Radiomics-based intracranial thrombus features on preoperative noncontrast CT predicts successful recanalization of mechanical thrombectomy in acute ischemic stroke
Introduction
Acute ischemic stroke (AIS), one of the two main subtypes of stroke, is caused by the thrombosis of cerebral arteries (1). Following the “time is brain” paradigm, clinicians must shorten the time from symptom onset to intervention, as every minute of delay in treatment can significantly expand infarct volume and worsen outcomes (2,3). Mechanical thrombectomy (MTB) is a standard treatment for AIS secondary to large vessel occlusion, among which stent retriever (SR) treatment is recommended as an evidence-supported first-line treatment strategy (4-6).
Achieving rapid recanalization and complete or near complete reperfusion has been associated with improved clinical outcomes and reduced complications (7,8). Since recognizing the significant role of recanalization in stroke, a key issue has been the selection of eligible patients who would benefit from SR treatment. The current application of imaging techniques in AIS have been focused on identifying the presence and extent of potentially salvageable areas upon recanalization. In this regard, brain tissues and arteries are considered the targets of recanalization, and thrombus evaluation is essential for predicting response to MTB. It has been recently shown that patient response to successful recanalization may differ according to the clinical and demographic factors (9,10). Moreover, the image characteristics of the thrombus, such as length and density, are also linked to recanalization success (11,12). While the thrombus can be detected by computed tomography (CT) and magnetic resonance imaging (MRI), most studies on thrombus imaging for recanalization have been focused on CT (13,14). Compared to MRI, CT is more easily and widely used for patients with AIS, especially in emergency settings and less-developed communities. Nonetheless, using these factors to reliably predict recanalization with SR treatment in patients with proximal intracranial thrombus is still limited.
Radiomics, a newly emerging computer-assisted technique, allows for high-throughput analysis of imaging data revealing quantitative information beyond visual assessment by expert (15,16). These features depict voxel intensity, three-dimensional shape and size, coarseness of surface, and gray level. A recent study reported that the texture of the thrombus is more predictive of recanalization success after intravenous alteplase therapy for AIS than previously identified thrombus imaging features such as length, volume, and permeability (17). Another study found that radiomics analysis of thrombus was able to predict a direct aspiration first-pass technique (ADAPT) technique for successful recanalization in AIS and select patients who are most likely to benefit from the intervention (18). However, as another treatment regimen, no radiomics study has focused on the predicting success of recanalization following SR based on pretherapy CT images.
Thus, the present study aimed to investigate whether the thrombus radiomics features derived from thin-section CT can be predictive of recanalization after intra-arterial MTB treatment. We also constructed different machine learning (ML) classifiers to compare which classifier is most suitable in this study according to statistical analysis and determine whether a combined model consisting of clinical-radiological risk variables and a radiomics model could better discriminate the recanalization success than a radiomics model alone. We present the following article in accordance with the STARD reporting checklist (available at https://qims.amegroups.com/article/view/10.21037/qims-22-599/rc).
Methods
Patients
The study was conducted in accordance with the Declaration of Helsinki (as revised in 2013). This study was approved by the institutional review board of Northern Jiangsu People’s Hospital (No. 2022ky058), and individual consent for this retrospective analysis was waived. 256 patients newly diagnosed AIS between March 2017 and September 2020 from two institutes, including the first affiliated hospital of Soochow university (institute I) and Northern Jiangsu People’s hospital (institute II), were enrolled continuously and retrospectively. Inclusion criteria: (I) AIS with internal carotid artery/M1 middle cerebral artery segment thrombus; (II) received MTB treatment using the SR technique, followed by additional devices (e.g., ADAPT technique) at the operator’s discretion if failed; (III) all patients underwent CT imaging, including noncontrast CT (NCCT), CT angiography (CTA), and/or CT perfusion. Successful recanalization was defined as a Thrombolysis In Cerebral Infarction score 2b or 3 on the final digital subtracted angiography during MTB, as it represents a powerful predictor of treatment success and clinical outcome (19). Patients with unsatisfactory image quality, such as motion or metal artifacts, and incomplete clinical information were excluded. The eligible patients from institute I were randomly divided into training and internal validation cohorts at a ratio of 7 to 3, and patients from institute II were allocated to the external validation cohort. This diagnostic study did not register with a clinical trial platform and the study protocol has not been published. The flowchart shows the analysis pathway for this study (Figure 1A).
