A radiomics model based on aortic computed tomography angiography: the impact on predicting the prognosis of patients with aortic intramural hematoma (IMH)
Introduction
Aortic intramural hematoma (IMH) is a type of acute aortic syndrome characterized by the absence of an obvious intimal defect or direct communication between the true and false lumens (1). It remains unclear if an IMH is caused by vasa vasorum rupture in the aortic wall or by small intimal tears (2). Several imaging studies have demonstrated subtle intimal tears in patients with IMH (3), with other research showing these intimal tears can be confirmed by surgeries (4).
Computed tomography angiography (CTA) plays an important role of high diagnostic performance in the initial diagnosis and follow-up of patients. The prognosis of IMH is unpredictable. Although stabilization or regression are the outcomes of many patients with IMH, progression to aortic dissection (AD), aneurysm, and even rupture also frequently occur in these patients (5). Management of IMH is further dependent on several morphologic features that may be recognized with CTA imaging (6). Currently, the risk stratification of patients with acute IMH is mainly based on their symptoms and imaging characteristics. However, its efficacy in identifying patients with poor prognoses is not satisfactory.
As an advanced image analysis technique, the radiomics-based method has been extensively studied in the differential diagnosis and prognosis of oncologic patients (7). It has also been proposed as one of the investigative tools in cardiovascular imaging research, including for identifying coronary plaques (8,9), characterizing perivascular fat in cardiac risk prediction (10), exploring the pathophysiology of atherosclerosis (11), and predicting acute coronary syndrome (12). CT-based radiomics was also demonstrated to be a screening tool for AD (13,14). However, evidence for the value of radiomics methods for the prediction of prognosis in patients with IMH is lacking.
Therefore, this study aimed to investigate the value of CT-based radiomics in predicting the natural history of patients with IMH. The radiomics method was further combined with traditional clinical factors to evaluate whether its predictive performance could be improved. We present the following article in accordance with the TRIPOD reporting checklist (available at https://qims.amegroups.com/article/view/10.21037/qims-22-480/rc).
Methods
Patients
The study was conducted in accordance with the Declaration of Helsinki (as revised in 2013). The study was approved by the local institutional ethics committee (Beijing Anzhen Hospital Ethics Committee), and individual consent for this retrospective analysis was waived. This retrospective analysis of anonymous data was approved by the institutional review board of Beijing Anzhen Hospital. The entire cohort dataset was obtained by reviewing medical records at Beijing Anzhen Hospital between August 2015 and December 2020 to select cases diagnosed as IMH. Patients were included: if (I) data were obtained fewer than 14 days from onset, (II) aortic CTA was performed in Beijing Anzhen Hospital during the acute stage, and (III) the initial treatment was medical therapy. Patients were excluded from the current study if (I) they were aged below 18 years; (II) their diagnosis was complicated with AD, aortic aneurysm, arteritis, or Marfan syndrome; (III) they had traumatic or iatrogenic IMH; (IV) they were not followed up; and (V) their clinical data were incomplete or contained poor imaging quality. The diagnostic criteria of IMH were aortic wall thickening greater than 5mm, a round or crescent shape, and no contrast-enhanced false lumen (1). Finally, 120 patients selected from cases fulfilled the criteria and were randomly divided at a ratio of 7:3 into a training cohort (n=84) and a validation cohort (n=36). Figure 1 shows the patient recruitment pathway. Patients were hospitalized and underwent continuous monitoring of vital signs with medication to control their heart rate, hypertension, and other symptoms. The median follow-up duration was 232 days (range, 32–1,346 days), and aortic CTA follow-up was completed in all patients. Progression of these patients was classified as follows: (I) destabilization, referring to deterioration in the aortic condition, including significant increases in the thickness of the IMH, the progression of IMH to a penetrating aortic ulcer (PAU), the progression to AD, or rupture; or (II) stabilization, referring to an unchanged appearance or decrease in the size or disappearance of the IMH (15).
