Contrast enhanced magnetic resonance imaging–based radiomics nomogram for preoperatively predicting expression status of Ki-67 in meningioma: a two-center study
Introduction
Meningioma is one of the most frequent primary central nervous system (CNS) tumors (1,2). According to the 2016 World Health Organization (WHO) classification of CNS tumors, meningioma can be classified into 3 histological grades and 15 subtypes (3), among which grade I accounts for 81% to 95% of meningioma (2,4). However, the 5-year recurrence rate of grade I meningioma is more than 10% after complete resection (5,6), and some have the potential to transform into high-grade subtypes (7). In the past half century, prediction of meningioma recurrence and invasiveness has relied solely upon histological features, which, however, has proven inadequate in evaluating survival outcomes (8). Thus, it is essential to explore new auxiliary diagnostic techniques or histological markers to evaluate the complex clinical and biological behavior of meningioma (9,10). Proliferation markers include the p53 gene, Ki-67 proliferation index (Ki-67 PI), argyrophilic nucleolar organizer regions, 5-bromo-2-deoxyuridine, and proliferating cell nuclear-antigen. Among these markers, Ki-67 PI is the most valuable, as it is expressed in all active phases of the cell cycle (G1, S, G2, M) except the G0 phase (11). Recently, its negative effects on clinical outcomes have been extensively proven for most solid tumors (12-16). Many studies have demonstrated that Ki-67 is an important surrogate marker of meningioma grade, prognosis, and malignant transformation (17-19). Telugu et al. reported that Ki-67 PI correlated well with increasing the histological grade and biological behavior of meningioma (17). Furthermore, it has been found that higher Ki-67 PI expression is negatively associated with survival in meningioma, with Ki-67 PI >4% being more appropriate for prognosis prediction; it has been thus highly recommended that patients with elevated expression of Ki-67 PI undergo stricter follow-up (18).
At present, the expression of Ki-67 PI in clinical practice is mainly detected through immunohistochemical (IHC) analysis of postoperative specimens. However, sampling error, delays, and the high cost of IHC analysis reduce the value of Ki-67 PI in preoperative evaluation and treatment planning of patients with meningioma (20). In contrast, radiomics, which involves analyzing the distribution and relationship of pixel or voxel gray levels in the lesion area, has been used to quantitatively assess tumor heterogeneity in more detail than has visual evaluation (21,22). It can evaluate the holistic characteristics of meningioma comprehensively, noninvasively, and in a timely manner (23). Previous studies showed that analysis using magnetic resonance imaging (MRI)–based radiomics features perform well in predicting Ki-67 PI of CNS tumors (24,25).
Considering the above cumulatively, our study aimed to develop and validate a classifier and integrate radiological characteristics with radiomics features to construct a nomogram for preoperatively predicting Ki-67 PI expression in meningioma. The aim was to provide valuable information for predicting the intrinsic clinical and biological behavior of meningioma. The following article is presented in accordance with the TRIPOD reporting checklist (available at https://qims.amegroups.com/article/view/10.21037/qims-22-689/rc).
Methods
Patients
The study was conducted in accordance with the Declaration of Helsinki (as revised in 2013) and approved by the ethics board of the First Affiliated Hospital of Kunming Medical University (ethics No. 2021-L-6). Individual consent for this retrospective analysis was waived. The data of consecutive patients with pathologically confirmed meningioma who were hospitalized in 2 centers between June 2016 and June 2020 were reviewed. Patients were included only if they met all of the following criteria: (I) received neurosurgery treatment and diagnosed with meningioma based on pathology results, (II) underwent head MRI scanning within 1 month before neurosurgery, (III) underwent postoperative IHC analysis of Ki-67 PI, and (IV) accurate results (Ki-67 PI ≤4% was recorded as low expression, and Ki-67 PI >4% was recorded as high expression) (18,26,27). Patients were excluded if they met any of the following criteria: (I) insufficient quality of MRI images or (II) incomplete clinical or pathological information. Patients from center I were enrolled in the training and test cohort, while center II patients were enrolled in the external validation cohort.
MRI image acquisition and evaluation
The following MRI scanners were used: center I, Philips 1.5-Tesla (Achieva, Philips, Amsterdam, The Netherlands); and center II, Siemens (Area) 1.5-Tesla (Siemens, Munich, Germany). The MRI protocol of center I was as follows: T1-weighted imaging [T1WI; repetition time/time to echo (TR/TE) =550 ms/15 ms], T2-weighted imaging (T2WI; TR/TE =4,000 ms/100 ms), fluid-attenuated inversion recovery (FLAIR; TR/TE/TI =7,000 ms/120 ms/2,200 ms), and diffusion-weighted imaging (DWI; TR/TE =2,533 ms/94 ms, b =1,000 s/mm2); apparent diffusion coefficient (ADC) maps were automatically reconstructed and contrast enhanced T1-WI was used (CE-T1WI; TR/TE =250 ms/2.5 ms). All sequences were acquired with the following range of parameters: slice thickness =6 mm, slice spacing =1 mm, field of view (FOV) =240 mm × 240 mm, and number of excitations (NEX) =1. For contrast medium, gadopentetate Di meglumine (Gd-DTPA) was injected into the cubital vein using a high-pressure syringe at a dose of 0.2 mmol/kg and a rate of 2.0 mL/s.
Radiological characteristics were assessed randomly by 2 radiologists (YL and YHG) with more than 8 years’ experience in CNS radiology. Both were blinded to the histopathological information of patients. Any disagreement was resolved through consultation.
