Improved image quality in transcatheter aortic valve implantation planning CT using deep learning-based image reconstruction
Introduction
Transcatheter aortic valve implantation (TAVI) has emerged as the predominant treatment for patients with symptomatic severe aortic valve stenosis. The technique was originally developed for patients who are ineligible for open aortic valve replacement due to comorbidities (1). Subsequently, the safety, efficacy and non-inferiority to open surgery has also been demonstrated in operable patients at high (2), intermediate (3) and even low risk (4).
Computed tomographic angiography (CTA) of the heart, aorta and iliac arteries down to the groin is the standard imaging method for pre-interventional evaluation and planning of the TAVI procedure (5). The CT examination provides detailed information about the size and geometry of the aortic annulus. The annulus is a virtual ring formed from the most basal attachment points of all three aortic valve cusps. Measurement of the aortic annulus is essential for selecting the appropriate prosthesis size. Established measurements of the aortic annulus include minimum and maximum diameters as well as effective diameters derived from circumference and area (6). Appropriate sizing of the prosthesis is essential to reduce the rate of paravalvular regurgitation and avoid annular rupture (7,8). CT also allows optimal assessment of the morphology and calcifications of the aortic valve as well as the aortic root including the distance of the coronary ostia from the aortic annulus. These parameters guide selection of the optimal prosthesis model. Furthermore, suitability of the aorto-iliac access is assessed on CT to avoid complications during transfemoral delivery of the prosthesis.
Optimized CT scanning protocols for pre-interventional CT imaging are of major importance for optimal sizing of the valve prosthesis and technical success of the TAVI procedure. Various iterative image reconstruction algorithms have been developed to reduce image noise, increase image quality (9). Recently, deep learning-based image reconstruction (DLIR) was developed applying convolutional neural networks to reduce noise in CT images (10-12). Previous studies have demonstrated great potential of DLIR in cardiovascular CT including coronary CT angiography (13-15) and CT of the aorta (16) with substantial improvements in image quality beyond what is achieved with hybrid iterative reconstruction methods.
Several vendors have implemented model-based iterative reconstruction methods, which use information about the acquisition process, system geometry and image statistics for improving image quality (9). Routine application of this approach has been hampered by very long reconstruction times. Image reconstruction time is fastest for hybrid iterative reconstruction methods [such as adaptive statistical iterative reconstruction V (ASIR-V), approx.. 25 frames/s], followed by DLIR methods (approx. 10 frames/s), whereas model-based iterative reconstruction requires significantly more reconstruction time (approx. 0.2–0.5 frames/s) (9,16,17).
Our study aimed to investigate the effect of a novel commercial DLIR algorithm on objective and subjective image quality of TAVI-planning CT angiography and to compare it with a state-of-the-art advanced iterative reconstruction algorithm as the reference standard.
Methods
Patient selection and study design
In this retrospective singe-center cohort study included 50 patients. Eligible individuals were identified by searching the picture archiving and communications system (PACS) of our radiology department. Patients were included if they underwent clinically indicated CT angiography for TAVI evaluation performed in our department between May and August 2020. Non-diagnostic examinations and differing clinical indications were excluded.
Ethical approval and informed consent
The study was approved by the responsible Institutional Review Board (Ethical committee, Rostock University Medical Center) with waiver of informed consent and was conducted in accordance with the Declaration of Helsinki (as revised in 2013).
CT acquisition protocol
CT acquisition parameters are summarized in Table 1. All patients were examined on a 256-slice CT system (Revolution CT, GE Healthcare) with a gantry rotation time of 0.28 s/rotation, tube voltage 100 kV, tube current modulation (range, 120–570 mA) with a reference noise index of 20 for the thorax and 30 for the abdomen. The scan range covered the entire thorax (including the subclavian arteries) in electrocardiogram-gated (ECG-gated) axial acquisition and the abdominal aorta and iliac arteries down to the groin in non-ECG-gated helical acquisition mode. Prospective ECG-triggering was used with the acquisition window in an end-systolic phase (centered at 300 ms after the R-wave), since end-systole is the recommended phase for measuring the aortic annulus in TAVI planning (18).