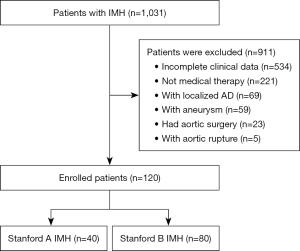
CT image acquisition
An electrocardiography-gated contrast-enhanced multidetector CT scan was performed with a 256-slice CT scanner (Revolution; GE Healthcare, Milwaukee, WI, USA), a 320-slice CT scanner (Aquilion One; Toshiba Medical Systems, Tokyo, Japan), or a second-generation 128-slice dual-source CT scanner (Somatom Definition Flash; Siemens Healthcare, Erlangen, Germany). The contrast material was injected into an antecubital vein (60–90 mL at 3–5 mL/s). Scanning parameters were 100–120 kVp and 300–680 mAs. The density of the ascending aorta was monitored after the injection of the contrast medium. CT data acquisition was initiated when the density in the ascending aorta was greater than 200 Hounsfield units. The axial image reconstruction parameters were a 512×512 matrix and a slice thickness and interval of 1 mm. Axial and multiplanar reformations (MPR), maximum intensity projection, and volume-rendered images of the aorta were produced.
Evaluation of CT characteristics
All the clinical data, including as age and sex, were obtained by reviewing the medical records, with blinding of participants and personnel. All CT images were retrieved from the institutional picture archiving and communication systems (PACS) and saved in Digital Imaging and Communications in Medicine (DICOM) format. All CT scans were reviewed by 2 radiologists with 5 (Ding; reader 1) and 8 years (Zhao; reader 2) of experience in aortic CTA. In cases of disagreement, images were reviewed together by both readers to adjudicate the result. They recorded the Stanford type, maximal hematoma thickness (MHT), maximal aortic diameter (MAD), ulcer-like projection (ULP), intramural blood pool (IBP), aortic atherosclerosis, pleural effusion, and pericardial effusion. MHT and MAD were measured at the thickest area of the hematoma, and ULP was defined as a localized blood-filled pouch protruding into the hematoma of the aortic wall, with a wide communicating orifice >3 mm (16). ULP is a localized contrast medium-filled pool inside IMH appearing on postcontrast CT images with no communication between the contrast medium-filled pool and the true lumen. Detailed information is shown in Figure S1.
Radiomics analysis
The region of interest (ROI) was manually drawn on the transverse axial CT images using the ITK-SNAP software (Version 3.8.0, www.itksnap.org) for segmentation. To correct the variability from parameters related to voxel size, images were resampled to 1 mm × 1 mm × 1 mm using linear interpolation (17). The DICOM format file was saved in neuroimaging informatics technology initiative (NIFTI) format. First, the aorta and the hematoma were labeled as “Label1”, and then the transparency of Label1 was adjusted so that the lumen of the aorta could be clearly displayed. The aortic lumen was covered by Label2 on the basis of Label1, so every sample with Label1 on it covered with Label2. Finally, the overlap area of Label1 and Label2 was removed, and only the hematoma outside the aortic lumen was preserved (Figure S2). After segmentation was completed on the transverse axial images, the target region was also checked and corrected on the coronal and sagittal images. The segmentation was accomplished by reader 1. To assess the segmentation availability, the image segmentation was examined by reader 2. If the ROI was questioned and was resegmented after the 2 radiologists reached a consensus.
Radiomic features were extracted using Artificial Intelligence Kit Software version 3.2.0 (GE Healthcare) back ended with Pyradiomics version 3.0.1 (https://pyradiomics.readthedocs.io/en/latest/).
The extracted 1,316 radiomics features were categorized into 3 groups: (I) first-order features, including 18 intensity statistics and 14 shape features; (II) 75 multidimensional texture features, including 24 gray level co-occurrence matrix (GLCM), 16 gray level size zone matrix (GLSZM), 16 gray level run length matrix (GLRLM), 14 gray level dependence matrix (GLDM), and 5 neighboring gray tone difference matrix (NGTDM) features; and (III) 1,209 transformed first-order and textural features, including 744 wavelet-decomposed features in low (L) or high (H) frequency channels (LHL, LLH, HHH, HLH, HLL, HHL, LHH, and LLL), 186 Laplacian of Gaussian (LoG) filtered features with a sigma of 2.0 and 3.0 mm, and 279 3D local binary pattern (LBP) filtered texture features with 2-level spherical harmonics and an icosphere with a radius of 1 and 1 subdivision.
First, the intraclass correlation coefficient (ICC) values of both intra- and interobserver agreement analyses were calculated. Second, the univariate difference analysis between the destabilization and nonworsening groups was conducted using an independent 2-sample t-test or Mann-Whitney test, and the features with a significant difference (P<0.05) were retained. Third, correlation analysis with a cut-off value of 0.8 was carried out to eliminate redundant and irrelevant features. Finally, the least absolute shrinkage and selection operator (LASSO) logistic regression with maximum area under the curve (AUC) criteria was conducted to select the optimized subset of features to construct the final model.
