An integrated nomogram combined semantic-radiomic features to predict invasive pulmonary adenocarcinomas in subjects with persistent subsolid nodules
Introduction
The prevalence and detection rate of pulmonary subsolid nodules (SSNs) has increased in recent years due to the high utilization of low-dose lung computed tomography (CT) for lung cancer screening worldwide, especially in Asian populations with a high prevalence of non-smoking-related lung cancer (1-6). Lung adenocarcinoma spectrum lesions are the most common histological subtype of lung cancer in Asian populations. They are divided into atypical adenomatous hyperplasia (AAH), adenocarcinoma in situ (AIS), minimally invasive adenocarcinoma (MIAs), and invasive pulmonary adenocarcinomas (IPAs) according to the International Association for the Study of Lung Cancer (IASLC), American Thoracic Society (ATS), and European Respiratory Society (ERS) in 2011 (7,8). This classification has important prognostic implications because preinvasive lesions like AAH, AIS, and MIA have a more favorable prognostic outcome than IPA lesions (9-11). As persistent SSNs have a higher likelihood of lung adenocarcinoma spectrum lesions with heterogeneous growth or aggressive behavior, it is important for radiologists and clinical physicians to correctly identify these indolent preinvasive lesions from IPAs preoperatively (12-16).
Given the inconsistent diagnosis by radiologists, an increasing number of studies have attempted to incorporate clinical profiles, CT features, and radiomic biomarkers to predict IPA lesions in persistent pulmonary SSNs, such as part-solid nodules (PSNs) or pure ground-glass nodules (GGNs) (17-24). However, previous studies have demonstrated inconclusive results regarding the benefit of combined clinical, semantic, or radiomic CT features to improve IPAs prediction in different study settings (17,19-26). In this study, we hypothesized that combined semantic-radiomic features in thin-slice CT settings have superior diagnostic performance in differentiating IPAs from preinvasive lesions in patients with persistent SSNs, which is important in guiding surgical decision-making. This study tests the hypothesis that combination of semantic-radiomic features has a better classification ability than traditional models. This study aimed to develop and validate the least absolute shrinkage and selection operator (LASSO)-derived nomogram integrating semantic-radiomic features in differentiating preinvasive and IPA lesions, and to compare the predictive ability of the other six prediction models, including the clinical-semantic model (model 2), radiologist’s performance (model 3–4), and semantic model (model 5–7). We present the following article in accordance with the STARD reporting checklist (available at https://qims.amegroups.com/article/view/10.21037/qims-22-308/rc).
Methods
Study cohort
The study was conducted in accordance with the Declaration of Helsinki (as revised in 2013). The study was reviewed and approved by the Institutional Review Board of Kaohsiung Veterans General Hospital (IRB number: VGHKS19-CT2–09). And patient consent was waived owing to the retrospective design of the study.
The inclusion criteria for the study participants were specified as follows: (I) patients with persistent SSNs ≤30 mm in the longest diameter, (II) patients not receiving preoperative therapy or treatment before surgical resection, (III) patients underwent surgical resection within 3 months of thin-slice CT, (IV) patients who underwent preoperative chest CT scan with thin-slice thickness setting before the surgical intervention (slice thickness: ≤2.5 mm), and (V) patients with a pathologically confirmed diagnosis of pulmonary adenocarcinoma spectrum lesions based on the IASLC/ATS/ERS classification. The training and validation cohorts were grouped according to the time of enrollment, and the research design followed the validation rules (27). The study participants (n=260) were divided into two groups: a training cohort (n=195) and validation cohort (n=65) in a 3:1 ratio (Figure 1). The study population consisted of 195 patients including 195 SSNs with surgical pathology from February 2016 to March 2020 in the training cohort, and 65 patients including 65 SSNs with surgical pathology from April 2020 to August 2020 in the validation cohort.
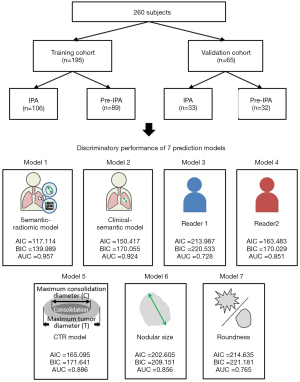
CT image acquisition
All patients underwent thin-slice chest CT within 3 months of surgical resection at our hospital. All preoperative thin-slice chest CT scans were performed using a 16-slice CT scanner (Somatom Sensation 16, Siemens Healthcare, Erlangen, Germany), 64-slice CT scanner (Aquilion 64; Toshiba Medical Systems), 64-slice CT scanner (Discovery CT750 HD, GE Healthcare, Milwaukee, USA), and 256-slice CT scanner (Revolution CT, GE Healthcare, Milwaukee, USA) from the lung apex to the lung base. CT scans were acquired at full inspiration without contrast agent administration. The details of the scanning protocol for different vendors are listed as follows: tube voltage of 120 kVp; slice thickness, 1–2.5 mm; and reconstruction algorithm, soft tissue kernel.
