Quantitative evaluation of density variability in the lesion–lung boundary zone to differentiate pulmonary subsolid nodules
Introduction
With the widespread use of multidetector computed tomography (CT) for lung cancer screening, the detection of incidental pulmonary nodules, especially subsolid nodules (SSNs), has become viable (1-4). SSNs can be classified into part-solid nodules (PSNs) and pure ground-glass nodules (pGGNs) based on whether they contain solid components (5-8). Several SSNs are caused by inflammation or hemorrhage, which can be spontaneously absorbed without intervention. However, some stable or steadily growing SSNs may indicate early lung cancer or preinvasive lesions (9,10). Early diagnosis and treatment of such neoplastic SSNs greatly improve the overall survival rate and prognosis of patients (11). Therefore, it is important to differentiate malignant SSNs from benign lesions.
Many studies have been conducted on the differentiation between benign and neoplastic SSNs, but these have mainly been based on the morphological characteristics of SSNs, such as lobulation, spiculation, border, margin, vacuole, air bronchogram, and pleural indentation (1,12-15). Although these studies demonstrated that neoplastic SSNs tended to have more lobulation, spiculation, air bronchogram, or pleural indentation compared to benign SSNs, some of these features were also common in benign SSNs. The border is particularly important in differentiating benign from malignant SSNs because the proportion of lesions with ill-defined borders in benign SSNs (40.0–66.7%) was significantly higher than that in neoplastic ones (1.6–18.8%) in several studies (13,14,16). However, the SSN border is subjectively evaluated by radiologists, and the results rely on their experience (17). Thus, a method to quantitatively evaluate whether the SSN border is well-defined should be explored.
On CT images of homogeneous substances, the standard deviation (SD) of density reflects image noise, whereas, in heterogeneous substances, SD mainly reflects the density variation (18). Thus, varied CT values of each voxel in the lesion–lung boundary zone of pulmonary nodules mainly represent tissue density transition, which could be quantitatively evaluated by measuring the SD. In addition, measuring the SD value of the lesion–lung boundary zone density has the potential to be an objective method in determining whether the SSN border is well-defined.
In this study, we aimed to evaluate the SD values of the lesion–lung boundary zone in benign and neoplastic SSNs with different degrees of invasiveness to identify whether the SD values could be used to differentiate between benign and neoplastic SSNs. We present the following article in accordance with the STARD reporting checklist (available at https://qims.amegroups.com/article/view/10.21037/qims-22-510/rc).
Methods
Patients
The study was conducted in accordance with the Declaration of Helsinki (as revised in 2013). This study was approved by the institutional review board of the First Affiliated Hospital of Chongqing Medical University (No. 2019-062), and individual consent for this retrospective analysis was waived.
From January 2012 to July 2021, chest CT data of patients with SSNs in the same period were retrospectively and nonconsecutively collected and reviewed. Patients’ data were included if (I) they contained lesions manifested as SSN on CT images with lung window settings, (II) they had SSNs surgically resected and pathologically confirmed by pathological examination, and (III) there was a time interval of less than 1 month between CT examination and surgery. Exclusion criteria were as follows: (I) absence of thin-section CT (≤1 mm) data; (II) presence of excessive solid components that occupied more than 50% of the nodule, or vacuoles, bubble-like lucency, or excessive blood vessels and bronchus in or around the SSNs affecting the measurement; (III) imaging artifacts affecting the lesion evaluation (motion artifacts, metal artifacts, or ray hardening artifact); and (IV) SSNs with measured background noise values greater than 15. The background noise of images was evaluated by drawing regions of interest (ROIs) (50 to 70 mm2) at the aortic arch and descending aorta (the seventh thoracic vertebral level), respectively. Finally, 313 SSNs (229 neoplastic and 84 benign) in 313 patients were enrolled in this study (Figure 1). Among the 229 neoplastic SSNs, 30 were atypical adenomatous hyperplasia (AAH; 18 pGGNs and 12 PSNs), 67 were adenocarcinoma in situ (AIS; 38 pGGNs and 29 PSNs), 70 were minimally invasive adenocarcinoma (MIA; 22 pGGNs and 48 PSNs), and 62 were invasive adenocarcinoma (IA; 7 pGGNs and 55 PSNs). Among the 84 benign GGNs (50 pGGNs and 34 PSNs), 82 were nonspecific inflammation, and 2 were focal interstitial fibrosis. Moreover, 39 patients had 2 or more SSNs. Among the 39 patients, only the SSN with the highest possibility of malignancy was resected in 37 cases at the time when we collected data. Thus, only 1 SSN was confirmed in each patient, and the other unconfirmed SSNs were included or used for analysis although some of them had a higher possibility of being malignant or benign lesions. Another 2 patients had 2 confirmed SSNs, respectively, but only 1 in each patient was studied (the other 2 SSNs contained excessive solid components, which affected the data measurement).
