Prognostic potential of a voxelwise invasion risk map of nasopharyngeal carcinoma based on a coordinate system of the nasopharynx
Introduction
Locoregional tumor invasion is an important prognostic factor in nasopharyngeal carcinoma (NPC) (1). In general, the tumor invasion risk (TIR) classification is based on the incidence rates of tumor invasion into anatomical sites surrounding the nasopharynx (2-4). Invasion of an anatomical site by NPC is diagnosed by magnetic resonance imaging (MRI). However, only information on the anatomical site invaded is available by MRI, and no information on the degree of invasion of the anatomical site is provided. Prognostic accuracy relies on an accurate description of the incidence of tumor invasion. Therefore, designing a coordinate system of the nasopharynx to obtain a meticulous TIR will support the accurate prognosis of NPC.
Coordinate systems have been successfully applied in many studies (5,6), and anatomical landmarks have often been used to define coordinate systems. The results of most neuroimaging studies have been reported with Montreal Neurological Institute (MNI) coordinates (7-10). In addition, a nonrigid image registration method, namely, large deformation diffeomorphic metric mapping, has been used to transform the MRI data of participants into the standard coordinate system (11,12). Brandt et al. developed a breast coordinate system based on the location of the pectoral muscle and nipple and the shape of the breast boundary (13). Englander et al. used a 3-dimensional (3D) coordinate system based on the anatomic features of bones to measure the knee abduction angles (14). Based on mesial temporal lobe anatomical landmarks, a stereotactic coordinate system was defined to facilitate the planning and delineate the extent of ablation or region of stimulation within the mesial temporal lobe structures (15). A 3D coordinate system was constructed with 4 bony landmark points to correct for changes in pelvic inclination (16). A reference coordinate system was defined for the human patella on the basis of 7 marked anatomical landmarks (17). A parametric coordinate system was defined on the basis of skull boundaries, location of the eye sockets, and head pose to predict intracranial structures (18). A common coordinate system based on cranial position was designed for predicting gestational age and neurodevelopmental maturation of a fetus (19). A normalized, abdominal coordinate system, the origin of which was located at the at the geometrical center of the first lumbar vertebra body, was defined in computed tomography (CT) and MR volume images to provide initial positions for abdominal organ segmentation (20).
Some researchers have attempted to explore the coordinate system of the nasopharynx. Satoh et al. used the cephalo-metric landmarks derived from a coordinate system that was pointed on bones surrounding the nasopharynx to clarify the growth characteristics of the nasopharynx (21). In verifying the hypothesis that differences in maxillary sinus size and shape may impede mucociliary clearance, Kim et al. collected 29 3D coordinate landmarks and 7 linear measurements of maxillary sinus morphology (22). A polar coordinate system was used to conduct trans-nasal video-endoscopic recordings of the Eustachian tube (23). The landmarks and definition of the plane of the choanae might reflect the inherent anatomical characteristics of the nasopharynx (24). However, within the body of research on the coordinate system of the nasopharynx, no coordinate system has been designed for TIR. This may be due to the following inherent challenges in constructing the nasopharynx coordinate system to study the risk of NPC invasion:
- The nasopharynx, which includes soft tissue and bony structures, is the beginning of the respiratory tract, and its internal structure is complex and differs significantly between individuals. This structural complexity challenges the definition of the NPC coordinate system.
- The MRI images used in TIR studies are all of patients with NPC, and NPC morphology is characterized by deformation of the nasopharyngeal cavity (flat or asymmetric), thickening of the nasopharyngeal lateral wall, and changes in the peripharyngeal soft tissue and space (25). Individual differences between the medical images of patients with NPC are significant, making it difficult to conduct image registration methods based on organ and image features.
- The images in our collection vary significantly with regard to acquisition configurations, including superior-inferior coverage, sizes, and spatial resolutions. Such variation strongly challenges the accuracy of image registration methods.
In view of the above technical challenges, we aimed to define a 3D coordinate system of the nasopharynx based on stable anatomical landmarks for the imaging study of NPC. Based on the coordinate system, the meticulous voxelwise TIR map of NPC was automatically obtained after registering the tumor region of interest (ROI) of all patients with NPC into the coordinate system. The voxelwise TIR map provides a new method for the prognostic analysis of NPC and can also be applied to delineate the clinical target volume (CTV) for NPC. In this paper, we focus on the application of the TIR map in prognostic analysis in order to obtain stable prognostic factors for NPC.
Methods
Study design
The study was conducted in accordance with the Declaration of Helsinki (as revised in 2013) and approved by the Ethics Committee of Sun Yat-sen University Cancer Center. Individual consent for this retrospective analysis was waived.
The study design is shown in Figure 1. The coordinate system of the nasopharynx was defined by stable anatomical landmarks and validated by the MRI volumes of 18 normal participants. The tumors in the MRI volumes of the patients with NPC were registered to the defined coordinate system using the transformation matrix, which was calculated based on the anatomical landmarks. The voxelwise TIR map was obtained by superposition operation, and the prognostic analysis of NPC was based on 778 patients.
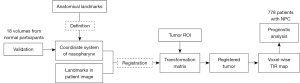
Definition of the coordinate system with anatomical landmarks
As shown in Figure 2, a total of 6 landmarks were used in constructing the coordinate system. Four landmarks occupy the transverse section: the right/left internal acoustic pore (RIA/LIA) and the right/left ascending segment of the internal carotid artery in the posterior cavernous sinus (RAS/LAS). Around the nasopharynx, the positional relationship between the landmarks of the bilateral internal acoustic pore and the bilateral internal carotid artery is stable. In addition, these landmarks are often used for the construction of coordinate systems (26,27). Furthermore, the 4 points are symmetrical and almost in the same plane. In our experimental data, the 4 landmarks were on the same slice in approximately 75% of the images. The 4 landmarks were not in the same slice in all of the images because the landmarks can be affected by a patient’s posture during image acquisition. In obtaining the parameters for 3D image registration, 2 landmarks in the sagittal section were used: the posterior clinoid (PC) process and the midpoint of the upper edge of the anterior arch of C1 (MC). The PC and MC are located in the central position of the posterior cranial fossa, closer to the nasopharynx.