Table 1
Parameter | Value |
---|---|
Acquisition parameters | |
Tube voltage (kV) | 100 |
Tube current | Tube current modulation |
Reference noise index | 20 (chest)/30 (abdomen) |
ECG-triggering | Chest only, 300 ms after R-wave |
Contrast protocol | |
Contrast volume (mL) | 80 |
Contrast concentration (mg/mL) | 400 |
Flow rate (mL/s) | 4 |
Saline chaser | 40 mL at 4 mL/s |
Reconstruction parameters | |
Reconstruction method | ASIR-V 60%; DLIR-H |
Reconstruction kernel | HD Stnd; HD Stnd/Stnd |
Slice thickness (mm) | 0.625; 0.625 |
Slice increment (mm) | 0.625; 0.625 |
Radiation metrics | |
CTDIvol chest (mGy) | 5.7 [5.5–5.7] |
CTDIvol abdomen (mGy) | 6.2 [5.9–6.3] |
DLP chest (mGy·cm) | 131 [130–132] |
DLP abdomen (mGy·cm) | 277 [247–295] |
Total DLP (mGy·cm) | 411 [385–429] |
Data is shown as median [25th–75th percentile] for DLP and CTDIvol. CT, computed tomography; ECG, electrocardiogram; ASIR-V, adaptive statistical iterative reconstruction V; DLIR-H, deep learning-based reconstruction-high strength; HD, high definition; Stnd, standard; CTDIvol, volume computed tomography dose index; DLP, dose length product.
All patients were asked to hold their breath in inspiration during the examination. 80 mL of intravenous contrast agent iomeprol (Imeron® 400 mg/mL, Bracco Imaging) were injected at a flow rate of 4 mL/s, followed by 40 mL of saline injected at the same flow rate. A bolus triggering algorithm was used, which automatically started the scan 3 s after a prespecified threshold of 150 Hounsfield units (HU) was reached in descending thoracic aorta.
Image reconstruction
Axial image series were reconstructed with a slice thickness of 0.625 mm and 0.625 mm slice interval both for adaptive statistical iterative reconstruction V [ASIR-V (60%)], which is a hybrid iterative reconstruction, and DLIR (TrueFidelity, high strength). The strength of noise reduction in ASIR-V can be adjusted in percentages by “mixing” ASIR-V with filtered back projection. We chose the 60% setting since this is our clinical standard. The DLIR algorithm allows the user to choose between three different strengths: low, medium and high. Based on previous work demonstrating that high strength DLIR provides the best imaging quality in CT angiography applications, we opted for the high strength setting (14). Reconstruction time was slower for DLIR (10 frames per second) than for ASIR-V (25 frames per second).
Radiation dose metrics
The volumetric CT dose indices (CTDIvol) as well as the dose length products (DLP) were retrieved from the dose reports stored in the picture archiving and communication system (IMPAX, AGFA Healthcare).
Analysis of objective image quality
The intravascular attenuation, image noise, signal-to-noise ratio (SNR) and contrast-to-noise ratio (CNR) were quantitatively analyzed to evaluate the objective image quality. For intravascular attenuation assessment circular regions of interest (ROIs) were placed on axial slices within the lumen of the aorta and iliac artery. Multiple ROIs were used to cover the full access route of TAVI catheters:
- Ascending aorta at the level of the main pulmonary artery;
- Descending thoracic aorta at the level of the aortic valve;
- Abdominal aorta at the level of the origin of the superior mesenteric artery;
- Right external iliac artery at the level of the femoral ligament.
Paraspinal muscles were used as attenuation reference providing homogeneous attenuation. Fat deposits were avoided. Image noise was defined as the standard deviation of the intravascular CT attenuation in the above-mentioned localizations. SNR was calculated as CT attenuation/image noise in each ROI localization. CNR was calculated as (intravascular CT attenuation − CT attenuation in the paraspinal muscle)/image noise.
For the edge sharpness evaluation, a profile line was manually drawn in the ASIR-V image and the coordinates were automatically transferred to the corresponding DLIR image (Figure 1). The value ranges of the sampled profile curves and correspond to the pixel values along the profile lines (CT values). Due to the simplicity the notation is generally used for and in following. Since both, the left and the right flank (vessel edge) of the profile curves are piecewise linear, both flanks can be defined by the respective neighboring pairs of values in the vicinity of half the height.
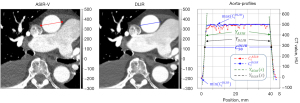
with , by the regression line
approximated such that , where is the inverse function of and the set of pixel numbers as well as the geometric position. The difference can be understood here as vessel contrast and the slope/descent of the regression line along the left and right edge of the vessel is defined as edge sharpness.
Measurements of the edge sharpness were performed on the both ASIR-V and DLIR reconstructions, by using an analyzer tool, which was implemented as python script. The used environment consists as a collection of the Microsoft Visual Code IDE (Version: 1 .7.0.2), the Python interpreter (Version: 3.10.6) with the software libraries NumPy (Version: 1.23.1), PyDicom (Version: 2.3.0), SciPy (Version: 1.9.0) and Matplotlib (Version: 3.5.2) for the visualization.
