Histogram analysis of breast diffusion kurtosis imaging: a comparison between readout-segmented and single-shot echo-planar imaging sequence
Introduction
Dynamic contrast-enhanced magnetic resonance imaging (DCE-MRI) is the most widely used MR imaging sequence for identification breast lesions (1). However, the specificity of DCE-MRI varies from 37% to 97% in the diagnosis of breast lesions due to the background parenchymal enhancement and the overlap of the time intensity curves (2,3). Diffusion-weighted imaging (DWI) of the breast has gained clinical acceptance for being an effective adjunctive sequence to DCE-MRI, which is widely used to evaluate the morphological changes and assess the semi-quantitative kinetic parameters of the breast lesions (4-6). The apparent diffusion coefficient (ADC) estimates the diffusion behavior of the water molecules within the tissues in a conventional DWI based on the mono-exponential decay of the signal intensity (SI) (7,8). ADC is based on the Gaussian diffusion model, which assumes that the water molecules diffuse freely and uniformly in the tissues (9). However, the tissue microstructure is highly complex, and the DWI signal intensity decay plot deviates from a simple mono-exponential pattern as the b-value increases (10,11). Jensen et al. proposed the diffusion kurtosis imaging (DKI) method in 2005 to describe the non-Gaussian diffusion of the water molecules (12). DKI estimates the kurtosis or skewed distribution of water diffusion and diffusivity or the kurtosis-corrected diffusion coefficient (13,14). Several studies have demonstrated that DKI probes non-Gaussian movement of water molecules in tissue environments and the kurtosis value calculated by the DKI model through more advanced mathematical curve fitting presumably reflects the heterogeneity of cellular microstructure (15-18). Sun et al. demonstrated that DKI may be a noninvasive method to evaluate the effects of neoadjuvant chemotherapy for breast cancer (10).
Currently, single-shot echo-planar imaging (ss-EPI) is the most commonly used DWI sequence in clinical practice for breast tumor detection and characterization (19). However, ss-EPI is associated with susceptibility-induced geometric distortion and image blurring (20,21). Readout segmented echo-planar imaging (rs-EPI) is a multi-shot strategy that divides the k-space into multiple segments along the readout direction and can overcome the shortcomings of the ss-EPI technique (22). Several studies have demonstrated that the rs-EPI sequence provided significantly higher image quality and lesion conspicuity than the ss-EPI sequence (23,24). The major challenge faced with rs-EPI is a comparatively long scan time than that for single-shot echo-planar imaging. This can be explained by the higher actual resolution of the rs-EPI sequence compared with single-shot echo-planar imaging and the more efficient k-space coverage of single-shot echo-planar imaging (25).
Histogram analysis can be used to estimate intratumor heterogeneity and compare lesions because it describes the statistical information of the MRI parameters for the lesions (23). Histogram analysis of the MRI parameters from the ss-EPI sequence is a promising approach to differentiate the breast lesions and predict the risk of breast cancer recurrence (24,26). However, to our knowledge, histogram analysis of the DKI image parameters for the rs-EPI and ss-EPI sequences have not been compared in breast cancer. Therefore, the aim of the present study was to compare the diagnostic accuracy and reliability of the rs-EPI and ss-EPI sequences based on histogram analysis of the DKI parameters. We present the following article in accordance with the STARD reporting checklist (available at https://qims.amegroups.com/article/view/10.21037/qims-22-475/rc).
Methods
Study patients
The study was conducted in accordance with the Declaration of Helsinki (as revised in 2013). This educational review includes a statement on ethics approval and consent from the ethics committee of Tongji Hospital, Tongji Medical College, Huazhong University of Science. Written informed consent was received from each participant before participation in the study. 406 women with suspected breast lesions diagnosed as Breast Imaging reporting and Data System (BI-RADS) category 3 or higher at mammography or ultrasonography in our hospital were selected for breast MR imaging between May 2016 and March 2017. The inclusion criteria included: (I) 18 years or older; (II) no pregnancy or lactation. 387 patients were approached for inclusion in the study, and 342 consented to participate. The exclusion criteria included (I) poor image quality with severe susceptibility or motion artifacts; (II) previous treatment including radiotherapy or neoadjuvant chemotherapy for breast lesions before MRI; (III) absence of visible lesions on the b-1000 images of two DWI sequences; (IV) lesion size (minimum diameter) <5 mm on the b-1000 images of two DWI sequences; and (V) patients without histopathological results confirmed by surgery or needle biopsy within two weeks after breast MRI. Finally, 205 patients (mean age ± SD, 40.6±16.5 years; age range, 22–63 years) were enrolled in this study. The flowchart of this study is displayed on Figure 1.
