The emerging potential of quantitative MRI biomarkers for the early prediction of brain metastasis response after stereotactic radiosurgery: a scoping review
Introduction
Brain metastasis (BM), which occurs frequently in non-small cell lung cancer (NSCLC), breast cancer, and melanoma (1,2), is associated with poor survival and presents distinct clinical problems. At present, multiple treatments are available, including neurosurgical resection, radiotherapy, chemotherapy, and immunotherapy (1,3), making it difficult to formulate a patient-specific treatment plan. Owing to the anatomical location of BMs and the blood-brain barrier (4), radiotherapy has become a particularly promising treatment method for BM. Stereotactic radiosurgery (SRS), as opposed to whole brain radiotherapy (WBRT), is recommended by the American Society of Radiation Oncology (5) and the International Stereotactic Radiosurgery Society consensus guidelines (6) due to the absence of compromise in survival outcomes as well as the absence of a significant increase in neurocognitive toxicities (1,3,7).
Simple prognostic models based on clinical information have been developed to help predict the prognosis of patients with BM, including the recursive partitioning analysis (8) scale and graded prognostic assessment (GPA) (9) score. Moreover, in light of the increasing awareness of the influence of primary tumor type and molecular alterations on patient outcomes, disease-specific GPAs (DS-GPA) (10) and/or relevant molecular updates (11-14), if available, are presently used in both clinical practice and trial design. Nevertheless, few studies have added advanced quantitative imaging biomarkers in an attempt to improve these models.
Neuroimaging’s role in the diagnosis, treatment planning, and posttherapy assessment of brain tumors is continually evolving. Magnetic resonance imaging (MRI) uses a strong magnetic field to provide high-resolution anatomical information that allows for the easy identification of blood vessels, masses, and adjacent soft tissues (15). Furthermore, advanced MRI sequences are capable of further characterizing tumor biology by providing quantitative functional parameters that are known to have biological significance, such as tissue cellularity, vascular perfusion or permeability, and hypoxia (16). Most studies (17-23) tend to use cerebral MRI to clarify aspects of tumor diagnosis, including true progression, false progression, edema zone, tumor hemorrhage, etc., while very few studies have attempted (with limited success) to evaluate the treatment response using quantitative MRI within a few days after SRS.
In this article, we review the literature on the potential of early quantitative MRI biomarkers to indicate the local control or failure of patients with BMs undergoing SRS, and discuss their potential utilities and limitations as imaging biomarkers to guide treatment individualization for patients with BMs. We believe that there is a promising future for the clinical application of quantitative imaging biomarkers. We present the following articles in accordance with the PRISMA-ScR reporting checklist (available at https://qims.amegroups.com/article/view/10.21037/qims-22-412/rc).
Methods
Search strategy and selection criteria
This review was not registered, and the protocol was not prepared. We selected relevant studies published between February 1, 1991, and April 11, 2022, by searching the PubMed, Embase, Cochrane, Web of Science, and Clinical Trials.gov databases without language restrictions. We used the following medical subject heading (Medical Subject Headings, MeSH) terms: “Brain Metastases”, “Radiosurgery”, “Imaging, Magnetic Resonance”, and “Outcome, Treatment” or “Prognoses”. The complete advanced search method used for PubMed is detailed in Appendix 1. All potentially eligible studies were considered for our review, regardless of the primary outcome or the language. We also performed a manual search using the English reference lists of the main articles.
Study selection and data extraction
A study was considered to be eligible if it was performed on patients with BMs who had received brain SRS alone or who received SRS combined with chemotherapy or immunotherapy. The patients included in the study were also required to have definitive pathological confirmation of the primary tumor. Furthermore, all of the included patients were followed up with regular brain MRI examinations before and after SRS. The exclusion criteria were as follows: (I) not treatise studies, (II) studies with fewer than 5 participants, (III) studies incorporating patients who had undergone prior surgery, and (IV) studies without reliable statistics.
The studies involving quantitative MRI (qMRI) biomarkers as early prognostic indicators for patients with BMs undergoing SRS were classified into 4 groups according to the treatment-related changes after radiotherapy. The outcomes were generalized according to the following aspects: (I) the anatomical/morphological changes in BMs following radiosurgery, (II) the relationship between the microstructural changes and SRS, (III) the relationship between the vascular changes and SRS, and (IV) the metabolic changes in BMs following radiosurgery.
Two investigators (J Hu and X Hu) independently reviewed study titles and abstracts and identified the studies meeting the inclusion criteria for full-text assessment. In cases of disagreement between these 2 investigators, another senior physician (X Xie) was asked for her viewpoint. Trials selected for detailed analysis were examined ulteriorly by 2 investigators (J Hu and X Hu) independently. We extracted the following data from each selected study: total number of participants, age, primary tumor pathology, radiation dose, time of radiological follow-up, magnetic field strength, MRI sequences, the range, of accessed tissue, and the quantitative biomarkers for predicting SRS response of BMs. Subsequently, X Xie confirmed the main findings of each selected study.
Results
We identified 2162 studies, of which 26 (published between 2003 and 2022) were included in our analysis (Figure 1). Given the thousands of qMRI parameters being extracted from different sequences, it is difficult to select the optimal statistics of biomarkers for the early prediction of local outcomes in metastatic brain tumors after stereotactic radiotherapy. We aimed to review the current state of research into the predictive imaging biomarkers for delineating the treatment response of SRS-treated BMs using traditional and advanced MRI techniques and to summarize the findings inferred from MRI results regarding the underlying biological changes. Table 1 provides an overview of the accessible publications regarding qMRI parameters predicting the prognosis of BMs after SRS.