IHC analysis
The surgical specimens were fixed in 10% buffered neutral formalin entirely sampled and embedded in paraffin, and 5-µm sections were stained with hematoxylin and eosin (HE). Ki-67 PI was recorded as the percentage of positively labeled tumor nuclei with Ki-67 monoclonal antibody per 1,000 tumor cells. Tumor grading was classified according to the 2016 WHO classification (3).
Tumor segmentation and radiomics feature extraction
The axial CE-T1WI images of all patients were imported into IBEX radiomics software (version 1.0, http://bit.ly/IBEX_MDAnderson) with the DICOM format. The tumor contours were manually delineated using outermost boundaries slice by slice. Two regions of interest (ROIs) were independently delineated by two radiologists (ZQO and YZ) and reviewed by a senior radiologist (XJS) with more than 30 years’ experience in CNS radiology. Before extraction of radiomics features, resample voxel size and a Laplacian filter were used to preprocess these delineated ROIs. In total, 838 radiomics features were extracted by IBEX from 3 categories, including (I) shape and size features, (II) first-order statistics features, and (III) texture-based features, which were calculated from gray-level co-occurrence matrix (GLCM) and gray-level run-length matrix (GLRLM).
Radiomics feature selection and model construction
To avoid dimensional disarray and choose related features that made a major contribution to the predictive models, a 4-stage feature selection was performed. First, the inter-operator reproducibility of 2 ROIs was evaluated using the intraclass correlation coefficient (ICC) (28). Radiomics features with an ICC value >0.75 were categorized as good agreement with high reproducibility. Second, the radiomics features with an ICC value >0.75 were normalized using Z-scores to eliminate differences introduced due to value scales. Then, the normalized data were randomly divided into training and test cohorts using a random function with a 7:3 ratio. Third, the radiomics features of the training cohort were preliminarily screened using 1-way analysis of variance (ANOVA). Finally, a least absolute shrinkage and selection operator (LASSO) binary logistic regression and 10-fold cross-validation were conducted to select the effective radiomics features with nonzero coefficients in the training cohort.
After the above steps were conducted, the features selected by the training cohort were entered into the following classifiers to construct a radiomics signature: logistic regression (LR), decision tree (DT), support vector machine (SVM), and adaptive boost (AdaBoost). Additionally, the 4 classifiers were internally validated in the test cohort. In addition, radiological characteristics with significant differences were combined with the radiomics signature to construct a combined model. Based on the combined model, a radiomics nomogram was constructed to preoperatively quantify the risk of Ki-67 high expression in patients with meningioma. The formula of the radiomics score was fitted using the linear combination of the selected features, which were weighted by their LASSO coefficients. For the specific methods, refer to Wu et al. (29). Finally, the radiomics score was included in the nomogram to calculate the risk of high expression Ki-67 in patients with meningioma.
External validation and model evaluation
The data obtained from center II were normalized with Z-scores to perform external validation of the above models. Receiver operating characteristic (ROC), area under curve (AUC), sensitivity, specificity, and accuracy were used to evaluate the performances of the prediction models. Moreover, a decision curve (DC) was used to compare the overall net benefits between the radiology signature, radiomics signature, and the combined model. A calibration curve (CC) was used to evaluate the reliability of the nomogram.
The workflow is shown in Figure 1.
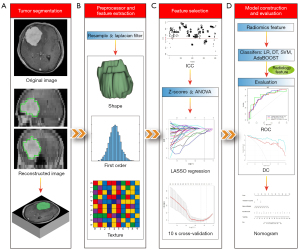
Statistical analysis
The sample size of our study was determined using binormal ROC curve analysis, which was performed using diagnostic accuracy studies in PASS software (version 15.0; NCSS LLC, Kaysville, UT, USA; https://www.ncss.com). Statistical analysis was performed using SPSS software (version 23.0; IBM Corp, Armonk, NY, USA) and the R software package (version 4.0.2; The R Foundation for Statistical Computing, Vienna, Austria; https://www.r-project.org). Differences in clinical characteristics were assessed by t-test, chi-squared test, or Fisher exact test, as appropriate, and a 2-sided P value <0.05 was considered statistically significant.
Results
Clinical, pathological, and radiological characteristics of patients
A total of 240 eligible patients, including 120 patients with high Ki-67 expression and 120 patients with low Ki-67 expression were enrolled in our study. Among these patients, 168 cases were randomized to the training cohort, and the remaining 72 cases were enrolled in the test cohort. As is shown in Table 1, except for expression of Ki-67 PI (P<0.001) and postoperative pathological grade (P=0.002 and P=0.011, respectively), no significant differences were found between groups regarding age and gender in either cohort. Table 2 shows the radiological characteristic data; only enhanced homogeneity showed significant differences in both the training and test cohorts (P=0.008 and P=0.009, respectively). The above results demonstrated that pathological grade was positively correlated with a high expression of Ki-67, which is consistent with results of previous studies (18,26,30-32), and patients with meningioma with higher Ki-67 PI expression were more likely to exhibit less homogeneous/heterogeneous enhancement on MRI.