Subjective assessment of image quality
The analysis of image quality was performed independently by one radiologist and one interventional cardiologist in random order and blinded to each other’s evaluation results. Both observers viewed images in a 3D MPR viewing tool within our PACS that allowed obtaining oblique multiplanar reformations. Observers were encouraged to freely adjust window settings for optimal assessment. Since subjective image quality is task-dependent, we pre-specified the following four tasks specific for TAVI planning:
- Measuring the aortic annulus;
- Assessing the morphology and calcifications of the aortic valve;
- Assessing the distance of the coronary ostia from the annulus and;
- Assessing the suitability of the aortoiliac access route.
For each of these tasks, both observers rated the image quality (how well images were suited for performing the specific task) on a 5-point scale: 5= excellent; 4= good; 3= sufficient; 2= poor; 1= non-diagnostic.
Measurements of the aortic annulus
Measurements of the aortic annulus were performed by the radiologist on both ASIR-V and DLIR reconstructions. For this purpose, the aortic annulus was set in its true plane in the 3D MPR tool and manually contoured. The circumference and area of the annulus were recorded. Circumference-derived effective diameter was calculated as circumference/π. Area-derived effective diameter was calculated as (18).
Statistical analysis
Statistical analysis was performed with GraphPad Prism version 9.3.1 (GraphPad Software LLC). Values for objective and subjective image quality were presented as median and interquartile range (25th to 75th percentiles). Wilcoxon signed rank test for paired data was used to compare image quality parameters and measurements between ASIR-V and DLIR reconstructions. To account for multiple testing at four different anatomical locations/four different image interpretation tasks, differences were considered statistically significant at an adjusted P value of <0.0125.
Results
Patient characteristics
Patient characteristics are summarized in Table 2. TAVI planning CTA examinations from 50 consecutive patients (25 men, 25 women) with a median age of 80 years were analyzed. The median body weight was 82 kg with a median BMI of 28.0 kg/m2.
Table 2
Characteristics | All patients (N=50) | Men (N=25) | Women (N=25) |
---|---|---|---|
Age (years) | 80 [77–85] | 80 [76–84] | 81 [78–86] |
Body weight (kg) | 82 [75–92] | 85 [77–95] | 78 [62–90] |
BMI (kg/m2) | 28 [24.8–31.2] | 27.3 [24.9–29.4] | 29.4 [24.0–33.1] |
Data is shown as median [25th–75th percentile]. BMI, body mass index.
Radiation dose
Median DLP 131.3 mGy·cm (range, 130.2–131.8 mGy·cm) for the prospectively ECG-triggered thoracic part of the scan and 277.1 mGy·cm (range, 246.9–294.9 mGy·cm) for the non-ECG-gated acquisition of the abdomen and pelvis with a total median DLP of 410.5 mGy·cm (range, 384.7–428.5 mGy·cm).
Objective image quality
There were no clinically meaningful differences in intravascular attenuation between ASIR-V and DLIR reconstructions with a relative change <1% at all anatomical locations, although these minimal differences were statistically significant (Table 3 and Figure 2). DLIR significantly reduced median image noise by 29–57% at all anatomical locations (all P<0.001). Accordingly, median SNR improved by 44–133% (all P<0.001) and median CNR improved by 44–125% (all P<0.001, Table 3 and Figure 2).
Table 3
Image quality parameter | ASIR-V | DLIR | P value |
---|---|---|---|
Attenuation paraspinal muscle | 56 [52–61] | 56 [52–60] | 0.834 |
Intravascular attenuation | |||
Ascending aorta | 469 [413–533] | 470 [413–532] | <0.001 |
Thoracic descending aorta | 463 [409–504] | 466 [407–505] | 0.009 |
Abdominal aorta | 472 [418–559] | 473 [418–561] | <0.001 |
Pelvic arteries | 463 [400–508] | 466 [404–511] | <0.001 |
Intravascular image noise | |||
Ascending aorta | 51 [47–54] | 22 [20–24] | <0.001 |
Thoracic descending aorta | 50 [46–54] | 23 [21–25] | <0.001 |
Abdominal aorta | 28 [26–30] | 20 [16–23] | <0.001 |
Pelvic arteries | 26 [21–30] | 18 [15–21] | <0.001 |
Signal-to-noise ratio | |||
Ascending aorta | 9 [9–10] | 21 [19–24] | <0.001 |
Thoracic descending aorta | 9 [9–10] | 19 [18–23] | <0.001 |
Abdominal aorta | 16 [14–20] | 24 [21–27] | <0.001 |
Pelvic arteries | 18 [15–21] | 26 [20–30] | <0.001 |
Contrast-to-noise-ratio | |||
Ascending aorta | 8 [7–9] | 18 [16–21] | <0.001 |
Thoracic descending aorta | 8 [7–9] | 17 [15–20] | <0.001 |
Abdominal aorta | 14 [12–18] | 21 [18–24] | <0.001 |
Pelvic arteries | 16 [13–19] | 23 [18–27] | <0.001 |
Edge sharpness (HU/pixel) | 133 [129–138] | 132 [129–136] | 0.2856 |
Data is shown as median [25th–75th percentile]. ASIR-V, adaptive statistical iterative reconstruction V; DLIR, deep learning-based image reconstruction; HU, Hounsfield units.