Six machine learning-based algorithms, including random forest (RF), K-nearest neighbor (KNN), Gaussian Naive Bayes, decision tree, logistic regression, and support vector machine (SVM) were used to establish radiomics models based on selected features. SVM used 2 different kernel functions: linear kernel function and radial basis function. Area under the receiver operator characteristic (ROC) curve (AUC) was used to validate the performance of the developed radiomics models in both the training and validation groups. The best predictive model was determined, and the probability output by the model was recorded as a radiomics score (Radscore).
Predictive nomogram building and diagnostic performance validation
The independent risk factors among all clinical variables were assessed using univariate and multivariate logistic regression analyses. We used the multivariable logistic regression analysis to construct the clinical model based on the clinical factors with P values <0.05 in the univariate analyses. A radiomics nomogram combining the radiomics signature (Radscore) and clinical risk factors was finally developed. The ROC analysis was conducted to evaluate the model’s discrimination performance, and DeLong’s test was used to compare whether the model’s AUC was different between the paired models. The calibration of the combined nomogram was evaluated with calibration curve analysis and the Hosmer-Lemeshow test. Decision curve analysis was conducted to estimate the clinical usefulness of the nomogram by quantifying the net benefits based on the threshold probabilities.
Statistical analysis
All statistical analyses for the present study were performed with R (version 4.1.1; www.r-project.org; The R Project for Statistical Computing). The statistical significance for all 2-sided tests was set at P<0.05. The continuous variables with normal distribution are described as mean ± standard deviation (SD), and the statistical difference between 2 groups was tested using an independent 2-sample t-test. The continuous variables without normal distribution are described as median ± interquartile range (IQR), and the statistical difference between 2 groups was tested using the Mann-Whitney test. The statistical difference for categorical variables was tested using the chi-square test or Fisher exact test. ICC was used to quantify interobserver and intraobserver reproducibility. The cut-off value for the ROC curves was determined at the maximized Youden index in the training group and applied in the validation dataset, which was used to derive the sensitivity, specificity, and accuracy.
Results
Clinical characteristics
The 120 patients (90 males, 30 females; mean age 57.96±9.67 years; range 34–79 years) included 60 cases of destabilization and 60 cases of stabilized IMH. All patients were randomly assigned to a training cohort (n=84) or a validation cohort (n=36). Table 1 shows the clinical characteristics of patients in the training cohort and validation cohort. There were no significant differences in clinical features between the training and validation groups except Stanford type and pericardial effusion (Figure S3 shows an IMH evolution to dissection over a 110-day period).
Table 1
Feature | Training cohort, n=84 | Validation cohort, n=36 | t/χ2/U | P value |
---|---|---|---|---|
Destabilization, n (%) | 42 (50.00) | 18 (50.00) | 0 | 1 |
Stanford A, n (%) | 23 (27.38) | 17 (47.22) | 4.46 | 0.035 |
Age (years) | 57.48±9.89a | 59.14±9.18 | –0.862 | 0.391 |
Male, n (%) | 66 (78.57) | 24 (66.67) | 1.91 | 0.168 |
BMI (kg/m2) | 25.0±2.64 | 25.87±3.13 | –1.548 | 0.124 |
Hypertension, n (%) | 67 (79.76) | 29 (80.56) | 0.01 | 0.921 |
Hyperlipidemia, n (%) | 19 (22.62) | 7 (19.44) | 0.15 | 0.70 |
Diabetes, n (%) | 8 (9.52) | 2 (5.56) | 0.515 | 0.721 |
Smoking, n (%) | 53 (63.10) | 20 (55.56) | 0.601 | 0.438 |
CTA images | ||||
MHT (mm) | 10.785 (8.353,13.26)b | 10.55 (8.828,13.408) | 1453.5 | 0.738 |
MAD (mm) | 38.035 (35.108,45.105) | 40.66 (36.623,48.333) | 1272 | 0.169 |
Branch involvement, n (%) | 40 (47.62) | 24 (66.67) | 3.673 | 0.055 |
ULP, n (%) | 57 (67.86) | 21 (58.33) | 1.005 | 0.316 |
IBP, n (%) | 16 (19.05) | 5 (13.89) | 0.465 | 0.496 |
Pericardial effusion, n (%) | 5 (5.95) | 10 (27.78) | 10.884 | 0.001 |
Pleural effusion, n (%) | 18 (21.43) | 10 (27.78) | 0.568 | 0.451 |
Atherosclerosis, n (%) | 47 (55.95) | 20 (55.56) | 0.002 | 0.968 |
Lab examination | ||||
D-dimer >500 μg/L | 48 (57.14) | 23 (63.89) | 0.475 | 0.491 |
WBC (×109/L) | 10.92±3.42 | 11.05±3.33 | 0.199 | 0.843 |
Two-sample t-test or Mann-Whitney test was used for continuous variables; χ2 test or Fisher exact test was used for categorical variables. a, continuous variables with a normal distribution are described as mean ± standard deviation (SD); b, the continuous variables without a normal distribution are described as median ± interquartile range (IQR). BMI, body mass index; CTA, computed tomography angiography; MHT, maximal hematoma thickness; MAD, maximal aortic diameter; ULP, ulcer-like projection; IBP, intramural blood pool; WBC, white blood cell.
