Influence of computed tomography slice thickness on deep learning-based, automatic coronary artery calcium scoring software performance
Introduction
The coronary artery calcium (CAC) score obtained from a noncontrast-enhanced, electrocardiogram (ECG)-gated computed tomography (CT) scan is a strong predictor of adverse cardiovascular events in asymptomatic individuals (1). Conventional CAC scoring is performed by manual identification of the calcified coronary artery lesions in each image using dedicated software, which is labor-intensive and time-consuming. Artificial intelligence could help replace this tedious work and increase clinical efficiency.
Several studies evaluated deep learning (DL)-based, automatic CAC scoring software using standard cardiac CT and chest CT (2-5). A recent validation study demonstrated that a DL-based, atlas-based automatic CAC scoring system showed high reliability for Agatston score (AS) and volume [intraclass correlation coefficient (ICC) 0.99 for both] measurements and a high accuracy for risk categorization [kappa (κ) value =0.94] in three cardiac CT cohort datasets from a single institution (6).
Standard CAC scoring on noncontrast-enhanced ECG-gated cardiac CT requires 2.5–3 mm slice thickness scan. However, CT images with thinner slice reconstruction tend to increase the detection of small CACs and result in higher CAC scores than the standard 3 mm slice thickness (7,8). Moreover, non-ECG-gated chest CT with thinner slices (1–1.25 mm) has been increasingly used for the assessment of the presence and severity of CACs (9,10). Although thinner slices may improve the reliability of CAC scoring by decreasing partial volume effects, they increase image noise, which may negatively affect the automatic detection of CACs. To date, the performance of DL-based, automatic CAC scoring software has not been explored for thinner slice thickness. We hypothesized that a cardiac CT scan reconstructed with thinner slices would increase false-positive results and lower the performance of DL-based, automatic CAC scoring software.
The aim of this study was to evaluate the influence of CT slice thickness on the performance of DL-based, automatic CAC scoring software by evaluating the agreement of CAC scores and risk category classification at 1.5 and 3 mm slice thickness, CAC scoring CT with manual scoring as the reference standard.
Methods
Patients
The study was conducted in accordance with the Declaration of Helsinki (as revised in 2013). The study was approved by the institutional review board of Severance Hospital and individual consent for this retrospective analysis was waived.
We retrospectively enrolled 1,058 consecutive CT examinations obtained from asymptomatic subjects who underwent cardiac CT for health evaluation at our institution between September 2013 and October 2020. Images of CAC scoring scans in cardiac CT for health evaluation were reconstructed with two slice thickness values (1.5 and 3 mm). Patients with prior history of coronary revascularization (n=30) and without a complete set of reconstructions of two different slice thickness (n=151) were excluded. If a patient had multiple cardiac CT scans (n=33), we included only the most recent examination. A total of 844 patients (mean age, 58.9±10.7; 477 men) consisted of final study population (Figure 1). Of these patients, 550 were included in a study by Kim et al. (8). The previous study focused on the prognostic value of CAC scores from 1.5 mm slice reconstructions of ECG-gated CT scan in asymptomatic subjects. However, our study focused on the impact of CT slice thickness on DL-based automatic CAC scoring software performance.
CT image acquisition
All subjects underwent cardiac CT using second- or third-generation dual-source CT scanner (SOMATOM Definition Flash or SOMATOM Definition Force, Siemens Healthineers). The standard protocol by the Society of Cardiovascular Computed Tomography was used for image acquisition (11). Noncontrast CT images for CAC scoring were performed with a prospective ECG-gated acquisition protocol (tube voltage; 120 kVp, tube current; 50 mA). Images were obtained with prospective ECG-gating at 70% or 35% of the R-R interval, depending on the heart rate. A medium-sharp kernel and filtered back projection (B35f), specifically designed to enhance the depiction of calcifications, were used for image reconstruction.
From the acquired raw data, the whole volume was reconstructed in nonoverlapping datasets of 1.5 and 3 mm slice thickness. The radiation dose of CAC scoring CT was assessed using the dose-length product.
Automatic CAC scoring software overview
For automatic CAC scoring, we used commercial, DL-based, automated CAC scoring software (AVIEW CAC, Coreline Soft, Co. Ltd.), which automatically calculates the CAC score based on the 3D U-net architecture. First, the software finds the four main coronary arteries: the left main artery (LM), left anterior descending artery (LAD), left circumflex artery (LCX), and right coronary artery (RCA). Second, it finds the regions with attenuation higher than 130 HU that are regarded as calcium candidates on CT images. Then, CAC is calculated by intersecting the segmented coronary artery and the regions considered as calcium candidates. Details of the DL algorithm are specified in a recent study (6). All dataset for training the algorithm was noncontrast-enhanced, ECG-gated CT with 2.5 or 3 mm slice thickness. There was no image preprocessing step for 1.5 mm slice thickness scan before an inference.