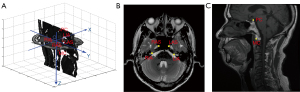
The origin of the coordinate system is located at the midpoint of the connecting line between the landmarks of the LIA and RIA. The x-axis points from RIA to LIA. The y-axis is defined as the direction perpendicular to the x-axis in the transverse section and from back to front. The z-axis is defined as a top-down direction perpendicular to the transverse section. The unit of each coordinate axis is the millimeter, which was chosen to eliminate the influence of different resolutions in three dimensions.
Volume registration for the NPC ROI
Figure 3 shows a schematic diagram of the patient tumor registration into the coordinate system. The transformation matrix from the patient tumor volume registration to the coordinate system can be obtained by the positional relationship between the anatomical landmarks in the coordinate system and that in the patient images. The spatial transformation of tumor volume primarily includes shift, scale, and rotation.
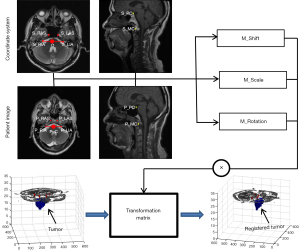
The shift transformation matrix, M_Shift, can be described by the positional relationship between the midpoints of the line between the RIA and LIA in the 2 images, as shown in the following equation:
where (Ox, Oy, Oz) is the origin coordinate value of the coordinate system and (P_Cx, P_Cy, P_Cz) is the coordinate value of the midpoint between the RIA and the LIA.
The scale transformation matrix, M_Scale, can be described as follows:
where Sx=Cx/Px is used to describe the scale transformation of the x-axis, and Cx and Px are the lengths of connections of the LIA and the RIA in the coordinate system and the patient image, respectively, as shown below:
where Sy=Cy/Py describes the scale transformation of the y-axis, and Cy and Py are the line lengths of the connection between the midpoint of the LIA/RIA and the LAS/RAS in the standard coordinate system and patient image, respectively, as shown below:
where
and where Sz=Cz/Pz is used to describe the scale transformation of the y-axis, and Cz and Pz are the differences between the z-axis coordinate values of PC and MC in the coordinate system and patient image, respectively, as defined below:
The rotation transformation usually should include rotations in the transverse, sagittal, and coronal sections. However, only the rotations in the transverse and sagittal section are considered in the process of image registration. During MRI data acquisition, the patients are lying on their back and their heads are fixed. The rotation angle change in the Z direction in the coordinate system is small. Furthermore, it is difficult to find stable anatomical landmarks to use for image registration since the coronal images of the head vary greatly with the patient’s posture and there is low resolution between slices. Therefore, the rotation in the transverse section can be defined as follows:
where θ1 is the intersection angle between the line of RIA/LIA in the coordinate system and that in the patient image.
Similarly, the rotation in the sagittal section can be defined as follows:
where θ2 is the intersection angle between the line of PC-MC in the coordinate system and that in the patient image.
In addition, the rotation transformation matrix M_Rotation can be described as follows:
Therefore, the transformation matrix can be calculated as follows:
Once the transformation matrix of a patient with NPC is obtained, the registered tumor in the coordinate system can be obtained.
TIR map computation
The tumor ROIs of 778 patients with NPC were registered to the coordinate system. In expanding the data volume, the registered ROIs were mirrored to double the data set, given that the human head is symmetrical. The voxelwise TIR map for NPC obtained by superposition of all registered and mirrored tumor ROIs. Therefore, the voxelwise tumor invasion frequency (F) can be defined as follows:
where Roi_Pi is the registered tumor ROI for a patient with NPC, and Roi_Pmi is the mirrored tumor ROI. The voxel-wise TIR (IR) can be defined as normalized F, that is:
Prognostic analysis
We applied the TIR map to the retrospective prognostic analysis of NPC in order to verify the prognostic potential of the TIR map for NPC. As shown in Figure 4, the minimum risk (MinR) point of the NPC ROI is defined as the point with the risk of minimum invasion in the coordinate system of the outer contour of the ROI. Therefore, the MinR can describe the extent of tumor-outward spread in patients with NPC. The smaller the MinR value is, the wider the outward spread of the tumor. We used MinR to perform a prognostic analysis of NPC.
The patients with NPC were grouped by a cutoff value for prognostic analysis. A density plot, which is independent of a patient’s prognostic information, was used to compute and draw kernel density estimates. The density plot was performed using the geom_density function provided by ggplot2 package in R v. 3.3.5 (The R Project for Statistical Computing). Restricted cubic spline (RCS) function was performed using the “rms” package of R to evaluate the effectiveness of the cutoff value, and the “rcs” formula parameter was used to build the Cox regression model for overall survival (OS). The difference in 5-year OS rate was calculated by the log-rank test, and the hazard ratio (HR) and adjusted P value were calculated by multivariable Cox regression.
Results
Validation of the coordinate system
We performed the registration experiment of the lateral pterygoid muscle (LPM) and the longus capitis muscle (LCM) in 18 normal participants to illustrate the effectiveness of the proposed coordinate system. The LPM is in the infratemporal fossa. The upper part starts from the infratemporal and infratemporal ridges of the great wing of the sphenoid bone. The lower part starts from the outer side of the outer wing plate, and the fibers run backward and outward, ending in the pterygoid muscle fossa of the condylar neck. The LPM is symmetrically distributed on the left and right sides of a regularly shaped nasopharynx. The LCM is an anterior vertebral muscle and originates as 4 slender tendons from the anterior tubercles of the C3-C6 transverse processes and overlaps with the high incidence area of NPC. In summary, the registration experimental results of the LPM and LCM are representative.
A total of 18 normal participants were involved in the experiment. The head MRIs were acquired from the Cancer Center of Sun Yat-sen University. The bilateral LPMs and 6 anatomical landmarks were delineated by a doctor. Randomly selected images of patients were used as reference images and others as floating images. The overlapping rate, R, which was used to evaluate the performance of the registration, was defined as follows:
where Ng is the number of voxels inside the LPM region of the reference image, and is the number of voxels in the LPM region in the reference image and registered result. The performance evaluation of the registration is shown in Figure 5.
