Multimodal investigation of bladder cancer data based on computed tomography, whole slide imaging, and transcriptomics
Introduction
With the advancements in medical diagnostic technologies, an abundance of multimodal data in clinic, such as pathology, radiology, and molecular characteristics, has enabled us to obtain multidimensional information about diseases, deepening our understanding of their nature. Integrative analysis of multimodal data can aid in identifying new features of complex diseases, such as cancer (1).
Bladder cancer is the ninth most common tumor worldwide and is the highest incidence tumor of the urinary system (2). Approximately 75% of newly diagnosed cases are non-muscle-invasive bladder cancer (NMIBC), which is characterized by painless hematuria, while approximately 25% of patients have muscle-invasive bladder cancer (MIBC), which has a 5-year survival rate as low as 40–60% (3-5).
Features of bladder cancer have been extensively studied at the subcellular level, and comprehensive molecular analysis has identified four molecular subtypes: basal, luminal, neuronal, and squamous (6,7). Recent studies have shown that molecular typing is correlated with the prognosis of patients and thus, is of value in predicting response to neoadjuvant chemotherapy (8-10). However, the confirmatory procedure requires the cancer tissues to be sequenced after surgery, and the difficulty in accessing specimens and the expense of sequencing limit its clinical application. One alternative to molecular typing is to infer the molecular type on the basis of other easily accessible features, such as radiological imaging features. Recently, histopathological slides have been used to predict the molecular subtype of MIBC based on convolution neural networks, providing a potential way to confirm the molecular subtypes (11).
For patients with cancer, CT images and histopathological slices are conventionally used for diagnosis. CT images primarily record patterns of density in the body, and histopathological slides usually serve as the gold standard for diagnosis. Quantitative omics, such as radiomics and pathomics, capture tissue or cell characteristics including morphology and density, and demonstrate great promise in clinical applications (12-16). Many previous studies on pathologic (17,18) and molecular typing (19,20), recurrence (21), and chemosensitivity (22) of bladder cancer have used machine learning based on CT or pathological whole slide imaging (WSI). Currently, the associations between molecular, pathological, and radiological features in bladder cancer remain obscure and have rarely been the focus of research.
In the present work, we aimed to investigate the relevance of quantitative imaging features to pathology, radiology, and molecular signatures in bladder cancer. To achieve this, we collected data at the molecular, cellular, and tissue levels. Additionally, we built a deep learning model to learn the latent associations between different modalities and attempt to predict the molecular subtypes of patients with bladder cancer. Finally, we analyzed the contributions of features in the model to distinguish certain subtypes using visualization strategies. We present the following article in accordance with the STARD reporting checklist (available at https://qims.amegroups.com/article/view/10.21037/qims-22-679/rc).
Methods
Patient cohorts and data filtering
The participants in this study were from three retrospective cohorts. Consecutive cases that met our inclusion criteria were enrolled. The inclusion criteria for patients were as follows: (I) had undergone transurethral resection or cystectomy and were pathologically confirmed to have bladder cancer; and (II) had not received tumor-related treatment (chemotherapy or radiotherapy) before surgery. The exclusion criteria were as follows: (I) patients whose contrast-enhanced CT imaging (before surgery), WSI, or clinicopathological diagnostic report was incomplete; and (II) patients with low-quality CT or WSI images.
The principle of sample collection was to obtain as many samples as possible in line with the inclusion and exclusion criteria. After selection, the first and second cohorts consisted of 19 (19 males) and 32 (4 females and 28 males) participants who underwent surgery in two first-level hospitals (The Third Affiliated Hospital of Shenzhen University and Cancer Hospital Chinese Academy of Medical Sciences) from 2018 to 2020. Arterial-phase CT images, WSI images, and clinicopathological diagnostic reports were gathered from the local hospital information system. Among the second cohort of 32 patients, 23 of bladder cancer tissues were RNA-sequenced. Patients from The Cancer Genome Atlas-Bladder Urothelial Carcinoma (TCGA-BLCA) cohort underwent transurethral resection or cystectomy between 2010 and 2014 (23). The original 120 arterial-phase CT images in Digital Imaging and Communications in Medicine (DICOM) format and the digital pathologic slices in WSI (40× magnification) were gathered from the open-source The Cancer Imaging Archive (TCIA) database. The corresponding clinicopathological, survival information, and raw count files measuring gene expression levels in bladder cancer cells were gathered from TCGA database. Finally, 76 participants (17 females and 59 males) were selected for the analysis.
