ambiguity of the included COVID-19 patients is a limitation. For each patient from the B.1.1.7. group, the time elapsed between symptom onset and CT examination was assessed and a patient from the wild-type group matched in terms of sex and age was assigned, resulting in a balanced data set. Apart from the matched time intervals between symptom-onset and CT examination of the two COVID-19 groups, this is only an anamnestically reported information, subjectively perceived by the patient, and only represents a surrogate parameter for the actual time interval between onset of infection and imaging. Yet, this statement is the most applicable and regularly obtained information to estimate the time passed after infection, future research should take this into account. Although up-to-date there is no evidence that the B.1.1.7 variant causes a faster or chronologically different pulmonary affection, a precisely documented time after infection would be desirable for this statement. Lastly, despite manual validation, the automated lung pathology segmentation might have included very small non-pneumonic changes (micronodules) in the determined volume fractions.
Nevertheless, advantages of the present study are the exact genetic sequencing of the included COVID-19 cases, the analysis of a balanced data set mentioned above as well as the inclusion of a test and comparison data sets from viral non-COVID-19 pneumonia. Current CT data sets were generated on identical CT scanners using similar scanner configurations. CT segmentation ran automatically with a high degree of retest reliability. 3D segmentations were made using an open-source tool, giving any research team the opportunity to conduct the same analysis (26).
In summary, the present study represents the first matched quantitative comparison of fully 3D lung segmentation between the characteristic CT morphological appearance of COVID-19 pneumonia caused by the SARS-CoV-2 wild-type and the novel B.1.1.7 variant. We found no significant differences between these two genetic variants. But our semiautomatic approach demonstrated a high degree of validity and detected differences to other non-COVID-19 viral or bacterial pneumonias in accordance with existing literature.
Conclusions
In conclusion the benefit of the present analysis consists of the initiation and establishment of a quantitative method to detect possible differences of a different COVID-19 subtype caused by a mutation of the SARS-CoV-2 virus. The present approach should also be applied to other pneumonia caused by other SARS-CoV-2 mutations. In particular, the time course of various pathological lung changes could be quantitatively analyzed in relation to all COVID-19 subtypes. In case of the clinically less severe virus variants that have recently become known, such as the Omicron B.1.1.529 variant, it could potentially prospectively be shown whether there is a less extensive lung involvement in the course of time (39). However, this was beyond the purpose of this study which focused on a high-quality data set of B.1.1.7. Furthermore, the present study suggests that there are no CT morphological differences between COVID-19 wild-type and B1.1.7. variant, the clinical radiological diagnostic evaluation for this variant should remain the same and the diagnostic decision should be based on the known criteria.
But in the context of further possible mutations and new worldwide COVID-19 variants of concern, it is potentially possible that the genetic component responsible for the characteristic CT morphological appearance may change. A change in the distinct appearance with peripherally accentuated bilateral stage-dependent infiltrates could reduce the diagnostic performance of CT-examination and consequently the specificity of COVID-19 imaging. Early detection of changes in CT-characteristics of infections caused by a virus constantly undergoing genetic change should be a core component of future science and pandemic preparedness.
Acknowledgments
Funding: This research was supported by the German Federal Ministry of Education and Research (BMBF) as part of the University Medicine Network (Project No. RACOON, 01KX2021). The funders had no role in the design of the study, the collection, analyses, interpretation of data, writing of the manuscript, or in the decision to publish the results.
Footnote
Conflicts of Interest: All authors have completed the ICMJE uniform disclosure form (available at https://qims.amegroups.com/article/view/10.21037/qims-22-718/coif). DM received speaker’s honoria from Philips Healthcare, unrelated to the presented work. RS is an employee of Philips Healthcare unrelated to the presented work, there is no direct or indirect influence on the result of the present work. The other authors have no conflicts of interest to declare.