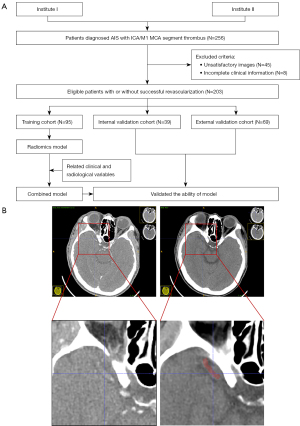
Image examination
All examinations were performed on multi-detector CT scanners (GE Healthcare, USA; United imaging, China). The scanning ranged from the top of the skull to the base. The scanning parameters were: tube voltage 120 kV, tube current 200–360 mA, field of view 320 mm, matrix 512×512, and layer thickness 5 mm.
Image preprocessing and segmentation
All CT images were saved as Digital Imaging and Communications in Medicine (DICOM) files and loaded into ITK-SNAP, an open-source software (www.itksnap.org). NCCT images were reconstructed with a voxel of 1×1×1 mm3 and gray-scale discretization to remove the potential differences of CT images acquired by different CT scanners. Gray-scale discretization was performed between µ ± 3σ [µ, the mean value of the gray levels within the region of interest (ROI); σ, the standard deviation]. A senior neuroradiologist performed manual contouring of thrombus in a slice-by-slice manner from axial views of NCCT images using ITK-SNAP by viewing the corresponding co-registered CTA images for guidance (Figure 1B). Another neuroradiologist independently segmented the ROIs in 40 randomly selected patients to analyze the variability of segmentation. They were blind to the clinical information and final treatment effect.
Radiomics feature extraction and selection
Overall, 1,130 radiomics features were automatically extracted from the voxel of interest (VOI) using PyRadiomics, an open-source and widely used platform for radiomics analysis. This allowed to compute first-order statistics and higher order textural features using gray level co-occurrence matrix features (GLCM), gray level size zone matrix features (GLSZM), gray level run length matrix (GLRLM), neighboring gray tone difference matrix (NGTDM), and gray level dependence matrix features (GLDM). Additional wavelet filtering (eight decompositions per level) and three different Laplacian of Gaussian filters (with sigma values of one, three, and five) were applied to the original images for further higher order features after pre-processing with gray-scale discretization.
Before selecting features, the features values of all VOIs were normalized with a Z-score [(x – µ)/σ; x, the feature value; µ, the average of the feature values among all patients; σ, the corresponding standard deviation] to eliminate the unit limit for each feature. Single-factor logistic regression analysis was conducted to select highly significant and correlated features. Then, the least absolute shrinkage and selection operator (LASSO) regression method was performed based on maximum area under curve (AUC) criteria in the training cohort. A 10-fold cross-internal validation method was adopted to choose the optimized subset of features.
Model construction and evaluation
The selected radiomics features and ML classifiers were used to construct models in training cohort. The internal validation cohort was used for model optimization. The ML classifiers included the naïve Bayes (NB), random forest (RF), logistic regression (LR), linear support vector machine (L.SVM), radial SVM (R.SVM), and artificial neural network (ANN). These classifiers are widely used and proven to be effective. Grid search was used for hyperparameter tuning, and we set cost 10−4 to 104 for L.SVM, cost 10−4 to 104 and gamma 10−4 to 104 for R.SVM, ntree 100 to 500 for RF, hidden 1 to 5 for ANN. The parameters of the six ML classifiers are showed in Table 1. The sensitivity, specificity, positive predictive value (PPV), negative predictive value (NPV) and Matthews correlation coefficient (MCC) for each model were calculated via a confusion matrix to evaluate discriminative ability. The MCC takes into account true positives, true negatives, false positives and false negatives, and is generally considered to be a well-balanced metric that can be applied even when the sample sizes of the two groups vary greatly. This metric is essentially a correlation coefficient describing the actual classification and the predicted classification, its value range is −1 to 1. A value of 1 indicates a perfect prediction of the subject, 0 indicates the predicted result is not as good as the random prediction, and −1 means that the predicted classification and the actual classification are completely inconsistent (20).
Table 1
Classifiers | Package | Parameters |
---|---|---|
L.SVM | e1071 | kernel: linear; cost: 0.1; number of support vectors: 53 |
R.SVM | e1071 | kernel: radial; cost: 1; gamma: 0.1; number of Support vectors: 63 |
RF | randomForest | number of trees: 500; no. of variables tried at each split: 2; OOB estimate of error rate: 16.84% |
NB | e1071 | laplace: 0; na.action: na.pass |
LR | glmnet | residual: 86; null deviance: 128.6; residual deviance: 64.34; AIC: 82.34 |
ANN | neuralnet | hidden: 3; err.fct: ce; linear.output: false; erro: 31.9826; steps: 9102 |
ML, machine learning; L.SVM, linear support vector machine; R.SVM, radial SVM; RF, random forest; NB, naïve Bayes; LR, logistic regression; ANN, artificial neural network; OOB, out of bag; AIC, Akaike information criterion.