The study was conducted in accordance with the Declaration of Helsinki (as revised in 2013) and was approved by the Research Ethics Committee of Shenzhen Luohu People’s Hospital (No. 2022-LHQRMYY-KYLL-067). All data used were acquired with institutional review board-approved protocols. The need to obtain informed consent was waived since this was a retrospective and observational study.
A flow diagram describing the data filtering and selection is shown in Figure 1. The basic and clinical characteristics of the participants are shown in Table 1. In summary, 127 patients (21 females and 106 males; mean age ± standard deviation, 65.6±10.1 years) who met the criteria were retrospectively analyzed in our study. There were 104 (81%) participants diagnosed with high-grade bladder cancer, and 79 (62%) participants diagnosed with MIBC. There was a class imbalance between the three cohorts (Table S1); therefore, we merged them into a single large dataset to perform the prediction task.
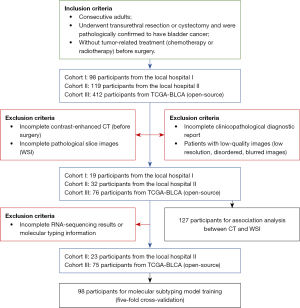
Table 1
Basic characteristics | Value or amount |
---|---|
Participants (cases) | 127 |
Female | 21 |
Male | 106 |
Age (years) | 65.6 (10.1)* |
Muscle invasiveness | |
Non-invasive | 48 |
Invasive | 79 |
Tumor grade | |
High grade | 104 |
Low grade | 23 |
Molecular subtype | |
Luminal | 71 |
Basal | 27 |
Not available | 29 |
* indicates the data are presented as the mean value (standard deviation).
Data preprocessing and feature extraction
Because the whole dataset consisted of three cohorts, batch effects posed a major challenge in data preprocessing. Generally, batch effects come from technical variation across samples. In CT imaging, slice thickness and gray values are the main variability-causing factors when images are from different cohorts. In WSI, the use of tissue samples from different laboratories can introduce systematic technical differences, such as depth of color, which are unrelated to the biological variation of tissues. Batch effects affect the mean and variance (location and spread) of the data and the performance of machine learning models. Although the causes of batch effects for each data modality are different, the methods are still applicable to different data modalities. For CT normalization in the present study, we adjusted the resolution of raw CT images to 1.5×1.0×1.0 mm3 using the interpolation method and normalized each image by centering it at the mean with standard deviation based on all gray values (24). We used ComBat to normalize the gene expression data (25,26). To remove batch effects in WSI images, we performed color normalization as described in previous studies (11,27).
We performed additional quality screening for the CT images in all three cohorts to exclude CT images of low resolution (slice thickness >5 mm) and CT images that did not contain the bladder. After the correction, the bladder and cancer regions in CT images were manually annotated by two radiologists, who had each been in clinical practice for more than 5 years, and two well-trained clinical graduate students. To speed up the annotation process, we trained a deep learning model based on the three-dimensional (3D) U-Net and performed automatic semantic segmentation on the rest of the data. Then, the segmentation results were examined and amended by radiologists, who further delineated accurate bladder and cancer boundaries. After annotation, the image voxel values were normalized, and 200 radiological features of the bladder and cancer voxels, roughly consisting of texture, morphological, and statistical features, were extracted using PyRadiomics (v 3.0.1) (24).
The pathologic cancer tissue slices were processed and digitalized at various institutions. To avoid a batch effect, each slice was split into tiles of 1,024×1,024 pixels and then normalized to the same reference tile as described by Anand et al. (27). The cancer cell phenotypes were quantified from the normalized tiles using CellProfiler software (v 4.1.3) (28). Four types of features of the nucleus and plasma of cells were extracted by CellProfiler: shape, intensity, texture, and radial distribution-related features. For each type of feature, statistical indicators, such as the mean, median, and standard deviation values, were calculated and concatenated for further analysis.
Expression levels of cancer genes were obtained through RNA sequencing of 23 participants from the second cohort. Raw gene counts were calculated through alignment to human reference genome GRCh38. We performed batch effect adjustment using ComBat, and then transcripts per million were calculated as the measurement of the gene expression level. We combined the bladder cancer molecular subtypes luminal, luminal-papillary, luminal-infiltrated, and neuronal as one class (luminal) and the basal subtype as the other class (basal). Molecular subtyping (luminal/basal) of bladder cancer was performed by hierarchical clustering with a panel of 48 genes according to the TCGA subtype approach (6). The molecular classification of the study participants is shown in Table 1.