The automated analysis software provides AS, volume, and mass score; we used AS and calcium volume (mm3) for data analysis in this study. To exclude noise, we set a calcium candidate to have at least three pixels with attenuation higher than 130 HU on both manual and automatic scoring methods.
Reference standard CAC scoring and data reporting
A manual CAC scoring method was set as the reference standard, with the use of post-processing software (AVIEW CAC, Coreline Soft, Co. Ltd.). All 1,688 CT scans were analyzed, in consensus, by two radiologists (YJS and SYK) with 13 and 3 years of experience in cardiac CT, respectively. Any discrepancies in individual scoring were resolved through discussion. The images were randomly ordered, and the observers were blinded to the CAC scores on other slice thickness images or scores by another reader. Images with 3 mm thickness were reviewed first, followed by the 1.5 mm images. A minimum of 1 month was scheduled as a washout period between the scoring of two different slice thickness images to reduce recall bias. Every calcified coronary region of interest was manually identified and color-coded according to the anatomical location. Then, the software calculated the total AS and calcium volume (mm3) by summation of the individual lesions with CACs. The result of CAC scoring was respectively reported at the total and per-vessel levels, i.e., LM, LAD, LCx, and RCA. We classified the CAC severity of the subjects into four categories based on AS: none (score =0), mild (score 1–100), moderate (score 101–400), and severe (score >400) (12). One hundred (11.8%) CT scans were randomly selected for both thickness values, with even distribution over the CAC severity categories (25 cases in each category), to evaluate inter-observer agreement of manual CAC scoring. Image noise was defined as the standard deviation (SD) of the measured pixel values in HU within a circular region of interest in the ascending aorta at the level of the LM and measured on the 1.5 and 3 mm slice images.
Per-lesion analysis
Per-lesion comparisons between the manual and automatic methods were conducted on both 1.5 and 3 mm scans. Calcified coronary lesions were selected based on the structural information generated by the automatic software. All the mismatched lesions were reviewed by two radiologists to analyze the cause of the mismatches and the lesion locations. The mismatched lesions were divided into false-positive results (e.g., wrong vessel segmentation or image noise) and false-negative results (i.e., coronary calcifications missed by automatic software) errors. The per-lesion sensitivity was calculated as (true positive lesion number/total lesion number) ×100. The false-positive rate was calculated as (number of false-positive lesions/total patient number).
Statistical analysis
The mean, SD, and median values were calculated for AS and calcium volume (mm3) obtained using the reference standard and the automatic software. The paired t-test was applied to determine the statistical significance of the differences in the image noise between 1.5 and 3 mm scans. Inter-observer agreement of manual CAC scoring and the agreement of the reference standard and the automatic software for AS and calcium volume (mm3) were evaluated using ICC and Bland-Altman analysis with 95% limits of agreement (LOA) for both 1.5 and 3 mm datasets. ICCs were interpreted as follows: 0.50, poor; 0.50–0.75, moderate; 0.75–0.9, good; and 0.9–1.0, excellent. We applied the half-normal distribution method for non-uniform differences, since the measurement error of CAC score increased with higher CAC scores (13). The 95% repeatability limits were calculated by multiplying the coefficient by (14). The agreement of the CAC severity categories (AS 0, 1–100, 101–400, >400) between automatic CAC scoring and the reference standard was analyzed using weighted κ statistics for both 1.5 and 3 mm datasets. Kappa values were interpreted as follows: <0.4, poor; 0.41–0.6, moderate; 0.61–0.8, good; and 0.81–1.0, excellent agreement. A P value of <0.05 was considered statistically significant. All analyses were performed using SAS (version 9.2, SAS Institute Inc.).
Results
Patient characteristics
Four-hundred seventy-seven of 844 subjects (56.5%) were men, and the mean age was 58.9±10.7 years (Table 1). The mean body mass index was 24.0±2.8 kg/m2 (range, 15.6 to 36.8 kg/m2). Prevalence of diabetes mellitus, hypertension, and dyslipidemia were 16.5%, 24.4%, and 42.9%, respectively, and 16.6% were current smokers.