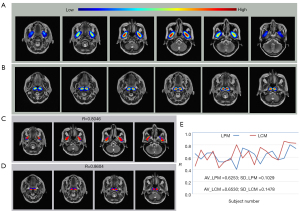
The areas of the bilateral LPM and LCM can be overlapped in the coordinate system. The average R values reached 0.6253 and 0.6530, respectively. These results show that the coordinate system was stable in the MRI image analysis of the head, and thus could be applied to the registration of tumor in the nasopharynx.
Voxelwise TIR map for NPC
The voxelwise TIR was obtained through a superposition operation after the tumor ROIs of all NPC participants were registered to the coordinate system. All registration results were confirmed by the radiologist to be reasonable. We performed a mirror operation on the tumor ROI of each patient with NPC since the human nasopharynx is symmetrical from left to right. The voxelwise TIR map based on 778 patients with NPC is shown in Figure 6. The TIR map also demonstrates symmetry. It was found that the risk of NPC invasion was largest in the posterior wall of the nasopharynx, gradually decreasing outward.
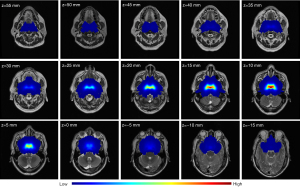
We obtained the tumor invasion of each voxel in the coordinate system from the TIR map. The TIR map shows the statistical significance for tumors in space since each voxel is located on the anatomical structure of the nasopharynx. Therefore, the TIR map was used for the prognostic analysis of NPC.
Prognostic analysis of NPC based on the TIR map
Database for prognostic analysis
The MRI data in the experimental test were obtained from the Cancer Center of Sun Yat-sen University and were used to assign prediction scores to patients with NPC (28). A total of 778 MRI head volumes from patients with NPC were used for the volume registration experiment and the invasion risk analysis. Sociodemographic and clinical characteristics of the participants are provided in Table 1.
Table 1
Characteristic | Total data (n=778) | MinR <0.0162 (n=209) | MinR ≥0.0162 (n=569) | Chi-squared P value |
---|---|---|---|---|
Age (years) | 45 [38–53] | 47 [38–56] | 44 [37–51] | 0.026 |
Sex | 0.020 | |||
Male | 567 (72.9%) | 165 (78.9%) | 402 (70.7%) | |
Female | 211 (27.1%) | 44 (21.1%) | 167 (29.3%) | |
Histologic type | 0.488 | |||
WHO type I/II | 46 (5.9%) | 10 (4.8%) | 36 (6.3%) | |
WHO type III | 732 (94.1%) | 199 (95.2%) | 533 (93.7%) | |
EBV DNA (1,000 copy/mL) | 0.000 | |||
<1 | 351 (45.1%) | 60 (28.7%) | 291 (51.1%) | |
1–10 | 183 (23.5%) | 51 (24.4%) | 132 (23.2%) | |
>10 | 244 (31.4%) | 98 (46.9%) | 146 (25.7%) | |
T classification | 0.000 | |||
T1 | 201 (25.8%) | 4 (1.9%) | 197 (34.6%) | |
T2 | 94 (12.1%) | 8 (3.8%) | 86 (15.1%) | |
T3 | 290 (37.3%) | 60 (28.7%) | 230 (40.4%) | |
T4 | 193 (24.8%) | 137 (65.6%) | 56 (9.8%) | |
N classification | 0.001 | |||
N0 | 176 (22.6%) | 27 (12.9%) | 149 (26.2%) | |
N1 | 433 (55.7%) | 129 (61.7%) | 304 (53.4%) | |
N2 | 111 (14.3%) | 34 (16.3%) | 77 (13.5%) | |
N3 | 58 (7.5%) | 19 (9.1%) | 39 (6.9%) | |
Staging | 0.000 | |||
I | 71 (9.1%) | 1 (0.5%) | 70 (12.3%) | |
II | 172 (22.1%) | 8 (3.8%) | 164 (28.8%) | |
III | 297 (38.2%) | 54 (25.8%) | 243 (42.7%) | |
iVa | 238 (30.6%) | 146 (69.9%) | 92 (16.2%) | |
Treatment | 0.000 | |||
RT | 102 (13.1%) | 5 (2.4%) | 97 (17.0%) | |
CCRT | 293 (37.7%) | 68 (32.5%) | 225 (39.5%) | |
IC + CCRT | 383 (49.2%) | 136 (65.1%) | 247 (43.4%) | |
MinR | 0.0498 (0.0137–0.1234) | 0.0043 (0.0009–0.0086) | 0.0816 (0.0421–0.1485) | 0.000 |
ggfind_best | 0.000 | |||
MinR <0.0162 | 209 (26.9%) | 209 (100%) | 0 (0%) | |
MinR ≥0.0162 | 569 (73.1%) | 0 (0%) | 569 (100%) | |
OS | 0.000 | |||
Events | 85 (10.9%) | 46 (22.0%) | 39 (6.9%) | |
5-year surv. (%) | 88.4 | 76.8 | 92.6 | |
Follow-up (months) | 62.2 (3.4–83.4) | 60.4 (4.6–83.4) | 62.8 (3.4–82.8) | 0.001 |
Data are presented as median [IQR (min-max)] and n (%). WHO, World Health Organization; EBV, Epstein-Barr virus; RT, radiotherapy; CCRT, concurrent chemoradiotherapy; IC, induction chemotherapy; MinR, minimum risk; OS, overall survival; surv., survival rate.
The MRI images in the transverse and sagittal sections were obtained using a 3D fast-recovery fast spin-echo sequence. MRI was performed on patients using a 3.0-T system (Magnetom Tim Trio; Siemens Healthineeers, Erlangen, Germany) or a 1.5-T system (Signa CV/I; General Electric Healthcare, Chicago, IL, USA). The image parameters of the transverse section acquisition changed between the different volumes. The matrix varied from 320×320 to 768×768 pixels, and the slice thickness varied from 4 to 6.5 mm. In each volume, 36 slices were presented to cover the whole head and neck.
A healthy volunteer was recruited to participate in the establishment of a standard coordinate system. The parameters of the transverse section image were as follows: matrix =512×512 pixels and slice thickness =5 mm (36 slices). Meanwhile, the parameters of the sagittal section image were as follows: matrix =512×512 pixels and slice thickness =5 mm.