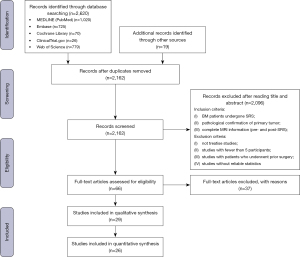
Table 1
Reference | Number of patients | Patient age (years) | Primary tumor | Radiation dose | Timing of radiological follow-up | Magnetic field strength (T) | MRI sequence | Tissue assessed | Main finding/alteration |
---|---|---|---|---|---|---|---|---|---|
Nardone et al., 2016 | 38 | 52–87 | NSCLC | SRS: 14–23 Gy | At 4 weeks, 12–16 weeks, then every 6 months | – | T1-, T2-, FLAIR- | ROI of BM | Entropy (P=0.013), uniformity (P=0.013), and kurtosis (P=0.046) were associated with local progression, and kurtosis (P=0.023) associated with a new BM (diagnostic MRI) |
SRT: 18–24 Gy | |||||||||
Tini et al., 2017 | 42 | 51–87 | NSCLC | 10–30 Gy | At 4 weeks, 12–16 weeks | – | T1w, T2w | ROI of BM structures and PE | BM with perilesional edema at diagnosis had a worse response to SRS (in-field recurrence: P=0.029; out-field recurrence: P=0.025) (diagnostic MRI) |
Karami et al., 2019 | 100 | 21–92 | NSCLC, MM, BC, RCC, CRC, other | SRT: 25–35 Gy in 5 F | Every 2–3 months post-SRT | 1.5 | T1w, T2 FLAIR | ROI of tumor and edema, tumor margin, and lesion-margin region | 13 features on pretreatment MRI were correlated with the overall (5/13), 6-months (4/13), and 12-months (4/13) local outcome, respectively (AUC.632+ =0.79, 0.80, and 0.80 respectively) (pretreatment MRI) |
Nardone et al., 2019 | 46 | 46–87 | NSCLC | 16–30 Gy | At 6 weeks, 12–16 weeks | – | T1, T2 FLAIR | ROI of GTV and PE | (I) Lower PE/GTV ratio: development of a new BM (HR =0.302) |
(II) Higher TV: worse OS (HR =1.038) | |||||||||
(Diagnostic MRI) | |||||||||
Mouraviev et al., 2020 | 87 | 34–88 | LC, BC, MM, other | 14–25 Gy | Every 2–3 months post-SRS | 1.5 | T1c, T2 FLAIR | ROI of tumor core and peritumoral region | Top 10 pretreatment radiomics features plus clinical variables help predict LF (AUC mean =0.793) (baseline MRI) |
Kawahara et al., 2020 | 54 | 32–86 | MM | 15 Gy for < 4.2 cc, 18 Gy for ≥4.2 cc to ≤14.1 cc, 24 Gy for >14.1cc | Every 3 months post-SRS | 1.5 | T1w | ROI of GTV | A NN model constructed by selected 7 radiomics features from SRS planning MRI was predictive of treatment outcome (AUC =0.87). (The radiomics process follows the IBSI) (planning MRI) |
Jaberipour et al., 2021 | 120 | 21–92 | LC, BC, MM, CRC, RCC, other | SRT: 22.5–35 Gy in 5 F | Every 2–3 months post-SRT | 1.5 | CE-T1w, T2-FLAIR | ROI of tumor and peritumoral region | An optimal qMRI biomarker consisting of 4 features at pretreatment for predicting LC/LF (AUC =0.87) (pretreatment MRI) |
Park et al., 2021 | 83 | 25–84 | LC | 12–24 Gy | Within 6 months post-SRS | 1.5 | CE-T1w, T2w | ROI of tumor | Higher RLN was predictive of poor local control; Higher SRE was associated with better low risk of local tumor progression. (Harrell’s concordance index =0.79) (pretreatment MRI) |
Gutsche et al., 2021 | 150 | 25–84 | NSCLC, SCLC, BC, MM, RCC, GC, other, | 18–20 Gy | Within 180 d post-SRS | 1.5 or 3.0 | CE-T1w | PTV | The radiomics model comprised 10 features and allowed for the prediction of early SRS response: probability >67% as a responder (AUC =0.74). (pretreatment MRI) |
Wang et al., 2021 | 28 | 28–85 | LC, BC, MM, EC | 16–36 Gy | Every 3 months post-SRS | 1.5 or 3.0 | T1w | ROI of tumor without margins | 10 radiomics features (1 shape feature, 6 MR images, and 3 dose distribution features) for the early prediction of tumor LF after SRS (AUC =0.78) (planning MRI) |
Zheng et al., 2021 | 44 | 33–77 | BC | 15–20 Gy | Every 3 months after GKRS | 1.5 | CE-T1w | BM | CE-T1W-based kurtosis combined with age predicted the PFS after GKS therapy (confidence interval =0.70) (pre-treatment MRI) |
Jiang et al., 2022 | 137 | 55.2±12.7 | LC | 15–20 Gy | 3 months after GKRS | 3.0 | T2w, T1w, T2-FLAIR, ADC, CBV | ROI of tumor core and peritumoral edema | Top 10 multimodality radiomics features plus gender and histological subtype predicted the response of LCBM to GKRS (AUC =0.930, in primary cohort; AUC =0.852, in validation cohort) (pretreatment MRI) |
Jakubovic et al., 2016 | 42 | 45–64 | LC, BC, MM, RCC, other | SRS: 15–24 Gy; hypofractionated SRS: 35 Gy in 5 F; WBRT: 20 Gy in 5 F or 30 Gy in 10 F | At 1 week and 1 month post-radiation | 1.5 | DWI | ROI of tumor and the contralateral unaffected white matter | Lower relative ADC at 1 week (OR =0.619) and 1 month (OR =0.694) after radiation distinguished responders from nonresponders (MRI at 1 week and 1 month post-RT) |
Chen et al., 2017 | 41 | 61.2±6.5 | LC, BC, RCC, head and neck cancer, sarcoma | 16–26 Gy | 1 month and 6 months post-SRS | 3.0 | DWI | Tumor-enhanced regions | A lower DI at baseline and 1 month distinguished responders from nonresponders (P=0.002 and P=0.001, respectively) (MRI at baseline and 1 month post-SRS) |
Essig et al., 2003 | 18 | 29–69 | MM, RCC, bronchial cancer, OC, PC, CC, BC | 15–20 Gy | 6 weeks and 3 months post-RT | 1.5 | DSC | BM, normal WM | rCBV ↓ at the 6-week follow-up was predictive of treatment outcome (sensitivity =91%, specificity =71%) (MRI at 6 weeks post-RT) |
Weber et al., 2004 | 25 | 25–73 | Bronchial cancer, BC, MM, RCC, CRC, testicular cancer, GC, bladder carcinoma | 16–20 Gy | at 6, 12, 24 weeks post-SRS | 1.5 | DSC, ASL (Q2TIPS) | ROI on the BM and contralateral hemisphere | rrCBF ratio of Met/GM: ↓ at the 6-week follow-up was predictive of tumor response (MRI at 6 weeks post-SRS) |
Almeida-Freitas et al., 2014 | 26 | 24–73 | NSCLC, MM, BC, RCC, non–seminomatous germ cell cancer | 12–25 Gy | 4–8 weeks post-SRS | 1.5 | DCE | ROI of the tumor | (I) Ktrans: ↓4–8 weeks after SRS |
(II) An early increase of 15% of Ktrans shows an increased risk of tumor progression (HR =1.5) | |||||||||
(MRI at 4–8 weeks post-SRS) | |||||||||
Jakubovic et al., 2014 | 44 | 48.5–66 | BC, LC, MM, RCC, uterine cancer, germ cell cancer | SRS: 15–35 Gy; WBRT: 20 or 30 Gy | At 1 week and 1 month post-radiation | 1.5 | DCE, DSC | ROI around the tumor and contralateral mirror region | (I) K2trans: ↓ at 1 week post-RT was predictive of responders (OR =1.949) (MRI at 1 week after radiation) |
(II) rCBV: ↓ at 1 month post-RT was predictive of progression (OR =0.522) (MRI at 1 month after radiation) | |||||||||
Taunk et al., 2017, 2018 | 41 | 36–71 | NSCLC | 18–22 Gy | Within 12 weeks post SRS | 1.5 or 3.0 | DCE | ROI of tumor and cystic/necrotic changes | (I) Ktrans SD (cutoff value of 0.017) predicted response to SRS (AUC =0.73); (MRI within 12 weeks post-SRS) |
(II) ve predicted KRAS mutant status | |||||||||
Huang et al., 2020 | 16 | 33–73 | NSCLC, OC, EC, CRC, tonsillar cancer, thyroid cancer | 17–21 Gy | At 1 week and 12 weeks after SRS | 3.0-T at week 1, 1.5-T at baseline and week 12 | PWI | ROI of BM and contralateral WM | Higher rCBV at 1 week after SRS exhibited a borderline association with local recurrence (HR =1.07) (MRI at 1 week post-SRS) |
Swinburne et al., 2020 | 41 | 36–71 | NSCLC | 18–22 Gy | Within 12 weeks | 1.5 or 3.0 | DCE | ROI of treated lesions | Lower ΔIFP kurtosis (AUC =0.71) and mean (AUC =0.72) after SRS within 12 weeks was predictive of objective response (MRI within 12 weeks post-SRS) |
Shah et al., 2021 | 16 | 35–85 | LC, MM, BC, other | – | At 72 h post-SRS | 3.0 | DWI, DCE | ROI of tumor | (I) Higher f value at 72 h after SRS demonstrated progression (MRI at 72 h post-SRS) (q=0.041) |
(II) Higher ve and Ktrans in nonresponders (pre-SRS MRI) (q=0.041) | |||||||||