Table 1
Variables | Patients (n=844) |
---|---|
Age (years) | 58.9±10.7 |
Male, n (%) | 477 (56.5) |
Body mass index (kg/m2) | 24.0±2.8 |
Hypertension, n (%) | 206 (24.4) |
Diabetes mellitus, n (%) | 139 (16.5) |
Dyslipidemia, n (%) | 362 (42.9) |
Systolic blood pressure (mmHg) | 123.2±15.4 |
Smoking history, n (%) | |
Never smoker | 481 (57.0) |
Former smoker | 218 (25.8) |
Current smoker | 139 (16.5) |
Not available | 6 (0.7) |
Pack-years of smoking (n=50) | 38.4±22.9 |
Total cholesterol (mg/dL) | 195.6±39.8 |
HDL cholesterol (mg/dL) | 52.7±13.8 |
LDL cholesterol (mg/dL) | 117.3±35.5 |
Data expressed as number (%) or mean ± standard deviation. HDL, high-density lipoprotein; LDL, low-density lipoprotein.
Inter-observer agreement of manual CAC scoring and agreement between automatic scoring and reference standard
Inter-observer agreement for manual CAC scoring was excellent on both 1.5 and 3 mm slice thickness scans (ICC 0.99 and κ value for risk category 0.992 for both). Bland-Altman plots show the difference between CAC scores by two readers (Figure S1). A confusion matrix for the categorization of CAC severity between both readers is presented in Table S1.
Based on manual scoring, the median AS was 4.28 (interquartile range, 0–55.74) for 1.5 mm scans and median 0 (interquartile range, 0–31.43) for 3 mm scans (Table 2). With the manual scoring method, the proportion of subjects with an AS of zero was 281/844 (33.3%) and 478/844 (56.6%) for 1.5 and 3 mm scans, respectively. There was excellent agreement in AS between 1.5 and 3 mm slice thickness scans with both manual and automatic scoring methods (ICC 0.967 for both; Table S2). The agreement for CAC severity categorization between 1.5 and 3 mm slice thickness reconstructions was good for both reference standard and automatic software [weighted κ; 0.711 (95% confidence interval, 0.675–0.747) for reference standard and 0.634 (95% confidence interval, 0.594–0.675) for automatic software]. The agreement between the automatic software and the reference standard with respect to the AS and calcium volume (mm3) is summarized in Table 2. For the AS, automatically obtained CAC scores yielded high ICCs for both 1.5 and 3 mm scans (0.982 and 0.969, respectively). For the per-vessel evaluation, the ICCs for the AS of the LM, LAD, LCX, and RCA were 0.810, 0.975, 0.895, and 0.973 for 1.5 mm scans and 0.805, 0.936, 0.867, and 0.982 for 3 mm scans (Table 2). Bland-Altman plots show differences between CAC scores obtained from two methods (Figure 2). ICC for calcium volume was also high for both 1.5 and 3 mm scans (0.980 and 0.970, respectively), with similar values yielded by the ICC for AS in the per-vessel analysis. The mean dose-length product was 51.0±6.0 mGy∙cm (range 15 to 96 mGy∙cm). Image noise was significantly lower in 3 mm thickness than that with 1.5 mm slice thickness (mean ± SD, 19.8±4.6 vs. 27.1±6.4, P<0.001).
Table 2
Index | Total | LM | LAD | LCX | RCA |
---|---|---|---|---|---|
1.5 mm slice thickness | |||||
Agatston score, median | 4.28 [0, 55.74] | 0 [0, 0] | 0 [0, 30.68] | 0 [0, 1.22] | 0.58 [0, 9.09] |
Agatston score, ICC | 0.982 (0.979, 0.984) | 0.810 (0.785, 0.832) | 0.975 (0.971, 0.978) | 0.895 (0.881, 0.908) | 0.973 (0.97, 0.977) |
Agatston score, LOA | 10.101 (6.219, 13.983) | −0.222 (−1.498, 1.055) | 4.300 (2.276, 6.323) | 2.315 (0.724, 3.906) | 3.708 (1.644, 5.772) |
Volume, mm3, median | 8.86 [0, 58.55] | 0 [0, 0] | 0 [0, 27.77] | 0 [0, 3.34] | 1.73 [0, 16.04] |
Volume, mm3, ICC | 0.980 (0.977, 0.982) | 0.810 (0.785, 0.832) | 0.976 (0.972, 0.979) | 0.896 (0.882, 0.908) | 0.966 (0.961, 0.97) |
Volume, mm3, LOA | 8.071 (4.73, 11.413) | −0.338 (−1.335, 0.659) | 3.359 (1.793, 4.925) | 1.603 (0.303, 2.904) | 3.447 (1.508, 5.387) |
3 mm slice thickness | |||||
Agatston score, median | 0 [0, 31.43] | 0 [0, 0] | 0 [0, 15.92] | 0 [0, 0] | 0 [0, 1.37] |
Agatston score, ICC | 0.969 (0.965, 0.973) | 0.805 (0.78, 0.827) | 0.936 (0.927, 0.944) | 0.867 (0.849, 0.883) | 0.982 (0.979, 0.984) |
Agatston score, LOA | 6.648 (2.013, 11.282) | −0.732 (−2.058, 0.594) | 3.641 (0.538, 6.744) | 2.18 (0.541, 3.818) | 1.559 (0.065, 3.053) |
Volume, mm3, median | 0 [0, 34.38] | 0 [0, 0] | 0 [0, 18.42] | 0 [0, 0] | 0 [0, 4.06] |
Volume, mm3, ICC | 0.970 (0.966, 0.974) | 0.798 (0.772, 0.822) | 0.942 (0.933, 0.949) | 0.874 (0.857, 0.889) | 0.977 (0.974, 0.98) |
Volume, mm3, LOA | 5.878 (2.019, 9.737) | −0.641 (−1.723, 0.441) | 3.005 (0.607, 5.404) | 1.918 (0.58, 3.257) | 1.595 (0.133, 3.057) |
Numbers in parentheses indicate 95% confidence intervals. Numbers in brackets indicate interquartile range. ICC, intraclass correlation coefficient; LOA, limits of agreement; LAD, left anterior descending artery; LCX, left circumflex artery; LM, left main artery; RCA, right coronary artery.