Table 1
Characteristics | Training cohort | Test cohort | |||||||
---|---|---|---|---|---|---|---|---|---|
Ki-67≤4% | Ki-67>4% | t/χ2 | P value | Ki-67≤4% | Ki-67>4% | t/χ2 | P value | ||
Gender | 0.544 | 0.461 | 0.071 | 0.789 | |||||
Male | 21 (25.0) | 17 (20.2) | 9 (25.0) | 10 (27.8) | |||||
Female | 63 (75.0) | 67 (79.8) | 27 (75.0) | 26 (72.2) | |||||
Age (years) | 52.8±11.4 | 52.6±11.6 | −0.067 | 0.947 | 52.2±11.9 | 50.5±15.0 | 0.549 | 0.585 | |
Ki-67 PI (%) | 1.82±0.81 | 7.41±5.74 | −6.886 | <0.01 | 2.04±0.83 | 10.43±8.55 | −4.683 | <0.001 | |
Pathological grade (WHO) | 11.087 | 0.002 | 8.824 | 0.011 | |||||
I | 76 (90.5) | 59 (70.2) | 33 (91.7) | 23 (63.9) | |||||
II | 8 (9.5) | 23 (27.4) | 3 (8.3) | 8 (22.2) | |||||
III | 0 (0) | 2 (2.4) | 0 (0) | 5 (13.9) |
Numerical data are presented as mean ± standard deviation, categorical data as numbers (%). PI, proliferation index; WHO, World Health Organization.
Table 2
Characteristics | Training cohort | Test cohort | |||||||
---|---|---|---|---|---|---|---|---|---|
Ki-67≤4% | Ki-67>4% | t/χ2 | P value | Ki-67≤4% | Ki-67>4% | t/χ2 | P value | ||
Number of lesions | 1.701 | 0.192 | 0.860 | 0.354 | |||||
Single | 81 (96.4) | 77 (91.7) | 32 (88.9) | 35 (97.2) | |||||
Multiple | 3 (3.6) | 7 (8.3) | 4 (11.1) | 1 (2.8) | |||||
Location | 6.655 | 0.225 | 5.461 | 0.242 | |||||
Cerebral convexity | 55 (65.5) | 62 (73.8) | 22 (61.1) | 17 (47.3) | |||||
Deep brain | 2 (2.4) | 3 (3.6) | 0 (0) | 0 (0) | |||||
Cerebellar hemisphere | 11 (13.1) | 6 (7.1) | 1 (2.8) | 3 (8.3) | |||||
Peri skull base | 6 (7.1) | 9 (10.7) | 8 (22.2) | 8 (22.2) | |||||
Cerebellopontine angular | 9 (10.7) | 3 (3.6) | 5 (13.9) | 4 (11.1) | |||||
Lateral ventricle | 1 (1.2) | 1 (1.2) | 0 (0) | 4 (11.1) | |||||
Shape | 0.867 | 0.351 | 0.000 | 1.000 | |||||
Round-like | 50 (59.5) | 44 (52.4) | 20 (55.6) | 20 (55.6) | |||||
Irregular | 34 (40.5) | 40 (47.6) | 16 (44.4) | 16 (44.4) | |||||
Peritumoral edema | 0.902 | 0.337 | 0.900 | 0.343 | |||||
Yes | 50 (59.5) | 56 (66.7) | 18 (50.0) | 22 (61.1) | |||||
No | 34 (40.5) | 28 (33.3) | 18 (50.0) | 14 (38.9) | |||||
Enhanced degree | 0.256 | 0.613 | 0.514 | 0.473 | |||||
Mild/moderate | 1 (1.2) | 3 (3.6) | 0 (0) | 2 (5.6) | |||||
Marked | 83 (98.8) | 81 (96.4) | 36 (100.0) | 34 (94.4) | |||||
Enhanced homogeneity | 9.665 | 0.008 | 9.533 | 0.009 | |||||
Homogeneous | 55 (65.5) | 37 (44.0) | 25 (69.4) | 14 (38.8) | |||||
Less homogeneous | 19 (22.6) | 23 (27.4) | 9 (25.0) | 11 (30.6) | |||||
Heterogeneous | 10 (11.9) | 24 (28.6) | 2 (5.6) | 11 (30.6) | |||||
Diffusion limited | 4.550 | 0.033 | 0.000 | 1.000 | |||||
Yes | 8 (9.5) | 18 (21.4) | 2 (5.6) | 3 (8.3) | |||||
No | 76 (90.5) | 66 (78.6) | 34 (94.4) | 33 (91.7) | |||||
Dural tail sign mater | 1.532 | 0.216 | 0.223 | 0.637 | |||||
Yes | 49 (58.3) | 41 (48.8) | 20 (55.6) | 18 (50.0) | |||||
No | 35 (41.6) | 43 (51.2) | 16 (44.4) | 18 (50.0) | |||||
Maximum diameter | 3.66±1.76 | 4.61±1.81 | −3.446 | 0.001 | 3.82±1.57 | 4.26±1.52 | −1.226 | 0.224 |
Numerical data are presented as mean ± standard deviation, categorical data as numbers (%).
Analysis of radiomics feature
In total, 838 radiomics features were extracted from the CE-T1WI image. Among these, 783 radiomics features were regarded as stable features after being assessed with ICC. In all, 622 radiomics features showed significant differences between the high and low expression groups according to ANOVA. These features were then imported into the LASSO regression with 10-fold cross-validation to select the most important features (Figure 2). Finally, 14 radiomics features with nonzero coefficients were selected (Table 3).