In each volume, the NPC ROI, the 4 landmarks (RIA, LIA, RAS, and LAS) in the transverse section, and the 2 landmarks (PC and MC) in the sagittal section were labeled by a doctor with more than 5 years of work experience and confirmed by a professor of medical imaging.
Prognostic analysis
The patients with NPC were grouped by a cutoff value for prognostic analysis. The procession of the cutoff value selection is shown in Figure 7. The density plot and RCS analysis were carried out on the MinR value of each of 778 patients with NPC. The results are shown in Figure 5A,5B. At the MinR value of 0.0162, the curve reached its peak, which was used as the best cuttoff value. In addition, the risk of mortality decreased continuously with an increase in MinR up to 0.0162 (the lower curve of the RCS). The curve showed a downward trend when the MinR was between 0 and 0.0162, and the risk almost did not change at all when the MinR was over 0.0162. Therefore, an MinR of 0.0162 was considered as the best cutoff value for the prognostic analysis.
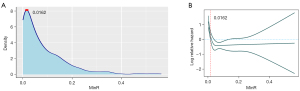
A total of 778 patients with NPC were divided into 2 groups: group 1 (MinR <0.0162) and group 2 (MinR ≥0.0162). The sociodemographic and clinical characteristics of the participants in the 2 groups are summarized in Table 1. The regions where the MinR points of the 2 groups were located are shown in Figure 8; group 1 represents the group with a low risk of NPC invasion, and group 2 represents the group with a high risk of NPC invasion.
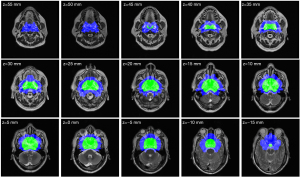
Kaplan-Meier survival analysis
The Kaplan-Meier survival method and log-rank test was conducted for the 2 groups. A summary of the univariable analysis of the confounding variables of OS and the multivariable analysis of the model cohort on OS is provided in the Tables S1,S2. To illustrate the advantages of MinR in the prognostic analysis of NPC, we compared the prognostic performance of MinR with the current tumor/node (T/N) classification and the American Joint Committee on Cancer (AJCC) staging system. The Kaplan-Meier survival curves are shown in Figure 9. As shown in Figure 9A, the OS for the 2 groups was 76.8% (low-TIR group) vs. 92.6% (high-TIR group) (P<0.001; HR =1/0.45; 95% CI: 0.27–0.77; adjusted P=0.004). Patients with a low TIR had a poor prognosis, whereas patients with a high TIR had a good prognosis.
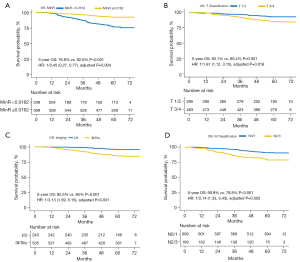
It was found that the difference in OS between the 2 groups was larger when the MinR was used than when the AJCC staging or T/N classification was used (76.8% vs. 92.6%; P<0.001). Multivariable analysis showed that the MinR was an independent variable. Furthermore, Harrell’s concordance index (C-index) was applied, and the results are summarized in Table S3. The results indicated that the MinR had a better ability to grade the prognosis of patients compared to the AJCC staging or T/N classification systems. This result indicates that the MinR is an independent prognostic factor of OS in patients with NPC.
Discussion
Based on previous reports, this study is the first to construct a coordinate system for the TIR of NPC. We constructed a stable nasopharynx coordinate system based on anatomical landmarks to obtain an accurate TIR assessment of NPC. The coordinate system was validated by the registration experiment of the lateral pterygoid and LCMs (Figure 5). In addition, we successfully obtained the voxelwise TIR map based on 778 patients with NPC (Figure 6). The posterior wall of the nasopharynx was the anatomical site with the highest risk of invasion. The distribution of TIR was related to that reported in the literature (29,30). In the literature, the distribution of TIR was calculated on the basis of whether the anatomical site was invaded or not, and the TIR was at the anatomical site level. The voxelwise TIR map obtained by the new method is potentially more accurate and more clinically applicable.
The prognostic analysis of NPC relies on the clinical application of TIR. Tumor invasion is an important prognostic factor of NPC (31,32). Bakst et al. hypothesized that tumor invasion and spread were of great significance to the prognosis of NPC (33). Invasion of the skull base (3,34,35) and parapharyngeal space (36,37) have often been used to analyze the prognosis of NPC. Huang et al. identified parapharyngeal space involvement based on the number of subspaces involved (prestyloid space, carotid space, and areas outside the carotid space). Moreover, the risk of mortality increased with the increase in the number of parapharyngeal subspaces (38). This finding indicated that a detailed analysis of anatomical sites would improve the prognosis of NPC. In our experiment, patients with NPC were divided into 2 groups based on the MinR cutoff value calculated by density plot and RCS. The OS was 76.8% in the low-TIR group and 92.6% in the high-TIR group (P<0.001; HR =1/0.45; 95% CI: 0.27–0.7; adjusted P=0.004). Patients with a low TIR have a poor prognosis, whereas those with a high TIR have a good prognosis. This result indicates that patients with poor prognosis of NPC can be accurately selected by the TIR map. Therefore, the voxelwise TIR map that we proposed in this study potentially provides a novel method for the prognostic analysis of NPC. Furthermore, the nasopharynx coordinate system that we defined may be a productive contribution to the study of NPC prognosis.
In addition, the TIR can be used to delineate the CTV of NPC. The delineation of CTV is crucial for tumor control and normal tissue protection during intensity-modulated radiation therapy. Based on the cumulative incidence of tumor invasion, anatomical sites around the nasopharynx can be divided into 3 risk levels to evaluate the locoregional extension and failure patterns of NPC and then improve CTV delineation (39,40). The CTV can be reduced on the basis of the contralateral expansion of NPC in patients with unilateral tumors (41). Consensus guidelines on CTV definition include geometric expansion beyond the visible gross tumor volume (42). In one study, the radius (1–2 cm) of expansion of the gross tumor volume was selected by the clinician when considering tumor histology, degree of diffuseness on MRI, size of tumor, age, and health of patients (43). CTV follows the European guideline with a 2-cm safety margin around the contrast-enhanced lesion and resection cavity on MRI gross tumor volume (44,45). In Miao’s study, the CTV was the gross tumor plus a 5-mm margin (46). Fixed geometric expansion is the mainstream method of CTV determination. Whether the TIR map obtained in this study has guiding significance for the geometric expansion of CTV determination deserves further study. In the future, CTV delineation and clinical verification based on the TIR map will be an important and clinical application.