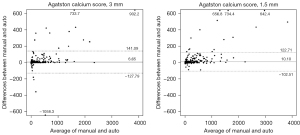
Categorical agreement of CAC severity between automatic scoring and reference standard
A confusion matrix for the categorization of CAC severity is presented in Table 3. The categorical agreement of CAC severity between automatic scoring and the manual reference standard was excellent for both 1.5 and 3 mm scans, with better agreement for 3 mm scans [weighted κ; 0.851 (95% confidence interval, 0.823–0.879) for 1.5 mm and 0.961 (95% confidence interval, 0.945–0.974) for 3 mm]. In total, 106 (12.6%) and 30 (3.5%) scans were misclassified by automatic scoring in the 1.5 and 3 mm scans, respectively; 81 were overestimated and 25 underestimated in the 1.5 mm scans, and 21 were overestimated and nine underestimated in the 3 mm scans. All misclassified scans in 1.5 and 3 mm slice thickness reconstructions were off by one category; 77 and 20 of those shifting from AS 0 on manual scoring to AS 1–100 on automated scoring. No scan was off by two categories in either 1.5 or 3 mm scans.
Table 3
Standard reference | Automatic software | |||||||
---|---|---|---|---|---|---|---|---|
No (0) | Mild (1–100) | Moderate (101–400) | Severe (>400) | Total | Same | Shift up | Shift down | |
1.5 mm slice thickness | ||||||||
No (0) | 204 | 77 | 0 | 0 | 281 | 204 | 77 | – |
Mild (1–100) | 6 | 397 | 4 | 0 | 407 | 397 | 4 | 6 |
Moderate (101–400) | 0 | 10 | 82 | 0 | 92 | 82 | 0 | 10 |
Severe (>400) | 0 | 0 | 9 | 55 | 64 | 55 | – | 9 |
Total | 210 | 484 | 95 | 55 | 844 | |||
3 mm slice thickness | ||||||||
No (0) | 458 | 20 | 0 | 0 | 478 | 458 | 20 | – |
Mild (1–100) | 0 | 229 | 1 | 0 | 230 | 229 | 1 | 0 |
Moderate (101–400) | 0 | 6 | 76 | 0 | 82 | 76 | 0 | 6 |
Severe (>400) | 0 | 0 | 3 | 51 | 54 | 51 | – | 3 |
Total | 458 | 255 | 80 | 51 | 844 |
Columns to the right demonstrate a summary of risk category shifting. CAC, coronary artery calcium.
Per-lesion analysis
Among 844 subjects, the numbers of identified lesions using the automatic CAC scoring software were 8,168 and 3,086 on the 1.5 and 3 mm scans, respectively. Automatic software yielded per-lesion sensitivity of 91.8% (7,495 of 8,168 lesions) and 95.9% (2,958 of 3,086 lesions) and a false-positive rate of 0.8 and 0.15 per subject (673 and 128 lesions among 844 subjects) for the 1.5 and 3 mm scans, respectively.