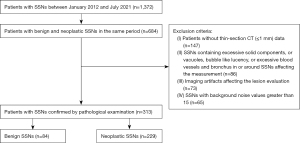
CT examination
Nonenhanced chest CT scans were performed using a 128-slice CT scanner (SOMATOM Perspective, Siemens Healthineers, Erlangen, Germany). All CT scans were performed at the end of inspiration during a single breath-hold. The scan range was from the thoracic inlet to the costophrenic angle. The spiral scanning parameters were as follows: tube voltage of 120 kVp, tube current-time product of 60−140 mAs with automatic exposure control technology, rotation time of 0.5 s, image slice thickness and slice interval of 5/5 mm, pitch of 1.1, detector collimation of 0.6×64 mm, reconstruction slice thickness of 1 mm, and matrix of 512×512. All images were reconstructed using high spatial frequency algorithms (iterative reconstruction: SAFIRE 3; convolution kernel: B80f) for the lung and standard algorithms (iterative reconstruction: SAFIRE 3; convolution kernel: I50f) for the mediastinum. The latter dataset was used to measure the values.
No adverse reactions occurred in any of the patients due to the conventional dose CT plain scans.
Image analysis
All CT images were sent to the picture archiving and communication system (PACS) workstation (Carestream Vue PACS, Rochester, NY, USA) and independently evaluated on the lung window [width 1,200 Hounsfield units (HU); level –600 HU] by 2 senior chest radiologists with 20 and 15 years of experience in chest CT, respectively, who were blinded to pathological results and clinical information. Any discrepancy in qualitative evaluation of the type and border of SSNs was resolved by consensus.
SSNs were evaluated in the following aspects: (I) size, comprising the mean of the longest diameter and perpendicular diameter on axial images; (II) location, comprising the right upper/middle/lower lobe or left upper/lower lobe; and (III) border, comprising well-defined, partly well-defined, or ill-defined.
Two radiologists independently selected axial images with the largest SSN section or the most ground-glass components in the SSN. The CT values of the ground-glass component (CT1) and adjacent normal lung tissue (CT2) in the same section and lobe, excluding the blood vessels, bronchus, or other abnormal structures, were measured by drawing a circular ROI. The density of the lesion–lung boundary zone was measured using the following steps: (I) determining or estimating for a well-defined nodule and partly well-defined or ill-defined nodule, respectively, the SSN boundary line; (II) selecting a point on the boundary, then taking it as the center, and drawing a circular ROI that covered approximately 50% of the ground-glass component and peripheral normal lung tissue, respectively [but that avoided any non-ground-glass opacity (GGO) components]; and (III) recording the mean CT (CT3) and SD values (Figure 2). The ROI varied depending on the SSN size, ranging from 10 to 20 mm2. Similarly, the number of ROIs was 4 to 6, which were evenly distributed on the borderline with similar spacing, with the average value being calculated as the final result after measurement.