In this study, four related clinical variables, including age, gender, atrial fibrillation, national institutes of health stroke scale (NIHSS) on admission, and two radiological variables, including HDVS (hyperdense vessel sign) and thrombus volume, were incorporated into the radiomics model to develop the combined model (21-23). Moreover, the best classifier was chosen to construct a clinical model based on the selected clinical and radiological variables. Receiver operating characteristic (ROC) curves for models were also generated. These models were constructed in the training cohort and optimized in the internal validation. The external validation was used for the independently verification.
Statistical analysis
Statistical analysis was performed using SPSS (version 22.0) and R statistical software (version 3.6.2). For continuous variables, difference was calculated using the Student’s t-test or the Mann-Whitney U test as appropriate. Continuous data are represented as mean ± standard deviation or as median with interquartile range depending on whether or not the data were normally distributed. For categorical variables, difference was calculated with Chi-square or Fisher’s exact test as appropriate. ROC curves were compared using Delong test. The threshold for significance was set at P<0.05.
Results
Patients
After strict inclusion and exclusion, 45 patients with unsatisfactory image quality and eight with incomplete clinical information were excluded. Finally, 203 patients were enrolled. Among them, 95 patients were used for training and 39 patients used for internal validation from institute I, and 69 patients from institute II were used for external validation. Moreover, 128 (62.75%) cases achieved successful recanalization, including 56 (58.95%), 28 (71.79%) and 44 (63.77%) cases in the training, internal validation and external validation cohorts, respectively. There were no statistically significant differences of baseline demographic characteristics, clinical variables, and radiological variables between the patient groups, as seen in Table 2. The average interval was 158.4 min (range, 60–270 min) between preoperative CT examination and MTB performed.
Table 2
Variables | Training cohort (n=95) | Internal validation cohort (n=39) | External validation cohort (n=69) |
---|---|---|---|
Age in years* | 66.81±10.84 | 68.13±13.20 | 64.12±12.56 |
Male, n (%) | 55 (57.89) | 21 (53.85) | 37 (53.62) |
History, n (%) | |||
Hypertension | 61 (64.21) | 29 (74.36) | 49 (71.01) |
Diabetes | 16 (16.84) | 8 (20.51) | 8 (11.59) |
Anticoagulant therapy | 24 (25.26) | 9 (23.08) | 15 (21.74) |
Stroke | 22 (23.16) | 8 (20.51) | 8 (11.59) |
Coronary heart disease | 13 (13.68) | 6 (15.38) | 3 (4.35) |
Atrial fibrillation | 23 (24.21) | 13 (33.33) | 25 (36.23) |
Alcohol consumption | 23 (24.21) | 13 (33.33) | 11 (15.94) |
Smoking | 38 (40.00) | 10 (25.64) | 12 (17.39) |
Admission SBP (mmHg)* | 150.67±23.27 | 151.85±22.98 | 126.12±23.27 |
Admission DBP (mmHg)* | 84.74±14.38 | 86.205±14.68 | 71.32±14.96 |
Admission NIHSS* | 16.92±8.34 | 19.08±9.69 | 16.16±4.80 |
Time to admission (h)# | 3.00 (2.00, 4.50) | 4.00 (2.00, 5.00) | 3.50 (2.00, 5.00) |
MCA, n (%) | 84 (88.42) | 31 (79.49) | 57 (82.61) |
HDVS, n (%) | 52 (54.74) | 23 (58.97) | 38 (55.07) |
Thrombus volume (mm3)# | 62.25 (37.87, 91.75) | 63.75 (31.00, 104.38) | 70.55 (53.05, 90.31) |
*, represented as mean ± standard deviation; #, represented as median with interquartile range. SBP, systolic blood pressure; DBP, diastolic blood pressure; NIHSS, National Institutes of Health Stroke Scale; MCA, middle cerebral artery; HDVS, hyperdense vessel sign.