Branch involvement, ULP, and white blood cell (WBC) count were identified as clinical risk factors during univariate logistic regression analysis and further involved in multivariate logistic regression analysis (Table 2). A clinical model based on these 3 features was developed, and the AUC was 0.715 (95% CI, 0.602–0.827) in the training cohort and 0.596 (95% CI, 0.413–0.796) in the validation cohort.
Table 2
Feature | Univariate | Multivariate | |||
---|---|---|---|---|---|
Odds ratio (95% CI) | P value | Odds ratio (95% CI) | P value | ||
Stanford A | 1.434 (0.548–3.841) | 0.464 | |||
Age (years) | 1.02 (0.976–1.067) | 0.376 | |||
Male | 1.328 (0.466–3.884) | 0.595 | |||
BMI ≥25 kg/m2 | 0.417 (0.17–0.993) | 0.051 | |||
Hypertension | 0.863 (0.291–2.52) | 0.786 | |||
Diabetes | 1.757 (0.402–9.054) | 0.462 | |||
Hyperlipidemia | 2 (0.712–5.987) | 0.197 | |||
Smoking | 1.108 (0.455–2.706) | 0.821 | |||
CTA | |||||
Branch involvement | 2.647 (1.109–6.52) | 0.031 | 2.394 (0.931–6.338) | 0.072 | |
ULP | 3.511 (1.353–9.794) | 0.012 | 2.471 (0.876–7.274) | 0.091 | |
IBP | 1 (0.332–3.016) | >0.999 | |||
MAD | 0.991 (0.934–1.051) | 0.767 | |||
MHT | 1.03 (0.898–1.184) | 0.673 | |||
Pericardial effusion | 4.316 (0.605–86.453) | 0.2 | |||
Pleural effusion | 1.328 (0.466–3.884) | 0.595 | |||
Atherosclerosis | 1.337 (0.564–3.198) | 0.51 | |||
Lab examination | |||||
D-dimer >500 μg/L | 2.2 (0.919–5.41) | 0.08 | |||
WBC (×109/L) | 0.839 (0.708–0.973) | 0.011 | 0.839 (0.708–0.973) | 0.029 |
BMI, body mass index; CTA, computed tomography angiography; ULP, ulcer-like projection; IBP, intramural blood pool; MAD, maximal aortic diameter; MHT, maximal hematoma thickness; WBC, white blood cell; CI, confidence interval.
Machine learning models construction
A total of 1,316 features were extracted from each ROI. Of these, 694 features with ICCs higher than 0.80 in the interobserver and the intraobserver analysis were selected. There were 170 features retained after univariate analysis and 42 features retained after correlation analysis. Finally, 12 optimized radiomics features were selected using LASSO in the training cohort (Figure 2). Figure 3 shows the ROC curves of machine learning models in the training and validation cohorts. In the validation cohort, SVM-radial basis function (SVM-RBF) was the best model with an AUC of 0.765 (95% CI, 0.593–0.906). The SVM-RBF model was used to calculate the radiomics signature Radscore.