The diagram of the integrative analysis framework is shown in Figure 2. The features of CT and WSI images were used to explore multimodal associations, molecular subtype prediction, and prognosis in patients with bladder cancer.
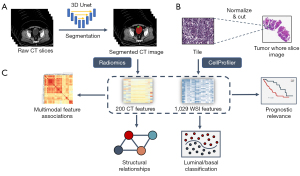
Multimodal association analysis
To explore the associations between CT and WSI features, feature selection was performed for the redundant WSI features, and we found that the patterns became much clearer after selection. We removed the highly correlated (Pearson correlation >0.7) WSI features since these features represent similar properties of WSI, such as smoothness. After selection, the pattern block of the cross-modal matrix could be identified, and we obtained 200 and 428 CT and WSI features, respectively, from the association analysis. First, the modal correlation matrices were calculated by HallA (v 0.8.18) (29). Then, blocks of associated features with significant correlations were determined by hierarchical clustering and the Gini impurity of the splits.
To further explore the statistical dependency between CT features, WSI features, clinicopathological characteristics, and molecular subtypes of bladder cancer, we constructed a structural equation model (SEM) using the aforementioned features with lavaan (v 0.6–8) (30). The standardized beta coefficient (β) was used to quantify the influence of the change in independent variables on the dependent variables. Other indices such as the root mean square error of approximation (RMSEA), compare fit index (CFI), Tucker-Lewis Index (TLI), and Standardized Root Mean Residual square (SRMR) were used to measure the model fitting effect.
Statistical analysis
The differential features of high- and low-grade cancer were identified by Welch’s two-sample t test. Cox regression analysis and survival analysis were used to assess the impact of multimodal features on the overall prognosis of patients with bladder cancer. All these machine learning experiments and statistical analyses were conducted in python (v3.8) and R (v3.6.3). A statistical result was considered significant when the P value was less than 0.05. The uncertainty of an estimate, such as its accuracy and the area under the receiver operating characteristic curve (AUC), was quantified at the 95% CI. To quantitatively measure the heterogeneity of bladder cancer across different scales, the Shannon entropy of the frequency distribution was calculated for each modality.
Deep learning algorithm and model interpretability analysis
A convolutional neural network was constructed to predict the molecular subtype for each participant with a multimodal feature fusion approach. To avoid overfitting, we randomly split the dataset into five parts and applied five-fold cross-validation. Performance metrics included accuracy and the AUC.
To explore the contributions of different features to model decision-making, a score-class activation map (Score-CAM) was used as a post hoc explanation method (31). With Score-CAM, a large score suggests that a feature affects the substance of the model’s decisions.
Results
Strong associations among multimodal features
A total of 200 CT and 428 WSI features from 127 participants were processed with HAllA to investigate the associations between modalities. Seventy-seven densely associated blocks were identified in the modal correlation matrix between CT and WSI features (Figure S1). Block 1 had the largest number of notable feature pairs clustered together (Figure 3A). The significance level of feature pairs in block 1 was verified using the Pearson correlation test. One feature pair of significance is illustrated in Figure 3B. A positive correlation (R=0.537±0.113; P<0.001) was found between cancer_firstorder_TE (CT feature: cancer first-order Total Energy) and nucleus_mean_Texture_CORH3 (WSI feature: nucleus Texture Correlation Hematoxylin; Figure 3B). The second and third blocks (plasmic and nucleic blocks) reflected the associations of plasmic and nucleic features of cancer cells with CT features, respectively (Figure S2). Texture features of cancer cell plasma are associated with gray-level matrix-relevant features in CT (P<1.49×10–6). Nucleus-related features were accompanied by first-order features that described the distribution of voxel intensities within the bladder (P<4.08×10–6).
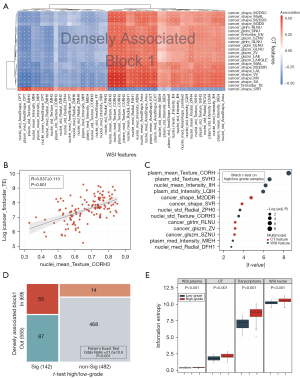
To explore the features with significant differences between high- and low-grade bladder cancer, we also performed a supervised analysis with a two-sample t test. Texture, intensity, and area shape-related features were distinctive in high- and low-grade WSI. Among the radiological features, shape-related features and gray level matrix (glcm, glrlm, and glszm) exhibited a significant difference between high- and low-grade bladder cancer (Figure 3C). Moreover, most features in block 1 were in line with the differential features between high- and low-grade bladder cancer (odds ratio =21.0±10.8; P<0.001; Figure 3D). This result suggests that the features in block 1 revealed the pathological grade characteristics (grade block).