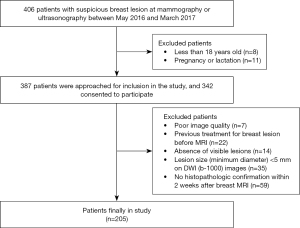
MRI acquisition
The breast MRI examinations were performed on a 3T MRI scanner (MAGNETOM Skyra, Siemens Healthcare) equipped with a dedicated bilateral 16-channel phased-array breast coil. The MR imaging was performed with the patient in a prone position using the following sequences: (I) axial fat-saturated T2-weighted imaging (T2WI); (II) single-shot echo-planar imaging (ss-EPI); (III) readout-segmented echo-planar imaging (rs-EPI); (IV) axial T1-weighted DCE-MRI. The parameters of the sequences are shown in Table 1.The ss-EPI and rs-EPI sequences were applied with 4 b values (0, 50, 1,000, and 2,000 s/mm2).
Table 1
Parameters | T2-weighted | DWI sequences | DCE-MRI | |
---|---|---|---|---|
ss-EPI | rs-EPI | |||
Repetition time (ms) | 3,700 | 5,000 | 5,000 | 5.40 |
Echo time (ms) | 101 | 96 | 68 | 2.46 |
Field of view (mm2) | 320×320 | 169×280 | 169×280 | 270×320 |
Voxel size (mm3) | 1×1×4 | 1.5×1.5×5 | 1.5×1.5×5 | 1×1×1.5 |
Flip angle (°) | 137 | 180 | 180 | 10 |
Slice thickness (mm) | 4 | 5 | 5 | 1.5 |
Parallel imaging | GRAPPA2 | GRAPPA2 | GRAPPA2 | GRAPPA2 + CAIPIRINHA2 |
b-value (s/mm2) | – | 0, 50, 1,000, 2,000 | 0, 50, 1,000, 2,000 | – |
Readout segments | – | – | 5 | – |
Averages | – | 5 | 1 | – |
Acquisition time (min) | 2:06 | 4:35 | 4:27 | 5:57 |
MRI, magnetic resonance imaging; DWI, diffusion-weighted imaging; ss-EPI, single-shot echo-planar imaging; rs-EPI, readout-segmented echo planar imaging; DCE-MRI, dynamic contrast-enhanced magnetic resonance imaging; GRAPPA, generalized autocalibrating partially parallel acquisition; CAIPIRINHA, controlled aliasing in parallel imaging results in higher acceleration; mm, millimeter.
Image analysis
All the imaging datasets were analyzed with the prototype software, Body Diffusion Toolbox (version 0.2.2, Siemens Healthcare). The apparent diffusion for non-Gaussian distribution (Dapp) and the apparent diffusional kurtosis (Kapp) values were calculated for both the DWI sequences using the following equation:
Where S(b) is the signal intensity at the echo time, Dapp is the apparent diffusion for non-Gaussian distribution, and Kapp is the apparent diffusional kurtosis; Where S(b) represents the DWI signal intensity at a particular b value; S(0) represents the baseline signal at b=0; b represents the degree of diffusion weighting applied to calculate Dapp and Kapp; and O(b3) represents the fit error associated with the measurements (11).
Two experienced radiologists with 10 years (reader 1) and 7 years (reader 2) of experience in DW imaging of the breast independently evaluated all the images simultaneously. Disagreement between the two observers was resolved in consensus. The readers were blinded to patient clinical histories, histopathologic diagnoses, and sequence information. Two-dimensional regions of interest (ROIs) were manually delineated on the rs-EPI images with a b value of 1,000 s/mm2. Freehand ROIs (>200 mm2 quasi-circular area) were drawn along the outer edge of the lesions. The areas with sizeable cystic necrosis, calcifications, or blood vessels were avoided by referring to the corresponding T2WI and DCE-MRI images. The same ROIs were copied to the ss-EPI images and the parametric maps. Histogram parameters including the mean, 25th, 50th, 75th and 100th percentiles, skewness, and kurtosis were recorded for all the ROIs and compared.
Statistical analysis
SPSS version 20.0 (SPSS, Chicago, IL, USA) and MedCalc version 12.0 (MedCalc Software, Mariakerke, Belgium) software were used for the statistical analysis. The data with normal distribution were analyzed using the Kolmogorov-Smirnov test. Inter-class agreements for the histogram parameter measurements by the two readers were assessed by calculating the intra-class correlation coefficients (ICCs) (27). ICC values less than 0.4 were considered poor; ICC values between 0.40 to 0.59 were considered fair; ICC values between 0.60 to 0.74 were considered good, and ICC values greater than 0.75 were considered excellent (28).