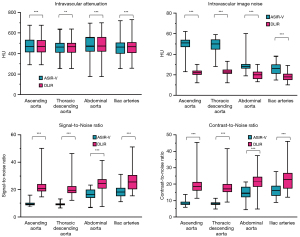
The reduction in image noise was >50% for the thoracic part of the examination (22 vs. 51 HU for the ascending aorta and 23 vs. 50 HU for the descending thoracic aorta, both P<0.001). Accordingly, the median SNR and the median CNR increased by >100% for the ascending aorta (SNR 21 vs. 9; CNR 18 vs. 8) and the descending thoracic aorta (SNR 29 vs. 9; CNR 17 vs. 8, all P<0.001). The image noise in the abdominal aorta (20 vs. 28 HU) and pelvic arteries (18 vs. 26 HU) was reduced by 29–36% at DLIR (P<0.001). SNR and CNR increased by 44–50% for the abdominal aorta and iliac arteries (P<0.001, Table 3 and Figure 2). There was no significant difference in edge sharpness between ASIR-V and DLIR reconstructions (Table 3).
Subjective image quality
Representative results of the ASIR-V and DLIR reconstruction methods are visualized in Figure 3. The results for the visual assessment of subjective image quality are summarized in Table 4. DLIR significantly improved subjective image quality for all four pre-specified TAVI-specific tasks (measuring the annulus, assessing valve morphology and calcifications, the coronary ostia, and the suitability of the aorto-iliac access route) for both the radiologist and the interventional cardiologist (p≤0.001). For the radiologist, median subjective image quality improved from “good” (grade 4) to “excellent” (grade 5) for all four tasks (P<0.001). For the interventional cardiologist, subjective image quality improved from “sufficient” (grade 3) to “good” (grade 4) for measuring the aortic annulus and assessing the morphology and calcifications of the aortic valve (P<0.001). The interventional cardiologist’s median rating for assessing the distance of the coronary ostia from the annulus and assessing the suitability of the aortoiliac access route remained “good”, but significant improvements were seen also for these tasks (P≤0.001).
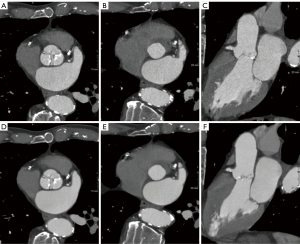
Table 4
Image quality assessment | ASIR-V | DLIR | P value |
---|---|---|---|
Reader 1 (Radiologist) | |||
Measurement annulus | 4 [3–4] | 5 [5–5] | <0.001 |
Assessment of morphology and calcifications of the aortic valve | 4 [3–4] | 5 [5–5] | <0.001 |
Distance of the coronary ostia from the annulus | 4 [4–4] | 5 [5–5] | <0.001 |
Aortoiliac access suitable for TAVI | 4 [4–4] | 5 [5–5] | <0.001 |
Reader 2 (Interventional Cardiologist) | |||
Measurement annulus | 3 [3–4] | 4 [3–5] | <0.001 |
Assessment of morphology and calcifications of the aortic valve | 3 [3–4] | 4 [3–5] | <0.001 |
Distance of the coronary ostia from the annulus | 4 [3–4] | 4 [4–5] | <0.001 |
Aortoiliac access suitable for TAVI | 4 [4–5] | 4 [4–5] | 0.001 |
Data is shown as median [25th–75th percentile]. ASIR-V, adaptive statistical iterative reconstruction V; DLIR, deep learning-based image reconstruction; TAVI, transcatheter aortic valve implantation.
Influence on measurements of the aortic annulus
There were no differences between the circumference and cross-sectional area of the aortic annulus measured on ASIR-V and DLIR reconstructions (P>0.05, Table 5). The calculated effective diameters derived from measurements of circumference and area also remained unchanged P>0.05).