Desmond et al., 2017 | 25 | 48–76 | LC, BC, MM, RCC | 18–20 Gy | At 1 week and 1 month post-SRS | 3.0 | CEST | ROI of tumor and the contralateral NAWM | NOE peak amplitude in NAWM (R =0.69) and width in tumor (R =−0.55) at 1 week post-SRS was associated with tumor volume changes 1 m after SRS (MRI at 1 week post-SRS) |
Jia et al., 2020 | 68 | 48–71 | NSCLC | SRT: 48–60 Gy in 6–8 fractions | (I) Every 3 months within first 3 years | 3.0 | T1w, T2w, DCE, 1H-MRS | ROI of tumor | Cho/Cr value: >1.46 implies worse survival (OS, HR =2.956; PFS, HR =3.925) (diagnostic MRI) |
(II) Every 6 months during the following year | |||||||||
(III) Annually thereafter post-SRS | |||||||||
Lee et al., 2021 | 11 | – | NSCLC, RCC, BC, CRC | – | At pretreatment, 6 months after SRS, or prior to death | 3.0 | Hyperpolarized 13C MRI | ROI of BM | The tumor 13C-lactate signals pre-SRS were predictive of progression of BMs at 6 months post-SRS (AUC =0.77) (pre-SRS MRI) |
Mehrabian et al., 2017 | 19 | 38–85 | LC, BC, endometrial cancer, thyroid cancer, rectal cancer | 18–20 Gy | At 1 week and 1 month post-SRS | 3.0 | DCE | ROI of tumor | kIE: ↑ at 1 week post-SRS was predictive of tumor shrinkage at 1 month and long-term response (R =−0.76) (MRI at 1 week post-SRS) |
qMRI, quantitative magnetic resonance imaging; SRS, stereotactic radiosurgery; BM, brain metastasis; T, Tesla; NSCLC, non-small cell lung cancer; SRT, stereotactic radiotherapy; ROI, region of interest; FLAIR, fluid attenuated inversion recovery; LC, lung cancer; MM, melanoma; BC, breast cancer; RCC, renal cell cancer; CRC, colorectal cancer; GC, gastrointestinal cancer; EC, esophagus cancer; OC, ovarian carcinoma; PC, pancreatic carcinoma; CC, cervical carcinoma; GKRS, gamma knife radiosurgery; WBRT, whole brain radiation therapy; RT, radiation therapy; LC, local control; LF, local failure; NN, neutral network; PTV, plan tumor volume; CE, contrast enhancement; NAWM, normal-appearing white matter; T1w, T1-weighted; T2w, T2-weighted; PE, perilesional edema; DWI, diffusion-weighted imaging; ADC, apparent diffusion coefficient; DI, diffusion index; PWI, perfusion-weighted imaging; DCE, dynamic contrast enhancement; DSC, dynamic susceptibility contrast; ASL, arterial spin labeling; 1H, proton; Met/GM, metastasis/gray matter; RLN, run-length nonuniformity; SRE, short-run emphasis; ve, volume of extravascular extracellular space; CBF, cerebral blood flow; CBV, cerebral blood volume; Ktrans, volume transfer constant between blood plasma and extravascular extracellular space; kIE, intraextracellular water exchange rate constant; IFP, interstitial fluid pressure; IFV, interstitial fluid velocity; f, fraction; NOE, nuclear Overhauser effect; Cho, choline; Cr, creatine; HR, hazard ratio; OR, odds ratio; R, correlation; IBSI, image biomarker standardization initiative; OS, overall survival; PFS, progression-free survival; ↓, decrease; ↑, increase. cc., cubic centimeter; F, fraction.
The relationship between anatomical/morphological changes and SRS
It is acknowledged that the traditional cranial MRI techniques, including T1-weighted (T1w), T2-weighted (T2w), and contrast-enhanced images, can provide legible anatomical or morphological information (15), and these are consequently implemented clinically as the standard modality for the diagnosis and follow-up of BM. Researchers previously focused on the alteration of tumor volume (TV) and heterogeneity, as well as the perilesional edema (PE) in structural MRI, despite the lack of quantitative measurements to assess the change in metastatic brain tumors. With the development of imaging and analysis technologies, qMRI biomarkers for predicting treatment response have gradually been acquired.
Several studies (24,25) focused on the quantification of the peritumoral region before irradiation, while others (26-35) developed optimal quantitative prognostic models (with or without clinical data) by examining multiple geometrical and textural features of MR images pre-SRS with different sophisticated radiomics analysis frameworks to predict early treatment response. However, since the algorithms for image quantification were not standardized, the robustness and reproducibility of the relevant results were poor. In 2020, an image biomarker standardization initiative (IBSI) was proposed to standardize radiomics features. Generally speaking, it aims to standardize the extraction of biomarkers from acquired imaging for high-throughput quantitative image analysis (radiomics). The current consensus is that the results based on this guideline are considered more reliable (36).
Quantification of peritumoral region
Tini et al. (25) divided the maximal extent of peritumoral edema in 42 patients with BM from NSCLC into minor (<10 mm) and major (≥10 mm) according to the classification proposed by Schoenegger for glioblastoma (37). They reported that minor edema was associated with a better response to SRS treatment (range, 10–30 Gy) and a reduced risk of developing new brain lesions. In a monocentric retrospective study, Nardone et al. (24) analyzed 46 patients with 1–2 BMs treated with SRS (range, 16–30 Gy) and suggested that patients with BMs with a lower PE/gross tumor volume (GTV) ratio (hazard ratio, HR 0.302) at diagnosis are more prone to developing new brain lesions. Additionally, patients with higher PE, GTV, and TV showed worse overall survival (OS).
Quantitative prognostic models without clinical data
Some researchers have investigated qMRI biomarkers through a radiomics analysis framework constructed using various geometrical and textural features extracted from T1w and T2w images within the tumor and edema regions to predict the treatment outcome in patients with BM treated with radiotherapy. Nardone et al. (26) evaluated the prognostic value of MRI texture analysis parameters (mean, standard deviation, skewness, kurtosis, entropy, and uniformity) of 38 patients with NSCLC with oligo-metastases treated with SRS (range, 14–23 Gy) and stereotactic radiation therapy (SRT; range, 18–24 Gy, in 3–5 fractions). They found that there was a significant correlation between entropy, uniformity, and local progression, while kurtosis was associated both with local progression and new BMs. Another study by Park et al. (27) detected multiple textural features extracted from pretreatment MRI scans in 279 BM patients treated with SRS with a marginal dose ranging from 12 to 24 Gy. The authors speculated that 2 independent textural features, run-length nonuniformity and short-run emphasis values, may provide valuable information regarding the underlying tumor heterogeneity, radiosensitivity, and/or vascularization, which could, in turn, be related to the SRS treatment response. Karami et al. (29) found that the optimal qMRI biomarkers consisted of 5 features for overall local control or failure (LC/LF) outcomes and 4 features for the 6-month and 12-month outcomes through a multistep feature reduction and selection method in 100 patients with BM treated with hypofractionated SRT (range, 25–35 Gy, in 5 fractions). The selected 13 features on pretreatment MRI mainly characterize the heterogeneity in the surrounding regions of the tumor, including edema, tumor margin, and lesion margin. Similarly, Gutsche et al. (28) retrospectively analyzed the pretreatment T1w MR images of 150 patients with BMs treated with SRS (range, 17–20 Gy). They constructed an optimal radiomics model comprising 10 features that allowed for prediction of the early response to SRS that outperformed the visual assessment of contrast enhancement patterns.