Ethical Statement: The authors are accountable for all aspects of the work in ensuring that questions related to the accuracy or integrity of any part of the work are appropriately investigated and resolved. The study was conducted according to the guidelines of the Declaration of Helsinki (as revised in 2013), and approved by the Ethics Committee of Cologne University (No. 20-1676). The requirement for informed consent was waived, due to the study was a retrospective study without any contact with patients. All the imaging data were anonymized. The study process did not reveal the patients’ private information, increase the patients’ pain, or cause any damage.
Open Access Statement: This is an Open Access article distributed in accordance with the Creative Commons Attribution-NonCommercial-NoDerivs 4.0 International License (CC BY-NC-ND 4.0), which permits the non-commercial replication and distribution of the article with the strict proviso that no changes or edits are made and the original work is properly cited (including links to both the formal publication through the relevant DOI and the license). See: https://creativecommons.org/licenses/by-nc-nd/4.0/.
References
- Li Q, Guan X, Wu P, Wang X, Zhou L, Tong Y, et al. Early Transmission Dynamics in Wuhan, China, of Novel Coronavirus-Infected Pneumonia. N Engl J Med 2020;382:1199-207. [Crossref] [PubMed]
- Rubin GD, Ryerson CJ, Haramati LB, Sverzellati N, Kanne JP, Raoof S, et al. The Role of Chest Imaging in Patient Management during the COVID-19 Pandemic: A Multinational Consensus Statement from the Fleischner Society. Radiology 2020;296:172-80. [Crossref] [PubMed]
- Pan F, Ye T, Sun P, Gui S, Liang B, Li L, Zheng D, Wang J, Hesketh RL, Yang L, Zheng C. Time Course of Lung Changes at Chest CT during Recovery from Coronavirus Disease 2019 (COVID-19). Radiology 2020;295:715-21. [Crossref] [PubMed]
- Chung M, Bernheim A, Mei X, Zhang N, Huang M, Zeng X, Cui J, Xu W, Yang Y, Fayad ZA, Jacobi A, Li K, Li S, Shan H. CT Imaging Features of 2019 Novel Coronavirus (2019-nCoV). Radiology 2020;295:202-7. [Crossref] [PubMed]
- Tanaka N, Matsumoto T, Kuramitsu T, Nakaki H, Ito K, Uchisako H, Miura G, Matsunaga N, Yamakawa K. High resolution CT findings in community-acquired pneumonia. J Comput Assist Tomogr 1996;20:600-8. [Crossref] [PubMed]
- Ito I, Ishida T, Togashi K, Niimi A, Koyama H, Ishimori T, Kobayashi H, Mishima M. Differentiation of bacterial and non-bacterial community-acquired pneumonia by thin-section computed tomography. Eur J Radiol 2009;72:388-95. [Crossref] [PubMed]
- Zheng F, Li L, Zhang X, Song Y, Huang Z, Chong Y, Chen Z, Zhu H, Wu J, Chen W, Lu Y, Yang Y, Zha Y, Zhao H, Shen J. Accurately Discriminating COVID-19 from Viral and Bacterial Pneumonia According to CT Images Via Deep Learning. Interdiscip Sci 2021;13:273-85. [Crossref] [PubMed]
- Kang M, Hong KS, Chikontwe P, Luna M, Jang JG, Park J, Shin KC, Park SH, Ahn JH. Quantitative Assessment of Chest CT Patterns in COVID-19 and Bacterial Pneumonia Patients: a Deep Learning Perspective. J Korean Med Sci 2021;36:e46. [Crossref] [PubMed]
- Vilar J, Domingo ML, Soto C, Cogollos J. Radiology of bacterial pneumonia. Eur J Radiol 2004;51:102-13. [Crossref] [PubMed]
- Miller WT Jr, Mickus TJ, Barbosa E Jr, Mullin C, Van Deerlin VM, Shiley KT. CT of viral lower respiratory tract infections in adults: comparison among viral organisms and between viral and bacterial infections. AJR Am J Roentgenol 2011;197:1088-95. [Crossref] [PubMed]