The Student’s t-test was used to compare the histogram parameters of MK and MD from the ss-EPI and rs-EPI sequences for the benign and malignant lesions, respectively, and the differences in the histogram parameters of Dapp and Kapp between the benign and malignant breast lesions in the ss-EPI or the rs-EPI sequences. Receiver operating characteristic (ROC) curves were used to evaluate the diagnostic accuracy of the histogram parameters in differentiating the malignant lesions from the benign lesions. The area under the receiver operating characteristic (AUC), sensitivity, and specificity values were estimated for all the histogram parameters of Dapp and Kapp using the ROC curves. The differences in the AUC values between the ss-EPI and rs-EPI sequences were compared for all the histogram parameters. P<0.05 was considered statistically significant.
Results
Basic clinicopathologic characteristics of the included study subjects
Among the 205 breast lesions confirmed by histopathology, 65 (32%) were benign and 140 (68%) were malignant. The histologic types of the breast lesions are shown in Table 2. The ss-EPI and rs-EPI images of two representative cases with benign and malignant breast lesions are shown in Figure 2.
Table 2
Groups | Histological types | Number |
---|---|---|
Benign (N=65) | Fibroadenoma | 45 |
Adenosis | 11 | |
Fibrous epithelial tumor | 1 | |
Benign phyllode tumor | 2 | |
Intraductal papilloma | 6 | |
Malignant (N=140) | Invasive ductal carcinoma | 116 |
Invasive lobular carcinoma | 3 | |
Ductal carcinomas in situ | 16 | |
Malignant phyllode tumor | 5 |
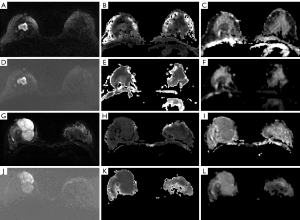
Histogram analysis shows significant differences in the DKI parameters between malignant and benign breast lesions
In both the DKI sequences, the inter-reader agreement was excellent for the histogram parameters such as the mean and the 25th, 50th, 75th, and 100th percentiles of Dapp and Kapp (ICC: 0.776 to 0.863), and good for skewness and kurtosis of Dapp and Kapp (ICC: 0.695 to 0.734).
The histogram analysis showed significant differences in the DKI parameters between the benign and the malignant lesions for both sequences (Table 3 and Figure 3). The malignant breast lesions showed significantly lower mean and 25th, 50th, 75th, and 100th percentiles of the Dapp values and significantly higher mean and 25th, 50th, 75th and 100th percentiles of the Kapp values compared with the benign breast lesions for both the sequences (all P<0.05). The skewness values of Dapp and Kapp were significantly higher for the malignant breast lesions than for the benign breast lesions (P<0.05). The kurtosis values of Dapp and Kapp showed no difference between the benign and malignant breast lesions for both DWI sequences (P>0.05).
Table 3
Variables | ss-EPI | rs-EPI | |||||
---|---|---|---|---|---|---|---|
Benign lesions | Malignant lesions | P | Benign lesions | Malignant lesions | P | ||
Histogram Kapp | |||||||
Mean | 0.77±0.19 | 0.98±0.14 | <0.01 | 0.65±0.19 | 0.89±0.14 | <0.01 | |
25th | 0.72±0.17 | 0.89±0.14 | <0.01 | 0.59±0.18 | 0.80±0.14 | <0.01 | |
50th | 0.77±0.19 | 0.98±0.16 | <0.01 | 0.66±0.19 | 0.89±0.16 | <0.01 | |
75th | 0.82±0.22 | 1.08±0.20 | <0.01 | 0.72±0.20 | 0.98±0.20 | <0.01 | |
100th | 1.04±0.34 | 1.41±0.73 | <0.01 | 0.92±0.30 | 1.31±0.43 | <0.01 | |
Skewness | −0.24±1.20 | −0.01±1.10 | 0.03 | −0.43±1.06 | 0.01±1.15 | <0.01 | |
Kurtosis | 5.13±3.82 | 4.53±3.28 | 0.29 | 5.18±2.85 | 4.93±4.34 | 0.43 | |
Histogram Dapp | |||||||
Mean | 1.68±0.39 | 1.21±0.28 | <0.01 | 1.66±0.34 | 1.23±0.27 | <0.01 | |
25th | 1.57±0.39 | 1.06±0.26 | <0.01 | 1.54±0.35 | 1.08±0.23 | <0.01 | |
50th | 1.67±0.39 | 1.18±0.28 | <0.01 | 1.65±0.35 | 1.20±0.26 | <0.01 | |
75th | 1.78±0.39 | 1.34±0.32 | <0.01 | 1.77±0.35 | 1.35±0.31 | <0.01 | |
100th | 2.27±0.63 | 1.90±0.50 | <0.01 | 2.36±0.57 | 1.89±0.49 | <0.01 | |
Skewness | 0.40±0.78 | 0.80±0.85 | <0.01 | 0.48±0.84 | 0.73±0.71 | <0.01 | |
Kurtosis | 4.32±2.45 | 4.29±2.55 | 0.48 | 4.63±3.14 | 3.95±1.93 | 0.07 |
Data are expressed as mean ± standard deviation. ss-EPI, single-shot echo-planar imaging; rs-EPI, readout-segmented echo planar imaging; Kapp, apparent diffusional kurtosis; Dapp, apparent diffusion for non-Gaussian distribution.