Quantitative prognostic models with clinical data
As the IBSI guidelines were proposed in 2020, it is worth mentioning a study from Kawahara et al. (30). The authors investigated the radiomics features extracted from the radiotherapy planning MRI scans of 54 patients with melanoma BM treated with gamma knife radiosurgery (GKRS) at a dose of 24 Gy for TV <4.2 cc (cubic centimeters), 18 Gy for TV ≥4.2 cc to ≤14.1 cc, and 15 Gy for TV >14.1 cc. They proposed a promising neural network (NN) model using the 7 selected radiomics features of the tumor image from the planning MRI for predicting the local response of BMs to GKRS, with an accuracy of 0.87. This study emphasized the importance of the IBSI guidelines and precontrast T1 MR radiomics for predicting the outcome of GKRS.
Moreover, several studies have succeeded in incorporating radiomics features with clinical and dosimetric features to improve the local response prediction of SRS-treated BMs. Mouraviev et al. (31) extracted a total of 440 radiomics features from the tumor core and peritumoral regions using the baseline standard volumetric postcontrast T1 (T1c) and volumetric T2 fluid-attenuated inversion recovery MRI sequences in a cohort of 87 mixed-histology BM patients treated with SRS (range, 14–25 Gy). They found that the addition of the top 10 radiomics features provided additional information regarding the standard routinely available clinical variables for predicting LF in BM following SRS. Similarly, Jaberipour et al. (32) developed a predictive model using the pretreatment qMRI and clinical features of 100 patients, which was evaluated using an independent test set with data from 20 patients. All of the patients with BMs underwent SRT with a total dose of 22.5–35 Gy over 5 fractions. The authors demonstrated that the incorporation of a qMRI biomarker, consisting of 4 features with 2 heterogenous features in the edema area, 1 characterizing intratumor heterogeneity and the other describing tumor morphology, could improve the overall performance of predicting LC/LF by up to 16% of the area under the curve (AUC).
Zheng et al. (35) reported that pretreatment T1c-based kurtosis combined with age provided better performance for survival prediction in 81 patients with breast cancer BMs undergoing GKRS (range, 15–20 Gy). Moreover, Wang et al. (34) retrospectively reviewed a subset of 28 patients who received single-fraction SRS with doses ranging from 15 to 25 Gy. They stated that 10 radiomics features (including 1 shape feature, 6 MR images, and 3 dose distribution features) from planning MRIs and dose maps showed promise for the early prediction of tumor LF in SRS. Jiang et al. (33) retrospectively analyzed 137 patients with lung cancer BMs (LCBMs) who received GKRS (range, 15–20 Gy). The authors extracted valuable radiomics features of the tumor core and peritumoral edema from pretreatment multimodality MRI images using random forests. They finally developed a radiomics approach that integrates the top 10 multimodality MRI-based radiomics features and clinical factors (gender and histological subtype) to predict the posttreatment response of LCBM to GKRS.
The relationship between microstructural changes and SRS
A few studies have assessed the radiation-induced microstructure changes of BM with diffusion imaging and succeeded in identifying the optimal quantitative diffusion MRI parameters to predict the radiobiological response (15). A retrospective investigation by Chen et al. (38) accessed a diffusion index (DI) generated from the apparent diffusion coefficient (ADC) and tumor volume at baseline and at 1 and 6 months post-SRS (range, 14–18 Gy) in a mixed-histology cohort of 41 patients with BM. They proved a lower DI at baseline and at 1 month could distinguish responders from nonresponders. In a pilot study, Jakubovic et al. (39) demonstrated that lower relative ADC values could distinguish between radiation responders and nonresponders as early as 1 week and 1 month posttherapy (SRS, SRT, or WBRT) in 42 patients with histologically diverse BMs. Additionally, Shah et al. (40) prospectively studied a mixed-histology cohort of 16 patients with BM who received pre-SRS MRI and early (within 72 h) post-SRS MRI, including diffusion-weighted imaging (DWI) and dynamic contrast enhancement (DCE), and analyzed the DWIs using the monoexponential and intravoxel motion model. They confirmed that higher perfusion fraction (f) values derived from DWI early post-SRS were predictive of responders (in terms of stable disease, partial regression, and complete regression). Consequently, a lower ADC (at 1 week and 1 month postradiation therapy) and DI (pre-SRS, 1 month post-SRS) indicate responders, while higher f values (72 h post-SRS) indicate nonresponders.
The relationship between vascular changes and SRS
Radiation-induced vascular changes can be detected with perfusion-weighted imaging (PWI) methods such as dynamic susceptibility contrast (DSC), DCE, and arterial spin labeling (ASL) (15).
Researchers have investigated the prognostic value of quantitative perfusion MRI biomarkers for SRS response in patients with BMs and consequently observed that a reduction of relative cerebral blood volume (rCBV) (41), relative regional cerebral blood flow (rrCBF) (42), quadratic of time-dependent leakage (Ktrans2) (43), and interstitial fluid pressure (IFP) (44) is associated with tumor response after SRS, while an increase of Ktrans (40,45,46) and extracellular extravascular volume (ve) (40) is correlated with long-term progressive disease.
DCE
Using DCE, Almeida-Freitas et al. (45) prospectively observed a significant reduction in the Ktrans values of 34 cerebral metastases from a mixed-histology cohort of 26 patients with BM 4–8 weeks after SRS. The researchers reported that an early increase of 15% in Ktrans after SRS (range, 12–25 Gy) was associated with an increased risk of tumor progression at the midterm MRI follow-up (mean 7.9±4.7 months). Jakubovic et al. (43) prospectively investigated the predictive capacity of early changes in rCBV, relative cerebral blood flow, and Ktrans2 from DSC, and DCE MRI in 70 histologically diverse BMs of 44 patients who received either SRS or WBRT. The authors found that early Ktrans2 reduction at 1 week posttreatment significantly differentiated responders from nonresponders, whereas a lower rCBV at 1 month could distinguish disease progression from nonprogression. Taunk et al. (46) retrospectively calculated the Ktrans, blood plasma volume, and ve for 53 NSCLC BMs treated with SRS (range, 18–21 Gy) from 41 patients. They demonstrated that a post-SRS Ktrans standard deviation cutoff value of 0.017 within 12 weeks was highly sensitive (89%) for predicting long-term progressive disease (PD) and non-PD. Additionally, Shah et al. (40) reported that higher ve and Ktrans values derived from DCE MRI pre-SRS in a mixed-histology cohort of 16 BM patients were associated with nonresponse. Another retrospective study by Swinburne et al. (44) of 43 lung cancer BMs subjected to SRS (range, 18–22 Gy) examined the correlation between long-term local tumor control and early (within 12 weeks) intratumoral changes in IFP and interstitial fluid velocity estimated from computational fluid modeling using DCE MRI. They demonstrated that lower post-SRS tumor heterogeneity represented by a reduction of IFP skewness and kurtosis was associated with the objective response.
DSC
Using DSC, Essig et al. (41) observed a decrease in the region CBV value of 18 patients with BM after single high-dose SRT (range, 15–20 Gy) at the 6-week follow-up that was highly sensitive for treatment outcome prediction. Similarly, Weber et al. (42) reported a decrease in the rrCBF value determined by DSC and ASL perfusion MRI at the 6-week follow-up in 25 patients with BM post-SRS (range, 16–20 Gy), which was capable of correctly predicting the tumor response. Additionally, in a pilot study by Huang et al. (47) that enrolled 16 patients with BM treated with SRS (range, 17–21Gy) who received PWI 1 week pre- and posttreatment, the authors found that a higher rCBV at 1 week 1 had a borderline association with shorter time to local recurrence.
Contrary to the findings of Essig et al. (41) and Huang et al. (47), the results of Jakubovic et al. (43) suggested that a lower rCBV at 1 month post-SRS could predict disease progression. The apparent discrepancy between these studies is probably explained by the different times at which rCBV was measured, given the fact that vascular changes after radiation treatment have shown to be highly time-dependent (48). In summary, lower rrCBF (6 weeks post-SRS) and Ktrans2 (1 week post-RT) portend tumor response, while higher Ktrans (pre-SRS, 4–8 weeks post-SRS) and ve (pre-SRS) are more likely to be indicative of nonresponse.