Table 5
Measurements | ASIR-V | DLIR | P value |
---|---|---|---|
Circumference in mm | 76 [71–81] | 76 [71–80] | 0.345 |
Area in mm2 | 430 [362–485] | 432 [365–487] | 0.813 |
Circumference-based effective diameter (mm) | 24 [22–26] | 24 [22–26] | 0.535 |
Area-based effective diameter (mm) | 23 [21–25] | 23 [22–25] | 0.438 |
Data is shown as median [25th–75th percentile]. ASIR-V, adaptive statistical iterative reconstruction V; DLIR, deep learning-based image reconstruction.
Discussion
The number of TAVI procedures performed has increased steadily in recent years (19). This is based on improved procedural technology, increasing experience of the interventional cardiology community, as well as low mortality and complication rates with simultaneously shortened hospital stay and recovery time compared to operative care (20). Since CT has become the standard imaging modality for TAVI planning, the increasing number of TAVI procedures goes along with an equivalent increase in the number of CT examinations performed for planning the procedure. These trends make it even more important to optimize CT protocols for TAVI planning CT. In particularly, limiting contrast volume is highly relevant in TAVI candidates since this is an elderly population with a high prevalence of renal impairment.
DLIR, which uses deep learning to substantially reduce image noise, is one of the most recent innovations in CT technology (10). DLIR has been shown to substantially improve image quality in cardiovascular CT applications including coronary CT angiography (13-15) and CT of the aorta (16). Gains in SNR and CNR were much greater than with hybrid iterative image reconstruction, the current standard of clinical care. Beyond cardiovascular imaging, DLIR was shown to be valuable in a variety of CT applications and body regions including low-dose chest CT (21), abdominal CT (22), pediatric head CT (23) and high-resolution computed tomography angiography in Moyamoya disease (12).
The potential of DLIR to save radiation dose was shown in a phantom study by Lee et al. (24). The colleagues investigated the effects of different tube voltages and tube currents on the image quality of abdominal CT, reconstructed with filtered back projection (FBP), ASIR-V and DLIR. They were able to reduce the radiation dose with the DLIR algorithms by 66% to 68% compared to the reference image, while the image quality remained comparable. In our CT protocol, radiation exposure was already quite low with a median DLP of 410.5 mGy·cm. A cross-sectional observational study in the UK published in 2020 reported a median DLP of 675 mGy·cm for CT studies prior to TAVI (25). Considering that most TAVI candidates are multimorbid septuagenarians or octogenarians (median age was 80 years in our cohort), further reductions in radiation exposure in this population are unlikely to be of any benefit for the patients. However, in the TAVI population, gains in CNR and SNR could be invested in reducing the required volume of contrast agent.
Patients planned for TAVI have severe, symptomatic aortic valve stenosis and often multiple comorbidities (26,27). They often suffer from impaired kidney function with an increased risk of contrast medium-induced nephropathy (28). To minimize this risk, it is important to use a low contrast dose. On the other hand, sufficient contrast for the aortic root and aortoiliac access pathway must be ensured to allow selection and sizing or the prosthesis and planning of the procedure. TAVI protocols with up to 120 mL of contrast agent are described in the literature (29,30). In our protocol for pre-TAVI CT, a fixed volume of 80 mL of contrast agent (iodine concentration of 400 mg/mL) was used. A study by Kok and colleagues investigated the possibility of saving even more contrast medium (31). For this, they used a BMI-adapted scan and injection protocol: group 1 with 40 mL of contrast medium (300 mg/mL) and 70 kV or group 2 with 53 mL contrast medium with 80 kV. It could be shown that despite the reduced amount of contrast medium and low kV protocol, sufficient image quality could be achieved. Here, the DLIR algorithm could be useful to ensure sufficient image quality at low contrast agent levels and kV values, which should be investigated in further studies.
The subjective quality of medical imaging critically depends on the diagnostic tasks that are to be performed on these images. Therefore, rather than rating overall subjective image quality, we pre-specified four tasks specific for TAVI planning and asked observers to rate how well images were suited for performing these specific tasks. We chose one radiologist and one interventional cardiologist as readers, since it varies between institutions whether the measurements specific for planning the TAVI procedure are performed by radiologists, interventional cardiologists, or both. Evaluation of the aorta and iliac arteries is important as the transfemoral approach is the preferred vascular access for the TAVI procedure. Significant stenosis and/or kinking in the transfemoral access route may require subclavian access or even a transapical approach. Measuring the aortic annulus, assessing the morphology and calcifications of the aortic valve and measuring the distance of the coronary ostia from the annulus are of great importance to the interventional cardiologist for the correct choice of prosthesis type and size (18).