Clinical and semantic features
The clinical information of the study participants, including age, sex, and smoking history, was collected from the electronic medical record system at the Kaohsiung Veterans General Hospital. In this study, nine CT radiologic semantic features were identified to characterize pulmonary subsolid nodules. Semantic CT features included the following: (I) lesion location, (II) nodular size along the longest axis, (III) nodular type according to the Fleischer classification (SSNs categorized as GGNs or PSNs) (28) (IV) solid part in the lung window setting with a window level of 1,500 Hounsfield units (HU) and a window width of −430 HU in the picture archiving system; (V) cystic change (defined as a low attenuation area within the SSNs), (VI) air-bronchogram (defined as small dilated, distorted bronchi passing through the SSNs), (VII) shape (smooth, lobulated, and spiculated border), (VIII) roundness (oval or irregular), and (IX) consolidation to tumor ratio (CTR). The CTR was defined as the ratio of the maximum size of consolidation (solid part in the lung window) to the maximum tumor size in the lung window. Each semantic CT feature was reviewed and defined by a consensus between two experienced thoracic radiologists (15 and 6 years of experience, respectively). The selection of the three clinical features and nine semantic features were extracted through LASSO regression to construct the clinical-semantic predictive model for differentiating preinvasive and IPA lesions according to the rationale design (29).
Radiomic feature extraction
The LifeX package open-source software (http://www.lifesoft.org) for nodule segmentation and volume of interest (VOI)-based radiomic analysis was employed to extract thin-slice CT imaging features manually by an experienced thoracic radiologist (30). A total of 41 radiomic features were grouped and extracted according to the LifeX package and previous studies. The following features were extracted for the histogram of the grey-level distribution: minimum, maximum, mean, and standard deviation of the HU distribution. The following features were extracted for the first-order metrics extracted from the histogram: SkewnessH, KurtosisH, EntropyH, and EnergyH. The following features were extracted for the second-order metrics calculated from the co-occurrence matrices: homogeneity, energy, contrast, correlation, entropy, and dissimilarity. For higher-order metrics extracted from the grey-level histogram, the parameters included features of neighborhood grey-level dependence matrix (NGLDM), grey-level co-occurrence matrix (GLCM), grey-level zone length matrix (GLZLM), and grey-level run length matrix (GLRLM).
Radiologist interpretation and predictive performance
Two experienced thoracic radiologists (with 15 and 6 years of experience, respectively) independently interpreted the pulmonary adenocarcinoma spectrum lesion subclassification of 260 SSNs according to the IASLC/ATS/ERS classification using blind evaluation methods. In addition, the pulmonary adenocarcinoma spectrum lesions were subclassified into the preinvasive lesion group (AAH, AIS, and MIA) and invasive lesion group (IPA).
Histological evaluation
All surgical specimens were routinely fixed in formalin and embedded in paraffin using hematoxylin and eosin staining for histopathological diagnosis. Two pathologists histopathologically analyzed the surgically resected specimens at a consensus conference to establish a final diagnosis. They were subclassified as AAH, AIS, MIA, and IPA lesions according to the revised lung adenocarcinoma (IASLC/ATS/ERS) classification of 2011. All 260 participants with 260 SSNs were categorized into preinvasive (AAH, AIS, and MIA lesions) and invasive lesion groups (invasive pulmonary adenocarcinoma lesions) according to the revised lung adenocarcinoma (IASLC/ATS/ERS) classification. Of all 260 patients with surgical proof, 78 (30%) patients underwent lobectomy, 24 (9.2%) underwent segmentectomy, and 158 (60.8%) underwent wedge resection.
LASSO-derived predictive model building and model comparison
A LASSO classifier was conducted using tenfold cross-validation on the training cohort to choose the optimized features and to build semantic-radiomic and clinical-semantic predictive models for IPA prediction. Six features were extracted through LASSO regression to construct a semantic-radiomic predictive model with the optimal lambda value that minimizes the error in cross-validation. Its predictive accuracy and discriminatory ability were compared with other predictive models, such as the clinical-semantic model, semantic model, or radiologist’s performance. A personalized nomogram based on the semantic-radiomic model in the training cohort was constructed to predict the degree of invasiveness of pulmonary adenocarcinoma spectrum lesions. Calibration plots with the Hosmer-Lemeshow test were used to evaluate the goodness of fit of this predictive model. The following seven predictive models were compared: combined semantic-radiomic model (Model 1); clinical-semantic model (Model 2), radiologist 1 performance (Model 3), radiologist 2 performance (Model 4), CTR model (Model 5), nodular size (Model 6), and roundness (Model 7). The discriminatory ability of these seven predictive models was evaluated and compared using C-statistics [area under the receiver operating characteristic (ROC) curve (AUC)], Akaike information criterion (AIC), and Bayesian information criterion (BIC). Higher c-statistics and lower AIC/BIC values indicated a more discriminatory model.
Statistical analyses
All statistical analyses were performed using SPSS 22.0 for Windows (SPSS Inc., Chicago, IL, USA), Stata version 13.1 (StataCorp, College Station, Texas, USA), and MedCalc 15.8 (MedCalc Software, Acacialaan 22, Ostend, Belgium). For the estimated sample size, sample size was determined by “rule of thumb” formula (31). An independent Student’s t-test was used to test the mean differences between the two groups if continuous variables are normally distributed under the null hypothesis. Continuous variables were presented as mean ± standard deviation (SD). Categorical variables were summarized as frequencies and percentages and compared using the chi-square or Fisher’s exact test to examine proportion differences between the two groups. A LASSO classifier was conducted using tenfold cross-validation on the training cohort to choose the optimized features and to build semantic-radiomic and clinical-semantic predictive models for IPA prediction. Therefore, univariate and multivariate logistic regressions were used to build a LASSO-derived predictive model/nomogram based on the selected semantic-radiomic features to differentiate IPA lesions from preinvasive lesions.