The relationship between metabolic changes and SRS
The development of advanced MRI techniques such as magnetic resonance spectroscopy (MRS) and chemical exchange saturation transfer (CEST) has provided an opportunity for researchers to investigate the metabolic and microenvironment changes of BMs after SRS on a more micro scale, and subsequently identify their value for predicting long-term treatment response.
MRS
MRS allows for the noninvasive detection of radiation-induced metabolic changes in the brain. The metabolites N-acetyl aspartate (a marker for neuronal viability), choline (Cho; a marker of cell membrane turnover), and creatine (Cr; a bioenergetic metabolite) have primarily been evaluated. Some studies have also investigated changes in lactate (Lac) as a marker for anaerobic metabolism and changes in lipids (Lip) as a marker for cell membrane disintegration. Both the absolute metabolite values and ratios between the metabolites have been considered (15,49-52).
In a retrospective analysis, Jia et al. (53) investigated the qualitative and quantitative parameters of baseline MRS metabolites to predict the tumor response after SRT in a cohort of 68 patients with NSCLC with BM (range, 48–60 Gy in 6–8 fractions). This study indicated that patients with elevated Cho/Cr values (Cho/Cr >1.46) exhibited a poorer prognosis than did those with Cho/Cr ≤1.46 [OS: P=0.002; progression-free survival (PFS): P=0.001]. Lee et al. (54) retrospectively assessed the potential of hyperpolarized 13C MRI to predict radiation treatment failure by probing Lac metabolism in vivo in 11 patients with intracranial metastases. They found that the positive predictive value of the 13C-lactate signal measured pre-SRS for the prediction of intracranial metastasis progression at 6 months post-SRS was 0.8 (P<0.05), and the AUC was 0.77 (P<0.05).
Chemical-water exchange
CEST is a new MRI technique that is sensitive to the exchange of proton pools with bulk water protons, forming an MRI image that may provide additional information as a tumor response biomarker (55-57). Endogenous CEST experimentation is sensitive to several chemical groups, including the labile protons in proteins, metabolites, and larger macromolecules (58). In a prospective study, Desmond et al. (59) compared the pre-SRS and 1-week post-SRS CEST metrics with the changes in tumor volume at 1 month in a mixed-histology cohort of 25 patients with BM who had received a single dose of SRS at 18 to 20 Gy. The authors reported a positive association between the changes in the nuclear Overhauser effect (NOE) peak amplitude in NAWM (normal-appearing white matter, both ipsi- and contralateral) at 1 week and the volume changes at 1 month. Additionally, they observed a negative correlation between the absolute change in width of the NOE peak between 1 week and the volume changes at 1 month.
Several techniques have been applied to measure the water exchange rate constant between intracellular and extracellular compartments (60-63). In a pilot study, Mehrabian et al. (64) included 19 patients with histologically diverse metastatic brain tumors who underwent SRS with a single dose of 18 to 20 Gy. They constructed a 3-water-compartment tissue model, which consisted of intracellular (I), extracellular-extracellular (E), and vascular (V) compartments using DCE-MRI pre-SRS (within 48 h) and post-SRS (either at 1 week or 1 month) to assess the intraextracellular water exchange rate constant (kIE), efflux rate constant, and water compartment volume fractions (M0,I, M0,E, and M0,V). The researchers compared the change in model parameters between the pre-SRS and 1-week post-SRS MRI scans with the change in tumor volume between the pre-SRS and 1-month post-SRS scans. Subsequently, the researchers discovered that early changes in kIE (1 week after SRS) were highly correlated with the long-term tumor response and could predict the extent of tumor shrinkage at 1 month post-SRS.
In conclusion, higher kIE (1 week post-SRS), lower IFP kurtosis, and mean (12 weeks post-SRS) may be regarded as markers of tumor response, while 13C-lactate signals (pre-SRS) and higher Cho/Cr (preradiation therapy) might be predictive of tumor progression. Moreover, the NOE peak amplitude in NAWM and the tumor width at 1 week post-SRS are associated with tumor volume changes at 1 month post-SRS.
Discussion
This review describes the current status of qMRI biomarkers derived from radiation-induced anatomical, morphological, and metabolic alteration for the prediction of treatment response in patients with metastatic brain tumor after SRS. These findings lend support to the implementation of MRI parameters as biomarkers for the early prediction of SRS response of BMs. Considering its significant morbidity and mortality, as well as the limited utility of existing prognostic models constructed by selective clinical data, BM remains a considerable clinical challenge. With the widespread clinical application of SRS for the treatment of cerebral tumors, it is of vital importance for practitioners to construct a promising quantifiable prognostic model that can provide an early prediction of treatment outcome in patients with BM after SRT. Moreover, multiple MRI techniques quantifying the tumor volume, heterogeneity, margins, vascular permeability, cytosis, and tumor microenvironment of cerebral tumors should be developed that leverage the opportunity to combine early noninvasive qMRI biomarkers with the clinical data of patients to improve the prognostic model for BMs treated with SRS.
Various qMRI biomarkers, which were constructed by multiple imaging features derived from conventional MRI sequences before SRS, have been reported as promising markers for predicting treatment response. Advanced MRI techniques facilitate the visualization of tissue changes that are not detectable with common T1- and T2-weighted MRI and can highlight early tissue alteration. The effect of irradiation on the tissue microstructure has been evaluated using DWI and revealed early imaging parameters such as lower relative ADC and higher f values as indicative of response. In PWI, rCBV, rrCBF, IFP, Ktrans2, and ve are indicative of the therapeutic effect of SRS. Furthermore, metabolic changes of Cho/Cr, NOE peak, and kIE obtained by MRS and CEST are related to the long-term response of SRS.
Correlation analysis and prognostic models are two different methods used in these biomarker studies. Correlation analysis is a statistical method for determining the relevance between 2 or more sets of variables, while a prognostic model is a type of clinical prediction model that uses multivariate models to estimate the probability of an outcome occurring in the future and is often applied to cohort studies. There are similarities and differences between these two approaches. First, the prognostic model is based on correlation analysis; only if the variables in question are highly correlated does it make sense to seek the specific the form of their correlation by performing regression analysis or machine learning. Second, the relationship between variables in predictive models is not reciprocal due to the distinction between the independent and dependent variables; however, this is not the case for correlation analysis.
There are differences between the time points for obtaining imaging biomarkers. Some (DI, Ktrans, ve, Cho/Cr, and 13C-lactate) are obtained before treatment, some (ADC, DI, f, Ktrans2, kIE, and NOE) in the early posttreatment phase (≤1 month), and others (rrCBF, Ktrans, and IFP) in the later stage of the disease course (>1 month). Therefore, it makes sense to differentiate the quantitative imaging markers according to the time points (Figure S1). The markers obtained before radiotherapy potentially influence the decision regarding whether or not to use SRS, while the markers obtained shortly after radiation help to predict the later outcomes, allowing for the salvaging of treatment in a timely manner.
There are 5 limitations in the current study that should be noted. First, most articles cited in our review were retrospective studies, and a potential risk of selection bias was inevitable. Second, a small sample size that only included a defined tumor type limit the generalizability of the results to brain metastatic lesions originating from other primary tumors. Third, the single-center studies included in this analysis used inconsistent imaging protocols and processing methods, resulting in the incomparability of the results of these studies. Fourth, the number of the searched websites was limited. Finally, despite the relatively complete selection criteria, there was subjectivity in the review processes.