Selection of the best radiomics features
Out of 1,130 radiomics features, 858 (75.93%) had good consistency [intraclass correlation coefficients (ICCs), 0.802–0.9230]. The subset of the eight most relevant radiomics features (log-sigma-3mm_ GLSZM_ LowGrayLevelZoneEmphasis, log-sigma-5mm_ GLRLM_ ShortRunHighGrayLevelEmphasis, wavelet-LHL_ GLSZM_ LargeAreaHighGrayLevelEmphasis, wavelet-HLH_ Firstorder_ Mean, wavelet-HHH_ GLRLM_ GrayLevelNonUniformityNormalized, wavelet-HHL_ GLRLM_ GrayLevelVariance, wavelet-HHL_ GLSZM_ GrayLevelNonUniformity, original_ GLRLM_ GrayLevelNonUniformityNormalized) were identified using single-factor logistic regression analysis and a LASSO algorithm in the training cohort. The cross internal validation of LASSO and the correlation coefficient matrix of selected radiomics features are showed in Figure 2. The detailed description of the selected radiomics features were reported in Table S1.
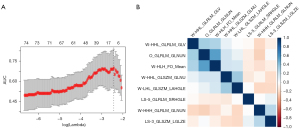
Performance of the radiomics models
In the training cohort, the six ML classifiers including L.SVM, R.SVM, RF, NB, LR and ANN obtained similar AUCs [0.933, 95% confidence interval (CI): 0.871–0.892; 0.942, 95% CI: 0.762–0.801; 0.889, 95% CI: 0.630–0.852; 0.924, 95% CI: 0.855–0.898; 0.925, 95% CI: 0.697–0.912; 0.950, 95% CI: 0.754–0.893], respectively. In the internal validation cohort, the six ML classifiers obtained AUCs [0.901, 95% CI: 0.734–0.795; 0.792, 95% CI: 0.840–0.992; 0.820, 95% CI: 0.763–0.859; 0.737, 95% CI: 0.803–0.855; 0.834, 95% CI: 0.769–0.958; 0.909, 95% CI: 0.772–0.804], respectively. The ANN classifier showed the highest AUCs in the training and internal validation cohorts. The ANN classifier showed the highest MCC of 0.913 in the training cohort, while the L.SVM classifier showed the highest MCC of 0.766 in the internal validation cohort.
ANN and L.SVM were regarded as the two most suitable ML classifiers and were developed using the external validation cohort with MCCs of 0.505 and 0.482, respectively (Figure 3A). While the ROC curves of ANN and L.SVM showed a significant difference (P<0.001), the ANN classifier outperformed L.SVM in distinguishing the successful and unsuccessful recanalization cases in the external validation cohort. The accuracy, sensitivity, specificity, PPV, NPV, and MCC for the models in the internal and external cohort were showed in Table 3. The corresponding ROC curves of six ML classifiers in the internal and external cohorts were shown in Figure 3B,3C.
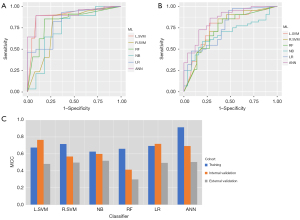
Table 3
Classifiers | MCC | AUC | SEN | SPE | PPV | NPV |
---|---|---|---|---|---|---|
External validation | ||||||
L.SVM | 0.482 | 0.746 | 0.773 | 0.720 | 0.829 | 0.643 |
R.SVM | 0.498 | 0.758 | 0.795 | 0.72 | 0.833 | 0.667 |
RF | 0.298 | 0.741 | 0.841 | 0.640 | 0.804 | 0.696 |
NB | 0.519 | 0.677 | 0.591 | 0.800 | 0.839 | 0.526 |
LR | 0.494 | 0.850 | 0.864 | 0.720 | 0.844 | 0.750 |
ANN | 0.505 | 0.808 | 0.680 | 0.920 | 0.929 | 0.561 |
Internal validation | ||||||
L.SVM | 0.766 | 0.901 | 0.893 | 0.909 | 0.962 | 0.769 |
R.SVM | 0.570 | 0.792 | 0.857 | 0.727 | 0.889 | 0.667 |
RF | 0.415 | 0.820 | 0.821 | 0.818 | 0.920 | 0.643 |
NB | 0.600 | 0.737 | 0.857 | 0.636 | 0.857 | 0.636 |
LR | 0.719 | 0.834 | 0.857 | 0.818 | 0.923 | 0.692 |
ANN | 0.693 | 0.909 | 0.893 | 0.909 | 0.962 | 0.769 |
ML, machine learning; MCC, Matthews correlation coefficient; AUC, area under curve; SEN, sensitivity; SPE, specificity; PPV, positive-predictive value; NPV, negative predictive value; L.SVM, linear support vector machine; R.SVM, radial support vector machine; RF, random forest; NB, naïve Bayes; LR, logistic regression; ANN, artificial neural network.