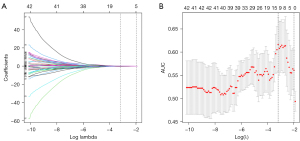
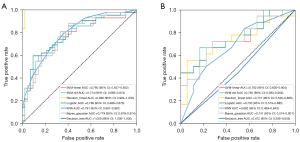
Nomogram development and performance validation
A combined nomogram based on clinical features and the radiomics signature Radscore was developed (Figure 4A). The nomogram incorporated branches, ULP, WBC, and Radscore. A score for each patient was obtained by drawing a vertical line to the top scale and reading off the variable’s score for each individual. The total score for an individual was obtained by summing up all the individual variable scores. A scale for the total score is given at the bottom of the nomogram. The nomogram had the best predictive performance, with an AUC of 0.811 (95% CI, 0.706–0.897) in the training cohort. The accuracy, sensitivity, and specificity were 76.2%, 69.0%, and 83.3%, respectively. In the validation cohort, the nomogram also performed well, with an AUC of 0.787 (95% CI, 0.619–0.923), and the accuracy, sensitivity, and specificity were 66.7%, 61.1%, and 72.2%, respectively (Figure 4B,4C). The results of DeLong’s test are summarized in Table 3. The AUC values of the combined nomogram model were significantly higher than those of the clinical model in both the raining (P=0.034) and validation cohorts (P=0.022), but they were not significantly higher than those of the radiomics model. The calibration curve with Hosmer-Lemeshow test was used to evaluate the calibration performance of the nomogram. It indicated no significant differences between the predicted and actual values in the training and validation cohorts (P=0.723 and P=0.224; Figure 5A,5B). Decision curve analysis was showed in Figure 5C.
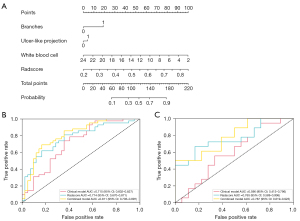
Table 3
Model | Model | P value |
---|---|---|
Training cohort | ||
Clinical model | Radscore | 0.351 |
Clinical model | Combined model | 0.034 |
Radscore | Combined model | 0.169 |
Validation cohort | ||
Clinical model | Radscore | 0.123 |
Clinical model | Combined model | 0.022 |
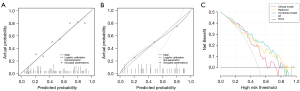
Discussion
The present study demonstrated the value of a radiomics-clinical model in predicting the prognosis of patients with medically treated IMH. First, this study independently evaluated the clinical factors and radiomic features of the aortic CTA. Then, a combined nomogram based on independent clinical factors and Radscore was established. The results showed that the nomogram could predict the prognosis of these patients. To our knowledge, this study is the first analysis using radiomics features and the first to build a nomogram combining clinical factors and radiomics features to predict the progression of IMH.
Prior studies identified the presence of ULP/focal intimal disruptions (FID) diagnosed in the acute phase to be related to poor prognosis (18-20). This finding was detected in more than 50% of patients in previous reports (4,21,22). IMH involving the aortic wall surrounding and extending into the ostia of the arch and visceral branch vessel may lead to luminal narrowing. Secondary insufficient perfusion of distal organs may result in adverse consequences. Research on branch involvements has mostly focused on patients with AD. Hashimoto et al. (23) found that branch involvement had a significant impact on short-term prognosis. However, there is still a lack of research on the involvement of aortic branches in patients with IMH, and thus its relationship to the prognosis of patients requires further research. Some aortic conditions are associated with “inflammatory-cell-mediated destructive remodeling” pathways (24). Higher WBC counts may be indirect evidence of these actions. Fan et al. (25) and Chen et al. (26) revealed WBC counts to be associated with death in the short-term in patients with AD.
Radiomics is a promising tool in medical imaging that can extract quantitative and reproducible information. It has revealed direct links between tissue imaging phenotyping and tissue biology, with important clinical implications (27). In this study, the radiomics features were extracted from original CT images and images derived from several filters. The filtration process could reduce the effect of photon noise and enhance biological heterogeneity (28). Wavelet features accounted for a large proportion of the extracted radiomic features in the current study. Specifically, 8 of the 12 optimized radiomics features were wavelet features, and the other 4 features were 2 LoG filtered features with a sigma of 3.0 mm and 2 3D LBP filtered features. This study calculated first-order and 5 different higher-order textural parameters, which were GLCM, GLSZM, GLRLM, GLDM, and NGTDM. The higher first-order intensity, kurtosis, and large dependence high gray level emphasis of the GLDM matrix showed a higher risk of destabilization in IMH, which might reflect a complex, widespread high attenuation within the hematoma. This combined application of different matrix features could give a more accurate description of the heterogeneous characteristics of the lesion in different dimensions.