Another potential clinical application of TIR may be dose-painting for intensity-modulated radiation therapy. Boosting the radiotherapy dose can provide better local control. However, dose escalation for NPC may increase treatment-related comorbidities due to the high-dose irradiation of normal tissues (47). Some studies have shown dose-painting to be a significant independent prognostic factor for NPC (48). The reliable segmentations of NPC areas and subregions provide evidence to guide the dose-painting for radiation therapy (49). The diffusion-weighted MRI-guided dose-painting intensity-modulated radiation therapy was proven to be associated with improved local tumor control and survival in NPC (50). The voxelwise TIR assessment describes the risk of NPC invasion to each voxel in the coordinate system. It provides fine tumor invasion information for dose-painting of intensity-modulated radiotherapy, which may make dose-painting more accurate and improve the effect of intensity-modulated radiotherapy for patients with NPC.
In general, the major contributions of our work consist of the following three aspects: (I) a stable nasopharynx coordinate system defined on the basis of anatomical landmarks; (II) a 3D medical image registration algorithm designed for the nasopharynx (although considerable difficulty arises in the characteristics of NPC), with the registration algorithm being validated in a registration experiment of LPM; and (III) a voxelwise TIR map of NPC. The TIR map demonstrated excellent performance in the prognostic analysis of NPC.
However, one of the limitations of this study is that the TIR map was restricted to a small sample size because the tumor ROI and 6 anatomical landmarks were delineated by doctors. However, the TIR map of NPC can be obtained automatically. We assessed the automatic localization method of anatomical landmarks in MRI head images and achieved good results (51). There are many reports on the automatic segmentation of tumor ROIs for NPC in medical images (52-55). Thus, using these above-mentioned techniques can automatically delineate the anatomical landmarks and tumor ROI, expand the sample size, and improve the accuracy of TIR.
Another limitation of this study was the registration accuracy, which needs to be further improved. Based on the registration results of the LPM, the accuracy of the ROI registration to the coordinate system needs to be increased. Only shift, scale, and rotation transformation were conducted in the study. Recently, we noticed that many deep learning methods have been applied to medical image registration (56,57). Zhu et al. proposed a Laplacian Eigenmaps-based deep learning network for 2D medical image registration (58). Sui et al. predicted 2 halfway deformations, which can move the original template and subject to a pseudomean space, simultaneously (59). Zhao et al. developed a superfast spherical surface registration framework for the cerebral cortex (60). Kim et al. presented a cycle-consistent deformable image registration to overcome the limitations in the preservation of original topology during deformation with registration vector fields (61). Zhou et al. integrated anatomy-preserving domain adaptation to a segmentation network into an anatomy-guided multimodal registration based on the robust point-matching machine (62). Hu et al. translated convolutional multimodal registration into a decision-making problem, where registration was achieved via an artificial agent trained by asynchronous reinforcement learning (63). Existing medical image registration methods are aimed at the registration between multimodal images or between multiple time series images based on object feature description. However, tumor morphology is characterized by deformation of the nasopharyngeal cavity (flat or asymmetric), thickening of the nasopharyngeal lateral wall, and changes of the peripharyngeal soft tissue and space. Thus, use of conventional image registration methods may be impracticable because of the large variety in tumor morphology, location, and performance characteristics. An efficient image registration of NPC to the new coordinate system will be considered in our future work.
Conclusions
We proposed a novel method for the prognostic analysis of NPC that is based on voxelwise TIR in the nasopharyngeal coordinate system. A stable nasopharynx coordinate system, validated by the registration experiments of LPM and LCM, was constructed on the basis of anatomical landmarks. The tumors were registered to the coordinate system through shift, scale, and rotation transformations. The first voxelwise TIR map of NPC was obtained based on 778 patients. The MinR point of the tumor region may be an independent prognostic factor for NPC. The cutoff value was calculated by density plot and validated by RCS, and then the patients were divided into 2 groups. The OS rate was 76.8% in the low-TIR group and 92.6% in the high-TIR group (P<0.001; HR =1/0.45; 95% CI: 0.27–0.77; adjusted P=0.004). Patients with a low TIR had poor prognosis, whereas patients with a high TIR had a good prognosis. The MinR appears to be better at grading the prognosis of patients compared to the AJCC staging or T/N classification systems. The voxelwise TIR map can provide comprehensive and reliable information to support the prognostic analysis of NPC.
Acknowledgments
Funding: The study was partially supported by project grants from the National Natural Science Foundation of China (Nos. 82260358 and 82171906).
Footnote
Conflicts of Interest: All authors have completed the ICMJE uniform disclosure form (available at https://qims.amegroups.com/article/view/10.21037/qims-22-744/coif). The authors have no conflicts of interest to declare.
Ethical Statement: The authors are accountable for all aspects of the work in ensuring that questions related to the accuracy or integrity of any part of the work are appropriately investigated and resolved. The study was conducted in accordance with the Declaration of Helsinki (as revised in 2013) and approved by the Ethics Committee of Sun Yat-sen University Cancer Center; individual consent for this retrospective analysis was waived.
Open Access Statement: This is an Open Access article distributed in accordance with the Creative Commons Attribution-NonCommercial-NoDerivs 4.0 International License (CC BY-NC-ND 4.0), which permits the non-commercial replication and distribution of the article with the strict proviso that no changes or edits are made and the original work is properly cited (including links to both the formal publication through the relevant DOI and the license). See: https://creativecommons.org/licenses/by-nc-nd/4.0/.