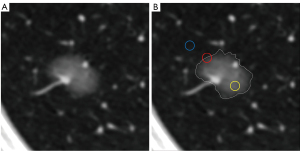
Statistical analysis
Statistical analyses were performed using SPSS 22.0 (IBM Corp, Armonk, NY, USA). Continuous variables are expressed as mean ± SD, whereas categorical variables are expressed as numbers and percentages. The t-test was used to compare the patients’ ages. The size, CT1, CT2, CT3, and SD values of benign and neoplastic SSNs were compared using the Mann-Whitney test. The SD values of SSNs with different degrees of invasiveness were compared using the Kruskal-Wallis test. The patients’ gender, location, type, and border of SSNs were compared using the Pearson chi-squared test. The interobserver consistency of continuous variables measured by the 2 radiologists was analyzed using the intraclass correlation coefficient (ICC). Pearson correlation analysis was used to analyze the correlation between SD values and SSNs with different degrees of invasiveness. The diagnosis performance of border, CT3, and SD values for predicting neoplastic SSNs was calculated using the receiver operating characteristic (ROC) curve. The threshold was determined based on Youden’s index. The Delong test was performed using MedCalc 19.6.4 (MedCalc Software Ltd., Ostend, Belgium) to compare the performance among the areas under the curve (AUCs). A P value less than 0.05 was considered to indicate a statistically significant difference.
Results
Clinical and CT characteristics
Among the 313 patients, 103 were men, 210 were women, and the mean age was 53.6±11.5 (range, 22–85) years. The clinical and CT features of SSNs are listed in Table 1. Compared with men, women patients more often had neoplastic SSNs than benign ones (P=0.011). Compared with benign SSNs, neoplastic ones were larger and had a higher proportion of mixed and well-defined lesions (each P value <0.05).
Table 1
Clinical and CT features | Benign SSN (n=84) | Neoplastic SSN (n=229) | P value |
---|---|---|---|
Gender | 0.011* | ||
Men | 37 (44.0) | 66 (28.8) | |
Women | 47 (56.0) | 163 (71.2) | |
Age (years) | 52.8±10.1 | 53.9±12.0 | 0.455† |
Size (mm) | 9.6±3.8 | 11.0±4.8 | 0.018† |
Location | 0.204* | ||
Right upper lobe | 34 (40.5) | 83 (36.2) | |
Right middle lobe | 2 (2.4) | 13 (5.7) | |
Right lower lobe | 19 (22.6) | 32 (14.0) | |
Left upper lobe | 19 (22.6) | 69 (30.1) | |
Left lower lobe | 10 (11.9) | 32 (14.0) | |
Type | <0.001* | ||
Pure GGN | 50 (59.5) | 85 (37.1) | |
PSN | 34 (40.5) | 144 (62.9) | |
Border | <0.001* | ||
Well-defined | 48 (57.1) | 174 (76.0) | |
Partly well-defined | 27 (32.1) | 49 (21.4) | |
Ill-defined | 9 (10.7) | 6 (2.6) |
Data in parentheses are expressed as a number (percentage) or mean ± standard deviation. *, statistical hypotheses of the Pearson chi-squared test: H0: the overall distribution of patients’ gender and the location, type, and border of SSNs in the 2 groups was the same; H1: the overall distribution of patients’ gender and the location, type, and border of SSNs in the 2 groups was not same; the test level was 0.05. †, statistical hypotheses of the t-test: H0: the overall mean of patients’ age and the size of SSNs in the 2 groups was equal; H1: the overall mean of patients’ age and the size of SSNs in the 2 groups was equal; the test level was 0.05. CT, computed tomography; SSN, subsolid nodule; GGN, ground-glass nodule; PSN, part-solid nodule.
Interobserver consistency test of CT1, CT2, CT3, SD, and background noise values
CT1, CT2, CT3, SD, and background noise values measured by 2 radiologists were all in good agreement (ICC 0.860–0.938; Table 2). The mean background noise of all CT images was 6.3±1.7 (range, 4.1–10.0).