The logistic results were expressed as odds ratios (ORs) with 95% confidence intervals (CIs). ROC curves for these predictive models were constructed, and the difference between AUCs was calculated to compare the diagnostic performance of these predictive models using DeLong’s method (32). Moreover, regarding the PPV, NPV, sensitivity, specificity, positive LR (LR+), and negative LR (LR−), the diagnostic accuracy was calculated to measure the overall accuracy of these predictive models.
The Hosmer-Lemeshow test was used to estimate and validate the goodness-of-fit of the combined semantic-radiomic predictive model (33). Calibration was evaluated using Hosmer-Lemeshow goodness-of-fit statistics and calibration graphs plotting predicted IPA lesions against the observed rates in deciles of predicted risk. A prediction nomogram was established based on the LASSO-derived combined semantic-radiomics model in the training cohort. Statistical significance for all tests was set at P value of less than 0.05.
Results
The study population characteristics
In total, 260 participants with pathologically confirmed SSNs were enrolled in this study, with 66.6% (n=195) in the training cohort and 33.3% (n=65) in the validation cohort. Of the 195 participants with pathologically confirmed pulmonary adenocarcinoma spectrum lesions in the training cohort, 56 (28.7%) were men and 139 (71.3%) were women; 106 had IPA lesions, and 89 had preinvasive lesions. The prevalence of IPA lesions in the training cohort was 54.35%. Of the 65 participants with pathologically confirmed pulmonary adenocarcinoma spectrum lesions in the validation cohort, 33 had IPA lesions, and 32 had preinvasive lesions. In the validation cohort, the prevalence of IPA lesions was 50.76%. Summary of cross tubulation of model 1 for diagnosis of invasive pulmonary adenocarcinomas in training and validation cohorts are shown in Figure S1. Table 1 summarizes the clinical and semantic characteristic features of the training cohort and validation cohort participants. For the clinical and semantic characteristic features, no significant differences were found in the percentage of sex ratio, smoking habit, lesion location, nodular type, presence of cystic change, presence of air bronchogram, shape (smooth, lobulated, or spiculated), and roundness (oval or irregular) between the two groups. Compared to the validation cohort group, no differences in age, nodular size, the solid component in the lung window setting, and CTR were observed in the training cohort (Table 1).
Table 1
Characteristics | Training cohort (n=195) | Validation cohort (n=65) | P value |
---|---|---|---|
Gender, n (%) | 0.187 | ||
Male | 56 (28.7) | 14 (21.5) | |
Female | 139 (71.3) | 51 (78.5) | |
Age, years (mean ± SD) | 60.23±9.489 | 58.09±9.655 | 0.119 |
Smoking history, n (%) | 0.288 | ||
No | 172 (88.2) | 54 (83.1) | |
Yes | 23 (11.8) | 11 (16.9) | |
Lesion location, n (%) | 0.939 | ||
Right upper lobe | 62 (31.8) | 24 (36.9) | |
Right middle lobe | 13 (6.7) | 3 (4.6) | |
Right lower lobe | 43 (22.1) | 12 (18.5) | |
Left upper lobe | 52 (26.7) | 17 (26.2) | |
Left lower lobe | 25 (12.8) | 9 (13.8) | |
Nodular type, Fleischer classification, n (%) | 0.701 | ||
GGN | 61 (31.3) | 22 (33.8) | |
PSN | 134 (68.7) | 43 (66.2) | |
Nodular size, range 0.25 to 2.99 cm (mean ± SD) | 1.667±1.026 | 1.529±1.053 | 0.350 |
Solid part, lung window (mean ± SD) | 0.764±0.843 | 0.761±0.793 | 0.980 |
Cystic change, n (%) | 0.130 | ||
Cystic change (−) | 178 (91.3) | 63 (96.9) | |
Cystic change (+) | 17 (8.7) | 2 (3.1) | |
Air bronchogram, n (%) | 0.112 | ||
Air bronchogram (−) | 104 (53.3) | 42 (64.6) | |
Air bronchogram (+) | 91 (46.7) | 23 (35.4) | |
Shape, n (%) | 0.813 | ||
Smooth | 58 (29.7) | 21 (32.3) | |
Lobulated | 94 (48.2) | 32 (49.2) | |
Spiculated | 43 (22.1) | 12 (18.5) | |
Roundness, n (%) | 0.828 | ||
Oval | 84 (43.1) | 29 (44.6) | |
Irregular | 111 (56.9) | 36 (55.4) | |
CTR (mean ± SD) | 0.347±0.286 | 0.349±0.295 | 0.960 |
SD, standard deviation; GGN, ground-glass nodule; PSN, part-solid nodule; CTR, consolidation/tumor ratio.