High-grade bladder cancer typically presents with a higher level of heterogeneity than does low-grade bladder cancer (32). We quantified this heterogeneity using the Shannon entropy, which is a specific measure of randomness. Figure 3E shows the entropy of high- and low-grade bladder cancer for different modalities. High-grade cancer had higher entropy in all scales. Also, bladder cancer had higher entropy at the microscopic level than at the macro level, which reflected the disorder of cancer at the microscopic scale. We assessed the efficacy of Shannon entropy in predicting the outcome of patients with bladder cancer, and the results showed that the entropy level in the cancer cell nucleus and plasma had a significant impact on clinical prognosis (Figure S3).
Statistical dependency among multimodal data
According to previous results and related studies, we hypothesized that both CT and WSI features were related to clinicopathology and the luminal/basal molecular subtype in bladder cancer (11). To further explore the statistical dependency between multimodal data, we constructed a hypothetical path diagram (Figure 4) and validated the hypothesis by an SEM.
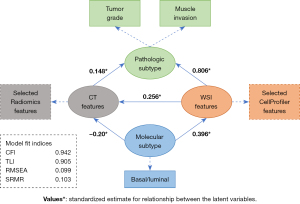
Specifically, texture, intensity, and area shape-related features were discriminative characteristics in high- and low-grade bladder cancer slides. High-grade samples tended to manifest with higher intensity and more variations in texture. The area shape of the nucleus in high-grade cancer cells was changeable, and a high coefficient of variation was observed in the radial intensity distribution in the nucleus. A similar phenomenon was observed in CT images. First-order statistics, describing the distribution of voxel intensities, in intensity level, nonuniformity, and zone variance in the gray level matrix (glcm, glrlm, and glszm) exhibited a significant difference between high- and low-grade cancer. These results are in line with the noteworthy pattern identified by HAllA, which demonstrated that the variation in texture and intensity distribution of bladder cancer cells in slides was correlated with the gray-level matrix variation of bladder cancer at the CT level.
The SEM analysis confirmed significant associations (P<0.05) along the proposed path. A strong association was observed between WSI features and pathologic subtype (β=0.806; P<0.001), which was consistent with pathological diagnosis. A moderate association was observed between WSI features and luminal/basal molecular subtype (β=0.396; P<0.001), which was in agreement with the conclusion that the molecular subtype of bladder cancer could be inferred by WSI features (11). Furthermore, weak associations were detected between CT features and pathologic subtype (β=0.148; P=0.007) and molecular subtype (β=–0.20; P=0.031), which indicated that molecular changes might also exert a certain influence on CT texture features. Fitting indices indicated that the proposed path fitted the data well (CFI: 0.942; TLI: 0.905; RMSEA: 0.099; SRMR: 0.103).
Molecular subtype prediction by multimodal feature fusion
Considering that the molecular signature of cancer impacted both CT features and WSI features, we constructed a deep learning model using features of CT and WSI to predict the luminal/basal subtype. As described in Figure 5A, a convolutional neural network for molecular subtype classification was constructed. Ninety-seven cases, each containing 200 CT features and 1,029 WSI features, were randomly split into a training set (79%, 77 cases) and a testing set (21%, 20 cases). Our model (Figure S4A) worked better than the unimodal models (Figure S4B,S4C) when either WSI or CT features were used (Figure S4D). The AUC was 0.89 (0.75–1.0 at the 95% CI) for luminal/basal molecular subtype classification on the fold-0 testing set (Figure 5B), while the average AUC of the five-fold cross-validation test was 0.84 (Figure S4E).
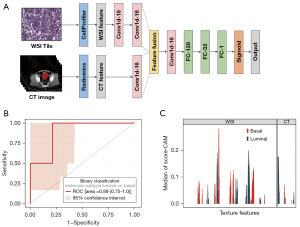
To explore the contribution of each feature to the model prediction, Score-CAM was used for the deep learning model. We calculated the score of each input feature for all the cases and took features with positive median scores as model-activated features (Figure 5C). In summary, the convolution neural network emphasized luminal/basal molecular classification. Features of WSI exhibited the highest scores and showed strong relations with molecular subtype, which was consistent with the results of the SEM analysis. The value of CT features in predicting the molecular subtype of bladder cancer was lower than that of WSI features.