For the 1.5 mm scans, the most common cause of false-positive results was image noise (66.4%, 447/673; Figure 3A), followed by wrong vessel segmentation (23.6%, 159/673) (Table 4). Among 29 aortic wall calcifications falsely detected by automatic software, 21 (72.4%) were classified as RCA lesions. Among 38 false-positive myocardial calcifications, 25 (65.8%) were categorized as LCX lesions. For the 3 mm scans, the main causes of false-positive results were wrong vessel segmentation (59.3%, 76/128; Figure 3B) and image noise (23.4%, 30/128). Wrong vessel segmentation includes errors originating from artery segmentation and labeling (e.g., LM labeled as LAD). After excluding wrong vessel segmentation, the number of ‘anatomically’ false-positive results decreased to 514 and 52 in 1.5 and 3 mm scans, respectively (0.6 and 0.06 false-positive lesions per patient, Table S3). There were 330 and 208 false-negative results in 1.5 mm (Figure 3C) and 3 mm scans, respectively, and the most common location of missed lesions was the LAD (1.5 mm, n=106, 32.1%; 3 mm, n=72, 34.6%).
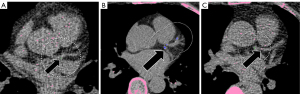
Table 4
False-positive/-negative | 1.5 mm slice thickness | 3 mm slice thickness |
---|---|---|
False-positive | n=673 | n=128 |
Wrong vessel segmentation | 159 | 76 |
LM | 76 (47.8) | 51 (67.1) |
LAD | 20 (12.6) | 13 (17.1) |
LCX | 21 (13.2) | 5 (6.6) |
RCA | 42 (26.4) | 7 (9.2) |
Image noise | 447 | 30 |
LM | 57 (12.8) | 2 (6.7) |
LAD | 92 (20.6) | 7 (23.3) |
LCX | 130 (29.1) | 3 (10.0) |
RCA | 168 (37.6) | 18 (60.0) |
Aortic wall | 29 | 11 |
LM | 8 (27.6) | 4 (36.4) |
LAD | 0 | 0 |
LCX | 0 | 0 |
RCA | 21 (72.4) | 7 (63.6) |
Myocardium | 38 | 10 |
LM | 0 | 0 |
LAD | 5 (13.2) | 0 |
LCX | 25 (65.8) | 6 (60.0) |
RCA | 8 (21.1) | 4 (40.0) |
Sternum | 1 | 1 |
RCA | 1 (100.0) | 1 (100.0) |
Rib | 5 | |
LAD | 5 (100.0) | |
Metal artifact | 1 | |
LAD | 1 (100.0) | |
False-negative | n=330 | n=208 |
LM | 59 (17.9) | 37 (17.8) |
LAD | 106 (32.1) | 72 (34.6) |
LCX | 70 (21.2) | 50 (24.0) |
RCA | 95 (28.8) | 49 (23.6) |
Data are number with percentage in the parentheses. CAC, coronary artery calcium; LAD, left anterior descending artery; LCX, left circumflex artery; LM, left main artery; RCA, right coronary artery.
Discussion
In this study, CAC quantification using DL-based automatic software was evaluated using a manual method as a reference standard for both 1.5 and 3 mm scans. Automatic CAC scoring demonstrated excellent agreement (ICC >0.9) in the AS and calcium volume measurements for both 1.5 and 3 mm scans compared with the reference standard. Although automatic CAC score-based risk group categorization was excellent for both slice thickness scans, 106 (12.6%) and 30 (3.5%) scans were misclassified in the 1.5 and 3 mm scans, respectively. Per-lesion sensitivity was high (1.5 mm, 91.8%; 3 mm, 95.9%), and false-positive rate was low (1.5 mm, 0.8; 3 mm, 0.15 false-positive lesions per subject). The most common causes of false-positive results were image noise on 1.5 mm scans and wrong vessel segmentation on 3 mm scans.
The reliability of the automatic CAC scoring method in this study (ICC for AS, 0.982 for 1.5 mm and 0.969 for 3 mm; κ value for risk category, 0.851 for 1.5 mm and 0.960 for 3 mm) was comparable to that reported by recent studies (ICC for AS 0.97–0.996, κ 0.919–0.97) (2,6,15). Although many studies using DL-based, automated CAC scoring methods on ECG-gated CAC scoring CT have been reported, to our knowledge, no study has evaluated the influence of reconstructed slice thickness on the accuracy of DL-based, automatic CAC scoring software.
Interestingly, the 1.5 mm slice thickness scan showed excellent correlation and agreement but demonstrated less accurate risk group categorization than the 3 mm scans. One reason could be because the majority of misclassification was from AS 0 using the reference standard to AS 1–100 using the automatic method. Thin-slice reconstruction increases sensitivity to detect small calcifications, but also increases image noise. Excessive image noise can mimic small, calcified lesions and lower the reliability of automatic CAC scoring.