Table 2
Parameters | ICC | 95% CI | P value | |
---|---|---|---|---|
Lower | Upper | |||
CT1 | 0.860 | 0.776 | 0.914 | <0.001 |
CT2 | 0.870 | 0.792 | 0.921 | <0.001 |
CT3 | 0.889 | 0.821 | 0.932 | <0.001 |
SD | 0.883 | 0.812 | 0.929 | <0.001 |
Background noise | 0.938 | 0.873 | 0.970 | <0.001 |
Statistical hypotheses of the ICC: H0: the CT1, CT2, CT3, and SD values measured by 2 observers were independent; H1: the CT1, CT2, CT3, and SD values measured by 2 observers were consistent; the test level was 0.05. CT, computed tomography; ICC, intraclass correlation coefficient; CI, confidence interval; SD, standard deviation.
Correlation between the SD value and subjective evaluation of the lesion boundary
A significant difference was observed in the SD value of the lesion–lung boundary zone among the well-defined, partly well-defined, and ill-defined SSNs (P<0.001). The SD value in the well-defined group (128.2±35.5) was higher than that in the ill-defined (92.8±30.5) and partly well-defined groups (108.0±31.2; each P value <0.001). However, no significant difference was observed between the ill-defined and partly well-defined groups (P=0.119).
Comparison of CT1, CT2, CT3, and SD values of SSNs between the benign and neoplastic groups
The comparison of CT1, CT2, CT3, and SD values between benign and neoplastic SSNs is summarized in Table 3. CT3 and SD values of neoplastic SSNs were significantly higher than those of benign SSNs (P<0.001).
Table 3
Parameters | Benign SSN | Neoplastic SSN | P value |
---|---|---|---|
CT1 (HU) | –632.1±98.3 | –647.2±122.4 | 0.198 |
CT2 (HU) | –885.6±36.9 | –888.1±32.4 | 0.690 |
CT3 (HU) | –792.6±73.9 | –736.1±51.0 | <0.001 |
SD | 83.6±20.6 | 135.6±29.6 | <0.001 |
Data are expressed as mean ± SD. Statistical hypotheses of the Mann-Whitney test: H0: the overall distribution of CT1, CT2, CT3, and SD values in the 2 groups was the same; H1: the overall distribution of CT1, CT2, CT3, and SD values in the 2 groups was not same; the test level was 0.05. CT, computed tomography; SD, standard deviation; SSN, subsolid nodule; HU, Hounsfield units.
SD value changes from benign SSNs to neoplastic SSNs
Significant differences in the SD value were observed between benign SSNs (83.6±20.6) and AAH (109.1±33.1), AIS (134.2±24.5), MIA (140.5±27.1), and IA (144.2±28.8; each P value <0.001). Among neoplastic SSNs, the SD value of AAH was significantly lower than those of AIS, MIA, or IA (each P value <0.01), whereas no significant differences were observed among AIS, MIA, and IA (each P value >0.05; Figure 3). The SD value increased with the invasive degree of SSNs, and the correlation coefficient was 0.657 (P<0.001; Figure 4).
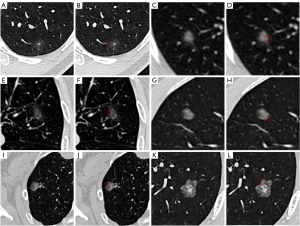
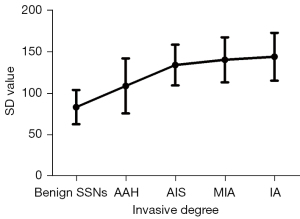
The ability of lesion border, CT3, and SD values to distinguish benign from neoplastic SSNs
Two ROCs were drawn based on the subjective evaluation results of the lesion–lung boundary to distinguish neoplastic SSNs from benign SSNs. One ROC was plotted with a well-defined boundary (Model 1), and the other ROC was plotted with a well-defined and partly well-defined boundary (Model 2). The results showed that the AUC in Model 1 was 0.594 (95% CI: 0.521–0.667; sensitivity 0.760; specificity 0.429) and that in Model 2 was 0.540 (95% CI: 0.466–0.615; sensitivity 0.893; specificity 0.107; Figure 5A).