Relevance of imaging features to prognosis
The molecular subtype of bladder cancer significantly impacts clinical prognosis; therefore, we performed Cox regression analysis on the features with positive median scores that influenced the prediction of molecular subtype (Figure 6A). Thirteen features of substance were identified; CT texture features, such as first-order statistics of voxel intensities (bladder_firstorder_10Percentile), and WSI features, such as the measurement in the nucleus area (nucleus_median_AreaShape_MinFeretDiameter), indicated a poor prognosis. The prognostic relevance of the 13 features was also verified by log rank test. Figure 6B illustrates the relevance of the nucleic WSI feature nucleus_StDev_Intensity_MeanIntensity_ Hematoxylin to overall survival in bladder cancer.
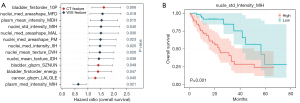
Discussion
In this paper, we delineated the multimodal characteristics of bladder cancer collected from 127 individuals on three different scales, namely at the molecular, cellular, and tissue levels. We identified novel associations in pathological and radiological features that distinguished high- and low-grade bladder cancer. Texture features of cancer cell plasma were found to be related to the gray level matrix features in CT, while the nucleic features reflected the intensity level of voxels in CT. Also, high-grade bladder cancer showed heterogeneity of significance across different scales, which was validated by the Shannon entropy, a generalizable indicator across all scales for grading and prognosis. We further explored the statistical dependency of modality features by an SEM and validated our main findings. A deep learning-based model was built to predict the molecular subtype of bladder cancer and achieved an average AUC of 0.84 in the five-fold cross-validation test.
There is some evidence of connections between medical imaging features and molecular characteristics in various cancers. Woerl et al. (11) constructed a deep convolution neural network to process WSI images and predict the basal, luminal and luminal p53-like molecular subtypes in bladder cancer. They achieved high predictive accuracy using only WSI images. Sirinukunwattana et al. (33) showed that four molecular subtypes defined by RNA expression profiles in colorectal cancer could be predicted using WSI images with a deep learning model. This classification model achieved a robust molecular-subtyping performance, with an AUC of 0.81 in the test set. Yan et al. (34) successfully developed a magnetic resonance imaging-based model for the prediction of glioma subtyping markers, including IDH gene mutation (AUC =0.88), 1p/19q chromosome deletion (AUC =0.82), and TERT gene promoter mutation (AUC =0.67). Lee et al. (35) investigated machine learning methods for magnetic resonance radiomics-based prediction of the luminal A and B, HER2-overexpressing, and triple-negative molecular subtypes of breast cancer. Among five machine learning methods, they found that an integrated random forest model showed the best performance (AUC =0.75). These studies evidence the potential of utilizing of medical images in the prediction of cancer molecular subtyping. Image-based models build a macro-to-micro connection between medical images and cancer genomes, and open the door for fast and low-cost molecular subtyping. Unlike these studies, our study aimed to determine the multimodal associations between radiological, pathologic, and molecular properties of bladder cancer. Based on the findings, we propose that the performance of molecular subtyping prediction could be improved by integrating multimodal medical images, including CT and WSI images.
Through multimodal association analysis, we found similar patterns at different scales within the same pathological grade. Additionally, nucleus-related features, such as the radial intensity distribution, were associated with basal molecular subtype in Score-CAM analysis. Pathological features are of particular importance in the prediction of molecular subtype and prognosis. Interestingly, bladder shape-related features on CT played an important role in predicting the luminal subtype. Unsupervised analysis revealed a similar feature association to supervised pattern detection, which indicates that these features are relevant to the transition in pathological type and can be used as an imaging biomarker.
Molecular features are crucial in today’s personalized treatment. The complex interactions between individuals’ molecular characteristics and phenotypes typically require diverse and large-scale data to be identified. A systematic multimodal data analysis approach is essential for the identification of novel signatures for diseases. Our work proffers a comprehensive framework for the signature recognition of multimodal data and is helpful in personalized medical management.
The present framework still has several limitations that require further improvement. First, sample imbalance existed—for example, in the number of patients with different tumor grades, batch effects (slice thickness and gray values) in CT images, and depth of color in WSI images—which might have caused potential bias and influenced the statistical results in the study. Although we applied normalization methods to the data, this bias could not be fully eliminated. Second, these findings remain to be replicated in a large population-based cohort before they can be applied in clinic. The limited number of samples may have resulted in a covariate shift; therefore, elements of our findings may be somewhat contingent. Additional efforts are needed to include more participants in future studies.