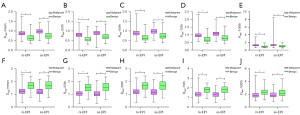
The histogram analysis showed significant differences in the mean and the 25th, 50th, 75th, and 100th percentiles of Dapp and Kapp between the ss-EPI and rs-EPI sequences for both benign and malignant breast lesions (all P<0.05; Table 4). Furthermore, the skewness of Dapp values between the ss-EPI and rs-EPI sequences were significantly different for both benign and the malignant breast lesions (P<0.05; Table 4).
Table 4
Variables | Benign lesion (n=65) | Malignant lesion (n=140) | |||||
---|---|---|---|---|---|---|---|
ss-EPI | rs-EPI | P | ss-EPI | rs-EPI | P | ||
Histogram Kapp | |||||||
Mean | 0.77±0.19 | 0.65±0.19 | <0.01 | 0.98±0.14 | 0.89±0.14 | <0.01 | |
25th | 0.72±0.17 | 0.59±0.18 | <0.01 | 0.89±0.14 | 0.80±0.14 | <0.01 | |
50th | 0.77±0.19 | 0.66±0.19 | <0.01 | 0.98±0.16 | 0.89±0.16 | <0.01 | |
75th | 0.82±0.22 | 0.72±0.20 | <0.01 | 1.08±0.20 | 0.98±0.20 | <0.01 | |
100th | 1.04±0.34 | 0.92±0.30 | <0.01 | 0.92±0.30 | 1.31±0.43 | <0.01 | |
Skewness | −0.24±1.20 | −0.43±1.06 | 0.14 | -0.01±1.10 | 0.01±1.15 | 0.33 | |
Kurtosis | 5.13±3.82 | 5.18±2.85 | 0.11 | 4.53±3.28 | 4.93±4.34 | <0.01 | |
Histogram Dapp | |||||||
Mean | 1.68±0.39 | 1.66±0.34 | <0.01 | 1.21±0.28 | 1.23±0.27 | <0.01 | |
25th | 1.57±0.39 | 1.54±0.35 | <0.01 | 1.06±0.26 | 1.08±0.23 | <0.01 | |
50th | 1.67±0.39 | 1.65±0.35 | <0.01 | 1.18±0.28 | 1.20±0.26 | <0.01 | |
75th | 1.78±0.39 | 1.77±0.35 | <0.01 | 1.34±0.32 | 1.35±0.31 | <0.01 | |
100th | 2.27±0.63 | 2.36±0.57 | <0.01 | 1.90±0.50 | 1.89±0.49 | <0.01 | |
Skewness | 0.40±0.78 | 0.48± 0.84 | <0.01 | 0.80±0.85 | 0.73±0.71 | <0.01 | |
Kurtosis | 4.32±2.45 | 4.63±3.14 | 0.06 | 4.29±2.55 | 3.95±1.93 | <0.01 |
Data are expressed as mean ± standard deviation. ss-EPI, single-shot echo-planar imaging; rs-EPI, readout-segmented echo planar imaging; Kapp, apparent diffusional kurtosis; Dapp, apparent diffusion for non-Gaussian distribution.
The diagnostic performance of the DKI parameters from the rs-EPI sequence is superior to those from the ss-EPI sequence
The results of the ROC curve analysis to distinguish the malignant and the benign breast lesions based on the histogram parameters are shown in Table 5 and Figure 4. The AUC values were higher for all the histogram parameters in the rs-EPI sequence compared with the ss-EPI sequence except for the skewness value of Dapp, but the differences were not statistically significant (all P>0.05). The AUC values were highest for the 75th percentile of Kapp (0.854 for rs-EPI, 0.844 for ss-EPI) and 25th percentile of Dapp (0.866 for rs-EPI, 0.858 for ss-EPI) among all the histogram parameters for both the DWI sequences.