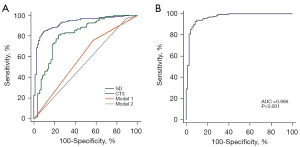
When CT3 was used as a factor to distinguish neoplastic SSNs from benign SSNs, the AUC was 0.814 (95% CI: 0.756–0.872). The optimal threshold of CT3 to determine neoplastic SSNs was −780.84 HU, and the corresponding sensitivity and specificity were 0.808 and 0.762, respectively. Furthermore, ROC analysis showed that the AUC was 0.927 (95% CI: 0.896–0.959) when the SD value was used as a factor to distinguish neoplastic SSNs from benign SSNs. The optimal threshold of the SD value to determine neoplastic SSNs was 106.98, and the corresponding sensitivity and specificity were 0.843 and 0.905, respectively. The performance of SD values to distinguish benign from neoplastic SSNs was significantly higher than that of the border and CT3 values (all P values <0.001; Figure 5A).
Considering the correlation between the SD value and ground-glass component density, the ability of the SD value to distinguish between benign and neoplastic SSNs was strongest when only SSNs with the CT1 value of ≥−715 HU were included (70 benign and 171 neoplastic SSNs). ROC analysis showed that the AUC increased to 0.966 (95% CI: 0.934–0.985). The optimal threshold to determine neoplastic SSNs was 109.9, and the corresponding sensitivity and specificity increased to 0.930 and 0.914, respectively (Figure 5B).
Discussion
Clinically, chest CT is usually used to diagnose SSNs, and some SSNs can be correctly diagnosed based on their morphological characteristics. However, most radiological features are subjective, and some may have poor repeatability due to the variability in personal experiences of radiologists (19,20). Therefore, a quantitative evaluation method to aid in diagnosis should be explored. The lesion–lung interface is one of the common CT features used to distinguish SSNs. Whether it is well-defined or not is mainly related to the density transition of the lesion–lung boundary zone, which could be quantitatively reflected by measuring its SD. The greater the CT value variability is, the faster the density of the lesion–lung boundary zone transits, the higher the SD value is, and the clearer the lesion boundary appears.
In this study, a significant difference in CT3 and its SD was observed between benign and neoplastic SSNs. The SD value was associated with whether the border was well-defined or not. However, the SD value’s ability to distinguish between benign and neoplastic SSNs was significantly higher than that of the border, especially for SSNs with a higher CT1. These results indicated that whether the SSN was benign or neoplastic was closely associated with the SD value of the lesion–lung boundary zone rather than the lesion border. Thus, the SD value could be a potential objective and quantitative indicator for differentiating benign from neoplastic SSNs.
Normally, most benign SSNs are caused by inflammation, which usually results in a series of pathophysiological changes, such as increased vascular permeability, inflammatory cell infiltration, and serous exudation (21,22). When inflammatory exudation peripherally diffuses through the alveolar pore, the peripheral lung tissues around nodules contain more cells and/or liquid and show an increased density (14,23). Therefore, the density difference between SSNs and adjacent lung tissues reduces. In contrast, with the increased invasiveness degree of neoplastic SSNs, the tumor cell arrangement on alveolar walls becomes dense and thickens but does not exhibit boundless infiltration, forming a well-defined lesion–lung border (22,24). Thus, the SD value in benign SSNs is significantly lower than that in neoplastic ones in the cases of similar CT1 and CT2 values.
When the SD value was used to distinguish SSNs, some SSNs still could not be correctly diagnosed. This may be because some SSNs had a slightly high density, especially neoplastic ones, which resulted in a relatively small density difference in the lesion–lung boundary zone. Therefore, even if the neoplastic SSN boundary was well-defined, its SD value was still lower. As expected, the diagnostic performance of the SD value to distinguish benign from neoplastic SSNs was enhanced when SSNs with a CT1 value of less than −715 HU were excluded. This finding suggests that the application of the SD value in differentiating benign SSNs from neoplastic SSNs was limited in lesions with a CT1 value of less than −715 HU. In this condition, differentiating SSNs should be combined with other CT features.