Adding clinical information into radiomics model and validation
The combined model, with clinical and radiological variables incorporated into the ANN-based radiomics model, did not show better ROC curves in the internal validation and external validation cohorts than the radiomics models alone (P>0.05). A clinical model was also constructed using ANN to evaluate its diagnostic efficacy. The ROC curves of the three models in the internal and external validation cohorts are presented in Figure 4, and the AUC, sensitivity, specificity, PPV, and NPV of the models were showed in Table 4 in detail.
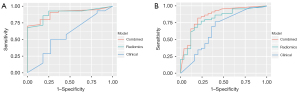
Table 4
Cohort | Model | AUC | MCC | SEN | SPE | PPV | NPV | P* |
---|---|---|---|---|---|---|---|---|
Internal validation | Radiomics | 0.873 | 0.693 | 0.926 | 0.750 | 0.893 | 0.818 | 0.567 |
Clinical | 0.568 | 0.245 | 0.500 | 0.727 | 0.571 | 0.680 | ||
Combined | 0.860 | 0.683 | 0.909 | 0.729 | 0.714 | 0.818 | ||
External validation | Radiomics | 0.805 | 0.505 | 0.787 | 0.682 | 0.841 | 0.600 | 0.200 |
Clinical | 0.663 | 0.353 | 0.767 | 0.600 | 0.514 | 0.824 | ||
Combined | 0.849 | 0.524 | 0.886 | 0.618 | 0.705 | 0.840 |
*, Delong test between radiomics and combined models; AUC, area under curve; MCC, Matthews correlation coefficient; SEN, sensitivity; SPE, specificity; PPV, positive-predictive value; NPV, negative predictive value.
Discussion
In this retrospective study, we aimed to predict recanalization success with MTB based on radiomics features derived from intracranial thrombus CT imaging in patients with AIS. By calculating the texture, size, shape, and higher order parameters of thrombus, we developed and validated ML classifiers to identify patients with recanalization following SR treatment. Overall, the ANN classifier developed by thrombus radiomics features achieved the best prediction of early recanalization. Adding clinical information into the radiomics model did not improve the final results. This may allow appropriate selection of first-line MTB to increase the clinical benefit for each patient.
A recent article argued that factors that are well established for a specific disease should always be included regardless of their statistical significance in a particular dataset (24). The recommended approach is to select important factors, rather than selecting factors via their significance in univariable analysis. Herein, four related clinical variables and two radiological variables, such as age, gender, atrial fibrillation status, were selected according to clinical practice (21). One possible reason that increased age and the presence of atrial fibrillation were contributors to successful recanalization may be stroke etiology (25). The differences in anatomical, pathophysiological, and biochemical factors, endogenous fibrinolytic activity, may lead to different responses to recanalization therapy between sexes (26). Many studies have sought to explore potential factors influencing recanalization efficacy after MTB. The image characteristics of the thrombus, such as length and density, are also linked to recanalization success (11,12). However, it is very difficult to measure thrombus length on NCCT images without the aid of specific software. What’s more, this measurement on CTA images is indirectly based on the presence of contrast opacification. The thrombus density on CT images may represent the compositions of the thrombus, and it has been previously shown that different biochemical compositions appear to affect their treatment response, in that the HU is higher in red blood cell-rich regions and lower in fibrin- or platelet-rich regions. Thus, thrombus with HDVS was easier to recanalize than fibrin-rich thrombus without HDVS. However, the distribution of each composition within the thrombus is inhomogeneous. So, the measurement of the thrombus may underestimate or overestimate its density.
Limitations on image characteristics in previous studies, demonstrate that objective, quantitative analysis is necessary. Recently, CT-based radiomics analysis has been applied to discriminate recanalization success after treatment with promising results (17,18). They found that thrombus images contain valuable information that can be used to predict alteplase and aspiration efficacy in the treatment of AIS. Another paper utilizes single-phase CTA for thrombus measurements and explores the efficiency of thrombus texture to predict more attempts to successful reperfusion in patients with anterior circulation stroke. These thrombus texture, however, were not related to functional outcome (27). Thus, we formulate a hypothesis that the biochemical characteristics of the thrombus can contributed to decision-making and treatment planning in patients receiving SR. Feature selection showed that the most contributory features to the classification between subtypes were derived from GLRLM (4/8) and GLSZM (3/8) features. The GLRLM quantifies gray level runs, which are defined as the length in number of pixels, of consecutive pixels that have the same gray level value. In this matrix, GLNUN measures the similarity of gray-level intensity values in the image, where a lower GLNUN value correlates with a greater similarity in intensity values (28). The GLSZM quantifies gray level zones in an image, and GLNU measures the variability of gray-level intensity values in the image, with a lower value indicating more homogeneity in intensity values (29). A thrombus with successful recanalization shows lower GLSZM_GLNU value than one with failed recanalization, meaning that these thrombi are more homogeneous.