Table 5
Variables | ss-EPI | rs-EPI | |||||
---|---|---|---|---|---|---|---|
Sensitivity (%) | Specificity (%) | AUC | Sensitivity (%) | Specificity (%) | AUC | ||
Histogram Kapp | |||||||
Mean | 76 | 77 | 0.822 | 94 | 68 | 0.850 | |
25th | 73 | 75 | 0.797 | 86 | 77 | 0.842 | |
50th | 68 | 88 | 0.829 | 93 | 72 | 0.853 | |
75th | 79 | 82 | 0.844 | 92 | 72 | 0.854 | |
100th | 79 | 72 | 0.786 | 87 | 68 | 0.812 | |
Skewness | 51 | 71 | 0.593 | 64 | 68 | 0.644 | |
Kurtosis | 58 | 54 | 0.543 | 54 | 72 | 0.611 | |
Histogram Dapp | |||||||
Mean | 74 | 82 | 0.835 | 87 | 69 | 0.850 | |
25th | 78 | 83 | 0.858 | 91 | 71 | 0.866 | |
50th | 93 | 62 | 0.839 | 79 | 80 | 0.855 | |
75th | 68 | 85 | 0.812 | 81 | 74 | 0.831 | |
100th | 56 | 74 | 0.678 | 66 | 80 | 0.751 | |
Skewness | 38 | 86 | 0.622 | 60 | 60 | 0.597 | |
Kurtosis | 40 | 68 | 0.518 | 69 | 46 | 0.561 |
ROC, receiver operating characteristic; ss-EPI, single-shot echo-planar imaging; rs-EPI, readout-segmented echo planar imaging; Kapp, apparent diffusional kurtosis; Dapp, apparent diffusion for non-Gaussian distribution; AUC, area under the curve.
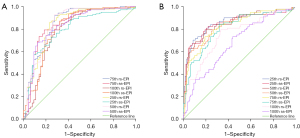
Discussion
The ICC values demonstrated good or excellent interclass agreement between the two readers, thereby confirming the reliability of our results. In this study, we performed histogram analysis of the DKI parameters (Dapp and Kapp) based on the ss-EPI and rs-EPI sequences for the benign and malignant breast lesions. The results demonstrated that most of the histogram parameters for the Dapp and Kapp values based on both the DWI sequences showed significant differences between the benign and the malignant breast lesions. Furthermore, the benign and malignant breast lesions showed significant differences between the ss-EPI and the rs-EPI sequence for the histogram parameters. ROC curve analysis demonstrated that the diagnostic accuracy of the histogram parameters for the Dapp and Kapp values based on the rs-EPI sequence was significantly higher compared with those from the ss-EPI sequence.
This is the first study to compare the histogram parameters between the ss-EPI and rs-EPI sequences for the breast lesions. The histogram parameters can accurately describe the intrinsic characteristics of the lesions and highlight small but important differences between the benign and malignant lesions without a requirement for additional imaging (29). Previous studies have reported histogram parameters of the breast lesions based on the ss-EPI sequence may provide better diagnostic performance for diagnosis (24,30). Furthermore, Kim et al. demonstrated that the image quality from the rs-EPI sequence was superior to that from the ss-EPI sequence (31). However, differences in the histogram parameters for the breast lesions between the two sequences have not been reported previously.
This study showed that the malignant and the benign breast lesions were clearly distinguishable based on the mean values and the 25th, 50th, 75th, and the 100th percentiles of Dapp and Kapp in both the DWI sequences. The Dapp values were lower, and the Kapp values were higher in the malignant breast lesions compared with the benign lesions. These results were consistent with the previously reported findings, which demonstrated that the malignant tumors were more complex and heterogeneous than the benign lesions (2,32). Therefore, the movement of the water molecules in the malignant tumors was more restricted than the water molecules in the benign lesions. The 75th percentile of Kapp and the 25th percentile of Dapp yielded the highest AUC values in their respective histogram parameter groups for differentiating the benign breast lesions from the malignant breast lesions. These results were consistent with a previously reported analysis of the DKI histogram parameters (24). The lower percentiles of Dapp coupled with the higher percentiles of Kapp are characteristic features of aggressive lesions with relatively dense malignant cells. Furthermore, the 100th percentile of Kapp did not show superior diagnostic accuracy because the higher values are adversely affected by noise and adjacent structures (30).