Both in the training and validation cohorts, AUCs of the nomogram were superior to those of the clinical models and comparable to those of the radiomics models. In this study, besides the traditional clinical risk factor models, we proposed a radiomics model and a clinical-radiomics combined nomogram model to provide a better predictor of the prognosis of IMH. The radiomics model and clinical-radiomics combined nomogram model significantly improved the prediction efficacy. It was better than the radiomics model, although the improvement in this efficacy was not statistically significant. We suggest this improvement might have been partly caused by the selection and the sample size of the retrospective cohort. In our center, most patients with large MHT, MAD, PAU, and other serious signs received surgery or endovascular therapy at baseline and were excluded from this study. In the future, multicenter and large-sample research will be conducted to obtain greater models. The current result might provide more evidence about the CT-based radiomics features in describing IMH.
In general, logistic regression, RF, and SVM are suitable for small-sample data and binary-class tasks (29). SVM is the most commonly used algorithm (30). However, there are limited studies on the analysis of aortic disease using radiomics and machine learning methods. Therefore, in this study, we used 6 machine learning algorithms to build a radiomics model to find the best machine learning algorithm for IMH. The SVM-RBF model appeared best suited to building the radiomics model for predicting the prognosis of patients with IMH treated by medical therapy.
In summary, radiomics is a promising interdisciplinary approach to medicine and computer science, but some characteristics restrict its extensive development. First, the effectiveness of radiomics depends on a great quantity of medical data, including imaging and clinical data, and requires a considerable amount of data processing. Multicenter and multiple scanners studies are necessary for radiomics to enable analyses with greater statistical power and generalizability. Therefore, the acquisition and harmonization of medical data are crucial to radiomics. Approaches have been developed to address the problem of harmonization, such as ComBat (31). Second, the traditional segmentation of the ROI relies on manual segmentation, which consumes a high amount of energy resources and time and requires certain expertise. Although many artificial intelligence algorithms have been applied to segmentation, the accuracy of manual segmentation is still the highest.
There are several limitations to this study. First, this study was a single-center retrospective study with a relatively small sample size, and thus bias might have been introduced. Therefore, in future research, multicenter, large-sample-size validation is needed. Second, images included in this study were not obtained with the same CT equipment although image preprocessing was conducted to eliminate the differences as much as possible.
In conclusion, a quantitative nomogram based on clinical factors and radiomic features of CT imaging was demonstrated to improve the prediction of the prognosis of patients with medically treated IMH. The model can serve as a potential decision-support tool to assist clinicians in evaluating the conditions of patients and facilitate appropriate management in a timely manner.
Acknowledgments
Funding: This work was supported by the National Natural Science Foundation of China (No. 82071875), the Beijing Natural Science Foundation (No. 7212025), and the Beijing Natural Science Foundation (No. 7222302).
Footnote
Reporting Checklist: The authors have completed the TRIPOD reporting checklist. Available at https://qims.amegroups.com/article/view/10.21037/qims-22-480/rc
Conflicts of Interest: All authors have completed the ICMJE uniform disclosure form (available at https://qims.amegroups.com/article/view/10.21037/qims-22-480/coif). SL is an employee of GE Healthcare (China). The other authors have no conflicts of interest to declare.
Ethical Statement: The authors are accountable for all aspects of the work in ensuring that questions related to the accuracy or integrity of any part of the work are appropriately investigated and resolved. This retrospective cohort study was conducted in accordance with the Declaration of Helsinki (as revised in 2013). The study was approved by the institutional ethics committee of Beijing Anzhen Hospital, and individual consent for this retrospective analysis was waived.
Open Access Statement: This is an Open Access article distributed in accordance with the Creative Commons Attribution-NonCommercial-NoDerivs 4.0 International License (CC BY-NC-ND 4.0), which permits the non-commercial replication and distribution of the article with the strict proviso that no changes or edits are made and the original work is properly cited (including links to both the formal publication through the relevant DOI and the license). See: https://creativecommons.org/licenses/by-nc-nd/4.0/.