LASSO-derived predictive models for invasiveness prediction
Logistic regression with LASSO penalization was conducted to help reduce the dimensions of feature selection through ten-fold cross-validation for IPA lesion prediction. Two LASSO-derived models were developed based on combined semantic-radiomic (Model 1) or clinical-semantic features (Model 2). The combined semantic-radiomic model (Model 1) with optimal value of lambda included the following six non-zero variables: “nodular size”, CTR, “roundness”, GLCM_Entropy_log10, “HISTO_Entropy_log10”, and “CONVENTIONAL_HUmean”. Summary of cross tubulation of model 1 for diagnosis of invasive pulmonary adenocarcinomas in training and validation cohorts are shown in Figure S1.
The clinical-semantic model (Model 2) with optimal value of lambda included the following five non-zero variables: “nodular size”, “CTR”, “roundness”, “shape”, and “air bronchogram”. Univariate and multivariate logistic analyses were performed in the training cohort to establish a combined semantic-radiomics predictive model for IPA lesions. The results of the univariate and multivariate logistic regression analyses for this combined semantic-radiomics predictive model are summarized in Table 2.
Table 2
Variable | Coefficient | OR | 95% CI | P value |
---|---|---|---|---|
Univariate model | ||||
Nodular size | 1.855 | 6.394 | 3.514–11.635 | <0.001 |
Roundness | 2.399 | 11.008 | 5.626–21.537 | <0.001 |
CTR | 7.042 | 1,143.598 | 194.833–6,712.490 | <0.001 |
CONVENTIONAL_HUmean | 0.016 | 1.016 | 1.011–1.020 | <0.001 |
HISTO_Entropy_log10 | 13.964 | 1,160,172.509 | 24,415.044–55,129,953.62 | <0.001 |
HISTO_Entropy_log2 | 4.204 | 66.927 | 20.932–213.988 | <0.001 |
GLCM_Entropy_log10 | 5.296 | 199.583 | 45.982–866.291 | <0.001 |
GLCM_Entropy_log2 (= joint entropy) | 1.594 | 4.925 | 3.166–7.662 | <0.001 |
LASSO-derived model (including nodular size, roundness, CTR, CONVENTIONAL_Humean, HISTO_Entropy_log10, GLCM_Entropy_log10) | 6.832 | 926.783 | 179.363–4,788.765 | <0.001 |
LASSO, least absolute shrinkage and selection operator; SSN, subsolid nodule; CTR, consolidation/tumor ratio; OR, odds ratio; CI, confidence interval.
Development and validation of the LASSO-derived model/nomogram based on semantic-radiomics features
A nomogram was established to predict IPA lesions based on LASSO-derived multivariable logistic regression according to the selected semantic-radiomic features shown in Figure 2. By adding the scores identified on the point scale for each parameter, a straight line was easily obtained to establish the estimated individual probability score for IPA lesions in the training cohort. For example, to explain the nomogram model, if a 63-year-old man has the following profile of one PSN in RLL: a nodular size of 1.21 cm, irregular shape, CTR value of 0.521, GLCM_Entrophy_log10 value of 3.31, HISTO_Entrophy_log10 value of 1.85, and CONVENTIONAL_HUmean value of −624.72, the probability of IPA lesion was estimated to be 99.7%. Finally, the pathological report demonstrated invasive pulmonary adenocarcinoma in the RLL, as shown in Figure 3A. In a 57-year-old female with a profile of one GGN in the LUL: a nodular size of 0.49 cm, oval shape, CTR value of 0; GLCM_Entrophy_log10 of 1.96, HISTO_Entrophy_log10 value of 1.32, and CONVENTIONAL_HUmean value of −623.54, the probability of IPA lesions was estimated to be 27%. Finally, the pathological report demonstrated adenocarcinoma in situ in the LUL, as shown in Figure 3B.
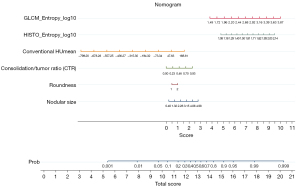
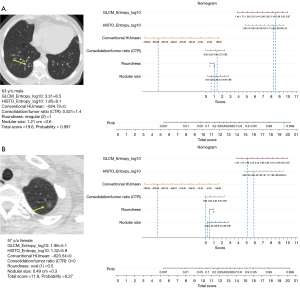
Using a tenfold cross-validation method, the Hosmer-Lemeshow test was used to estimate and validate the goodness-of-fit of the predictive model/nomogram. The pooled AUC of the nomogram was 0.956 (95% CI: 0.929–0.983) in the training cohort and 0.960 (95% CI: 0.9168–1.000) in the validation cohort. The ROC curve showed that the resulting model had excellent discrimination between the training and validation cohorts. The Hosmer-Lemeshow goodness-of-fit test yielded P values of 0.394 and 0.787 in the training and validation cohorts, respectively, indicating good calibration power, as displayed by the calibration curves in Figures 4,5.