In our study, histogram parameters, including the mean, median, 25th, 50th, 75th, and 100th percentile of Dapp and Kapp for the benign lesions, were significantly different from those for the malignant lesions based on the image data from both DKI sequences. Furthermore, the results of ROC curve analyses demonstrated that the diagnostic accuracies of all the histogram parameters, based on the rs-EPI sequence, were higher than the corresponding histogram parameters based on the ss-EPI sequence. These results may be related to the differences in the fat suppression quality between the two DKI sequences. Fat suppression quality is highly dependent on factors such as magnetic field homogeneity, coil sensitivity, air-tissue susceptibility differences and partial-volume effects from the intravoxel fat signals in the breast diffusion-weighted images (33). The conventional single-shot EPI acquisitions are performed using a single excitation with a gradient echo readout trajectory (34). However, long EPI readout and corresponding low bandwidth per pixel in the phase-encoding direction can cause susceptibility artifacts at the air-tissue interface and magnetic field homogeneity, especially at higher field strengths such as 3 Tesla (3T), which may lead to errors in breast quantitative measurements with the ss-EPI sequence (21). The rs-EPI sequence acquires several readout segments of k-space in the readout direction during each shot and significantly reduces the echo spacing by accelerating the k-space traversal along the phase-encoding direction (35). These effects significantly reduce the magnetic susceptibility artifacts and improve the fat suppression quality, which can affect the performance of breast DWI imaging. Therefore, the histogram parameters from the rs-EPI sequence were more reliable than those from the ss-EPI sequences for the breast lesions.
Our study has several limitations. Firstly, the pathological types of benign and malignant lesions were unevenly distributed and may have resulted in sample selection bias. Secondly, all the parameters in this study were calculated by manually drawing the ROIs, which can introduce errors. Therefore, in future research, automated segmentation of the ROIs may be more accurate. Thirdly, the optimal b-value for the DKI model remains to be confirmed for the breast lesions. Finally, we did not compare the parameters from the DKI model with ADC value and we need to explore it in future.
In conclusion, the histogram analysis of the DKI parameters demonstrated that the rs-EPI sequence was more reliable and accurate in differentiating malignant breast from benign breast lesions.
Acknowledgments
Funding: None.
Footnote
Reporting Checklist: The authors have completed the STARD reporting checklist. Available at https://qims.amegroups.com/article/view/10.21037/qims-22-475/rc
Conflicts of Interest: All authors have completed the ICMJE uniform disclosure form (available at https://qims.amegroups.com/article/view/10.21037/qims-22-475/coif). TY and RG are employees of Siemens Healthcare. However, Siemens Healthcare has no role in the design of the study, data analyses, or data interpretation. The data of this study was analyzed and controlled by authors who are not the employees of the medical industry. The other authors have no conflicts of interest to disclose.
Ethical Statement: The authors are accountable for all aspects of the work in ensuring that questions related to the accuracy or integrity of any part of the work are appropriately investigated and resolved. The study was conducted in accordance with the Declaration of Helsinki (as revised in 2013). This educational review includes a statement on ethics approval and consent from the ethics committee of Tongji Hospital, Tongji Medical College, Huazhong University of Science. Written informed consent was received from each participant before participation in the study.
Open Access Statement: This is an Open Access article distributed in accordance with the Creative Commons Attribution-NonCommercial-NoDerivs 4.0 International License (CC BY-NC-ND 4.0), which permits the non-commercial replication and distribution of the article with the strict proviso that no changes or edits are made and the original work is properly cited (including links to both the formal publication through the relevant DOI and the license). See: https://creativecommons.org/licenses/by-nc-nd/4.0/.