Based on the reviewed results, future studies on qMRI biomarkers should incorporate a few improvements. First, when investigating the optimal qMRI biomarkers for predicting LC or the long-term outcome of BMs after SRS, increased attention should be paid to the selection of the patients with BM, especially the number, pathological types, and additional treatments (other than SRS). Second, the magnet strength of the post-SRS MRIs should be consistent with the baseline MRIs for further analysis of the serial comparisons. Third, given the growing popularity of tumorous molecular biomarkers, incorporating cellular and/or molecular information into the prognostic model may be a sensible future development. Fourth, to improve future publications on early prognostic qMRI biomarkers, we suggest a more comprehensive description of the time-dependent vascular changes and relevant parametric changes. Finally, we speculate that the individualized qMRI biomarkers are also capable of predicting other treatment outcomes, such as chemo-, targeted, and immuno-therapy.
Conclusions
In the era of conformal photon beam techniques, in which the availability of proton and particle beam therapy is increasing, advanced MRI may provide objective measures for the selection of patients with BM. This review illustrates the potential of MRI in BM response assessment after SRS, and thus, this technique should be included in future prospective clinical trials.
Acknowledgments
Funding: This work was supported by the Chinese National Natural Science Foundation (No. 81972849).
Footnote
Reporting Checklist: The authors have completed the PRISMA-ScR reporting checklist. Available at https://qims.amegroups.com/article/view/10.21037/qims-22-412/rc
Conflicts of Interest: All authors have completed the ICMJE uniform disclosure form (available at https://qims.amegroups.com/article/view/10.21037/qims-22-412/coif). The authors have no conflicts of interest to declare.
Ethical Statement: The authors are accountable for all aspects of the work in ensuring that questions related to the accuracy or integrity of any part of the work are appropriately investigated and resolved.
Open Access Statement: This is an Open Access article distributed in accordance with the Creative Commons Attribution-NonCommercial-NoDerivs 4.0 International License (CC BY-NC-ND 4.0), which permits the non-commercial replication and distribution of the article with the strict proviso that no changes or edits are made and the original work is properly cited (including links to both the formal publication through the relevant DOI and the license). See: https://creativecommons.org/licenses/by-nc-nd/4.0/.
References
- Achrol AS, Rennert RC, Anders C, Soffietti R, Ahluwalia MS, Nayak L, Peters S, Arvold ND, Harsh GR, Steeg PS, Chang SD. Brain metastases. Nat Rev Dis Primers 2019;5:5. [Crossref] [PubMed]
- Boire A, Brastianos PK, Garzia L, Valiente M. Brain metastasis. Nat Rev Cancer 2020;20:4-11. [Crossref] [PubMed]
- Suh JH, Kotecha R, Chao ST, Ahluwalia MS, Sahgal A, Chang EL. Current approaches to the management of brain metastases. Nat Rev Clin Oncol 2020;17:279-99. [Crossref] [PubMed]
- Guan Z, Lan H, Cai X, Zhang Y, Liang A, Li J. Blood-Brain Barrier, Cell Junctions, and Tumor Microenvironment in Brain Metastases, the Biological Prospects and Dilemma in Therapies. Front Cell Dev Biol 2021;9:722917. [Crossref] [PubMed]
- Tsao MN, Rades D, Wirth A, Lo SS, Danielson BL, Gaspar LE, Sperduto PW, Vogelbaum MA, Radawski JD, Wang JZ, Gillin MT, Mohideen N, Hahn CA, Chang EL. Radiotherapeutic and surgical management for newly diagnosed brain metastasis(es): An American Society for Radiation Oncology evidence-based guideline. Pract Radiat Oncol 2012;2:210-25. [Crossref] [PubMed]
- Chao ST, De Salles A, Hayashi M, Levivier M, Ma L, Martinez R, Paddick I, Régis J, Ryu S, Slotman BJ, Sahgal A. Stereotactic Radiosurgery in the Management of Limited (1-4) Brain Metasteses: Systematic Review and International Stereotactic Radiosurgery Society Practice Guideline. Neurosurgery 2018;83:345-53. [Crossref] [PubMed]
- Sahgal A, Ruschin M, Ma L, Verbakel W, Larson D, Brown PD. Stereotactic radiosurgery alone for multiple brain metastases? A review of clinical and technical issues. Neuro Oncol 2017;19:ii2-ii15. [Crossref] [PubMed]
- Gaspar L, Scott C, Rotman M, Asbell S, Phillips T, Wasserman T, McKenna WG, Byhardt R. Recursive partitioning analysis (RPA) of prognostic factors in three Radiation Therapy Oncology Group (RTOG) brain metastases trials. Int J Radiat Oncol Biol Phys 1997;37:745-51. [Crossref] [PubMed]
- Sperduto PW, Berkey B, Gaspar LE, Mehta M, Curran W. A new prognostic index and comparison to three other indices for patients with brain metastases: an analysis of 1,960 patients in the RTOG database. Int J Radiat Oncol Biol Phys 2008;70:510-4. [Crossref] [PubMed]
- Sperduto PW, Chao ST, Sneed PK, Luo X, Suh J, Roberge D, Bhatt A, Jensen AW, Brown PD, Shih H, Kirkpatrick J, Schwer A, Gaspar LE, Fiveash JB, Chiang V, Knisely J, Sperduto CM, Mehta M. Diagnosis-specific prognostic factors, indexes, and treatment outcomes for patients with newly diagnosed brain metastases: a multi-institutional analysis of 4,259 patients. Int J Radiat Oncol Biol Phys 2010;77:655-61. [Crossref] [PubMed]
- Berghoff AS, Wolpert F, Holland-Letz T, Koller R, Widhalm G, Gatterbauer B, Dieckmann K, Birner P, Bartsch R, Zielinski CC, Weller M, Preusser M. Combining standard clinical blood values for improving survival prediction in patients with newly diagnosed brain metastases-development and validation of the LabBM score. Neuro Oncol 2017;19:1255-62. [Crossref] [PubMed]
- Sperduto PW, Yang TJ, Beal K, Pan H, Brown PD, Bangdiwala A, et al. Estimating Survival in Patients With Lung Cancer and Brain Metastases: An Update of the Graded Prognostic Assessment for Lung Cancer Using Molecular Markers (Lung-molGPA). JAMA Oncol 2017;3:827-31. [Crossref] [PubMed]
- Sperduto PW, Kased N, Roberge D, Xu Z, Shanley R, Luo X, et al. Effect of tumor subtype on survival and the graded prognostic assessment for patients with breast cancer and brain metastases. Int J Radiat Oncol Biol Phys 2012;82:2111-7. [Crossref] [PubMed]
- Sperduto PW, Jiang W, Brown PD, Braunstein S, Sneed P, Wattson DA, et al. Estimating Survival in Melanoma Patients With Brain Metastases: An Update of the Graded Prognostic Assessment for Melanoma Using Molecular Markers (Melanoma-molGPA). Int J Radiat Oncol Biol Phys 2017;99:812-6. [Crossref] [PubMed]