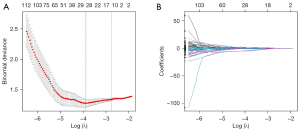
Table 3
Numbers | Sequence | Feature type | Feature name | LASSO coefficient |
---|---|---|---|---|
X1 | CE-T1WI | Shape | Max 3D diameter | 0.382 |
X2 | CE-T1WI | Shape | Spherical disproportion | −0.423 |
X3 | CE-T1WI | Shape | Sphericity | 0.058 |
X4 | CE-T1WI | Gradient orient histogram | 5th percentile | −0.133 |
X5 | CE-T1WI | Intensity direct | Local entropy max | −0.497 |
X6 | CE-T1WI | Intensity direct | 10th percentile | −0.037 |
X7 | CE-T1WI | Intensity histogram | 10th percentile | −2.025e-16 |
X8 | CE-T1WI | Intensity histogram | 10th percentile area | −0.324 |
X9 | CE-T1WI | GLCM | 333-4 correlation | 0.009 |
X10 | CE-T1WI | GLCM | 0-1 correlation | 0.548 |
X11 | CE-T1WI | GLCM | 180-1 correlation | 1.993e-14 |
X12 | CE-T1WI | GLCM | 270-7 correlation | −0.419 |
X13 | CE-T1WI | GLCM | 45-7 difference entropy | −1.686e-14 |
X14 | CE-T1WI | GLRLM | 0 short run low gray-level emphasis | −0.661 |
CE-T1WI, contrast-enhanced T1-weighted imaging; GLCM, gray-level co-occurrence matrix; GLRLM, gray-level run-length matrix; LASSO, least absolute shrinkage and selection operator.
Predictive performance of the models
The LR classifier demonstrated the best predictive performance in the training, test, and validation cohorts, with an AUC of 0.784, 0.779, and 0.800, respectively. The ROC and predictive performances of the 4 classifiers are shown in Figure S1 and Table 4. The odds ratio (OR) and P values of the 14 radiomics features are shown in Table S1. The combined model achieved the highest predictive performance in the training, test, and validation cohorts, with an AUC of 0.817, 0.822, and 0.845, respectively (Figure 3 and Table 5). Moreover, the DCs showed that the combined model consistently exhibited higher overall net benefits than did conventional radiological characteristics in the training (Figure 3), test, and validation cohorts (Figure S2).
Table 4
Classifiers | Cohort | AUC (95% CI) | Sensitivity | Specificity | Accuracy |
---|---|---|---|---|---|
LR | Training | 0.784 (0.716–0.852) | 69.0% | 69.0 % | 69.0% |
Test | 0.779 (0.673–0.886) | 63.9% | 75.0% | 69.4% | |
Validation | 0.800 (0.651–0.949) | 75.0% | 80.0% | 77.5% | |
DT | Training | 0.839 (0.781–0.898) | 78.6% | 79.8% | 79.2% |
Test | 0.605 (0.477–0.733) | 58.3% | 58.3% | 58.3% | |
Validation | 0.764 (0.614–0.913) | 60.0% | 85.0% | 72.5% | |
SVM | Training | 0.998 (0.994–1.000) | 96.4% | 95.2% | 95.5% |
Test | 0.769 (0.648–0.891) | 61.1% | 75.0% | 68.1% | |
Validation | 0.765 (0.601–0.929) | 50.0% | 95.0% | 72.5% | |
AdaBoost | Training | 0.732 (0.667–0.797) | 61.9% | 84.5% | 73.2% |
Test | 0.444 (0.333–0.556) | 75.0% | 35.0% | 55.0% | |
Validation | 0.450 (0.305–0.595) | 75.0% | 35.0% | 55.0% |
AdaBoost, adaptive boost; AUC, area under curve; CI, confidence interval; DT, decision tree; LR, logistic regression; SVM, support vector machine.
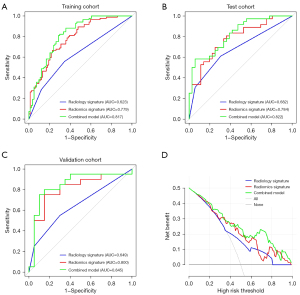
Table 5
Models | Cohort | AUC (95% CI) | Sensitivity | Specificity | Accuracy |
---|---|---|---|---|---|
Radiology | Training | 0.623 (0.547–0.699) | 56.0% | 65.4% | 60.7% |
Test | 0.682 (0.571–0.794) | 61.1% | 69.4% | 65.3% | |
Validation | 0.649 (0.495–0.802) | 55.0% | 70.0% | 62.5% | |
Radiomics | Training | 0.784 (0.716–0.852) | 69.0% | 69.0% | 69.0% |
Test | 0.779 (0.673–0.886) | 63.9% | 75.0% | 69.4% | |
Validation | 0.800 (0.651–0.949) | 75.0% | 80.0% | 77.5% | |
Combined | Training | 0.817 (0.753–0.881) | 79.8% | 71.4% | 75.6% |
Test | 0.822 (0.727–0.916) | 80.6% | 75.0% | 77.8% | |
Validation | 0.845 (0.708–0.982) | 75.0% | 85.0% | 80.0% |
AUC, area under curve; CI, confidence interval.
With the coefficients weighted by the LR algorithm, a radiomics score was calculated for each patient as follows: radiomics score = 16.884 + 0.962 × X1 – 3.176 × X2 – 2.661 × X3 – 1.418 × X4 – 7.242 × X5 – 0.418 × X6 + 0 × X7 – 0.922 × X8 + 0.717 × X9 + 2.355 × X10 + 0 × X11 + 0.289 × X12 + 0 × X13 – 5.429 × X14. A t-test confirmed that there were significant differences between Ki-67 PI high expression and low expression of the radiomics score in each cohort (Figure 4).
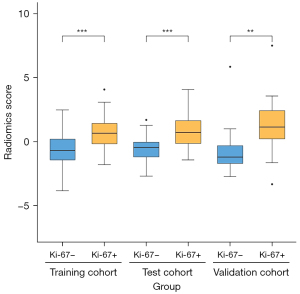
Development and validation of nomogram
The above analysis revealed that the radiomics score and enhanced homogeneity were significant predictive factors of Ki-67 expression in meningioma. The odds ratio (OR) and P values of the 2 variables are shown in Table S2. To facilitate the use in clinical practice, a nomogram was constructed for the combined model (Figure 5). In addition, the CCs of the radiomics nomogram demonstrated a good agreement between the predictive and actual performance of the Ki-67 expression status in each cohort (Figure 5).