We found that DLIR significantly improves objective image quality at all anatomical levels without influence on the measurement of the annulus itself. In the evaluation of subjective image quality, significant improvements were seen for all tasks specific for TAVI planning both for the radiologist and the interventional cardiologist. Readers noted that the edges appear sharper at organ interfaces, especially at the junction between different arterial contrast-enhancing structures. Metal artefacts and blooming artefacts due to the strong calcifications tend to appear attenuated. It is worth mentioning that reducing image noise by DLIR does of course not eliminate all potential limitations of image quality. Motion artefacts caused by breathing or cardiac motion were seen in a few patients in our cohort and were equally problematic with and without DLIR.
Our study has several limitations. Our analyses were conducted retrospectively in a single institution using the CT system and the DLIR algorithm of a specific vendor. The study population of 50 patients was relatively small but proved sufficient given the large observed differences in image quality and the paired sample design. No comparison was made with filtered back projection or model-based iterative reconstruction, as our aim was to compare DLIR with hybrid iterative reconstruction as the current standard of clinical care. Since hybrid iterative reconstruction methods are a combination of analytical and iterative methods, iterative image improvements are made in the image and/or raw domain after analytical image generation. They can be implemented into the reconstruction process and are less time consuming than model-based iterative reconstruction. Model-based iterative reconstruction methods use information about the acquisition process, system geometry and image statistics to implement a model for the improving the image quality (16). Due to long reconstruction times of model-based iterative reconstruction, hybrid iterative reconstruction methods, such as ASIR-V, are the state of the art in clinical usage.
Lastly, we did not investigate the effect of various strength settings of the DLIR algorithm. Rather, we only used the highest strength DLIR since this has been shown to lead to the best image quality in cardiovascular CT (14).
Conclusions
DLIR significantly improves objective image quality in pre-TAVI CT compared to a state-of-the-art iterative reconstruction without affecting measurements of the aortic annulus. Both radiologists and interventional cardiologist prefer DLIR images for TAVI planning. This may provide an opportunity to further reduce contrast medium volume in this population.
Acknowledgments
Funding: This study was supported by a research grant from GE Healthcare. The authors guarantee that they had full sovereignty of their data.
Footnote
Conflicts of Interest: All authors have completed the ICMJE uniform disclosure form (available at https://qims.amegroups.com/article/view/10.21037/qims-22-639/coif). FGM reports that he was supported by a research grant from GE Healthcare. The other authors have no conflicts of interest to declare.
Ethical Statement: The authors are accountable for all aspects of the work in ensuring that questions related to the accuracy or integrity of any part of the work are appropriately investigated and resolved. The study was approved by the responsible Institutional Review Board (Ethical committee, Rostock University Medical Center) with waiver of informed consent and was conducted in accordance with the Declaration of Helsinki (as revised in 2013).
Open Access Statement: This is an Open Access article distributed in accordance with the Creative Commons Attribution-NonCommercial-NoDerivs 4.0 International License (CC BY-NC-ND 4.0), which permits the non-commercial replication and distribution of the article with the strict proviso that no changes or edits are made and the original work is properly cited (including links to both the formal publication through the relevant DOI and the license). See: https://creativecommons.org/licenses/by-nc-nd/4.0/.