- Stefanidis K, Konstantelou E, Yusuf GT, Oikonomou A, Tavernaraki K, Karakitsos D, Loukides S, Vlahos I. Radiological, epidemiological and clinical patterns of pulmonary viral infections. Eur J Radiol 2021;136:109548. [Crossref] [PubMed]
- Reittner P, Ward S, Heyneman L, Johkoh T, Müller NL. Pneumonia: high-resolution CT findings in 114 patients. Eur Radiol 2003;13:515-21. [Crossref] [PubMed]
- Bai HX, Hsieh B, Xiong Z, Halsey K, Choi JW, Tran TML, Pan I, Shi LB, Wang DC, Mei J, Jiang XL, Zeng QH, Egglin TK, Hu PF, Agarwal S, Xie FF, Li S, Healey T, Atalay MK, Liao WH. Performance of Radiologists in Differentiating COVID-19 from Non-COVID-19 Viral Pneumonia at Chest CT. Radiology 2020;296:E46-54. [Crossref] [PubMed]
- Elmokadem AH, Bayoumi D, Abo-Hedibah SA, El-Morsy A. Diagnostic performance of chest CT in differentiating COVID-19 from other causes of ground-glass opacities. Egyptian Journal of Radiology and Nuclear Medicine 2021;52:12. [Crossref]
- Bai HX, Hsieh B, Xiong Z, Halsey K, Choi JW, Tran TML, Pan I, Shi LB, Wang DC, Mei J, Jiang XL, Zeng QH, Egglin TK, Hu PF, Agarwal S, Xie FF, Li S, Healey T, Atalay MK, Liao WH. Performance of Radiologists in Differentiating COVID-19 from Non-COVID-19 Viral Pneumonia at Chest CT. Radiology 2020;296:E46-54. [Crossref] [PubMed]
- Giannakis A, Móré D, Erdmann S, Kintzelé L, Fischer RM, Vogel MN, Mangold DL, von Stackelberg O, Schnitzler P, Zimmermann S, Heussel CP, Kauczor HU, Hellbach K. COVID-19 pneumonia and its lookalikes: How radiologists perform in differentiating atypical pneumonias. Eur J Radiol 2021;144:110002. [Crossref] [PubMed]
- Kasela S, Ortega VE, Martorella M, Garudadri S, Nguyen J, Ampleford E, et al. Genetic and non-genetic factors affecting the expression of COVID-19-relevant genes in the large airway epithelium. Genome Med 2021;13:66. [Crossref] [PubMed]
- Merleau NSC, Pénisson S, Gerrish PJ, Elena SF, Smerlak M. Why are viral genomes so fragile? The bottleneck hypothesis. PLoS Comput Biol 2021;17:e1009128. [Crossref] [PubMed]
- Sanjuán R, Domingo-Calap P. Mechanisms of viral mutation. Cell Mol Life Sci 2016;73:4433-48. [Crossref] [PubMed]
- Boehm E, Kronig I, Neher RA, Eckerle I, Vetter P, Kaiser L. Novel SARS-CoV-2 variants: the pandemics within the pandemic. Clin Microbiol Infect 2021;27:1109-17. [Crossref] [PubMed]
- Laha S, Chakraborty J, Das S, Manna SK, Biswas S, Chatterjee R. Characterizations of SARS-CoV-2 mutational profile, spike protein stability and viral transmission. Infect Genet Evol 2020;85:104445. [Crossref] [PubMed]
- Otto SP, Day T, Arino J, Colijn C, Dushoff J, Li M, Mechai S, Van Domselaar G, Wu J, Earn DJD, Ogden NH. The origins and potential future of SARS-CoV-2 variants of concern in the evolving COVID-19 pandemic. Curr Biol 2021;31:R918-29. [Crossref] [PubMed]
- Volz E, Mishra S, Chand M, Barrett JC, Johnson R, Geidelberg L, et al. Assessing transmissibility of SARS-CoV-2 lineage B.1.1.7 in England. Nature 2021;593:266-9. [Crossref] [PubMed]
- Fontanet A, Autran B, Lina B, Kieny MP, Karim SSA, Sridhar D. SARS-CoV-2 variants and ending the COVID-19 pandemic. Lancet 2021;397:952-4. [Crossref] [PubMed]
- Gu H, Chen Q, Yang G, He L, Fan H, Deng YQ, et al. Adaptation of SARS-CoV-2 in BALB/c mice for testing vaccine efficacy. Science 2020;369:1603-7. [Crossref] [PubMed]
- Bumm R, Lasso A, Kawel-Böhm N, Wäckerlin A, Ludwig P, Furrer M. First results of spatial reconstruction and quantification of COVID-19 chest CT infiltrates using lung CT analyzer and 3D slicer. British Journal of Surgery 2021;108:znab202.077.