In the present study, ML classifiers of features extracted from the thrombus on the baseline NCCT were useful to predict successful recanalization. Among six ML classifiers used in this study, ANN achieved best predictive performance. Universally applied in medical practice, the ANN classifier has proven its robustness against a variety of input features and random noise (30,31). The performance of classifiers varied between the internal and external cohort, which may due to the small sample size of the present study. The predictive performance of the combined model was not significantly improved compared to the radiomics model alone. It indicated that the combined model was weighted more heavily towards the radiomics features which produced better performances than clinical and radiological variables. The result further points to the potential of a radiomics-based objective and quantized strategy for more standardized diagnostic process.
There are several limitations to this work. Firstly, there was relatively small sample size and an unbalanced distribution between groups because of the retrospective nature of study and strict inclusion criteria. Secondly, the manual measurement of thrombus characteristics is inefficient in an emergency setting and could lead to a lack of reproducibility, and further studies will need to focus on automatic or semiautomatic segmentation and analysis. Thirdly, thrombus composition was not systematically analyzed, so we were unable to explore underlying biology of thrombus related to the CT-based radiomics features. This type of correlation will require in vitro studies that look at radiomics and thrombus pathology together. Finally, recanalization was used as the only biological marker of outcomes, while prognosis, such as mortality, was not evaluated.
Conclusions
Our findings suggest that preoperative thrombus-based radiomics analysis can help identify patients who are likely to achieve recanalization with SR treatment. Although, at present, the segmentation tools and the time needed to perform the analysis are not adapted for clinical practice, further studies should explore the role of artificial intelligence for providing a rapid comprehensive thrombus segmentation and image analysis that could help clinicians determine whether patients would recanalize in a reasonable timeframe with SR treatment.
Acknowledgments
This manuscript was edited by Charlesworth Academic Editing.
Funding: This work was supported by the scientific research fund of Northern Jiangsu People’s Hospital (No. SBKY21034) and Gusu Health Talent Project of Suzhou (No. GSWS2020003).
Footnote
Reporting Checklist: The authors have completed the STARD reporting checklist. Available at https://qims.amegroups.com/article/view/10.21037/qims-22-599/rc
Conflicts of Interest: All authors have completed the ICMJE uniform disclosure form (available at https://qims.amegroups.com/article/view/10.21037/qims-22-599/coif). The authors have no conflicts of interest to declare.
Ethical Statement: The authors are accountable for all aspects of the work in ensuring that questions related to the accuracy or integrity of any part of the work are appropriately investigated and resolved. The study was conducted in accordance with the Declaration of Helsinki (as revised in 2013). This study was approved by institutional review board of Northern Jiangsu People’s Hospital (No. 2022ky058), and individual consent for this retrospective analysis was waived.
Open Access Statement: This is an Open Access article distributed in accordance with the Creative Commons Attribution-NonCommercial-NoDerivs 4.0 International License (CC BY-NC-ND 4.0), which permits the non-commercial replication and distribution of the article with the strict proviso that no changes or edits are made and the original work is properly cited (including links to both the formal publication through the relevant DOI and the license). See: https://creativecommons.org/licenses/by-nc-nd/4.0/.
References