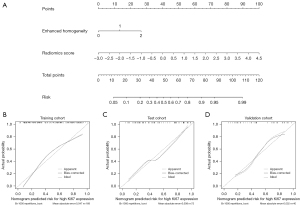
Discussion
In this study, we found the CE-T1WI–based radiomics features were predictive of Ki-67 expression in patients with meningioma. Subsequently, we developed and validated a radiomics nomogram, which combined a radiomics signature with radiological features to achieve higher sensitivity and accuracy than have previous models for individualized prediction of Ki-67 status (33).
The status of Ki-67 expression is recognized as a complementary factor, which reflects the biological behavior of meningioma. Preoperative assessment of Ki-67 expression can provide additional information for clinical decision-making (17-19,30). Previous studies have confirmed that using 4% as the cutoff value of Ki-67 in patients with meningioma can predict prognosis (18,26,27). However, since evaluation of Ki-67 is easily influenced by tumor heterogeneity, the whole specimen, and not only the core biopsy specimen, must be evaluated and correlated with mitotic count (31). A related study showed that the apparent diffusion coefficient (ADC) value of DWI was negatively correlated with Ki-67 level in meningioma (34). In another study, the metric kurtosis (MK) and radial kurtosis (RK) values of diffusion kurtosis imaging (DKI) were positively correlated with the Ki-67 level in meningioma (32). It appears that these quantitative parameters can be used as imaging biomarkers for predicting the expression of Ki-67 PI in meningioma. However, functional MRI sequences are not commonly used in clinical practice and merely provide a few quantitative parameters. In contrast, radiomics analysis cannot only extract thousands of quantitative radiomics features from routinely acquired images but can also evaluate the biological behavior of tumors in a timely and noninvasive manner (35,36).
Fourteen effective radiomics features were selected to construct classification models in this study. The correlations between the selected quantitative radiomics features and Ki-67 expression status can be interpreted on the basis of the biological behavior of tumors (37). Among the selected features, 3 were related to shape and size features because higher Ki-67 PI means the faster growth of tumors (33,38). In addition, 5 features were first-order statistical features, including entropy and percentile. Entropy, as one of the most representative parameters of tumor heterogeneity, can reflect the unpredictability of the information content in images (39). In general, entropy is positively correlated with signal dispersion, and the more scattered the tumor signals are, the stronger the uncertainty of the signals and the higher the entropy value in the ROI of the tumor (40). Entropy can make up for the deficiency of the resolution of the human eyes, which is not sufficiently high for identifying tumor heterogeneity in CE-T1WI images (41). The remaining features are texture-based features, including GLCM and GLRLM. As the second- or higher-order features pairwise relations between 2 or multi-neighboring pixels, GLCM and GLRLM can provide better results (42). It is conceivable that 2 kinds of meningioma with different Ki-67 expression statuses have a similar distribution of intensities with different spatial interrelationships. In such a situation, second- or higher-order features may be the preferred predictive factors.
Based on the selected radiomics features, we constructed and validated the radiomics models to predict the expression status of Ki-67 in meningioma. Among the 4 radiomics models, the LR-based model was superior at predicting status of Ki-67 expression with AUCs of 0.779 and 0.800 in the test cohort (internal validation) and validation cohort (external validation), respectively. In a recent study, a multiparametric MRI-based radiomics model was established to predict the Ki-67 expression of meningioma. This multiparametric model achieved an excellent performance in both the training and internal validation cohorts, with AUCs of 0.84 and 0.83, respectively (33). However, the robustness of the selected radiomics features and the generalization of the predictive model have not been proven due to a lack of external verification. In contrast, our result of external validation demonstrated that the predictive model established has a good stability and is valuable for preliminarily generalization. Furthermore, a previous study suggested that multiparametric MRI images can provide more radiomics features and achieve a better performance in the predictive model (33). Therefore, we extracted numerous radiomics features from multiparametric MRI images, including T1WI, T2WI, FLAIR, ADC, and CE-T1WI, to construct relative models for predicting Ki-67 expression in the pre-experimental setting. Unfortunately, the results showed that performances of the multiparametric MRI-based models were not as good as that of a CE-TIWI–based model even though we tried ANOVA, max-relevance, min-redundancy (mRMR), and LASSO regression to reduce the dimension of radiomics features. The main reason for this may be that too many radiomics features extracted from the multiparametric images led to poor fitting of the classifiers. Additionally, a multiparametric model requires patients to undergo additional MRI examinations, which could increase patients’ economic burden and hinder clinical practice (33,43). By contrast, our study demonstrated that the fewer radiomics features were included from CE-T1WI images, the better the efficacy was for predicting KI-67 expression in meningioma.
To further improve the accuracy and practical value of our model, we combined the radiomics signature with conventional radiological characteristics to construct a nomogram. Interestingly, the conventional radiological characteristic formed a good fit with the radiomics signature, and the AUCs of the combined model rose to 0.822 and 0.845 in the test and validation cohorts, respectively. It has been suggested that enhanced homogeneity could reflect the degree of damage to the blood–brain barrier and identify the heterogeneity of meningioma (42) in similar fashion to selected radiomics features. Compared with a previous study (44), the nomogram we established had a higher sensitivity (0.75 vs. 0.31, respectively) and a higher accuracy (0.80 vs. 0.56, respectively). In addition, this previous study was limited to predicting the status of Ki-67 of I grade meningioma (33), while the internal and external validations of the present nomogram showed that it has good predictive accuracy for Ki-67 status for various meningioma grades. Finally, the visual and quantitative prediction of Ki-67 expression was assessed using logistic regression to weight 2 predictors, and the usage of the nomogram is shown in Figure 6.