- Alnaser A, Gong B, Moeller K. Evaluation of open-source software for the lung segmentation. Current Directions in Biomedical Engineering 2016;2:515-8. [Crossref]
- Baker AC. Discrete mathematics with applications, by Susanna S. Epp. The Mathematical Gazette 1991;75:233. [Crossref]
- Fervers P, Fervers F, Jaiswal A, Rinneburger M, Weisthoff M, Pollmann-Schweckhorst P, Kottlors J, Carolus H, Lennartz S, Maintz D, Shahzad R, Persigehl T. Assessment of COVID-19 lung involvement on computed tomography by deep-learning-, threshold-, and human reader-based approaches-an international, multi-center comparative study. Quant Imaging Med Surg 2022;12:5156-70. [Crossref] [PubMed]
- Prokop M, van Everdingen W, van Rees Vellinga T, Quarles van Ufford H, Stöger L, Beenen L, Geurts B, Gietema H, Krdzalic J, Schaefer-Prokop C, van Ginneken B, Brink M. CO-RADS: A Categorical CT Assessment Scheme for Patients Suspected of Having COVID-19-Definition and Evaluation. Radiology 2020;296:E97-104. [Crossref] [PubMed]
- Heinemann J. Cluster Analysis of Untargeted Metabolomic Experiments. Methods Mol Biol 2019;1859:275-85. [Crossref] [PubMed]
- Döring N, Bortz J. Forschungsmethoden und Evaluation in den Human- und Sozialwissenschaften. Springer Berlin, Heidelberg, 2016:1051.
- Shapiro SS, Wilk MB. An analysis of variance test for normality (complete samples). Biometrika 1965;52:591-611. [Crossref]
- Wayne DW. Kruskal-Wallis one-way analysis of variance by ranks. In: Applied Nonparametric Statistics. 2nd edition. Boston: PWS-Kent, 1990:226-34.
- Abdi H. Bonferroni and Sidak corrections for multiple comparisons. In: Salkind NJ. editor. Encyclopedia of Measurement and Statistics. Thousand Oaks, CA, USA: Sage, 2007:103-7.
- McGraw KO, Wong SP. Forming Inferences about Some Intraclass Correlation Coefficients. Psychol Methods 1996;1:30-46. [Crossref]
- Cicchetti DV. Guidelines, Criteria, and Rules of Thumb for Evaluating Normed and Standardized Assessment Instruments in Psychology. Psychol Assess 1994;6:284-90. [Crossref]
- Simon J, Grodecki K, Cadet S, Killekar A, Slomka P, Zara SJ, Zsarnóczay E, Nardocci C, Nagy N, Kristóf K, Vásárhelyi B, Müller V, Merkely B, Dey D, Maurovich-Horvat P. Radiomorphological signs and clinical severity of SARS-CoV-2 lineage B.1.1.7. BJR Open 2022;4:20220016. [Crossref] [PubMed]
- Meo SA, Meo AS, Al-Jassir FF, Klonoff DC. Omicron SARS-CoV-2 new variant: global prevalence and biological and clinical characteristics. Eur Rev Med Pharmacol Sci 2021;25:8012-8. [PubMed]