References
- Yan F, Ye Z, Wang F, Wang L, Li W, Fu Z. Clinical and imaging characteristics of 53 ulcers of post-radiation nasopharyngeal necrosis in patients with nasopharyngeal carcinoma. Mol Clin Oncol 2016;5:351-6. [Crossref] [PubMed]
- Tian L, Li YZ, Mo YX, Liu LZ, Xie CM, Liang XX, Gong X, Fan W. Nasopharyngeal carcinoma with paranasal sinus invasion: the prognostic significance and the evidence-based study basis of its T-staging category according to the AJCC staging system. BMC Cancer 2014;14:832. [Crossref] [PubMed]
- Li YZ, Cai PQ, Xie CM, Huang ZL, Zhang GY, Wu YP, Liu LZ, Lu CY, Zhong R, Wu PH. Nasopharyngeal cancer: impact of skull base invasion on patients prognosis and its potential implications on TNM staging. Eur J Radiol 2013;82:e107-11. [Crossref] [PubMed]
- Li Y, Ou X, Shen C, Xu T, Li W, Hu C. Patterns of local failures and suggestions for reduction of clinical target volume for nasopharyngeal carcinoma patients without cervical lymph node metastasis. Onco Targets Ther 2018;11:2545-55. [Crossref] [PubMed]
- Liu W, Chen G, Xie J, Liang T, Zhang C, Liao X, Liao W, Song L, Zhang X. A New Coordinate System for Magnetic Resonance Imaging of the Vestibular System. Front Neurol 2022;12:789887. [Crossref] [PubMed]
- Liu J, Singh G. A’'Aref S, Lee B, Oleru O, Min JK, Dunham S, Sabuncu MR, Mosadegh B. Image Registration in Medical Robotics and Intelligent Systems: Fundamentals and Applications. Adv Intell Syst 2019;1:1900048. [Crossref]
- Wu J, Ngo GH, Greve D, Li J, He T, Fischl B, Eickhoff SB, Yeo BTT. Accurate nonlinear mapping between MNI volumetric and FreeSurfer surface coordinate systems. Hum Brain Mapp 2018;39:3793-808. [Crossref] [PubMed]
- Marino M, Liu Q, Brem S, Wenderoth N, Mantini D. Automated detection and labeling of high-density EEG electrodes from structural MR images. J Neural Eng 2016;13:056003. [Crossref] [PubMed]
- Barnhofer T, Reess TJ, Fissler M, Winnebeck E, Grimm S, Gärtner M, Fan Y, Huntenburg JM, Schroeter TA, Gummersbach M, Bajbouj M, Hölzel BK. Effects of Mindfulness Training on Emotion Regulation in Patients With Depression: Reduced Dorsolateral Prefrontal Cortex Activation Indexes Early Beneficial Changes. Psychosom Med 2021;83:579-91. [Crossref] [PubMed]
- Guo W, Koo BB, Kim JH, Bhadelia RA, Seo DW, Hong SB, Joo EY, Lee S, Lee JI, Cho KR, Shon YM. Defining the optimal target for anterior thalamic deep brain stimulation in patients with drug-refractory epilepsy. J Neurosurg 2020;134:1054-63. [Crossref] [PubMed]
- Ma W, Li M, Gao F, Zhang X, Shi L, Yu L, Zhao B, Chen W, Wang G, Wang X. DTI Analysis of Presbycusis Using Voxel-Based Analysis. AJNR Am J Neuroradiol 2016;37:2110-4. [Crossref] [PubMed]
- Zhou Y, Zhang H, Zhang L, Cao X, Yang R, Feng Q, Yap PT, Shen D. Functional MRI registration with tissue-specific patch-based functional correlation tensors. Hum Brain Mapp 2018;39:2303-16. [Crossref] [PubMed]
- Brandt SS, Karemore G, Karssemeijer N, Nielsen M. An anatomically oriented breast coordinate system for mammogram analysis. IEEE Trans Med Imaging 2011;30:1841-51. [Crossref] [PubMed]
- Englander ZA, Cutcliffe HC, Utturkar GM, Garrett WE, Spritzer CE, DeFrate LE. A Comparison of Knee Abduction Angles Measured by a 3D Anatomic Coordinate System Versus Videographic Analysis: Implications for Anterior Cruciate Ligament Injury. Orthop J Sports Med 2019;7:2325967118819831. [Crossref] [PubMed]
- Miller KJ, Halpern CH, Sedrak MF, Duncan JA, Grant GA. A novel mesial temporal stereotactic coordinate system. J Neurosurg 2018;130:67-75. [Crossref] [PubMed]
- Reiner CS, Williamson T, Winklehner T, Lisse S, Fink D, DeLancey JOL, Betschart C. The 3D Pelvic Inclination Correction System (PICS): A universally applicable coordinate system for isovolumetric imaging measurements, tested in women with pelvic organ prolapse (POP). Comput Med Imaging Graph 2017;59:28-37. [Crossref] [PubMed]
- Innocenti B, Bori E, Piccolo S. Development and validation of a robust patellar reference coordinate system for biomechanical and clinical studies. Knee 2020;27:81-8. [Crossref] [PubMed]
- Namburete AIL, Xie W, Yaqub M, Zisserman A, Noble JA. Fully-automated alignment of 3D fetal brain ultrasound to a canonical reference space using multi-task learning. Med Image Anal 2018;46:1-14. [Crossref] [PubMed]
- Namburete AI, Stebbing RV, Kemp B, Yaqub M, Papageorghiou AT, Alison Noble J. Learning-based prediction of gestational age from ultrasound images of the fetal brain. Med Image Anal 2015;21:72-86. [Crossref] [PubMed]
- Wang H, Bai J, Zhou Y, Zhang Y. Abdominal atlas mapping in CT and MR volume images using a normalized abdominal coordinate system. Comput Med Imaging Graph 2008;32:442-51. [Crossref] [PubMed]
- Satoh K, Wada T, Tachimura T, Sakoda S, Shiba R. A cephalometric study by multivariate analysis of growth of the bony nasopharynx in patients with clefts and non-cleft controls. J Craniomaxillofac Surg 1998;26:394-9. [Crossref] [PubMed]