The ability to accurately determine the presence and quantify the severity of CAC is important for the assessment of cardiovascular risk (16). The presence of CACs is associated with a higher risk of cardiovascular mortality and morbidity than subjects with AS 0, particularly in asymptomatic individuals (17,18). Moreover, the latest guidelines recommend statin therapy initiation in patients with AS >0 or AS >100 (19,20). Thus, false-negative results may lead to the delayed initiation of preventive management. Conversely, false-positive results may lead to unnecessary treatment. In this study, the specificity for detecting AS 0 subjects is high for both 3 mm [458/478 (95.8%)] and 1.5 mm scans [204/281 (72.6%)], and there was no false-negative in 1.5 or 3 mm scans. False-positive misclassification for subject with AS 0 on automatic scoring occurred in 9.1% (77/844) for 1.5 mm scans and 2.4% (20/844) for 3 mm scans. Considering the reported false-positive misclassification rate (1.9–7.0%) in previous studies of the performance of automatic CAC scoring on ECG-gated CT (2,6,15), the false-positive misclassification in our study was in the acceptable range, despite the slightly higher value for 1.5 mm scans.
Our results demonstrated that the automatic scoring adapted well to the lower slice thickness scan, although false-positive results were more frequent. Therefore, the CT protocol must be carefully considered when applying automatic CAC scoring, especially in non-ECG-gated chest CT of various slice thickness values. Generally, scan acquisition and reconstruction parameters such as slice thickness can affect the quantification of reference CAC scores and lead to differences in the agreement of the CAC severity category between ECG-gate CT and non-ECG-gated CT (9). Our study revealed that scan parameter also affects the performance of an automated CAC scoring algorithm. Some researchers have recently proposed DL-based, automatic CAC scoring for non-ECG-gated chest CT and reported good to excellent performance (2,21-23). However, the impact of slice thickness of chest CT on the performance of automated CAC scoring algorithms has not been well investigated in those studies. Our results suggest that further study using various types of training and validation datasets is needed for the generalization and application of automatic CAC scoring for routine clinical use, especially for non-ECG-gated chest CT.
Most of previous studies on the performance of DL-based, automatic CAC scoring (2,3,6,15) did not perform per-lesion analyses. According to Lee et al. (6), the main causes of false-positive results (0.11 per patient) were image noise or artifacts (29.1%), which is in line with our observations. However, wrong vessel segmentation (59.3%) was the main cause for false-positive results for 3 mm scans in this study, followed by image noise (23.4%).
There are limitations to this study. First, all CT scans were conducted at a single center with asymptomatic individuals. Second, a relatively small number (1.5 mm, n=64; 3 mm, n=54) of individuals with high AS (>400) could have skewed our results. Therefore, further multicenter investigations covering larger varieties of the disease spectrum are needed to assess the generalizability of our conclusions. Third, concerns about the accuracy and clinical impact of CAC scores on 1.5 mm scans could be raised because the current standard protocol for CAC scoring scan applies to 2.5 to 3 mm scans. However, the reference standard supported by double readings and a nearly perfect inter-observer agreement for 1.5 and 3 mm scans helped ensure the reliability of the CAC scoring in 1.5 mm scans. In addition, the prognostic value of the CAC scores on ECG-gated CT with 1.5 mm reconstructions should be further investigated in a future study. Fourth, inter-observer agreement was assessed in only small proportion (11.8%) of CT scans for 1.5 and 3 mm slice thickness scans.
In conclusion, automatic CAC scoring shows excellent agreement with the reference standard for both 1.5 and 3 mm slice thickness scans but results in lower agreement to the CAC severity category in 1.5 mm scans. Understanding the influence of slice thickness on the performance of automatic CAC scoring software is necessary when applying it for clinical practice.
Acknowledgments
Seonok Kim (Department of Medical Statistics, Asan Medical Center, University of Ulsan College of Medicine) kindly provided statistical advice for this manuscript.
Funding: This work was supported by the National Research Foundation of Korea (NRF) grant funded by the Korea government (MSIT) (No. 2021R1A2C4002195 to YJS).
Footnote
Conflicts of Interest: All authors have completed the ICMJE uniform disclosure form (available at https://qims.amegroups.com/article/view/10.21037/qims-22-835/coif). YJS reports that this work was supported by the National Research Foundation of Korea (NRF) grant funded by the Korea government (MSIT) (No. 2021R1A2C4002195); HS and HJP are employees of Coreline Soft, Co., Ltd., and the company supported the software (AVIEW CAC) for this study; DHY has contract of technology transfer with Coreline Soft, Co., Ltd. The other authors have no conflicts of interest to declare.
Ethical Statement: The authors are accountable for all aspects of the work in ensuring that questions related to the accuracy or integrity of any part of the work are appropriately investigated and resolve. The study was conducted in accordance with the Declaration of Helsinki (as revised in 2013). The study was approved by the institutional review board of Severance Hospital and individual consent for this retrospective analysis was waived.