- Zhang D, Wang M, Wu L, Zhao Y, Wang S, Yin X, Wu X. Assessing the characteristics and diagnostic value of plaques for patients with acute stroke using high-resolution magnetic resonance imaging. Quant Imaging Med Surg 2022;12:1529-38. [Crossref] [PubMed]
- Lapergue B, Blanc R, Gory B, Labreuche J, Duhamel A, Marnat G, Saleme S, Costalat V, Bracard S, Desal H, Mazighi M, Consoli A, Piotin MASTER Trial Investigators. Effect of Endovascular Contact Aspiration vs Stent Retriever on Revascularization in Patients With Acute Ischemic Stroke and Large Vessel Occlusion: The ASTER Randomized Clinical Trial. JAMA 2017;318:443-52. [Crossref] [PubMed]
- Foo LS, Harston G, Mehndiratta A, Yap WS, Hum YC, Lai KW, Mohamed Mukari SA, Mohd Zaki F, Tee YK. Clinical translation of amide proton transfer (APT) MRI for ischemic stroke: a systematic review (2003-2020). Quant Imaging Med Surg 2021;11:3797-811. [Crossref] [PubMed]
- Sivan-Hoffmann R, Gory B, Armoiry X, Goyal M, Riva R, Labeyrie PE, Lukaszewicz AC, Lehot JJ, Derex L, Turjman F. Stent-Retriever Thrombectomy for Acute Anterior Ischemic Stroke with Tandem Occlusion: A Systematic Review and Meta-Analysis. Eur Radiol 2017;27:247-54. [Crossref] [PubMed]
- Hong KS, Ko SB, Yu KH, Jung C, Park SQ, Kim BM, Chang CH, Bae HJ, Heo JH, Oh CW, Lee BC, Kim BT, Kim BS, Chung CS, Yoon BW, Rha JH. Update of the Korean Clinical Practice Guidelines for Endovascular Recanalization Therapy in Patients with Acute Ischemic Stroke. J Stroke 2016;18:102-13. [Crossref] [PubMed]
- Berkhemer OA, Fransen PS, Beumer D, van den Berg LA, Lingsma HF, Yoo AJ, et al. A randomized trial of intraarterial treatment for acute ischemic stroke. N Engl J Med 2015;372:11-20. [Crossref] [PubMed]
- Bourcier R, Saleme S, Labreuche J, Mazighi M, Fahed R, Blanc R, Gory B, Kyheng M, Marnat G, Bracard S, Desal H, Consoli A, Piotin M, Lapergue BASTER Trial Investigators. More than three passes of stent retriever is an independent predictor of parenchymal hematoma in acute ischemic stroke. J Neurointerv Surg 2019;11:625-9. [Crossref] [PubMed]
- Arnold M, Mattle S, Galimanis A, Kappeler L, Fischer U, Jung S, De Marchis GM, Gralla J, Mono ML, Brekenfeld C, Meier N, Nedeltchev K, Schroth G, Mattle HP. Impact of admission glucose and diabetes on recanalization and outcome after intra-arterial thrombolysis for ischaemic stroke. Int J Stroke 2014;9:985-91. [Crossref] [PubMed]
- Froehler MT, Tateshima S, Duckwiler G, Jahan R, Gonzalez N, Vinuela F, Liebeskind D, Saver JL, Villablanca JPUCLA Stroke Investigators. The hyperdense vessel sign on CT predicts successful recanalization with the Merci device in acute ischemic stroke. J Neurointerv Surg 2013;5:289-93. [Crossref] [PubMed]
- Goda T, Oyama N, Kitano T, Iwamoto T, Yamashita S, Takai H, Matsubara S, Uno M, Yagita Y. Factors Associated with Unsuccessful Recanalization in Mechanical Thrombectomy for Acute Ischemic Stroke. Cerebrovasc Dis Extra 2019;9:107-13. [Crossref] [PubMed]
- Grifoni E, Giglio D, Guazzini G, Cosentino E, Latini E, Dei A, et al. Age-related burden and characteristics of embolic stroke of undetermined source in the real world clinical practice. J Thromb Thrombolysis 2020;49:75-85. [Crossref] [PubMed]
- Hawkes MA, Farez MF, Pertierra L, Gomez-Schneider MM, Pastor-Rueda JM, Ameriso SF. Differential characteristics, stroke recurrence, and predictors of covert atrial fibrillation of embolic strokes of undetermined source. Int J Stroke 2018;13:190-4. [Crossref] [PubMed]
- Heo JH, Kim K, Yoo J, Kim YD, Nam HS, Kim EY. Computed Tomography-Based Thrombus Imaging for the Prediction of Recanalization after Reperfusion Therapy in Stroke. J Stroke 2017;19:40-9. [Crossref] [PubMed]
- El-Tawil S, Wardlaw J, Ford I, Mair G, Robinson T, Kalra L, Muir KW. Penumbra and re-canalization acute computed tomography in ischemic stroke evaluation: PRACTISE study protocol. Int J Stroke 2017;12:671-8. [Crossref] [PubMed]
- Bagher-Ebadian H, Janic B, Liu C, Pantelic M, Hearshen D, Elshaikh M, Movsas B, Chetty IJ, Wen N. Detection of Dominant Intra-prostatic Lesions in Patients With Prostate Cancer Using an Artificial Neural Network and MR Multi-modal Radiomics Analysis. Front Oncol 2019;9:1313. [Crossref] [PubMed]