Conclusions
High-grade bladder cancer showed heterogeneity of significance across different scales. The Shannon entropy was used as an indicator to differentiate high- and low-grade bladder cancer and predict the outcome. The characteristics of the plasma and nucleus of cancer cells in the pathological slides were correlated with gray-level matrix characteristics and voxel intensity characteristics at the CT level. Further, CT features also contributed to the molecular subtype prediction, albeit not as much as WSI features.
Our work illustrates the complex associations between multimodal features in bladder cancer. Our results further prove that the fusion of multimodal features can achieve higher accuracy in predicting molecular subtypes of bladder cancer than can unimodal-based methods. This work demonstrates the potential of multimodal data analysis to be used in personalized medicine and related clinical applications.
Acknowledgments
We thank the open-source databases used in this study, including The Cancer Imaging Archive (TCIA) and The Cancer Genome Atlas (TCGA), for use of their data.
Funding: This work was supported by the National Natural Science Foundation Fund of China (No. 61931024), Shenzhen Science and Technology Program (No. JCYJ20220818100015031 and No. RCJC20200714114557005), the Guangdong Basic and Applied Basic Research Foundation (No. 2019A1515110038), and the Shenzhen Municipal Science and Technology Innovation Commission (No. JSGG20180712090411521).
Footnote
Reporting Checklist: The authors have completed the STARD reporting checklist. Available at https://qims.amegroups.com/article/view/10.21037/qims-22-679/rc
Conflicts of Interest: All authors have completed the ICMJE uniform disclosure form (available at https://qims.amegroups.com/article/view/10.21037/qims-22-679/coif). The authors have no conflicts of interest to declare.
Ethical Statement: The authors are accountable for all aspects of the work in ensuring that questions related to the accuracy or integrity of any part of the work are appropriately investigated and resolved. The study was conducted in accordance with the Declaration of Helsinki (as revised in 2013). This study was approved by the Research Ethics Committee of Shenzhen Luohu People’s Hospital (No. 2022-LHQRMYY-KYLL-067). All data used were acquired with institutional review board-approved protocols. The need to obtain informed consent was waived since this was a retrospective and observational study.
Open Access Statement: This is an Open Access article distributed in accordance with the Creative Commons Attribution-NonCommercial-NoDerivs 4.0 International License (CC BY-NC-ND 4.0), which permits the non-commercial replication and distribution of the article with the strict proviso that no changes or edits are made and the original work is properly cited (including links to both the formal publication through the relevant DOI and the license). See: https://creativecommons.org/licenses/by-nc-nd/4.0/.
References
- Gao J, Li P, Chen Z, Zhang J. A Survey on Deep Learning for Multimodal Data Fusion. Neural Comput 2020;32:829-64. [Crossref] [PubMed]
- Siegel RL, Miller KD, Fuchs HE, Jemal A. Cancer statistics, 2022. CA Cancer J Clin 2022;72:7-33. [Crossref] [PubMed]
- Kamat AM, Hahn NM, Efstathiou JA, Lerner SP, Malmström PU, Choi W, Guo CC, Lotan Y, Kassouf W. Bladder cancer. Lancet 2016;388:2796-810. [Crossref] [PubMed]
- Alfred Witjes J, Lebret T, Compérat EM, Cowan NC, De Santis M, Bruins HM, Hernández V, Espinós EL, Dunn J, Rouanne M, Neuzillet Y, Veskimäe E, van der Heijden AG, Gakis G, Ribal MJ. Updated 2016 EAU Guidelines on Muscle-invasive and Metastatic Bladder Cancer. Eur Urol 2017;71:462-75. [Crossref] [PubMed]
- Saginala K, Barsouk A, Aluru JS, Rawla P, Padala SA, Barsouk A. Epidemiology of Bladder Cancer. Med Sci (Basel) 2020.