Open Access Statement: This is an Open Access article distributed in accordance with the Creative Commons Attribution-NonCommercial-NoDerivs 4.0 International License (CC BY-NC-ND 4.0), which permits the non-commercial replication and distribution of the article with the strict proviso that no changes or edits are made and the original work is properly cited (including links to both the formal publication through the relevant DOI and the license). See: https://creativecommons.org/licenses/by-nc-nd/4.0/.
References
- Detrano R, Guerci AD, Carr JJ, Bild DE, Burke G, Folsom AR, Liu K, Shea S, Szklo M, Bluemke DA, O'Leary DH, Tracy R, Watson K, Wong ND, Kronmal RA. Coronary calcium as a predictor of coronary events in four racial or ethnic groups. N Engl J Med 2008;358:1336-45. [Crossref] [PubMed]
- van Velzen SGM, Lessmann N, Velthuis BK, Bank IEM, van den Bongard DHJG, Leiner T, de Jong PA, Veldhuis WB, Correa A, Terry JG, Carr JJ, Viergever MA, Verkooijen HM, Išgum I. Deep Learning for Automatic Calcium Scoring in CT: Validation Using Multiple Cardiac CT and Chest CT Protocols. Radiology 2020;295:66-79. [Crossref] [PubMed]
- Martin SS, van Assen M, Rapaka S, Hudson HT Jr, Fischer AM, Varga-Szemes A, Sahbaee P, Schwemmer C, Gulsun MA, Cimen S, Sharma P, Vogl TJ, Schoepf UJ. Evaluation of a Deep Learning-Based Automated CT Coronary Artery Calcium Scoring Algorithm. JACC Cardiovasc Imaging 2020;13:524-6. [Crossref] [PubMed]
- Lessmann N, van Ginneken B, Zreik M, de Jong PA, de Vos BD, Viergever MA, Isgum I. Automatic Calcium Scoring in Low-Dose Chest CT Using Deep Neural Networks With Dilated Convolutions. IEEE Trans Med Imaging 2018;37:615-25. [Crossref] [PubMed]
- Wolterink JM, Leiner T, de Vos BD, van Hamersvelt RW, Viergever MA, Išgum I. Automatic coronary artery calcium scoring in cardiac CT angiography using paired convolutional neural networks. Med Image Anal 2016;34:123-36. [Crossref] [PubMed]
- Lee JG, Kim H, Kang H, Koo HJ, Kang JW, Kim YH, Yang DH. Fully Automatic Coronary Calcium Score Software Empowered by Artificial Intelligence Technology: Validation Study Using Three CT Cohorts. Korean J Radiol 2021;22:1764-76. [Crossref] [PubMed]
- Urabe Y, Yamamoto H, Kitagawa T, Utsunomiya H, Tsushima H, Tatsugami F, Awai K, Kihara Y. Identifying Small Coronary Calcification in Non-Contrast 0.5-mm Slice Reconstruction to Diagnose Coronary Artery Disease in Patients with a Conventional Zero Coronary Artery Calcium Score. J Atheroscler Thromb 2016;23:1324-33. [Crossref] [PubMed]
- Kim SY, Suh YJ, Lee HJ, Kim YJ. Progostic value of coronary artery calcium scores from 1.5 mm slice reconstructions of electrocardiogram-gated computed tomography scans in asymptomatic individuals. Sci Rep 2022;12:7198. [Crossref] [PubMed]
- Kim JY, Suh YJ, Han K, Choi BW. Reliability of Coronary Artery Calcium Severity Assessment on Non-Electrocardiogram-Gated CT: A Meta-Analysis. Korean J Radiol 2021;22:1034-43. [Crossref] [PubMed]
- Hecht HS, Cronin P, Blaha MJ, Budoff MJ, Kazerooni EA, Narula J, Yankelevitz D, Abbara S. 2016 SCCT/STR guidelines for coronary artery calcium scoring of noncontrast noncardiac chest CT scans: A report of the Society of Cardiovascular Computed Tomography and Society of Thoracic Radiology. J Cardiovasc Comput Tomogr 2017;11:74-84. [Crossref] [PubMed]
- Taylor AJ, Cerqueira M, Hodgson JM, Mark D, Min J, O'Gara P, et al. ACCF/SCCT/ACR/AHA/ASE/ASNC/NASCI/SCAI/SCMR 2010 appropriate use criteria for cardiac computed tomography. A report of the American College of Cardiology Foundation Appropriate Use Criteria Task Force, the Society of Cardiovascular Computed Tomography, the American College of Radiology, the American Heart Association, the American Society of Echocardiography, the American Society of Nuclear Cardiology, the North American Society for Cardiovascular Imaging, the Society for Cardiovascular Angiography and Interventions, and the Society for Cardiovascular Magnetic Resonance. J Am Coll Cardiol 2010;56:1864-94. [Crossref] [PubMed]