- Villanueva-Meyer JE, Mabray MC, Cha S. Current Clinical Brain Tumor Imaging. Neurosurgery 2017;81:397-415. [Crossref] [PubMed]
- Wong KH, Panek R, Bhide SA, Nutting CM, Harrington KJ, Newbold KL. The emerging potential of magnetic resonance imaging in personalizing radiotherapy for head and neck cancer: an oncologist's perspective. Br J Radiol 2017;90:20160768. [Crossref] [PubMed]
- Wang S, Martinez-Lage M, Sakai Y, Chawla S, Kim SG, Alonso-Basanta M, Lustig RA, Brem S, Mohan S, Wolf RL, Desai A, Poptani H. Differentiating Tumor Progression from Pseudoprogression in Patients with Glioblastomas Using Diffusion Tensor Imaging and Dynamic Susceptibility Contrast MRI. AJNR Am J Neuroradiol 2016;37:28-36. [Crossref] [PubMed]
- Patel P, Baradaran H, Delgado D, Askin G, Christos P, John Tsiouris A, Gupta A. MR perfusion-weighted imaging in the evaluation of high-grade gliomas after treatment: a systematic review and meta-analysis. Neuro Oncol 2017;19:118-27. [Crossref] [PubMed]
- Blasel S, Zagorcic A, Jurcoane A, Bähr O, Wagner M, Harter PN, Hattingen E. Perfusion MRI in the Evaluation of Suspected Glioblastoma Recurrence. J Neuroimaging 2016;26:116-23. [Crossref] [PubMed]
- Choi YJ. Kim h, Jahng GH, Kim SJ, Suh DC. Pseudoprogression in patients with glioblastoma: added value of arterial spin labeling to dynamic susceptibility contrast perfusion MR imaging. Acta Radiol 2013;54:448-54. [Crossref] [PubMed]
- Zhang H, Ma L, Wang Q, Zheng X, Wu C, Xu BN. Role of magnetic resonance spectroscopy for the differentiation of recurrent glioma from radiation necrosis: a systematic review and meta-analysis. Eur J Radiol 2014;83:2181-9. [Crossref] [PubMed]
- Muccio CF, Tarantino A, Esposito G, Cerase A. Differential diagnosis by unenhanced FLAIR T2-weighted magnetic resonance images between solitary high grade gliomas and cerebral metastases appearing as contrast-enhancing cortico-subcortical lesions. J Neurooncol 2011;103:713-7. [Crossref] [PubMed]
- Ercan N, Gultekin S, Celik H, Tali TE, Oner YA, Erbas G. Diagnostic value of contrast-enhanced fluid-attenuated inversion recovery MR imaging of intracranial metastases. AJNR Am J Neuroradiol 2004;25:761-5. [PubMed]
- Nardone V, Nanni S, Pastina P, Vinciguerra C, Cerase A, Correale P, Guida C, Giordano A, Tini P, Reginelli A, Cappabianca S, Pirtoli L. Role of perilesional edema and tumor volume in the prognosis of non-small cell lung cancer (NSCLC) undergoing radiosurgery (SRS) for brain metastases. Strahlenther Onkol 2019;195:734-44. [Crossref] [PubMed]
- Tini P, Nardone V, Pastina P, Battaglia G, Vinciguerra C, Carfagno T, Rubino G, Carbone SF, Sebaste L, Cerase A, Federico A, Pirtoli L. Perilesional edema in brain metastasis from non-small cell lung cancer (NSCLC) as predictor of response to radiosurgery (SRS). Neurol Sci 2017;38:975-82. [Crossref] [PubMed]
- Nardone V, Tini P, Biondi M, Sebaste L, Vanzi E, De Otto G, Rubino G, Carfagno T, Battaglia G, Pastina P, Cerase A, Mazzoni LN, Banci Buonamici F, Pirtoli L. Prognostic Value of MR Imaging Texture Analysis in Brain Non-Small Cell Lung Cancer Oligo-Metastases Undergoing Stereotactic Irradiation. Cureus 2016;8:e584. [Crossref] [PubMed]
- Park JH, Choi BS, Han JH, Kim CY, Cho J, Bae YJ, Sunwoo L, Kim JH. MRI Texture Analysis for the Prediction of Stereotactic Radiosurgery Outcomes in Brain Metastases from Lung Cancer. J Clin Med 2021; [Crossref] [PubMed]
- Gutsche R, Lohmann P, Hoevels M, Ruess D, Galldiks N, Visser-Vandewalle V, Treuer H, Ruge M, Kocher M. Radiomics outperforms semantic features for prediction of response to stereotactic radiosurgery in brain metastases. Radiother Oncol 2022;166:37-43. [Crossref] [PubMed]
- Karami E, Soliman H, Ruschin M, Sahgal A, Myrehaug S, Tseng CL, Czarnota GJ, Jabehdar-Maralani P, Chugh B, Lau A, Stanisz GJ, Sadeghi-Naini A. Quantitative MRI Biomarkers of Stereotactic Radiotherapy Outcome in Brain Metastasis. Sci Rep 2019;9:19830. [Crossref] [PubMed]
- Kawahara D, Tang X, Lee CK, Nagata Y, Watanabe Y. Predicting the Local Response of Metastatic Brain Tumor to Gamma Knife Radiosurgery by Radiomics With a Machine Learning Method. Front Oncol 2021;10:569461. [Crossref] [PubMed]
- Mouraviev A, Detsky J, Sahgal A, Ruschin M, Lee YK, Karam I, Heyn C, Stanisz GJ, Martel AL. Use of radiomics for the prediction of local control of brain metastases after stereotactic radiosurgery. Neuro Oncol 2020;22:797-805. [Crossref] [PubMed]
- Jaberipour M, Soliman H, Sahgal A, Sadeghi-Naini A. A priori prediction of local failure in brain metastasis after hypo-fractionated stereotactic radiotherapy using quantitative MRI and machine learning. Sci Rep 2021;11:21620. [Crossref] [PubMed]
- Jiang Z, Wang B, Han X, Zhao P, Gao M, Zhang Y, Wei P, Lan C, Liu Y, Li D. Multimodality MRI-based radiomics approach to predict the posttreatment response of lung cancer brain metastases to gamma knife radiosurgery. Eur Radiol 2022;32:2266-76. [Crossref] [PubMed]
- Wang H, Xue J, Qu T, Bernstein K, Chen T, Barbee D, Silverman JS, Kondziolka D. Predicting local failure of brain metastases after stereotactic radiosurgery with radiomics on planning MR images and dose maps. Med Phys 2021;48:5522-30. [Crossref] [PubMed]
- Zheng Y, Geng D, Yu T, Xia W, She D, Liu L, Yin B. Prognostic value of pretreatment MRI texture features in breast cancer brain metastasis treated with Gamma Knife radiosurgery. Acta Radiol 2021;62:1208-16. [Crossref] [PubMed]
- Zwanenburg A, Vallières M, Abdalah MA, Aerts HJWL, Andrearczyk V, Apte A, et al. The Image Biomarker Standardization Initiative: Standardized Quantitative Radiomics for High-Throughput Image-based Phenotyping. Radiology 2020;295:328-38. [Crossref] [PubMed]
- Schoenegger K, Oberndorfer S, Wuschitz B, Struhal W, Hainfellner J, Prayer D, Heinzl H, Lahrmann H, Marosi C, Grisold W. Peritumoral edema on MRI at initial diagnosis: an independent prognostic factor for glioblastoma? Eur J Neurol 2009;16:874-8. [Crossref] [PubMed]
- Chen Z, Zu J, Li L, Lu X, Ni J, Xu J. Assessment of stereotactic radiosurgery treatment response for brain metastases using MRI based diffusion index. Eur J Radiol Open 2017;4:84-8. [Crossref] [PubMed]
- Jakubovic R, Zhou S, Heyn C, Soliman H, Zhang L, Aviv R, Sahgal A. The predictive capacity of apparent diffusion coefficient (ADC) in response assessment of brain metastases following radiation. Clin Exp Metastasis 2016;33:277-84. [Crossref] [PubMed]