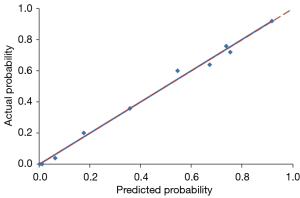
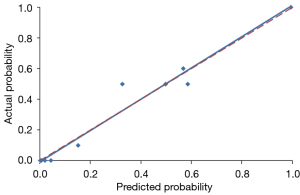
Comparison of the seven predictive models
Table 3 presents a summary of the discriminating ability and diagnostic performance of the seven predictive models, including the semantic-radiomic (Model 1), clinical-semantic (Model 2), radiologist 1 (Model 3), radiology 2 (Model 4), CTR (Model 5), nodular size (Model 6), and roundness models (Model 7). In this study, we hypothesized that the combined semantic-radiomic model would have superior diagnostic performance in differentiating IPAs from preinvasive lesions. The comparisons and differences between these predictive models are summarized in Table 4. Our study results demonstrated that the LASSO-based semantic-radiomic model has a significantly superior discrimination ability, higher c-statistics, and lower AIC and BIC values than the other four predictive models. Compared with other predictive models, the LASSO-derived semantic-radiomic nomogram model showed better diagnostic performance with an AUC of 0.957 (95% CI: 0.918–0.981) for the detection of IPA lesions in Asian populations with persistent SSNs, with balanced sensitivity (92.45%) and specificity (88.64%). In addition, the LASSO classifier provided optimal predictive models with AUC values of 0.957 and 0.924 for Models 1 and 2, respectively. The ROC curves for the combined semantic-radiomic model, clinical-semantic model, two-radiologist performance, and other semantic models are shown in Figure 1.
Table 3
Model | AIC | BIC | AUC (95% CI) | Cut-point | Sensitivity, % (95% CI) |
Specificity, % (95% CI) |
+LR (95% CI) | −LR (95% CI) | +PV (95% CI) | −PV (95% CI) | Accuracy |
---|---|---|---|---|---|---|---|---|---|---|---|
Model 1 | 117.1143 | 139.9893 | 0.957 (0.918–0.981) | >0.4979 | 92.45 (85.7–96.7) | 88.64 (80.1–94.4) | 8.14 (7.4–8.9) | 0.085 (0.04–0.2) | 90.7 (83.6–95.5) | 90.7 (82.4–95.9) | 0.903 |
Model 2 | 150.417 | 170.055 | 0.924 (0.878–0.957) | >0.4796 | 90.57 (83.3–95.4) | 83.15 (73.7–90.2) | 5.37 (4.8–6.0) | 0.11 (0.05–0.2) | 86.5 (78.7–92.2) | 88.1 (79.2–94.1) | 0.872 |
Model 3 | 213.9875 | 220.5335 | 0.728 (0.660–0.790) | >0 | 49.06 (39.2–59.0) | 96.63 (90.5–99.3) | 14.55 (11.9–17.7) | 0.53 (0.2–1.6) | 94.5 (84.9–98.9) | 61.4 (52.8–69.5) | 0.708 |
Model 4 | 163.4837 | 170.0297 | 0.851 (0.793–0.898) | >0 | 91.51 (84.5–96.0) | 78.65 (68.7–86.6) | 4.29 (3.8–4.8) | 0.11 (0.05–0.2) | 83.6 (75.6–89.8) | 88.6 (79.5–94.7) | 0.856 |
Model 5 | 165.0951 | 171.6411 | 0.886 (0.833–0.927) | >0.2977 | 85.85 (77.7–91.9) | 79.78 (69.9–87.6) | 4.24 (3.7–4.8) | 0.18 (0.09–0.3) | 83.5 (75.2–89.9) | 82.6 (72.9–89.9) | 0.826 |
Model 6 | 202.6057 | 209.1517 | 0.856 (0.799–0.902) | >1.08 | 91.51 (84.5–96.0) | 66.29 (55.5–76.0) | 2.71 (2.3–3.2) | 0.13 (0.06–0.3) | 76.4 (68.0–83.5) | 86.8 (76.4–93.8) | 0.795 |
Model 7 | 214.6351 | 221.1811 | 0.765 (0.699–0.823) | >1 | 81.13 (72.4–88.1 | 71.91 (61.4–80.9) | 2.89 (2.5–3.4) | 0.26 (0.2–0.4) | 77.5 (68.6–84.9) | 76.2 (65.7–84.8) | 0.769 |
Model 1: Semantic-radiomic model; Model 2: Clinical-semantic model; Model 3: Reader 1; Model 4: Reader 2; Model 5: CTR model; Model 6: Nodular size; Model 7: Roundness. AIC, Akaike information criterion; BIC, Bayesian information criterion; AUC, area under the ROC curve; CI, confidence interval; LR, likelihood ratio; PV, predictive value; CTR, consolidation to tumor ratio.
Table 4
Group | Difference between areas | SE | 95% CI | P value |
---|---|---|---|---|
Model 1 vs. Model 2 | 0.0328 | 0.0137 | 0.0059–0.0597 | 0.017 |
Model 1 vs. Model 3 | 0.229 | 0.0244 | 0.181–0.276 | <0.001 |
Model 1 vs. Model 4 | 0.107 | 0.0219 | 0.0642–0.150 | <0.001 |
Model 1 vs. Model 5 | 0.0718 | 0.0194 | 0.0337–0.110 | <0.001 |
Model 1 vs. Model 6 | 0.0963 | 0.0244 | 0.0485–0.144 | <0.001 |
Model 1 vs. Model 7 | 0.187 | 0.0290 | 0.131–0.244 | <0.001 |
Model 1: Semantic-radiomic model; Model 2: Clinical-semantic model; Model 3: Reader 1; Model 4: Reader 2; Model 5: CTR model; Model 6: Nodular size; Model 7: Roundness. SE, standard error; CI, confidence interval; CTR, consolidation to tumor ratio.