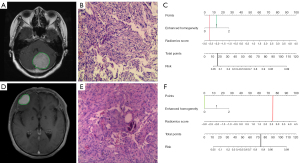
There are several limitations to our study. First, the design was retrospective and therefore had potential bias, and so additional studies that can prospectively validate our radiomics signature are needed. Second, we only validated whether the 1.5-Tesla–based model could be suitably applied in different machines (other 1.5-Tesla MR scanners) but did not validate different field strengths (3.0-Tesla). Third, we only constructed the radiomics signature based on CE-T1WI images due to the existence of overfitting, and further studies should address poor fitting to obtain better predictive effectiveness based on multiparametric images. Finally, the present study included only a relatively small number of participants enrolled from only 2 centers. To confirm the generalizability and the stability of the predictive model, future studies should be multicentered in design and recruit larger samples (35,45).
In conclusion, the CE-T1WI–based radiomics nomogram is a valuable reference tool for the preoperative assessment of cell proliferation during the onset of meningioma. It can accurately and robustly predict high Ki-67 expression in meningioma and provide supplementary information for accurate medical treatment and clinical decisions. However, extensive multicenter validations of the proposed approach are required prior to clinical application.
Acknowledgments
Funding: The study was supported by the Doctoral Scientific Foundation of the First Affiliated Hospital of Kunming Medical University (No. 2018BS019).
Footnote
Reporting Checklist: The authors completed the TRIPOD reporting checklist. Available at https://qims.amegroups.com/article/view/10.21037/qims-22-689/rc
Conflicts of Interest: All authors have completed the ICMJE uniform disclosure form (available at https://qims.amegroups.com/article/view/10.21037/qims-22-689/coif). The authors have no other conflicts of interest to declare.
Ethical Statement: The authors are accountable for all aspects of the work in ensuring that questions related to the accuracy or integrity of any part of the work are appropriately investigated and resolved. The study was conducted in accordance with the Declaration of Helsinki (as revised in 2013). This study was approved by the Ethics Committee of the First Affiliated Hospital of Kunming Medical University (No. 2021-L-6). Written informed consent was waived by the institutional review board.
Open Access Statement: This is an Open Access article distributed in accordance with the Creative Commons Attribution-NonCommercial-NoDerivs 4.0 International License (CC BY-NC-ND 4.0), which permits the non-commercial replication and distribution of the article with the strict proviso that no changes or edits are made and the original work is properly cited (including links to both the formal publication through the relevant DOI and the license). See: https://creativecommons.org/licenses/by-nc-nd/4.0/.
References
- Du Y, Lu T, Huang S, Ren F, Cui G, Chen J. Somatic mutation landscape of a meningioma and its pulmonary metastasis. Cancer Commun (Lond) 2018;38:16. [Crossref] [PubMed]
- Ostrom QT, Gittleman H, Liao P, Vecchione-Koval T, Wolinsky Y, Kruchko C, Barnholtz-Sloan JS. CBTRUS Statistical Report: Primary brain and other central nervous system tumors diagnosed in the United States in 2010-2014. Neuro Oncol 2017;19:v1-v88. [Crossref] [PubMed]
- Louis DN, Perry A, Reifenberger G, von Deimling A, Figarella-Branger D, Cavenee WK, Ohgaki H, Wiestler OD, Kleihues P, Ellison DW. The 2016 World Health Organization Classification of Tumors of the Central Nervous System: a summary. Acta Neuropathol 2016;131:803-20. [Crossref] [PubMed]
- Walsh KM. Epidemiology of meningiomas. Handb Clin Neurol 2020;169:3-15. [Crossref] [PubMed]
- Yuzawa S, Nishihara H, Tanaka S. Genetic landscape of meningioma. Brain Tumor Pathol 2016;33:237-47. [Crossref] [PubMed]
- Gallagher MJ, Jenkinson MD, Brodbelt AR, Mills SJ, Chavredakis E. WHO grade 1 meningioma recurrence: Are location and Simpson grade still relevant? Clin Neurol Neurosurg 2016;141:117-21. [Crossref] [PubMed]
- Narla S, Uppin MS, Saradhi MV, Sahu BP, Purohit AK, Sundaram C. Assessment of expression of epidermal growth factor receptor and p53 in meningiomas. Neurol India 2014;62:37-41. [Crossref] [PubMed]
- Rogers L, Barani I, Chamberlain M, Kaley TJ, McDermott M, Raizer J, Schiff D, Weber DC, Wen PY, Vogelbaum MA. Meningiomas: knowledge base, treatment outcomes, and uncertainties. A RANO review. J Neurosurg 2015;122:4-23. [Crossref] [PubMed]