- Robertson AG, Kim J, Al-Ahmadie H, Bellmunt J, Guo G, Cherniack AD, et al. Comprehensive Molecular Characterization of Muscle-Invasive Bladder Cancer. Cell 2017;171:540-556.e25. [Crossref] [PubMed]
- Comprehensive molecular characterization of urothelial bladder carcinoma. Nature 2014;507:315-22. [Crossref] [PubMed]
- McConkey DJ, Choi W. Molecular Subtypes of Bladder Cancer. Curr Oncol Rep 2018;20:77. [Crossref] [PubMed]
- Seiler R, Ashab HAD, Erho N, van Rhijn BWG, Winters B, Douglas J, et al. Impact of Molecular Subtypes in Muscle-invasive Bladder Cancer on Predicting Response and Survival after Neoadjuvant Chemotherapy. Eur Urol 2017;72:544-54. [Crossref] [PubMed]
- Tan TZ, Rouanne M, Tan KT, Huang RY, Thiery JP. Molecular Subtypes of Urothelial Bladder Cancer: Results from a Meta-cohort Analysis of 2411 Tumors. Eur Urol 2019;75:423-32. [Crossref] [PubMed]
- Woerl AC, Eckstein M, Geiger J, Wagner DC, Daher T, Stenzel P, Fernandez A, Hartmann A, Wand M, Roth W, Foersch S. Deep Learning Predicts Molecular Subtype of Muscle-invasive Bladder Cancer from Conventional Histopathological Slides. Eur Urol 2020;78:256-64. [Crossref] [PubMed]
- Yuan J, Xue C, Lo G, Wong OL, Zhou Y, Yu SK, Cheung KY. Quantitative assessment of acquisition imaging parameters on MRI radiomics features: a prospective anthropomorphic phantom study using a 3D-T2W-TSE sequence for MR-guided-radiotherapy. Quant Imaging Med Surg 2021;11:1870-87. [Crossref] [PubMed]
- Ou J, Wu L, Li R, Wu CQ, Liu J, Chen TW, Zhang XM, Tang S, Wu YP, Yang LQ, Tan BG, Lu FL. CT radiomics features to predict lymph node metastasis in advanced esophageal squamous cell carcinoma and to discriminate between regional and non-regional lymph node metastasis: a case control study. Quant Imaging Med Surg 2021;11:628-40. [Crossref] [PubMed]
- Wu K, Wu P, Yang K, Li Z, Kong S, Yu L, Zhang E, Liu H, Guo Q, Wu S. A comprehensive texture feature analysis framework of renal cell carcinoma: pathological, prognostic, and genomic evaluation based on CT images. Eur Radiol 2022;32:2255-65. [Crossref] [PubMed]
- Cao R, Yang F, Ma SC, Liu L, Zhao Y, Li Y, Wu DH, Wang T, Lu WJ, Cai WJ, Zhu HB, Guo XJ, Lu YW, Kuang JJ, Huan WJ, Tang WM, Huang K, Huang J, Yao J, Dong ZY. Development and interpretation of a pathomics-based model for the prediction of microsatellite instability in Colorectal Cancer. Theranostics 2020;10:11080-91. [Crossref] [PubMed]
- Feng L, Liu Z, Li C, Li Z, Lou X, Shao L, et al. Development and validation of a radiopathomics model to predict pathological complete response to neoadjuvant chemoradiotherapy in locally advanced rectal cancer: a multicentre observational study. Lancet Digit Health 2022;4:e8-e17. [Crossref] [PubMed]
- Zhang G, Xu L, Zhao L, Mao L, Li X, Jin Z, Sun H. CT-based radiomics to predict the pathological grade of bladder cancer. Eur Radiol 2020;30:6749-56. [Crossref] [PubMed]
- Yang Y, Zou X, Wang Y, Ma X. Application of deep learning as a noninvasive tool to differentiate muscle-invasive bladder cancer and non-muscle-invasive bladder cancer with CT. Eur J Radiol 2021;139:109666. [Crossref] [PubMed]
- Loeffler CML, Ortiz Bruechle N, Jung M, Seillier L, Rose M, Laleh NG, Knuechel R, Brinker TJ, Trautwein C, Gaisa NT, Kather JN. Artificial Intelligence-based Detection of FGFR3 Mutational Status Directly from Routine Histology in Bladder Cancer: A Possible Preselection for Molecular Testing? Eur Urol Focus 2022;8:472-9. [Crossref] [PubMed]
- Velmahos CS, Badgeley M, Lo YC. Using deep learning to identify bladder cancers with FGFR-activating mutations from histology images. Cancer Med 2021;10:4805-13. [Crossref] [PubMed]