Discussion
This single-hospital study analyzed the clinical-semantic-radiomic predictive model individually and in combination to develop and validate this combined predictive model to help identify IPA lesions manifesting as SSNs to guide clinical decision-making for surgical planning. This combined semantic-radiomic model had the highest AUC (0.957) and achieved the best diagnostic accuracy when compared with the other models (Model 2, AUC =0.924, P=0.017; Model 3, AUC =0.728, P<0.001; Model 4, AUC =0.851, P<0.001; Model 5, AUC =0.886, P<0.001; Model 6, AUC =0.856, P<0.001; Model 7, AUC =0.765, P<0.001).
This study had three major findings. First, a LASSO-derived combined nomogram predictive model was developed based on six predictors (nodular size, CTR, roundness, GLCM_Entropy_log10, HISTO_Entropy_log10, and CONVENTIONAL_HUmean) to predict IPA lesions in SSNs, providing an excellent level of diagnostic performance for identifying these lesions in SSNs. Second, the LASSO-derived semantic-radiomic model exhibited a significantly better discrimination ability and lower AIC and BIC values compared with those of the other models, including the clinical-semantic, radiologist 1, radiologist 2, CTR, roundness, and nodular size definition models (sensitivity, 92.45%; specificity, 88.64%; cutoff point of probability score, ≥0.4979). Third, the LASSO-derived risk-predictive model (Model 1) exhibited good calibration ability in the both training and validation cohorts.
However, previous studies have demonstrated controversial results regarding clinical, semantic, and radiomic features or combinations in predicting IPAs in patients with GGNs, PSNs, or SSNs (17,19-24). Wu et al. demonstrated that separating ground-glass and solid CT radiomic features yielded a significantly better predictive performance than clinical-semantic models in patients with PSNs (22). Similarly, a recent study by Weng demonstrated that the combined model outperformed only CT-based semantic models in PSNs (21). For GGN analysis, two studies demonstrated that combined models have superior performance compared to clinical models (20,23). However, a recent study by Xu et al. found that the selected radiomic features did not provide useful information for improving the performance of the combined predictive model (24). In addition, for diagnostic performance analyses based on different study designs in patients with GGNs or PSNs, the diagnostic performance in studies focusing on PSNs showed good-to-excellent diagnostic accuracy (21,22). However, the diagnostic performance in studies focusing on GGNs showed only good diagnostic accuracy (0.750–0.889) with a wide range of variability (20,23,24,34,35). The differences in the study results may be due to the misclassification of PSNs or GGNs.
This is the first study to combine semantic and radiomic features to establish an individual predictive model of SSNs. Previous studies have assessed conventional CT features or signs for IPA lesion prediction (29,36-41). A previous literature review investigated, for the first time, pure GGNs or PSNs to build and validate a predictive model based on combined clinical-semantic-radiomic features for IPA lesion identification in SSNs, and comprehensively compared the diagnostic performances of other predictive models, semantic features, or radiologists’ performance. However, these diagnoses based on semantic features would need to be examined by experienced radiologists, and interobserver differences have been found in previous studies (42,43). Previous studies have demonstrated conventional CT features for predicting the pathological invasiveness of SSNs with fair to good diagnostic accuracy (29,36-41). Our study results also support that conventional CT features such as nodular size, CTR, or roundness (shape) have fair to good diagnostic performance for IPAs.
In this study, Model 2 used clinical and semantic parameters with similar diagnostic performance compared with Model 1. For the current real-world clinical practice, Model 2 would be more convenient than Model 1. However, previous studies have demonstrated that inter-observer variability in CT-based semantic features is higher than that in CT-based radiomic features (25,42,43). In addition, we identified three radiomic-based features with high reproducibility for radiomic-based model development in differentiating IPA from preinvasive lesions, which could enhance radiomics application to facilitate its acceptance in clinical practice (44,45). Therefore, the reproducibility of Model 1 may be superior than that of Model 2 owing to the high interobserver agreement in radiomic feature analysis. Furthermore, a LASSO-derived predictive model incorporating semantic-radiomic features in pulmonary invasive adenocarcinoma lesions was built, which yielded substantially higher AUC values with balanced sensitivity and specificity than the semantic models. The combined semantic-radiomic models outperformed the performance of the two radiologists.
Therefore, this nomogram/predictive model could improve radiologists’ performance in diagnosing IPAs in SSNs. Our rationale was to develop a radiomic-based model to differentiate IPA from preinvasive lesions (AAH, AIS, and MIA) before surgery to guide clinical management strategies. Specifically, recent evidence supports the notion that lobectomy is generally preferred for patients with IPAs. Sublobar resection, especially wedge resection, has been generally accepted as a safe and effective surgical option for patients with preinvasive lesions, such as MIA/AIS lesions (46,47). The selected radiomic features reflected environmental habitat heterogeneity. It also exhibited good calibration ability in both the training and validation cohorts. In this study, we used a simplified, standardized non-contrast chest CT protocol and an open-source software platform designed to improve the feasibility of general clinical practice implementation. However, multicenter studies are needed to conduct external validation of the findings and model stability.