Between benign and neoplastic SSNs, the CT1 and CT2 values were all similar, but the CT3 values were significantly different. This might have occurred because the ROI was placed close to the lesion border when measuring CT3. Pathologically, the density of lesions gradually decreases from their center to their periphery in some inflammatory SSNs due to the spread of inflammatory exudation (23). Conversely, this condition is not significant in neoplastic SSNs. Consequently, the CT3 of benign SSNs was lower than that of neoplastic SSNs. Because CT3 was only correlated with CT1 and could not reflect the density variability in the lesion–lung boundary zone, its performance in differentiating benign SSNs from neoplastic SSNs was significantly lower than that of the SD value.
This study also showed that the SD value increased as the invasiveness degree of neoplastic SSNs increased, indicating that the higher the degree of invasion, the greater the density of the lesion–lung boundary zone transition, with a more well-defined SSN. Previous studies also report similar findings, in which the proportions of well-defined cases increased with the invasiveness degree of neoplastic SSNs (2,24-26). This may be related to the decreased gas content associated with the increased number of tumor cells and interlobular septal thickening as the tumor evolves and grows. Whether the SD value could be used to predict the invasiveness of pure GGO should be verified in further studies with large sample sizes.
In previous studies, the subjective evaluation revealed that more neoplastic SSNs than benign SSNs had well-defined borders (1,13,14,16). A similar result was also found in this study. Nevertheless, the performance of the subjectively evaluated lesion boundary in distinguishing SSNs was significantly lower than that of the SD value. Moreover, compared with the subjective evaluation of the SSN boundary, the measurement of SD value can overcome the radiologist’s subjectivity, especially for ambiguous SSNs. Additionally, this objective method has good interobserver consistency, which indicates it is suitable for clinical practice.
This study had several limitations. First, SSNs were not enrolled consecutively, and some PSNs with excessive solid components were not included in this study because their SD values could not be properly measured. This might have led to selection bias. Second, the sample size was relatively small, and the results should be verified in further studies. Third, although the interobserver consistency of the measurements in this study was good, interobserver variability in the manual measurement process was inevitable, which might have affected the results.
Conclusions
The lesion–lung boundary is a key factor for differentiating benign from neoplastic SSNs. Compared with the subjective evaluation of the lesion boundary, quantitatively measuring the SD value of the lesion–lung boundary zone can achieve this goal with a higher discriminative ability. Thus, the SD that reflects the density variability could be considered an objective index for differentiating benign from neoplastic SSNs, especially for SSNs with a density of GGO of ≥−715 HU, which have a higher possibility of neoplasm if the SD is >109.9.
Acknowledgments
Funding: This work was supported by the Joint Project of Chongqing Science and Technology Commission and Chongqing Public Health Commission (No. 2022MSXM050) and the National Natural Science Foundation of China (No. 81601545); Senior Medical Talents Program of Chongqing for Young and Middle-aged from Chongqing Health Commission (Receptor: ZG Chu).
Footnote
Reporting Checklist: The authors have completed the STARD reporting checklist. Available at https://qims.amegroups.com/article/view/10.21037/qims-22-510/rc
Conflicts of Interest: All authors have completed the ICMJE uniform disclosure form (available at https://qims.amegroups.com/article/view/10.21037/qims-22-510/coif). The authors have no conflicts of interest to declare.
Ethical Statement: The authors are accountable for all aspects of the work in ensuring that questions related to the accuracy or integrity of any part of the work are appropriately investigated and resolved. The study was conducted in accordance with the Declaration of Helsinki (as revised in 2013). This study was approved by the institutional review board of the First Affiliated Hospital of Chongqing Medical University (No. 2019-062), and individual consent for this retrospective analysis was waived.