- Shu J, Tang Y, Cui J, Yang R, Meng X, Cai Z, Zhang J, Xu W, Wen D, Yin H. Clear cell renal cell carcinoma: CT-based radiomics features for the prediction of Fuhrman grade. Eur J Radiol 2018;109:8-12. [Crossref] [PubMed]
- Qiu W, Kuang H, Nair J, Assis Z, Najm M, McDougall C, McDougall B, Chung K, Wilson AT, Goyal M, Hill MD, Demchuk AM, Menon BK. Radiomics-Based Intracranial Thrombus Features on CT and CTA Predict Recanalization with Intravenous Alteplase in Patients with Acute Ischemic Stroke. AJNR Am J Neuroradiol 2019;40:39-44. [Crossref] [PubMed]
- Hofmeister J, Bernava G, Rosi A, Vargas MI, Carrera E, Montet X, Burgermeister S, Poletti PA, Platon A, Lovblad KO, Machi P. Clot-Based Radiomics Predict a Mechanical Thrombectomy Strategy for Successful Recanalization in Acute Ischemic Stroke. Stroke 2020;51:2488-94. [Crossref] [PubMed]
- Carvalho A, Rocha M, Rodrigues M, Gregório T, Costa H, Cunha A, Castro S, Veloso M, Ribeiro M, Barros PJG. Time to Reset the Definition of Successful Revascularization in Endovascular Treatment of Acute Ischemic Stroke. Cerebrovasc Dis 2018;46:40-5. [Crossref] [PubMed]
- Chicco D, Jurman G. The advantages of the Matthews correlation coefficient (MCC) over F1 score and accuracy in binary classification evaluation. BMC Genomics 2020;21:6. [Crossref] [PubMed]
- Baek JH, Yoo J, Song D, Kim YD, Nam HS, Kim BM, Kim DJ, Lee HS, Heo JH. Predictive value of thrombus volume for recanalization in stent retriever thrombectomy. Sci Rep 2017;7:15938. [Crossref] [PubMed]
- Luthman AS, Bouchez L, Botta D, Vargas MI, Machi P, Lövblad KO. Imaging Clot Characteristics in Stroke and its Possible Implication on Treatment. Clin Neuroradiol 2020;30:27-35. [Crossref] [PubMed]
- Mishra SM, Dykeman J, Sajobi TT, Trivedi A, Almekhlafi M, Sohn SI, Bal S, Qazi E, Calleja A, Eesa M, Goyal M, Demchuk AM, Menon BK. Early reperfusion rates with IV tPA are determined by CTA clot characteristics. AJNR Am J Neuroradiol 2014;35:2265-72. [Crossref] [PubMed]
- Halligan S, Menu Y, Mallett S. Why did European Radiology reject my radiomic biomarker paper? How to correctly evaluate imaging biomarkers in a clinical setting. Eur Radiol 2021;31:9361-8. [Crossref] [PubMed]
- Phipps MS, Cronin CA. Management of acute ischemic stroke. BMJ 2020;368:l6983. [Crossref] [PubMed]
- Powers WJ, Rabinstein AA, Ackerson T, Adeoye OM, Bambakidis NC, Becker K, Biller J, Brown M, Demaerschalk BM, Hoh B, Jauch EC, Kidwell CS, Leslie-Mazwi TM, Ovbiagele B, Scott PA, Sheth KN, Southerland AM, Summers DV, Tirschwell DL. Guidelines for the Early Management of Patients With Acute Ischemic Stroke: 2019 Update to the 2018 Guidelines for the Early Management of Acute Ischemic Stroke: A Guideline for Healthcare Professionals From the American Heart Association/American Stroke Association. Stroke 2019;50:e344-418. [Crossref] [PubMed]
- van Voorst H, Bruggeman AAE, Yang W, Andriessen J, Welberg E, Dutra BG, et al. Thrombus radiomics in patients with anterior circulation acute ischemic stroke undergoing endovascular treatment. J Neurointerv Surg 2022. [Epub ahead of print]. pii: neurintsurg-2022-019085. doi:
10.1136/jnis-2022-019085 .10.1136/jnis-2022-019085 - Mayerhoefer ME, Materka A, Langs G, Häggström I, Szczypiński P, Gibbs P, Cook G. Introduction to Radiomics. J Nucl Med 2020;61:488-95. [Crossref] [PubMed]
- Thibault G, Angulo J, Meyer F. Advanced statistical matrices for texture characterization: application to cell classification. IEEE Trans Biomed Eng 2014;61:630-7. [Crossref] [PubMed]
- Tian Q, Yan LF, Zhang X, Zhang X, Hu YC, Han Y, Liu ZC, Nan HY, Sun Q, Sun YZ, Yang Y, Yu Y, Zhang J, Hu B, Xiao G, Chen P, Tian S, Xu J, Wang W, Cui GB. Radiomics strategy for glioma grading using texture features from multiparametric MRI. J Magn Reson Imaging 2018;48:1518-28. [Crossref] [PubMed]
- Miloš M, Marinković V. Assessing the sensitivity of the artificial neural network to experimental noise: a case study. FME Trans 2010;38:189-95.