- Tokuyama N, Saito A, Muraoka R, Matsubara S, Hashimoto T, Satake N, Matsubayashi J, Nagao T, Mirza AH, Graf HP, Cosatto E, Wu CL, Kuroda M, Ohno Y. Prediction of non-muscle invasive bladder cancer recurrence using machine learning of quantitative nuclear features. Mod Pathol 2022;35:533-8. [Crossref] [PubMed]
- Mi H, Bivalacqua TJ, Kates M, Seiler R, Black PC, Popel AS, Baras AS. Predictive models of response to neoadjuvant chemotherapy in muscle-invasive bladder cancer using nuclear morphology and tissue architecture. Cell Rep Med 2021;2:100382. [Crossref] [PubMed]
- Lerner SP, Duddalwar V, Huang E, Altun E, Bathala T, Kennish S, Ibarra J, Lucchesi F, Muglia VF, Thomas S, Vikram R, Vargas HA, Cen SY, Hwang D, King KG, Varghese B, Fevrier-Sullivan B, Kirby J, Jaffe C, Freymann J. Comprehensive radiogenomics analysis of qualitative and quantitative features of cross-sectional imaging in the TCGA project in MIBC. J Clin Oncol 2019;37:482. [Crossref]
- van Griethuysen JJM, Fedorov A, Parmar C, Hosny A, Aucoin N, Narayan V, Beets-Tan RGH, Fillion-Robin JC, Pieper S, Aerts HJWL. Computational Radiomics System to Decode the Radiographic Phenotype. Cancer Res 2017;77:e104-7. [Crossref] [PubMed]
- Johnson WE, Li C, Rabinovic A. Adjusting batch effects in microarray expression data using empirical Bayes methods. Biostatistics 2007;8:118-27. [Crossref] [PubMed]
- Zhang Y, Parmigiani G, Johnson WE. ComBat-seq: batch effect adjustment for RNA-seq count data. NAR Genom Bioinform 2020;2:lqaa078. [Crossref] [PubMed]
- Anand D, Ramakrishnan G, Sethi A. Fast GPU-Enabled Color Normalization for Digital Pathology. 2019 International Conference on Systems, Signals and Image Processing (IWSSIP) 2019:219-24.
- Jones TR, Carpenter AE, Lamprecht MR, Moffat J, Silver SJ, Grenier JK, Castoreno AB, Eggert US, Root DE, Golland P, Sabatini DM. Scoring diverse cellular morphologies in image-based screens with iterative feedback and machine learning. Proc Natl Acad Sci U S A 2009;106:1826-31. [Crossref] [PubMed]
- Ghazi AR, Sucipto K, Rahnavard A, Franzosa EA, McIver LJ, Lloyd-Price J, Schwager E, Weingart G, Moon YS, Morgan XC, Waldron L, Huttenhower C. High-sensitivity pattern discovery in large, paired multiomic datasets. Bioinformatics 2022;38:i378-85. [Crossref] [PubMed]
- Rosseel Y. lavaan: An R Package for Structural Equation Modeling. 2012 2012;48:36.
- Wang H, Wang Z, Du M, Yang F, Zhang Z, Ding S, Mardziel P, Hu X. Score-CAM: Score-weighted visual explanations for convolutional neural networks. Proceedings of the IEEE/CVF Conference on Computer Vision and Pattern Recognition Workshops 2020:24-5.
- Cheng L, Neumann RM, Nehra A, Spotts BE, Weaver AL, Bostwick DG. Cancer heterogeneity and its biologic implications in the grading of urothelial carcinoma. Cancer 2000;88:1663-70. [Crossref] [PubMed]
- Sirinukunwattana K, Domingo E, Richman SD, Redmond KL, Blake A, Verrill C, et al. Image-based consensus molecular subtype (imCMS) classification of colorectal cancer using deep learning. Gut 2021;70:544-54. [Crossref] [PubMed]
- Yan J, Zhang B, Zhang S, Cheng J, Liu X, Wang W, Dong Y, Zhang L, Mo X, Chen Q, Fang J, Wang F, Tian J, Zhang S, Zhang Z. Quantitative MRI-based radiomics for noninvasively predicting molecular subtypes and survival in glioma patients. NPJ Precis Oncol 2021;5:72. [Crossref] [PubMed]
- Lee JY, Lee KS, Seo BK, Cho KR, Woo OH, Song SE, Kim EK, Lee HY, Kim JS, Cha J. Radiomic machine learning for predicting prognostic biomarkers and molecular subtypes of breast cancer using tumor heterogeneity and angiogenesis properties on MRI. Eur Radiol 2022;32:650-60. [Crossref] [PubMed]