Open Access Statement: This is an Open Access article distributed in accordance with the Creative Commons Attribution-NonCommercial-NoDerivs 4.0 International License (CC BY-NC-ND 4.0), which permits the non-commercial replication and distribution of the article with the strict proviso that no changes or edits are made and the original work is properly cited (including links to both the formal publication through the relevant DOI and the license). See: https://creativecommons.org/licenses/by-nc-nd/4.0/.
References
- Fu BJ, Lv FJ, Li WJ, Lin RY, Zheng YN, Chu ZG. Significance of intra-nodular vessel sign in differentiating benign and malignant pulmonary ground-glass nodules. Insights Imaging 2021;12:65. [Crossref] [PubMed]
- Chu ZG, Li WJ, Fu BJ, Lv FJ. CT Characteristics for Predicting Invasiveness in Pulmonary Pure Ground-Glass Nodules. AJR Am J Roentgenol 2020;215:351-8. [Crossref] [PubMed]
- Wang J, Ma H, Ni CJ, He JK, Ma HT, Ge JF. Clinical characteristics and prognosis of ground-glass opacity nodules in young patients. J Thorac Dis 2019;11:557-63. [Crossref] [PubMed]
- Tsutsui S, Ashizawa K, Minami K, Tagawa T, Nagayasu T, Hayashi T, Uetani M. Multiple focal pure ground-glass opacities on high-resolution CT images: Clinical significance in patients with lung cancer. AJR Am J Roentgenol 2010;195:W131-8. [Crossref] [PubMed]
- Li J, Xia T, Yang X, Dong X, Liang J, Zhong N, Guan Y. Malignant solitary pulmonary nodules: assessment of mass growth rate and doubling time at follow-up CT. J Thorac Dis 2018;10:S797-806. [Crossref] [PubMed]
- Kobayashi Y, Ambrogio C, Mitsudomi T. Ground-glass nodules of the lung in never-smokers and smokers: clinical and genetic insights. Transl Lung Cancer Res 2018;7:487-97. [Crossref] [PubMed]
- Wu G, Woodruff HC, Shen J, Refaee T, Sanduleanu S, Ibrahim A, Leijenaar RTH, Wang R, Xiong J, Bian J, Wu J, Lambin P. Diagnosis of Invasive Lung Adenocarcinoma Based on Chest CT Radiomic Features of Part-Solid Pulmonary Nodules: A Multicenter Study. Radiology 2020;297:451-8. [Crossref] [PubMed]
- Zhang BW, Zhang Y, Ye JD, Qiang JW. Use of relative CT values to evaluate the invasiveness of pulmonary subsolid nodules in patients with emphysema. Quant Imaging Med Surg 2021;11:204-14. [Crossref] [PubMed]
- Lee SM, Park CM, Goo JM, Lee CH, Lee HJ, Kim KG, Kang MJ, Lee IS. Transient part-solid nodules detected at screening thin-section CT for lung cancer: comparison with persistent part-solid nodules. Radiology 2010;255:242-51. [Crossref] [PubMed]
- Digumarthy SR, Padole AM, Rastogi S, Price M, Mooradian MJ, Sequist LV, Kalra MK. Predicting malignant potential of subsolid nodules: can radiomics preempt longitudinal follow up CT? Cancer Imaging 2019;19:36. [Crossref] [PubMed]
- Aberle DR, Adams AM, Berg CD, Black WC, Clapp JD, Fagerstrom RM, Gareen IF, Gatsonis C, Marcus PM, Sicks JD. Reduced lung-cancer mortality with low-dose computed tomographic screening. N Engl J Med 2011;365:395-409. [Crossref] [PubMed]
- Qin Y, Xu Y, Ma D, Tian Z, Huang C, Zhou X, He J, Liu L, Guo C, Wang G, Zhang J, Wang Y, Liu H. Clinical characteristics of resected solitary ground-glass opacities: Comparison between benign and malignant nodules. Thorac Cancer 2020;11:2767-74. [Crossref] [PubMed]