References
- Leon MB, Smith CR, Mack M, Miller DC, Moses JW, Svensson LG, et al. Transcatheter aortic-valve implantation for aortic stenosis in patients who cannot undergo surgery. N Engl J Med 2010;363:1597-607. [Crossref] [PubMed]
- Smith CR, Leon MB, Mack MJ, Miller DC, Moses JW, Svensson LG, et al. Transcatheter versus surgical aortic-valve replacement in high-risk patients. N Engl J Med 2011;364:2187-98. [Crossref] [PubMed]
- Leon MB, Smith CR, Mack MJ, Makkar RR, Svensson LG, Kodali SK, et al. Transcatheter or Surgical Aortic-Valve Replacement in Intermediate-Risk Patients. N Engl J Med 2016;374:1609-20. [Crossref] [PubMed]
- Mack MJ, Leon MB, Thourani VH, Makkar R, Kodali SK, Russo M, et al. Transcatheter Aortic-Valve Replacement with a Balloon-Expandable Valve in Low-Risk Patients. N Engl J Med 2019;380:1695-705. [Crossref] [PubMed]
- Achenbach S, Delgado V, Hausleiter J, Schoenhagen P, Min JK, Leipsic JA. SCCT expert consensus document on computed tomography imaging before transcatheter aortic valve implantation (TAVI)/transcatheter aortic valve replacement (TAVR). J Cardiovasc Comput Tomogr 2012;6:366-80. [Crossref] [PubMed]
- Schultz CJ, Moelker A, Piazza N, Tzikas A, Otten A, Nuis RJ, Neefjes LA, van Geuns RJ, de Feyter P, Krestin G, Serruys PW, de Jaegere PP. Three dimensional evaluation of the aortic annulus using multislice computer tomography: are manufacturer's guidelines for sizing for percutaneous aortic valve replacement helpful? Eur Heart J 2010;31:849-56. [Crossref] [PubMed]
- Delgado V, Ng AC, van de Veire NR, van der Kley F, Schuijf JD, Tops LF, de Weger A, Tavilla G, de Roos A, Kroft LJ, Schalij MJ, Bax JJ. Transcatheter aortic valve implantation: role of multi-detector row computed tomography to evaluate prosthesis positioning and deployment in relation to valve function. Eur Heart J 2010;31:1114-23. [Crossref] [PubMed]
- Willson AB, Webb JG, Labounty TM, Achenbach S, Moss R, Wheeler M, Thompson C, Min JK, Gurvitch R, Norgaard BL, Hague CJ, Toggweiler S, Binder R, Freeman M, Poulter R, Poulsen S, Wood DA, Leipsic J. 3-dimensional aortic annular assessment by multidetector computed tomography predicts moderate or severe paravalvular regurgitation after transcatheter aortic valve replacement: a multicenter retrospective analysis. J Am Coll Cardiol 2012;59:1287-94. [Crossref] [PubMed]
- Geyer LL, Schoepf UJ, Meinel FG, Nance JW Jr, Bastarrika G, Leipsic JA, Paul NS, Rengo M, Laghi A, De Cecco CN. State of the Art: Iterative CT Reconstruction Techniques. Radiology 2015;276:339-57. [Crossref] [PubMed]
- Arndt C, Güttler F, Heinrich A, Bürckenmeyer F, Diamantis I, Teichgräber U. Deep Learning CT Image Reconstruction in Clinical Practice. Rofo 2021;193:252-61. [Crossref] [PubMed]
- Bernard A, Comby PO, Lemogne B, Haioun K, Ricolfi F, Chevallier O, Loffroy R. Deep learning reconstruction versus iterative reconstruction for cardiac CT angiography in a stroke imaging protocol: reduced radiation dose and improved image quality. Quant Imaging Med Surg 2021;11:392-401. [Crossref] [PubMed]
- Fukushima Y, Fushimi Y, Funaki T, Sakata A, Hinoda T, Nakajima S, Sakamoto R, Yoshida K, Miyamoto S, Nakamoto Y. Evaluation of moyamoya disease in CT angiography using ultra-high-resolution computed tomography: Application of deep learning reconstruction. Eur J Radiol 2022;151:110294. [Crossref] [PubMed]
- Hong JH, Park EA, Lee W, Ahn C, Kim JH. Incremental Image Noise Reduction in Coronary CT Angiography Using a Deep Learning-Based Technique with Iterative Reconstruction. Korean J Radiol 2020;21:1165-77. [Crossref] [PubMed]
- Wang M, Fan J, Shi X, Qin L, Yan F, Yang W. A deep-learning reconstruction algorithm that improves the image quality of low-tube-voltage coronary CT angiography. Eur J Radiol 2022;146:110070. [Crossref] [PubMed]
- Tatsugami F, Higaki T, Nakamura Y, Yu Z, Zhou J, Lu Y, Fujioka C, Kitagawa T, Kihara Y, Iida M, Awai K. Deep learning-based image restoration algorithm for coronary CT angiography. Eur Radiol 2019;29:5322-9. [Crossref] [PubMed]