References
- Erbel R, Aboyans V, Boileau C, Bossone E, Bartolomeo RD, Eggebrecht H, et al. 2014 ESC Guidelines on the diagnosis and treatment of aortic diseases: Document covering acute and chronic aortic diseases of the thoracic and abdominal aorta of the adult. The Task Force for the Diagnosis and Treatment of Aortic Diseases of the European Society of Cardiology (ESC). Eur Heart J 2014;35:2873-926. [Crossref] [PubMed]
- Mesar T, Lin MJ, Kabir I, Dexter DJ, Rathore A, Panneton JM. Medical therapy in type B aortic intramural hematoma is associated with a high failure rate. J Vasc Surg 2020;71:1088-96. [Crossref] [PubMed]
- Kitai T, Kaji S, Yamamuro A, Tani T, Kinoshita M, Ehara N, Kobori A, Kim K, Kita T, Furukawa Y. Detection of intimal defect by 64-row multidetector computed tomography in patients with acute aortic intramural hematoma. Circulation 2011;124:S174-8. [Crossref] [PubMed]
- Li Y, Zhang N, Xu S, Fan Z, Zhu J, Huang L, Chen D, Sun Z, Sun L. Acute type A aortic intramural hematoma and type A aortic dissection: correlation between the intimal tear features and pathogenesis. Quant Imaging Med Surg 2020;10:1504-14. [Crossref] [PubMed]
- Kruse MJ, Johnson PT, Fishman EK, Zimmerman SL. Aortic intramural hematoma: review of high-risk imaging features. J Cardiovasc Comput Tomogr 2013;7:267-72. [Crossref] [PubMed]
- Herrán FL, Bang TJ, Restauri N, Suby-Long T, Alvarez Gómez DI, Sachs PB, Vargas D. CT imaging of complications of aortic intramural hematoma: a pictorial essay. Diagn Interv Radiol 2018;24:342-7. [Crossref] [PubMed]
- Li Wen Y, Leech M. Review of the Role of Radiomics in Tumour Risk Classification and Prognosis of Cancer. Anticancer Res 2020;40:3605-18. [Crossref] [PubMed]
- Kolossváry M, Park J, Bang JI, Zhang J, Lee JM, Paeng JC, Merkely B, Narula J, Kubo T, Akasaka T, Koo BK, Maurovich-Horvat P. Identification of invasive and radionuclide imaging markers of coronary plaque vulnerability using radiomic analysis of coronary computed tomography angiography. Eur Heart J Cardiovasc Imaging 2019;20:1250-8. [Crossref] [PubMed]
- Kolossváry M, Karády J, Szilveszter B, Kitslaar P, Hoffmann U, Merkely B, Maurovich-Horvat P. Radiomic Features Are Superior to Conventional Quantitative Computed Tomographic Metrics to Identify Coronary Plaques With Napkin-Ring Sign. Circ Cardiovasc Imaging 2017;10:e006843. [Crossref] [PubMed]
- Oikonomou EK, Williams MC, Kotanidis CP, Desai MY, Marwan M, Antonopoulos AS, et al. A novel machine learning-derived radiotranscriptomic signature of perivascular fat improves cardiac risk prediction using coronary CT angiography. Eur Heart J 2019;40:3529-43. [Crossref] [PubMed]
- Cheng K, Lin A, Yuvaraj J, Nicholls SJ, Wong DTL. Cardiac Computed Tomography Radiomics for the Non-Invasive Assessment of Coronary Inflammation. Cells 2021;10:879. [Crossref] [PubMed]
- Shang J, Ma S, Guo Y, Yang L, Zhang Q, Xie F, Ma Y, Ma Q, Dang Y, Zhou K, Liu T, Yang J, Hou Y. Prediction of acute coronary syndrome within 3 years using radiomics signature of pericoronary adipose tissue based on coronary computed tomography angiography. Eur Radiol 2022;32:1256-66. [Crossref] [PubMed]
- Guo Y, Chen X, Lin X, Chen L, Shu J, Pang P, Cheng J, Xu M, Sun Z. Non-contrast CT-based radiomic signature for screening thoracic aortic dissections: a multicenter study. Eur Radiol 2021;31:7067-76. [Crossref] [PubMed]
- Zhou Z, Yang J, Wang S, Li W, Xie L, Li Y, Zhang C. The diagnostic value of a non-contrast computed tomography scan-based radiomics model for acute aortic dissection. Medicine (Baltimore) 2021;100:e26212. [Crossref] [PubMed]
- Liu YJ, Zhang QY, Du ZK, Yang L, Zhang L, He RX, Wang Y, Han YL, Wang XZ. Long-term follow-up and clinical implications in Chinese patients with aortic intramural hematomas. Int J Cardiol 2018;270:268-72. [Crossref] [PubMed]