- Bertero L, Dalla Dea G, Osella-Abate S, Botta C, Castellano I, Morra I, Pollo B, Calatozzolo C, Patriarca S, Mantovani C, Rudà R, Tardivo V, Zenga F, Garbossa D, Papotti M, Soffietti R, Ricardi U, Cassoni P. Prognostic Characterization of Higher-Grade Meningiomas: A Histopathological Score to Predict Progression and Outcome. J Neuropathol Exp Neurol 2019;78:248-56. [PubMed]
- Pham DT, Skaland I, Winther TL, Salvesen Ø, Torp SH. Correlation Between Digital and Manual Determinations of Ki-67/MIB-1 Proliferative Indices in Human Meningiomas. Int J Surg Pathol 2020;28:273-9. [Crossref] [PubMed]
- Roggendorf W, Schuster T, Peiffer J. Proliferative potential of meningiomas determined with the monoclonal antibody Ki-67. Acta Neuropathol 1987;73:361-4. [Crossref] [PubMed]
- Pan DH, Wen DY, Luo YH, Chen G, Yang H, Chen JQ, He Y. The diagnostic and prognostic values of Ki-67/MIB-1 expression in thyroid cancer: a meta-analysis with 6,051 cases. Onco Targets Ther 2017;10:3261-76. [Crossref] [PubMed]
- Besusparis J, Plancoulaine B, Rasmusson A, Augulis R, Green AR, Ellis IO, Laurinaviciene A, Herlin P, Laurinavicius A. Impact of tissue sampling on accuracy of Ki67 immunohistochemistry evaluation in breast cancer. Diagn Pathol 2016;11:82. [Crossref] [PubMed]
- Zhou Y, Hu W, Chen P, Abe M, Shi L, Tan SY, Li Y, Zong L. Ki67 is a biological marker of malignant risk of gastrointestinal stromal tumors: A systematic review and meta-analysis. Medicine (Baltimore) 2017;96:e7911. [Crossref] [PubMed]
- Tian Y, Ma Z, Chen Z, Li M, Wu Z, Hong M, Wang H, Svatek R, Rodriguez R, Wang Z. Clinicopathological and Prognostic Value of Ki-67 Expression in Bladder Cancer: A Systematic Review and Meta-Analysis. PLoS One 2016;11:e0158891. [Crossref] [PubMed]
- Berlin A, Castro-Mesta JF, Rodriguez-Romo L, Hernandez-Barajas D, González-Guerrero JF, Rodríguez-Fernández IA, González-Conchas G, Verdines-Perez A, Vera-Badillo FE. Prognostic role of Ki-67 score in localized prostate cancer: A systematic review and meta-analysis. Urol Oncol 2017;35:499-506. [Crossref] [PubMed]
- Telugu RB, Chowhan AK, Rukmangadha N, Patnayak R, Phaneendra BV, Prasad BC, Reddy MK. Histopathological and Immunohistochemical Evaluation of Meningiomas with Reference to Proliferative Markers p53 and Ki-67. J Clin Diagn Res 2016;10:EC15-9. [Crossref] [PubMed]
- Liu N, Song SY, Jiang JB, Wang TJ, Yan CX. The prognostic role of Ki-67/MIB-1 in meningioma: A systematic review with meta-analysis. Medicine (Baltimore) 2020;99:e18644. [Crossref] [PubMed]
- Bečulić H, Skomorac R, Jusić A, Alić F, Mašović A, Burazerović E, Omerhodžić I, Dorić M, Imamović M, Mekić-Abazović A, Efendić A, Udovčić-Gagula D. Correlation of peritumoral brain edema with morphological characteristics and ki67 proliferative index in resected intracranial meningiomas. Acta Clin Croat 2019;58:42-9. [PubMed]
- Ellis MJ, Suman VJ, Hoog J, Goncalves R, Sanati S, Creighton CJ, et al. Ki67 Proliferation Index as a Tool for Chemotherapy Decisions During and After Neoadjuvant Aromatase Inhibitor Treatment of Breast Cancer: Results From the American College of Surgeons Oncology Group Z1031 Trial (Alliance). J Clin Oncol 2017;35:1061-9. [Crossref] [PubMed]
- Lambin P, Leijenaar RTH, Deist TM, Peerlings J, de Jong EEC, van Timmeren J, Sanduleanu S, Larue RTHM, Even AJG, Jochems A, van Wijk Y, Woodruff H, van Soest J, Lustberg T, Roelofs E, van Elmpt W, Dekker A, Mottaghy FM, Wildberger JE, Walsh S. Radiomics: the bridge between medical imaging and personalized medicine. Nat Rev Clin Oncol 2017;14:749-62. [Crossref] [PubMed]
- Ding J, Zhao R, Qiu Q, Chen J, Duan J, Cao X, Yin Y. Developing and validating a deep learning and radiomic model for glioma grading using multiplanar reconstructed magnetic resonance contrast-enhanced T1-weighted imaging: a robust, multi-institutional study. Quant Imaging Med Surg 2022;12:1517-28. [Crossref] [PubMed]
- Ugga L, Perillo T, Cuocolo R, Stanzione A, Romeo V, Green R, Cantoni V, Brunetti A. Meningioma MRI radiomics and machine learning: systematic review, quality score assessment, and meta-analysis. Neuroradiology 2021;63:1293-304. [Crossref] [PubMed]
- Ugga L, Cuocolo R, Solari D, Guadagno E, D'Amico A, Somma T, Cappabianca P, Del Basso de Caro ML, Cavallo LM, Brunetti A. Prediction of high proliferative index in pituitary macroadenomas using MRI-based radiomics and machine learning. Neuroradiology 2019;61:1365-73. [Crossref] [PubMed]
- Sun X, Pang P, Lou L, Feng Q, Ding Z, Zhou J. Radiomic prediction models for the level of Ki-67 and p53 in glioma. J Int Med Res 2020;48:300060520914466. [Crossref] [PubMed]
- Abry E, Thomassen IØ, Salvesen ØO, Torp SH. The significance of Ki-67/MIB-1 labeling index in human meningiomas: a literature study. Pathol Res Pract 2010;206:810-5. [Crossref] [PubMed]