- Shah AD, Shridhar Konar A, Paudyal R, Oh JH, LoCastro E, Nuñez DA, Swinburne N, Vachha B, Ulaner GA, Young RJ, Holodny AI, Beal K, Shukla-Dave A, Hatzoglou V. Diffusion and Perfusion MRI Predicts Response Preceding and Shortly After Radiosurgery to Brain Metastases: A Pilot Study. J Neuroimaging 2021;31:317-23. [Crossref] [PubMed]
- Essig M, Waschkies M, Wenz F, Debus J, Hentrich HR, Knopp MV. Assessment of brain metastases with dynamic susceptibility-weighted contrast-enhanced MR imaging: initial results. Radiology 2003;228:193-9. [Crossref] [PubMed]
- Weber MA, Thilmann C, Lichy MP, Günther M, Delorme S, Zuna I, Bongers A, Schad LR, Debus J, Kauczor HU, Essig M, Schlemmer HP. Assessment of irradiated brain metastases by means of arterial spin-labeling and dynamic susceptibility-weighted contrast-enhanced perfusion MRI: initial results. Invest Radiol 2004;39:277-87. [Crossref] [PubMed]
- Jakubovic R, Sahgal A, Soliman H, Milwid R, Zhang L, Eilaghi A, Aviv RI. Magnetic resonance imaging-based tumour perfusion parameters are biomarkers predicting response after radiation to brain metastases. Clin Oncol (R Coll Radiol) 2014;26:704-12. [Crossref] [PubMed]
- Swinburne N, LoCastro E, Paudyal R, Oh JH, Taunk NK, Shah A, Beal K, Vachha B, Young RJ, Holodny AI, Shukla-Dave A, Hatzoglou V. Computational Modeling of Interstitial Fluid Pressure and Velocity in Non-small Cell Lung Cancer Brain Metastases Treated With Stereotactic Radiosurgery. Front Neurol 2020;11:402. [Crossref] [PubMed]
- Almeida-Freitas DB, Pinho MC, Otaduy MC, Braga HF, Meira-Freitas D, da Costa Leite C. Assessment of irradiated brain metastases using dynamic contrast-enhanced magnetic resonance imaging. Neuroradiology 2014;56:437-43. [Crossref] [PubMed]
- Taunk NK, Oh JH, Shukla-Dave A, Beal K, Vachha B, Holodny A, Hatzoglou V. Early posttreatment assessment of MRI perfusion biomarkers can predict long-term response of lung cancer brain metastases to stereotactic radiosurgery. Neuro Oncol 2018;20:567-75. [Crossref] [PubMed]
- Huang J, Milchenko M, Rao YJ, LaMontagne P, Abraham C, Robinson CG, Huang Y, Shimony JS, Rich KM, Benzinger T. A feasibility study to evaluate early treatment response of brain metastases one week after stereotactic radiosurgery using perfusion weighted imaging. PLoS One 2020;15:e0241835. [Crossref] [PubMed]
- Hlushchuk R, Riesterer O, Baum O, Wood J, Gruber G, Pruschy M, Djonov V. Tumor recovery by angiogenic switch from sprouting to intussusceptive angiogenesis after treatment with PTK787/ZK222584 or ionizing radiation. Am J Pathol 2008;173:1173-85. [Crossref] [PubMed]
- Soares DP, Law M. Magnetic resonance spectroscopy of the brain: review of metabolites and clinical applications. Clin Radiol 2009;64:12-21. [Crossref] [PubMed]
- Kaminaga T, Shirai K. Radiation-induced brain metabolic changes in the acute and early delayed phase detected with quantitative proton magnetic resonance spectroscopy. J Comput Assist Tomogr 2005;29:293-7. [Crossref] [PubMed]
- Usenius T, Usenius JP, Tenhunen M, Vainio P, Johansson R, Soimakallio S, Kauppinen R. Radiation-induced changes in human brain metabolites as studied by 1H nuclear magnetic resonance spectroscopy in vivo. Int J Radiat Oncol Biol Phys 1995;33:719-24. [Crossref] [PubMed]
- Chernov MF, Hayashi M, Izawa M, Abe K, Usukura M, Ono Y, Kubo O, Hori T. Early metabolic changes in metastatic brain tumors after Gamma Knife radiosurgery: 1H-MRS study. Brain Tumor Pathol 2004;21:63-7. [Crossref] [PubMed]
- Jia C, Li Z, Guo D, Zhang Z, Yu J, Jiang G, Xing X, Ji S, Jin F. Brain Metastases of Non-Small Cell Lung Cancer: Magnetic Resonance Spectroscopy for Clinical Outcome Assessment in Patients with Stereotactic Radiotherapy. Onco Targets Ther 2020;13:13087-96. [Crossref] [PubMed]
- Lee CY, Soliman H, Bragagnolo ND, Sahgal A, Geraghty BJ, Chen AP, Endre R, Perks WJ, Detsky JS, Leung E, Chan M, Heyn C, Cunningham CH. Predicting response to radiotherapy of intracranial metastases with hyperpolarized [Formula: see text]C MRI. J Neurooncol. 2021;152:551-7. [Crossref] [PubMed]
- Zhou J, Tryggestad E, Wen Z, Lal B, Zhou T, Grossman R, Wang S, Yan K, Fu DX, Ford E, Tyler B, Blakeley J, Laterra J, van Zijl PC. Differentiation between glioma and radiation necrosis using molecular magnetic resonance imaging of endogenous proteins and peptides. Nat Med 2011;17:130-4. [Crossref] [PubMed]
- Sagiyama K, Mashimo T, Togao O, Vemireddy V, Hatanpaa KJ, Maher EA, Mickey BE, Pan E, Sherry AD, Bachoo RM, Takahashi M. In vivo chemical exchange saturation transfer imaging allows early detection of a therapeutic response in glioblastoma. Proc Natl Acad Sci U S A 2014;111:4542-7. [Crossref] [PubMed]
- Dula AN, Arlinghaus LR, Dortch RD, Dewey BE, Whisenant JG, Ayers GD, Yankeelov TE, Smith SA. Amide proton transfer imaging of the breast at 3 T: establishing reproducibility and possible feasibility assessing chemotherapy response. Magn Reson Med 2013;70:216-24. [Crossref] [PubMed]
- Jones KM, Pollard AC, Pagel MD. Clinical applications of chemical exchange saturation transfer (CEST) MRI. J Magn Reson Imaging 2018;47:11-27. [Crossref] [PubMed]
- Desmond KL, Mehrabian H, Chavez S, Sahgal A, Soliman H, Rola R, Stanisz GJ. Chemical exchange saturation transfer for predicting response to stereotactic radiosurgery in human brain metastasis. Magn Reson Med 2017;78:1110-20. [Crossref] [PubMed]
- Bailey C, Moosvi F, Stanisz GJ. Mapping water exchange rates in rat tumor xenografts using the late-stage uptake following bolus injections of contrast agent. Magn Reson Med 2014;71:1874-87. [Crossref] [PubMed]
- Springer CS Jr, Li X, Tudorica LA, Oh KY, Roy N, Chui SY, Naik AM, Holtorf ML, Afzal A, Rooney WD, Huang W. Intratumor mapping of intracellular water lifetime: metabolic images of breast cancer? NMR Biomed 2014;27:760-73. [Crossref] [PubMed]
- Sobol WT, Jackels SC, Cothran RL, Hinson WH. NMR spin-lattice relaxation in tissues with high concentration of paramagnetic contrast media: evaluation of water exchange rates in intact rat muscle. Med Phys 1991;18:243-50. [Crossref] [PubMed]
- Buckley DL, Kershaw LE, Stanisz GJ. Cellular-interstitial water exchange and its effect on the determination of contrast agent concentration in vivo: dynamic contrast-enhanced MRI of human internal obturator muscle. Magn Reson Med 2008;60:1011-9. [Crossref] [PubMed]
- Mehrabian H, Desmond KL, Chavez S, Bailey C, Rola R, Sahgal A, Czarnota GJ, Soliman H, Martel AL, Stanisz GJ. Water Exchange Rate Constant as a Biomarker of Treatment Efficacy in Patients With Brain Metastases Undergoing Stereotactic Radiosurgery. Int J Radiat Oncol Biol Phys 2017;98:47-55. [Crossref] [PubMed]