References
- Kuhl CK, Jost P, Morakkabati N, Zivanovic O, Schild HH, Gieseke J. Contrast-enhanced MR imaging of the breast at 3.0 and 1.5 T in the same patients: initial experience. Radiology 2006;239:666-76. [Crossref] [PubMed]
- Hu Y, Zhan C, Yang Z, Zhang X, Zhang H, Liu W, Xia L, Ai T. Accelerating acquisition of readout-segmented echo planar imaging with a simultaneous multi-slice (SMS) technique for diagnosing breast lesions. Eur Radiol 2021;31:2667-76. [Crossref] [PubMed]
- Yabuuchi H, Matsuo Y, Sunami S, Kamitani T, Kawanami S, Setoguchi T, Sakai S, Hatakenaka M, Kubo M, Tokunaga E, Yamamoto H, Honda H. Detection of non-palpable breast cancer in asymptomatic women by using unenhanced diffusion-weighted and T2-weighted MR imaging: comparison with mammography and dynamic contrast-enhanced MR imaging. Eur Radiol 2011;21:11-7. [Crossref] [PubMed]
- Marini C, Iacconi C, Giannelli M, Cilotti A, Moretti M, Bartolozzi C. Quantitative diffusion-weighted MR imaging in the differential diagnosis of breast lesion. Eur Radiol 2007;17:2646-55. [Crossref] [PubMed]
- Martincich L, Deantoni V, Bertotto I, Redana S, Kubatzki F, Sarotto I, Rossi V, Liotti M, Ponzone R, Aglietta M, Regge D, Montemurro F. Correlations between diffusion-weighted imaging and breast cancer biomarkers. Eur Radiol 2012;22:1519-28. [Crossref] [PubMed]
- Fanariotis M, Vassiou K, Tsougos I, Fezoulidis I. Reproducibility of apparent diffusion coefficient measurements evaluated with different workstations. Clin Radiol 2018;73:141-8. [Crossref] [PubMed]
- Dijkstra H, Dorrius MD, Wielema M, Pijnappel RM, Oudkerk M, Sijens PE. Quantitative DWI implemented after DCE-MRI yields increased specificity for BI-RADS 3 and 4 breast lesions. J Magn Reson Imaging 2016;44:1642-9. [Crossref] [PubMed]
- Durando M, Gennaro L, Cho GY, Giri DD, Gnanasigamani MM, Patil S, Sutton EJ, Deasy JO, Morris EA, Thakur SB. Quantitative apparent diffusion coefficient measurement obtained by 3.0Tesla MRI as a potential noninvasive marker of tumor aggressiveness in breast cancer. Eur J Radiol 2016;85:1651-8. [Crossref] [PubMed]
- Christou A, Ghiatas A, Priovolos D, Veliou K, Bougias H. Accuracy of diffusion kurtosis imaging in characterization of breast lesions. Br J Radiol 2017;90:20160873. [Crossref] [PubMed]
- Sun K, Chen X, Chai W, Fei X, Fu C, Yan X, Zhan Y, Chen K, Shen K, Yan F. Breast Cancer: Diffusion Kurtosis MR Imaging-Diagnostic Accuracy and Correlation with Clinical-Pathologic Factors. Radiology 2015;277:46-55. [Crossref] [PubMed]
- Zhong Y, Li M, Zhu J, Zhang B, Liu M, Wang Z, Wang J, Zheng Y, Cheng L, Li X. A simplified scoring protocol to improve diagnostic accuracy with the breast imaging reporting and data system in breast magnetic resonance imaging. Quant Imaging Med Surg 2022;12:3860-72. [Crossref] [PubMed]
- Jensen JH, Helpern JA, Ramani A, Lu H, Kaczynski K. Diffusional kurtosis imaging: the quantification of non-gaussian water diffusion by means of magnetic resonance imaging. Magn Reson Med 2005;53:1432-40. [Crossref] [PubMed]
- Rosenkrantz AB, Sigmund EE, Johnson G, Babb JS, Mussi TC, Melamed J, Taneja SS, Lee VS, Jensen JH. Prostate cancer: feasibility and preliminary experience of a diffusional kurtosis model for detection and assessment of aggressiveness of peripheral zone cancer. Radiology 2012;264:126-35. [Crossref] [PubMed]
- Cho E, Baek HJ, Szczepankiewicz F, An HJ, Jung EJ, Lee HJ, Lee J, Gho SM. Clinical experience of tensor-valued diffusion encoding for microstructure imaging by diffusional variance decomposition in patients with breast cancer. Quant Imaging Med Surg 2022;12:2002-17. [Crossref] [PubMed]
- Raab P, Hattingen E, Franz K, Zanella FE, Lanfermann H. Cerebral gliomas: diffusional kurtosis imaging analysis of microstructural differences. Radiology 2010;254:876-81. [Crossref] [PubMed]
- Rosenkrantz AB, Sigmund EE, Winnick A, Niver BE, Spieler B, Morgan GR, Hajdu CH. Assessment of hepatocellular carcinoma using apparent diffusion coefficient and diffusion kurtosis indices: preliminary experience in fresh liver explants. Magn Reson Imaging 2012;30:1534-40. [Crossref] [PubMed]
- Tamura C, Shinmoto H, Soga S, Okamura T, Sato H, Okuaki T, Pang Y, Kosuda S, Kaji T. Diffusion kurtosis imaging study of prostate cancer: preliminary findings. J Magn Reson Imaging 2014;40:723-9. [Crossref] [PubMed]