- Perk J, De Backer G, Gohlke H, Graham I, Reiner Z, Verschuren M, et al. European Guidelines on cardiovascular disease prevention in clinical practice (version 2012). The Fifth Joint Task Force of the European Society of Cardiology and Other Societies on Cardiovascular Disease Prevention in Clinical Practice (constituted by representatives of nine societies and by invited experts). Eur Heart J 2012;33:1635-701. [Crossref] [PubMed]
- Hokanson JE, MacKenzie T, Kinney G, Snell-Bergeon JK, Dabelea D, Ehrlich J, Eckel RH, Rewers M. Evaluating changes in coronary artery calcium: an analytic method that accounts for interscan variability. AJR Am J Roentgenol 2004;182:1327-32. [Crossref] [PubMed]
- Bland JM. The half-normal distribution method for measurement error: two case studies. Available online: https://www-users.york.ac.uk/~mb55/talks/halfnor.pdf
- Sandstedt M, Henriksson L, Janzon M, Nyberg G, Engvall J, De Geer J, Alfredsson J, Persson A. Evaluation of an AI-based, automatic coronary artery calcium scoring software. Eur Radiol 2020;30:1671-8. [Crossref] [PubMed]
- Carr JJ, Jacobs DR Jr, Terry JG, Shay CM, Sidney S, Liu K, Schreiner PJ, Lewis CE, Shikany JM, Reis JP, Goff DC Jr. Association of Coronary Artery Calcium in Adults Aged 32 to 46 Years With Incident Coronary Heart Disease and Death. JAMA Cardiol 2017;2:391-9. [Crossref] [PubMed]
- Lee JH, Han D, Ó Hartaigh B, Rizvi A, Gransar H, Park HB, Park HE, Choi SY, Chun EJ, Sung J, Park SH, Han HW, Min JK, Chang HJ. Warranty Period of Zero Coronary Artery Calcium Score for Predicting All-Cause Mortality According to Cardiac Risk Burden in Asymptomatic Korean Adults. Circ J 2016;80:2356-61. [Crossref] [PubMed]
- Blaha MJ, Cainzos-Achirica M, Dardari Z, Blankstein R, Shaw LJ, Rozanski A, Rumberger JA, Dzaye O, Michos ED, Berman DS, Budoff MJ, Miedema MD, Blumenthal RS, Nasir K. All-cause and cause-specific mortality in individuals with zero and minimal coronary artery calcium: A long-term, competing risk analysis in the Coronary Artery Calcium Consortium. Atherosclerosis 2020;294:72-9. [Crossref] [PubMed]
- Greenland P, Blaha MJ, Budoff MJ, Erbel R, Watson KE. Coronary Calcium Score and Cardiovascular Risk. J Am Coll Cardiol 2018;72:434-47. [Crossref] [PubMed]
- Grundy SM, Stone NJ, Bailey AL, Beam C, Birtcher KK, Blumenthal RS, et al. 2018 AHA/ACC/AACVPR/AAPA/ABC/ACPM/ADA/AGS/APhA/ASPC/NLA/PCNA Guideline on the Management of Blood Cholesterol: A Report of the American College of Cardiology/American Heart Association Task Force on Clinical Practice Guidelines. Circulation 2019;139:e1082-143. [PubMed]
- Xu C, Guo H, Xu M, Duan M, Wang M, Liu P, Luo X, Jin Z, Liu H, Wang Y. Automatic coronary artery calcium scoring on routine chest computed tomography (CT): comparison of a deep learning algorithm and a dedicated calcium scoring CT. Quant Imaging Med Surg 2022;12:2684-95. [Crossref] [PubMed]
- Xu J, Liu J, Guo N, Chen L, Song W, Guo D, Zhang Y, Fang Z. Performance of artificial intelligence-based coronary artery calcium scoring in non-gated chest CT. Eur J Radiol 2021;145:110034. [Crossref] [PubMed]
- van Assen M, Martin SS, Varga-Szemes A, Rapaka S, Cimen S, Sharma P, Sahbaee P, De Cecco CN, Vliegenthart R, Leonard TJ, Burt JR, Schoepf UJ. Automatic coronary calcium scoring in chest CT using a deep neural network in direct comparison with non-contrast cardiac CT: A validation study. Eur J Radiol 2021;134:109428. [Crossref] [PubMed]