- Mirian C, Skyrman S, Bartek J Jr, Jensen LR, Kihlström L, Förander P, Orrego A, Mathiesen T. The Ki-67 Proliferation Index as a Marker of Time to Recurrence in Intracranial Meningioma. Neurosurgery 2020;87:1289-98. [Crossref] [PubMed]
- Xue C, Yuan J, Lo GG, Chang ATY, Poon DMC, Wong OL, Zhou Y, Chu WCW. Radiomics feature reliability assessed by intraclass correlation coefficient: a systematic review. Quant Imaging Med Surg 2021;11:4431-60. [Crossref] [PubMed]
- Wu S, Zheng J, Li Y, Yu H, Shi S, Xie W, Liu H, Su Y, Huang J, Lin T. A Radiomics Nomogram for the Preoperative Prediction of Lymph Node Metastasis in Bladder Cancer. Clin Cancer Res 2017;23:6904-11. [Crossref] [PubMed]
- Mukhopadhyay M, Das C, Kumari M, Sen A, Mukhopadhyay B, Mukhopadhyay B. Spectrum of meningioma with special reference to prognostic utility of ER,PR and Ki67 expression. J Lab Physicians 2017;9:308-13. [Crossref] [PubMed]
- Boros M, Moncea D, Moldovan C, Podoleanu C, Georgescu R, Stolnicu S. Intratumoral Heterogeneity for Ki-67 Index in Invasive Breast Carcinoma: A Study on 131 Consecutive Cases. Appl Immunohistochem Mol Morphol 2017;25:338-40. [Crossref] [PubMed]
- Xing F, Tu N, Koh TS, Wu G. MR diffusion kurtosis imaging predicts malignant potential and the histological type of meningioma. Eur J Radiol 2017;95:286-92. [Crossref] [PubMed]
- Khanna O, Fathi Kazerooni A, Farrell CJ, Baldassari MP, Alexander TD, Karsy M, Greenberger BA, Garcia JA, Sako C, Evans JJ, Judy KD, Andrews DW, Flanders AE, Sharan AD, Dicker AP, Shi W, Davatzikos C. Machine Learning Using Multiparametric Magnetic Resonance Imaging Radiomic Feature Analysis to Predict Ki-67 in World Health Organization Grade I Meningiomas. Neurosurgery 2021;89:928-36. [Crossref] [PubMed]
- Bozdağ M, Er A, Ekmekçi S. Association of apparent diffusion coefficient with Ki-67 proliferation index, progesterone-receptor status and various histopathological parameters, and its utility in predicting the high grade in meningiomas. Acta Radiol 2021;62:401-13. [Crossref] [PubMed]
- Gillies RJ, Kinahan PE, Hricak H. Radiomics: Images Are More than Pictures, They Are Data. Radiology 2016;278:563-77. [Crossref] [PubMed]
- Liu A, Wang Z, Yang Y, Wang J, Dai X, Wang L, Lu Y, Xue F. Preoperative diagnosis of malignant pulmonary nodules in lung cancer screening with a radiomics nomogram. Cancer Commun (Lond) 2020;40:16-24. [Crossref] [PubMed]
- Yip SS, Aerts HJ. Applications and limitations of radiomics. Phys Med Biol 2016;61:R150-66. [Crossref] [PubMed]
- Kim BW, Kim MS, Kim SW, Chang CH, Kim OL. Peritumoral brain edema in meningiomas: correlation of radiologic and pathologic features. J Korean Neurosurg Soc 2011;49:26-30. [Crossref] [PubMed]
- Molina D, Pérez-Beteta J, Martínez-González A, Martino J, Velásquez C, Arana E, Pérez-García VM. Influence of gray level and space discretization on brain tumor heterogeneity measures obtained from magnetic resonance images. Comput Biol Med 2016;78:49-57. [Crossref] [PubMed]
- Dastmalchian S, Kilinc O, Onyewadume L, Tippareddy C, McGivney D, Ma D, Griswold M, Sunshine J, Gulani V, Barnholtz-Sloan JS, Sloan AE, Badve C. Radiomic analysis of magnetic resonance fingerprinting in adult brain tumors. Eur J Nucl Med Mol Imaging 2021;48:683-93. [Crossref] [PubMed]
- Bi S, Li J, Wang T, Man F, Zhang P, Hou F, Wang H, Hao D. Multi-parametric MRI-based radiomics signature for preoperative prediction of Ki-67 proliferation status in sinonasal malignancies: a two-centre study. Eur Radiol 2022;32:6933-42. [Crossref] [PubMed]
- Cheng J, Huang W, Cao S, Yang R, Yang W, Yun Z, Wang Z, Feng Q. Correction: Enhanced Performance of Brain Tumor Classification via Tumor Region Augmentation and Partition. PLoS One 2015;10:e0144479. [Crossref] [PubMed]
- Hamerla G, Meyer HJ, Schob S, Ginat DT, Altman A, Lim T, Gihr GA, Horvath-Rizea D, Hoffmann KT, Surov A. Comparison of machine learning classifiers for differentiation of grade 1 from higher gradings in meningioma: A multicenter radiomics study. Magn Reson Imaging 2019;63:244-9. [Crossref] [PubMed]
- Zhao Y, Xu J, Chen B, Cao L, Chen C. Efficient Prediction of Ki-67 Proliferation Index in Meningiomas on MRI: From Traditional Radiological Findings to a Machine Learning Approach. Cancers (Basel) 2022;14:3637. [Crossref] [PubMed]
- Wang H, Xu X, Zhang X, Liu Y, Ouyang L, Du P, Li S, Tian Q, Ling J, Guo Y, Lu H. Elaboration of a multisequence MRI-based radiomics signature for the preoperative prediction of the muscle-invasive status of bladder cancer: a double-center study. Eur Radiol 2020;30:4816-27. [Crossref] [PubMed]