Study limitation
This study has some limitations and flaws. First, this was a retrospective study, and only patients with surgically resected persistent SSNs were enrolled. Therefore, selection bias is inherent in the study design. However, a wide spectrum of patients with SSNs was enrolled in this study rather than only those with GGNs or PSNs like in previous studies. Second, the ROI was segmented manually over time. In the future, automatic ROI segmentation through artificial intelligence should be addressed in a real-world setting, thereby improving patient care and helping physicians become more efficient and effective using a clinical decision nomogram through artificial intelligence segmentation. Third, different vendor settings will result in more bias with batch effects in radiomic feature analysis (45,48,49). The batch effect can be reduced using the ComBat approach, as demonstrated in recent studies. Further studies are warranted to reduce the batch effect using the ComBat approach (50).
Conclusions
A LASSO-derived predictive model was successfully developed and validated based on six parameters, including “nodular size”, “CTR”, “roundness”, “GLCM_Entropy_log10”, “HISTO_Entropy_log10”, and “CONVENTIONAL_HUmean”, providing good diagnostic performance in predicting IPA from preinvasive lesions. This nomogram could help clinicians identify IPA lesions to guide personalized medicine and make informed decisions in SSN management with good calibration.
Acknowledgments
Funding: This study was supported by grants from Kaohsiung Veterans General Hospital (Nos. KSVGH111-108, KSVGH111-D02-3, MOST 110-2314-B-075B-008).
Footnote
Reporting Checklist: The authors have completed the STARD reporting checklist. Available at https://qims.amegroups.com/article/view/10.21037/qims-22-308/rc
Conflicts of Interest: All authors have completed the ICMJE uniform disclosure form (available at https://qims.amegroups.com/article/view/10.21037/qims-22-308/coif). The authors have no conflicts of interest to declare.
Ethical Statement: The authors are accountable for all aspects of the work in ensuring that questions related to the accuracy or integrity of any part of the work are appropriately investigated and resolved. The study was conducted in accordance with the Declaration of Helsinki (as revised in 2013). The study was reviewed and approved by the Institutional Review Board of Kaohsiung Veterans General Hospital (No. VGHKS19-CT2–09). Considering the retrospective design of the study, the requirement for informed consent was waived by the institutional review board.
Open Access Statement: This is an Open Access article distributed in accordance with the Creative Commons Attribution-NonCommercial-NoDerivs 4.0 International License (CC BY-NC-ND 4.0), which permits the non-commercial replication and distribution of the article with the strict proviso that no changes or edits are made and the original work is properly cited (including links to both the formal publication through the relevant DOI and the license). See: https://creativecommons.org/licenses/by-nc-nd/4.0/.
References
- Aberle DR, Adams AM, Berg CD, Black WC, Clapp JD, Fagerstrom RM, Gareen IF, Gatsonis C, Marcus PM, Sicks JD. Reduced lung-cancer mortality with low-dose computed tomographic screening. N Engl J Med 2011;365:395-409. [Crossref] [PubMed]
- Hsu HT, Tang EK, Wu MT, Wu CC, Liang CH, Chen CS, Mar GY, Lai RS, Wang JC, Wu CL, Huang YL, Wu FZ. Modified Lung-RADS Improves Performance of Screening LDCT in a Population with High Prevalence of Non-smoking-related Lung Cancer. Acad Radiol 2018;25:1240-51. [Crossref] [PubMed]
- Lin KF, Wu HF, Huang WC, Tang PL, Wu MT, Wu FZ. Propensity score analysis of lung cancer risk in a population with high prevalence of non-smoking related lung cancer. BMC Pulm Med 2017;17:120. [Crossref] [PubMed]
- Wu FZ, Huang YL, Wu CC, Tang EK, Chen CS, Mar GY, Yen Y, Wu MT. Assessment of Selection Criteria for Low-Dose Lung Screening CT Among Asian Ethnic Groups in Taiwan: From Mass Screening to Specific Risk-Based Screening for Non-Smoker Lung Cancer. Clin Lung Cancer 2016;17:e45-56. [Crossref] [PubMed]
- Wu FZ, Huang YL, Wu YJ, Tang EK, Wu MT, Chen CS, Lin YP. Prognostic effect of implementation of the mass low-dose computed tomography lung cancer screening program: a hospital-based cohort study. Eur J Cancer Prev 2020;29:445-51. [Crossref] [PubMed]
- Zhou F, Zhou C. Lung cancer in never smokers-the East Asian experience. Transl Lung Cancer Res 2018;7:450-63. [Crossref] [PubMed]