- Nogueira L, Brandão S, Matos E, Nunes RG, Loureiro J, Ramos I, Ferreira HA. Application of the diffusion kurtosis model for the study of breast lesions. Eur Radiol 2014;24:1197-203. [Crossref] [PubMed]
- Kuroki Y, Nasu K, Kuroki S, Murakami K, Hayashi T, Sekiguchi R, Nawano S. Diffusion-weighted imaging of breast cancer with the sensitivity encoding technique: analysis of the apparent diffusion coefficient value. Magn Reson Med Sci 2004;3:79-85. [Crossref] [PubMed]
- Scheenen TW, Rosenkrantz AB, Haider MA, Fütterer JJ. Multiparametric Magnetic Resonance Imaging in Prostate Cancer Management: Current Status and Future Perspectives. Invest Radiol 2015;50:594-600. [Crossref] [PubMed]
- Porter DA, Heidemann RM. High resolution diffusion-weighted imaging using readout-segmented echo-planar imaging, parallel imaging and a two-dimensional navigator-based reacquisition. Magn Reson Med 2009;62:468-75. [Crossref] [PubMed]
- Klingebiel M, Ullrich T, Quentin M, Bonekamp D, Aissa J, Mally D, Arsov C, Albers P, Antoch G, Schimmöller L. Advanced diffusion weighted imaging of the prostate: Comparison of readout-segmented multi-shot, parallel-transmit and single-shot echo-planar imaging. Eur J Radiol 2020;130:109161. [Crossref] [PubMed]
- Li Z, Ai T, Hu Y, Yan X, Nickel MD, Xu X, Xia L. Application of whole-lesion histogram analysis of pharmacokinetic parameters in dynamic contrast-enhanced MRI of breast lesions with the CAIPIRINHA-Dixon-TWIST-VIBE technique. J Magn Reson Imaging 2018;47:91-6. [Crossref] [PubMed]
- Li T, Hong Y, Kong D, Li K. Histogram analysis of diffusion kurtosis imaging based on whole-volume images of breast lesions. J Magn Reson Imaging 2020;51:627-34. [Crossref] [PubMed]
- Bogner W, Pinker-Domenig K, Bickel H, Chmelik M, Weber M, Helbich TH, Trattnig S, Gruber S. Readout-segmented echo-planar imaging improves the diagnostic performance of diffusion-weighted MR breast examinations at 3.0 T. Radiology 2012;263:64-76. [Crossref] [PubMed]
- Wu J, Yan F, Chai W, Fu C, Yan X, Zhan Y, Sun K. Breast cancer recurrence risk prediction using whole-lesion histogram analysis with diffusion kurtosis imaging. Clin Radiol 2020;75:239.e1-8. [Crossref] [PubMed]
- Barnhart HX, Haber MJ, Lin LI. An overview on assessing agreement with continuous measurements. J Biopharm Stat 2007;17:529-69. [Crossref] [PubMed]
- Hallgren KA. Computing Inter-Rater Reliability for Observational Data: An Overview and Tutorial. Tutor Quant Methods Psychol 2012;8:23-34. [Crossref] [PubMed]
- Suo S, Zhang K, Cao M, Suo X, Hua J, Geng X, Chen J, Zhuang Z, Ji X, Lu Q, Wang H, Xu J. Characterization of breast masses as benign or malignant at 3.0T MRI with whole-lesion histogram analysis of the apparent diffusion coefficient. J Magn Reson Imaging 2016;43:894-902. [Crossref] [PubMed]
- Liu W, Wei C, Bai J, Gao X, Zhou L. Histogram analysis of diffusion kurtosis imaging in the differentiation of malignant from benign breast lesions. Eur J Radiol 2019;117:156-63. [Crossref] [PubMed]
- Kim YJ, Kim SH, Kang BJ, Park CS, Kim HS, Son YH, Porter DA, Song BJ. Readout-segmented echo-planar imaging in diffusion-weighted mr imaging in breast cancer: comparison with single-shot echo-planar imaging in image quality. Korean J Radiol 2014;15:403-10. [Crossref] [PubMed]
- Bogner W, Gruber S, Pinker K, Grabner G, Stadlbauer A, Weber M, Moser E, Helbich TH, Trattnig S. Diffusion-weighted MR for differentiation of breast lesions at 3.0 T: how does selection of diffusion protocols affect diagnosis? Radiology 2009;253:341-51. [Crossref] [PubMed]
- Baron P, Dorrius MD, Kappert P, Oudkerk M, Sijens PE. Diffusion-weighted imaging of normal fibroglandular breast tissue: influence of microperfusion and fat suppression technique on the apparent diffusion coefficient. NMR Biomed 2010;23:399-405. [Crossref] [PubMed]
- Partridge SC, Nissan N, Rahbar H, Kitsch AE, Sigmund EE. Diffusion-weighted breast MRI: Clinical applications and emerging techniques. J Magn Reson Imaging 2017;45:337-55. [Crossref] [PubMed]
- Zhang H, Huang H, Zhang Y, Tu Z, Xiao Z, Chen J, Cao D. Diffusion-Weighted MRI to Assess Sacroiliitis: Improved Image Quality and Diagnostic Performance of Readout-Segmented Echo-Planar Imaging (EPI) Over Conventional Single-Shot EPI. AJR Am J Roentgenol 2021;217:450-9. [Crossref] [PubMed]