- Heinrich A, Streckenbach F, Beller E, Groß J, Weber MA, Meinel FG. Deep Learning-Based Image Reconstruction for CT Angiography of the Aorta. Diagnostics (Basel) 2021;11:2037. [Crossref] [PubMed]
- Nelson RC, Feuerlein S, Boll DT. New iterative reconstruction techniques for cardiovascular computed tomography: how do they work, and what are the advantages and disadvantages? J Cardiovasc Comput Tomogr 2011;5:286-92. [Crossref] [PubMed]
- Blanke P, Schoepf UJ, Leipsic JA. CT in transcatheter aortic valve replacement. Radiology 2013;269:650-69. [Crossref] [PubMed]
- Carroll JD, Mack MJ, Vemulapalli S, Herrmann HC, Gleason TG, Hanzel G, Deeb GM, Thourani VH, Cohen DJ, Desai N, Kirtane AJ, Fitzgerald S, Michaels J, Krohn C, Masoudi FA, Brindis RG, Bavaria JE. STS-ACC TVT Registry of Transcatheter Aortic Valve Replacement. J Am Coll Cardiol 2020;76:2492-516. [Crossref] [PubMed]
- Otto CM. Informed Shared Decisions for Patients with Aortic Stenosis. N Engl J Med 2019;380:1769-70. [Crossref] [PubMed]
- Kim JH, Yoon HJ, Lee E, Kim I, Cha YK, Bak SH. Validation of Deep-Learning Image Reconstruction for Low-Dose Chest Computed Tomography Scan: Emphasis on Image Quality and Noise. Korean J Radiol 2021;22:131-8. [Crossref] [PubMed]
- Njølstad T, Schulz A, Godt JC, Brøgger HM, Johansen CK, Andersen HK, Martinsen ACT. Improved image quality in abdominal computed tomography reconstructed with a novel Deep Learning Image Reconstruction technique - initial clinical experience. Acta Radiol Open 2021;10:20584601211008391. [Crossref] [PubMed]
- Sun J, Li H, Wang B, Li J, Li M, Zhou Z, Peng Y. Application of a deep learning image reconstruction (DLIR) algorithm in head CT imaging for children to improve image quality and lesion detection. BMC Med Imaging 2021;21:108. [Crossref] [PubMed]
- Lee JE, Choi SY, Hwang JA, Lim S, Lee MH, Yi BH, Cha JG. The potential for reduced radiation dose from deep learning-based CT image reconstruction: A comparison with filtered back projection and hybrid iterative reconstruction using a phantom. Medicine (Baltimore) 2021;100:e25814. [Crossref] [PubMed]
- Harries I, Weir-McCall JR, Williams MC, Shambrook J, Roditi G, Bull R, Morgan-Hughes GJ, Nicol ED, Moss AJ. CT imaging prior to transcatheter aortic valve implantation in the UK. Open Heart 2020;7:e001233. [Crossref] [PubMed]
- Webb JG, Pasupati S, Humphries K, Thompson C, Altwegg L, Moss R, Sinhal A, Carere RG, Munt B, Ricci D, Ye J, Cheung A, Lichtenstein SV. Percutaneous transarterial aortic valve replacement in selected high-risk patients with aortic stenosis. Circulation 2007;116:755-63. [Crossref] [PubMed]
- Iung B, Cachier A, Baron G, Messika-Zeitoun D, Delahaye F, Tornos P, Gohlke-Bärwolf C, Boersma E, Ravaud P, Vahanian A. Decision-making in elderly patients with severe aortic stenosis: why are so many denied surgery? Eur Heart J 2005;26:2714-20. [Crossref] [PubMed]
- Yamamoto M, Hayashida K, Mouillet G, Chevalier B, Meguro K, Watanabe Y, Dubois-Rande JL, Morice MC, Lefèvre T, Teiger E. Renal function-based contrast dosing predicts acute kidney injury following transcatheter aortic valve implantation. JACC Cardiovasc Interv 2013;6:479-86. [Crossref] [PubMed]
- Gurvitch R, Webb JG, Yuan R, Johnson M, Hague C, Willson AB, Toggweiler S, Wood DA, Ye J, Moss R, Thompson CR, Achenbach S, Min JK, Labounty TM, Cury R, Leipsic J. Aortic annulus diameter determination by multidetector computed tomography: reproducibility, applicability, and implications for transcatheter aortic valve implantation. JACC Cardiovasc Interv 2011;4:1235-45. [Crossref] [PubMed]
- Jilaihawi H, Kashif M, Fontana G, Furugen A, Shiota T, Friede G, Makhija R, Doctor N, Leon MB, Makkar RR. Cross-sectional computed tomographic assessment improves accuracy of aortic annular sizing for transcatheter aortic valve replacement and reduces the incidence of paravalvular aortic regurgitation. J Am Coll Cardiol 2012;59:1275-86. [Crossref] [PubMed]
- Kok M, Turek J, Mihl C, Reinartz SD, Gohmann RF, Nijssen EC, Kats S, van Ommen VG, Kietselaer BL, Wildberger JE, Das M. Low contrast media volume in pre-TAVI CT examinations. Eur Radiol 2016;26:2426-35. [Crossref] [PubMed]