- Kim S, Ward LA, Butaric LN, Maddux SD. Ancestry-based variation in maxillary sinus anatomy: Implications for health disparities in sinonasal disease. Anat Rec (Hoboken) 2022;305:18-36. [Crossref] [PubMed]
- Cetin S, Teixeira MS, Alper CM. Quantitative representation of Eustachian tube component movements during swallowing. Auris Nasus Larynx 2018;45:73-80. [Crossref] [PubMed]
- Wang R, Li F, Chen S, Liu D, Yang R. A New Method for Anterior Boundary Demarcation of the Nasopharynx in Three-Dimensional Analysis. J Craniofac Surg 2022;33:400-3. [Crossref] [PubMed]
- Wang YW, Wu CS, Chang CH, Cheng KS, Chang YK, Huang IW, Lu CL, Yao WJ. Partial Volume Correction for Equivocal Retropharyngeal Nodal Metastases of Nasopharyngeal Carcinoma with Fluorodeoxyglucose Positron Emission Tomography-Computed Tomography. J Med Biol Eng 2015;35:218-25. [Crossref]
- Liu X, Wang H, Cheng K, Li Y. Relative Location of Fundus Meatus Acustici Interni Via Porus Acusticus Internus in Facial Nerve Decompression. J Craniofac Surg 2017;28:1586-8. [Crossref] [PubMed]
- Zhang Y, Tian Y, Song J, Li Y, Li W. Internal carotid artery in endoscopic endonasal transsphenoidal surgery. J Craniofac Surg 2012;23:1866-9. [Crossref] [PubMed]
- Cui C, Wang S, Zhou J, Dong A, Xie F, Li H, Liu L. Machine Learning Analysis of Image Data Based on Detailed MR Image Reports for Nasopharyngeal Carcinoma Prognosis. Biomed Res Int 2020;2020:8068913. [Crossref] [PubMed]
- Liang SB, Sun Y, Liu LZ, Chen Y, Chen L, Mao YP, Tang LL, Tian L, Lin AH, Liu MZ, Li L, Ma J. Extension of local disease in nasopharyngeal carcinoma detected by magnetic resonance imaging: improvement of clinical target volume delineation. Int J Radiat Oncol Biol Phys 2009;75:742-50. [Crossref] [PubMed]
- Cao C, Jiang F, Jin Q, Jin T, Huang S, Hu Q, Chen Y, Piao Y, Hua Y, Feng X, Chen X. Locoregional extension and patterns of failure for nasopharyngeal carcinoma with intracranial extension. Oral Oncol 2018;79:27-32. [Crossref] [PubMed]
- Chan JYW, Wong STS, Wei WI. Stage II recurrent nasopharyngeal carcinoma: Prognostic significance of retropharyngeal nodal metastasis, parapharyngeal invasion, and carotid encasement. Head Neck 2018;40:103-10. [Crossref] [PubMed]
- Orman G, Tran BH, Desai N, Meoded A, Kralik S, Smith V, Hicks J, Kirsch C, Huisman TAGM. Neuroimaging Characteristics of Nasopharyngeal Carcinoma in Children. J Neuroimaging 2021;31:137-43. [Crossref] [PubMed]
- Bakst RL, Glastonbury CM, Parvathaneni U, Katabi N, Hu KS, Yom SS. Perineural Invasion and Perineural Tumor Spread in Head and Neck Cancer. Int J Radiat Oncol Biol Phys 2019;103:1109-24. [Crossref] [PubMed]
- Xiao J, Wang D, Guo B, Wang L, Su M, Xu H. Observer agreement and accuracy of 18F-sodium fluoride PET/computed tomography in the diagnosis of skull-base bone invasion and osseous metastases in newly diagnosed nasopharyngeal carcinoma. Nucl Med Commun 2020;41:942-9. [Crossref] [PubMed]
- Wang Y, Zhao H, Zhang ZQ, Huang LL, Ye Y, Wang YB, Han MJ. MR imaging prediction of local control of nasopharyngeal carcinoma treated with radiation therapy and chemotherapy. Br J Radiol 2014;87:20130657. [Crossref] [PubMed]
- Tian YM, Xiao WW, Bai L, Liu XW, Zhao C, Lu TX, Han F. Impact of primary tumor volume and location on the prognosis of patients with locally recurrent nasopharyngeal carcinoma. Chin J Cancer 2015;34:247-53. [Crossref] [PubMed]
- Cui C, Li H, Ma H, Dong A, Xie F, Liang S, Li L, Zhou J, Xie C, Yan Y, Liu L. Staging of T2 and T3 nasopharyngeal carcinoma: Proposed modifications for improving the current AJCC staging system. Cancer Med 2020;9:7572-9. [Crossref] [PubMed]
- Huang W, Quan T, Zhao Q, Li S, Cai Y, Zhou J, Luo C, Ruan G, Cui C, Liang S, Li H, Liu L. MRI of nasopharyngeal carcinoma: parapharyngeal subspace involvement has prognostic value and influences T-staging in the IMRT era. Eur Radiol 2022;32:262-71. [Crossref] [PubMed]
- Li WF, Sun Y, Chen M, Tang LL, Liu LZ, Mao YP, Chen L, Zhou GQ, Li L, Ma J. Locoregional extension patterns of nasopharyngeal carcinoma and suggestions for clinical target volume delineation. Chin J Cancer 2012;31:579-87. [Crossref] [PubMed]
- Wang F, Jiang C, Wang L, Yan F, Piao Y, Ye Z, Xu M, Liu J, Fu Z, Jiang Y. Different Risk Target Volumes for Nasopharyngeal Carcinoma Treated with Simultaneous Integrated Boost Intensity-Modulated Radiotherapy. J Cancer 2020;11:5210-22. [Crossref] [PubMed]
- Li AC, Zhang YY, Zhang C, Wang DS, Xu BH. Pathologic study of tumour extension for clinically localized unilateral nasopharyngeal carcinoma: Should the contralateral side be included in the clinical target volume? J Med Imaging Radiat Oncol 2018; Epub ahead of print. [Crossref] [PubMed]
- Bernchou U, Arnold TST, Axelsen B, Klüver-Kristensen M, Mahmood F, Harbo FSG, Asmussen JT, Hansen O, Bertelsen AS, Hansen S, Brink C, Dahlrot RH. Evolution of the gross tumour volume extent during radiotherapy for glioblastomas. Radiother Oncol 2021;160:40-6. [Crossref] [PubMed]