- Evangelista A, Czerny M, Nienaber C, Schepens M, Rousseau H, Cao P, Moral S, Fattori R. Interdisciplinary expert consensus on management of type B intramural haematoma and penetrating aortic ulcer. Eur J Cardiothorac Surg 2015;47:209-17. [Crossref] [PubMed]
- Huang LF, Wan P, Xu DW, Jeong S, Feng MX, Zhang JJ, Xia Q. Nomogram predicting pulmonary metastasis of hepatocellular carcinoma after liver transplantation. Oncotarget 2017;9:2425-34. [Crossref] [PubMed]
- Ishizu K, Kaji S, Nakashima M, Kitai T, Kim K, Ehara N, Kinoshita M, Furukawa Y. Focal Intimal Disruption Size at Multidetector CT and Disease Progression in Type B Aortic Intramural Hematoma. Radiology 2021;301:311-9. [Crossref] [PubMed]
- Czerny M, Pacini D, Aboyans V, Al-Attar N, Eggebrecht H, Evangelista A, et al. Current options and recommendations for the use of thoracic endovascular aortic repair in acute and chronic thoracic aortic disease: an expert consensus document of the European Society for Cardiology (ESC) Working Group of Cardiovascular Surgery, the ESC Working Group on Aorta and Peripheral Vascular Diseases, the European Association of Percutaneous Cardiovascular Interventions (EAPCI) of the ESC and the European Association for Cardio-Thoracic Surgery (EACTS). Eur J Cardiothorac Surg 2021;59:65-73. [Crossref] [PubMed]
- Moral S, Cuéllar H, Avegliano G, Ballesteros E, Salcedo MT, Ferreira-González I, García-Dorado D, Evangelista A. Clinical Implications of Focal Intimal Disruption in Patients With Type B Intramural Hematoma. J Am Coll Cardiol 2017;69:28-39. [Crossref] [PubMed]
- Li Z, Lu B, Chen Y, Hou Z, Chen B, Zhang Y, An Y, Wei Y. Acute type B aortic intramural hematoma: the added prognostic value of a follow-up CT. Eur Radiol 2019;29:6571-80. [Crossref] [PubMed]
- Tanaka A, Leake S, Estrera AL. Management strategies in acute type B aortic intramural hematoma. Curr Opin Cardiol 2017;32:687-91. [Crossref] [PubMed]
- Hashimoto O, Saito Y, Nakayama T, Okino S, Sakai Y, Nakamura Y, Fukuzawa S, Himi T, Ishibashi I, Kobayashi Y. Prognostic Impact of Branch Vessel Involvement on Computed Tomography versus Clinical Presentation of Malperfusion in Patients With Type a Acute Aortic Dissection. Am J Cardiol 2021;152:158-63. [Crossref] [PubMed]
- Jiang D, Kuang F, Lai Y, Shan Z, Chen Q. Certain aortic geometries and hemodynamics are associated with FID development and impact the evolution of uncomplicated type B intramural hematoma during the acute phase. J Card Surg 2019;34:337-47. [Crossref] [PubMed]
- Fan X, Huang B, Lu H, Zhao Z, Lu Z, Yang Y, Zhang S, Hui R. Impact of Admission White Blood Cell Count on Short- and Long-term Mortality in Patients With Type A Acute Aortic Dissection: An Observational Study. Medicine (Baltimore) 2015;94:e1761. [Crossref] [PubMed]
- Chen ZR, Huang B, Lu HS, Zhao ZH, Hui RT, Yang YM, Fan XH. Admission white blood cell count predicts short-term clinical outcomes in patients with uncomplicated Stanford type B acute aortic dissection. J Geriatr Cardiol 2017;14:49-56. [PubMed]
- Gillies RJ, Kinahan PE, Hricak H. Radiomics: Images Are More than Pictures, They Are Data. Radiology 2016;278:563-77. [Crossref] [PubMed]
- Gao Y, Hua M, Lv J, Ma Y, Liu Y, Ren M, Tian Y, Li X, Zhang H. Reproducibility of radiomic features of pulmonary nodules between low-dose CT and conventional-dose CT. Quant Imaging Med Surg 2022;12:2368-77. [Crossref] [PubMed]
- Zhang L, Zhe X, Tang M, Zhang J, Ren J, Zhang X, Li L. Predicting the Grade of Prostate Cancer Based on a Biparametric MRI Radiomics Signature. Contrast Media Mol Imaging 2021;2021:7830909. [Crossref] [PubMed]
- DeJohn CR, Grant SR, Seshadri M. Application of Machine Learning Methods to Improve the Performance of Ultrasound in Head and Neck Oncology: A Literature Review. Cancers (Basel) 2022;14:665. [Crossref] [PubMed]
- Horng H, Singh A, Yousefi B, Cohen EA, Haghighi B, Katz S, Noël PB, Shinohara RT, Kontos D. Generalized ComBat harmonization methods for radiomic features with multi-modal distributions and multiple batch effects. Sci Rep 2022;12:4493. [Crossref] [PubMed]