- Gao F, Sun Y, Zhang G, Zheng X, Li M, Hua Y. CT characterization of different pathological types of subcentimeter pulmonary ground-glass nodular lesions. Br J Radiol 2019;92:20180204. [Crossref] [PubMed]
- Yang W, Sun Y, Fang W, Qian F, Ye J, Chen Q, Jiang Y, Yu K, Han B. High-resolution Computed Tomography Features Distinguishing Benign and Malignant Lesions Manifesting as Persistent Solitary Subsolid Nodules. Clin Lung Cancer 2018;19:e75-83. [Crossref] [PubMed]
- Li WJ, Lv FJ, Tan YW, Fu BJ, Chu ZG. Benign and malignant pulmonary part-solid nodules: differentiation via thin-section computed tomography. Quant Imaging Med Surg 2022;12:699-710. [Crossref] [PubMed]
- Fan L, Liu SY, Li QC, Yu H, Xiao XS. Multidetector CT features of pulmonary focal ground-glass opacity: differences between benign and malignant. Br J Radiol 2012;85:897-904. [Crossref] [PubMed]
- Wu L, Gao C, Xiang P, Zheng S, Pang P, Xu M. CT-Imaging Based Analysis of Invasive Lung Adenocarcinoma Presenting as Ground Glass Nodules Using Peri- and Intra-nodular Radiomic Features. Front Oncol 2020;10:838. [Crossref] [PubMed]
- Chun M, Choi YH, Kim JH. Automated measurement of CT noise in patient images with a novel structure coherence feature. Phys Med Biol 2015;60:9107-22. [Crossref] [PubMed]
- Singh S, Pinsky P, Fineberg NS, Gierada DS, Garg K, Sun Y, Nath PH. Evaluation of reader variability in the interpretation of follow-up CT scans at lung cancer screening. Radiology 2011;259:263-70. [Crossref] [PubMed]
- van Riel SJ, Sánchez CI, Bankier AA, Naidich DP, Verschakelen J, Scholten ET, de Jong PA, Jacobs C, van Rikxoort E, Peters-Bax L, Snoeren M, Prokop M, van Ginneken B, Schaefer-Prokop C. Observer Variability for Classification of Pulmonary Nodules on Low-Dose CT Images and Its Effect on Nodule Management. Radiology 2015;277:863-71. [Crossref] [PubMed]
- Li WJ, Lv FJ, Tan YW, Fu BJ, Chu ZG. Pulmonary Benign Ground-Glass Nodules: CT Features and Pathological Findings. Int J Gen Med 2021;14:581-90. [Crossref] [PubMed]
- Nambu A, Araki T, Taguchi Y, Ozawa K, Miyata K, Miyazawa M, Hiejima Y, Saito A. Focal area of ground-glass opacity and ground-glass opacity predominance on thin-section CT: discrimination between neoplastic and non-neoplastic lesions. Clin Radiol 2005;60:1006-17. [Crossref] [PubMed]
- Lin RY, Lv FJ, Fu BJ, Li WJ, Liang ZR, Chu ZG. Features for Predicting Absorbable Pulmonary Solid Nodules as Depicted on Thin-Section Computed Tomography. J Inflamm Res 2021;14:2933-9. [Crossref] [PubMed]
- Meng Y, Liu CL, Cai Q, Shen YY, Chen SQ. Contrast analysis of the relationship between the HRCT sign and new pathologic classification in small ground glass nodule-like lung adenocarcinoma. Radiol Med 2019;124:8-13. [Crossref] [PubMed]
- Sun Y, Li C, Jin L, Gao P, Zhao W, Ma W, Tan M, Wu W, Duan S, Shan Y, Li M. Radiomics for lung adenocarcinoma manifesting as pure ground-glass nodules: invasive prediction. Eur Radiol 2020;30:3650-9. [Crossref] [PubMed]
- Wu F, Tian SP, Jin X, Jing R, Yang YQ, Jin M, Zhao SH. CT and histopathologic characteristics of lung adenocarcinoma with pure ground-glass nodules 10 mm or less in diameter. Eur Radiol 2017;27:4037-43. [Crossref] [PubMed]