- Shusharina N, Söderberg J, Lidberg D, Niyazi M, Shih HA, Bortfeld T. Accounting for uncertainties in the position of anatomical barriers used to define the clinical target volume. Phys Med Biol 2021;66: [Crossref] [PubMed]
- Sipos D, László Z, Tóth Z, Kovács P, Tollár J, Gulybán A, Lakosi F, Repa I, Kovács A. Additional Value of 18F-FDOPA Amino Acid Analog Radiotracer to Irradiation Planning Process of Patients With Glioblastoma Multiforme. Front Oncol 2021;11:699360. [Crossref] [PubMed]
- Combs SE, Baumert BG, Bendszus M, Bozzao A, Brada M, Fariselli L, Fiorentino A, Ganswindt U, Grosu AL, Lagerwaard FL, Niyazi M, Nyholm T, Paddick I, Weber DC, Belka C, Minniti G. ESTRO ACROP guideline for target volume delineation of skull base tumors. Radiother Oncol 2021;156:80-94. [Crossref] [PubMed]
- Miao J, Di M, Chen B, Wang L, Cao Y, Xiao W, et al. A Prospective 10-Year Observational Study of Reduction of Radiation Therapy Clinical Target Volume and Dose in Early-Stage Nasopharyngeal Carcinoma. Int J Radiat Oncol Biol Phys 2020;107:672-82. [Crossref] [PubMed]
- Yan O, Wang H, Han Y, Fu S, Chen Y, Liu F. Prognostic Relevance of 18F-FDG-PET/CT-Guided Target Volume Delineation in Loco-Regionally Advanced Nasopharyngeal Carcinomas: A Comparative Study. Front Oncol 2021;11:709622. [Crossref] [PubMed]
- Liu F, Xi XP, Wang H, Han YQ, Xiao F, Hu Y, He Q, Zhang L, Xiao Q, Liu L, Luo L, Li Y, Mo Y, Ma HZ. PET/CT-guided dose-painting versus CT-based intensity modulated radiation therapy in locoregional advanced nasopharyngeal carcinoma. Radiat Oncol 2017;12:15. [Crossref] [PubMed]
- Qi Y, Li J, Chen H, Guo Y, Yin Y, Gong G, Wang L. Computer-aided diagnosis and regional segmentation of nasopharyngeal carcinoma based on multi-modality medical images. Int J Comput Assist Radiol Surg 2021;16:871-82. [Crossref] [PubMed]
- Fu S, Li Y, Han Y, Wang H, Chen Y, Yan O, He Q, Ma H, Liu L, Liu F. Diffusion-Weighted Magnetic Resonance Imaging-Guided Dose Painting in Patients With Locoregionally Advanced Nasopharyngeal Carcinoma Treated With Induction Chemotherapy Plus Concurrent Chemoradiotherapy: A Randomized, Controlled Clinical Trial. Int J Radiat Oncol Biol Phys 2022;113:101-13. [Crossref] [PubMed]
- Li S, Chen S, Li H, Ruan G, Ren S, Zhang T, Liu L, Chen H. Anatomical point-of-interest detection in head MRI using multipoint feature descriptor. IEEE Access 2020;8:173239-49.
- Tang P, Zu C, Hong M, Yan R, Peng X, Xiao J, Wu X, Zhou J, Zhou L, Wang Y. DA-DSUnet: Dual Attention-based Dense SU-net for automatic head-and-neck tumor segmentation in MRI images. Neurocomputing 2021;435:103-13. [Crossref]
- Men K, Chen X, Yang B, Zhu J, Yi J, Wang S, Li Y, Dai J. Automatic segmentation of three clinical target volumes in radiotherapy using lifelong learning. Radiother Oncol 2021;157:1-7. [Crossref] [PubMed]
- Wang H, Han G, Li H, Tao G, Zhuo E, Liu L, Cai H, Ou Y. A Collaborative Dictionary Learning Model for Nasopharyngeal Carcinoma Segmentation on Multimodalities MR Sequences. Comput Math Methods Med 2020;2020:7562140. [Crossref] [PubMed]
- Daoud B, Morooka K, Kurazume R, Leila F, Mnejja W, Daoud J. 3D segmentation of nasopharyngeal carcinoma from CT images using cascade deep learning. Comput Med Imaging Graph 2019;77:101644. [Crossref] [PubMed]
- Jiang X, Ma J, Xiao G, Shao Z, Guo X. A review of multimodal image matching: Methods and applications. Information Fusion 2021;73:22-71. [Crossref]
- Xiao H, Teng X, Liu C, Li T, Ren G, Yang R, Shen D, Cai J. A review of deep learning-based three-dimensional medical image registration methods. Quant Imaging Med Surg 2021;11:4895-916. [Crossref] [PubMed]
- Zhu F, Zhu X, Huang Z, Ding M, Li Q, Zhang X. Deep learning based data-adaptive descriptor for non-rigid multi-modal medical image registration. Signal Processing 2021;183:108023. [Crossref]
- Sui X, Zheng Y, He Y, Jia W. Symmetric Deformable Registration via Learning a Pseudomean for MR Brain Images. J Healthc Eng 2021;2021:5520196. [Crossref] [PubMed]
- Zhao F, Wu Z, Wang F, Lin W, Xia S, Shen D, Wang L, Li G. S3Reg: Superfast Spherical Surface Registration Based on Deep Learning. IEEE Trans Med Imaging 2021;40:1964-76. [Crossref] [PubMed]
- Kim B, Kim DH, Park SH, Kim J, Lee JG, Ye JC. CycleMorph: Cycle consistent unsupervised deformable image registration. Med Image Anal 2021;71:102036. [Crossref] [PubMed]
- Zhou B, Augenfeld Z, Chapiro J, Zhou SK, Liu C, Duncan JS. Anatomy-guided multimodal registration by learning segmentation without ground truth: Application to intraprocedural CBCT/MR liver segmentation and registration. Med Image Anal 2021;71:102041. [Crossref] [PubMed]
- Hu J, Luo Z, Wang X, Sun S, Yin Y, Cao K, Song Q, Lyu S, Wu X. End-to-end multimodal image registration via reinforcement learning. Med Image Anal 2021;68:101878. [Crossref] [PubMed]