- Austin JH, Garg K, Aberle D, Yankelevitz D, Kuriyama K, Lee HJ, Brambilla E, Travis WD. Radiologic implications of the 2011 classification of adenocarcinoma of the lung. Radiology 2013;266:62-71. [Crossref] [PubMed]
- Travis WD, Brambilla E, Noguchi M, Nicholson AG, Geisinger KR, Yatabe Y, et al. International association for the study of lung cancer/american thoracic society/european respiratory society international multidisciplinary classification of lung adenocarcinoma. J Thorac Oncol 2011;6:244-85. [Crossref] [PubMed]
- Travis WD, Brambilla E, Noguchi M, Nicholson AG, Geisinger K, Yatabe Y, Ishikawa Y, Wistuba I, Flieder DB, Franklin W, Gazdar A, Hasleton PS, Henderson DW, Kerr KM, Nakatani Y, Petersen I, Roggli V, Thunnissen E, Tsao M. Diagnosis of lung adenocarcinoma in resected specimens: implications of the 2011 International Association for the Study of Lung Cancer/American Thoracic Society/European Respiratory Society classification. Arch Pathol Lab Med 2013;137:685-705. [Crossref] [PubMed]
- Yoshizawa A, Motoi N, Riely GJ, Sima CS, Gerald WL, Kris MG, Park BJ, Rusch VW, Travis WD. Impact of proposed IASLC/ATS/ERS classification of lung adenocarcinoma: prognostic subgroups and implications for further revision of staging based on analysis of 514 stage I cases. Mod Pathol 2011;24:653-64. [Crossref] [PubMed]
- Travis WD, Asamura H, Bankier AA, Beasley MB, Detterbeck F, Flieder DB, Goo JM, MacMahon H, Naidich D, Nicholson AG, Powell CA, Prokop M, Rami-Porta R, Rusch V, van Schil P, Yatabe Y; International Association for the Study of Lung Cancer Staging and Prognostic Factors Committee and Advisory Board Members. The IASLC Lung Cancer Staging Project: Proposals for Coding T Categories for Subsolid Nodules and Assessment of Tumor Size in Part-Solid Tumors in the Forthcoming Eighth Edition of the TNM Classification of Lung Cancer. J Thorac Oncol 2016;11:1204-23.
- Godoy MC, Naidich DP. Overview and strategic management of subsolid pulmonary nodules. J Thorac Imaging 2012;27:240-8. [Crossref] [PubMed]
- Henschke CI, Yankelevitz DF, Mirtcheva R, McGuinness G, McCauley D, Miettinen OS. ELCAP Group. CT screening for lung cancer: frequency and significance of part-solid and nonsolid nodules. AJR Am J Roentgenol 2002;178:1053-7. [Crossref] [PubMed]
- Kim HY, Shim YM, Lee KS, Han J, Yi CA, Kim YK. Persistent pulmonary nodular ground-glass opacity at thin-section CT: histopathologic comparisons. Radiology 2007;245:267-75. [Crossref] [PubMed]
- Ren H, Liu F, Xu L, Sun F, Cai J, Yu L, Guan W, Xiao H, Li H, Yu H. Predicting the histological invasiveness of pulmonary adenocarcinoma manifesting as persistent pure ground-glass nodules by ultra-high-resolution CT target scanning in the lateral or oblique body position. Quant Imaging Med Surg 2021;11:4042-55. [Crossref] [PubMed]
- Shao X, Shao X, Niu R, Jiang Z, Xu M, Wang Y. Investigating the association between ground-glass nodules glucose metabolism and the invasive growth pattern of early lung adenocarcinoma. Quant Imaging Med Surg 2021;11:3506-17. [Crossref] [PubMed]
- Fan L, Fang M, Li Z, Tu W, Wang S, Chen W, Tian J, Dong D, Liu S. Radiomics signature: a biomarker for the preoperative discrimination of lung invasive adenocarcinoma manifesting as a ground-glass nodule. Eur Radiol 2019;29:889-97. [Crossref] [PubMed]
- Feng B, Chen X, Chen Y, Li Z, Hao Y, Zhang C, Li R, Liao Y, Zhang X, Huang Y, Long W. Differentiating minimally invasive and invasive adenocarcinomas in patients with solitary sub-solid pulmonary nodules with a radiomics nomogram. Clin Radiol 2019;74:570.e1-570.e11. [Crossref] [PubMed]
- She Y, Zhang L, Zhu H, Dai C, Xie D, Xie H, Zhang W, Zhao L, Zou L, Fei K, Sun X, Chen C. The predictive value of CT-based radiomics in differentiating indolent from invasive lung adenocarcinoma in patients with pulmonary nodules. Eur Radiol 2018;28:5121-8. [Crossref] [PubMed]
- Sun Y, Li C, Jin L, Gao P, Zhao W, Ma W, Tan M, Wu W, Duan S, Shan Y, Li M. Radiomics for lung adenocarcinoma manifesting as pure ground-glass nodules: invasive prediction. Eur Radiol 2020;30:3650-9. [Crossref] [PubMed]
- Weng Q, Zhou L, Wang H, Hui J, Chen M, Pang P, Zheng L, Xu M, Wang Z, Ji J. A radiomics model for determining the invasiveness of solitary pulmonary nodules that manifest as part-solid nodules. Clin Radiol 2019;74:933-43. [Crossref] [PubMed]
- Wu G, Woodruff HC, Shen J, Refaee T, Sanduleanu S, Ibrahim A, Leijenaar RTH, Wang R, Xiong J, Bian J, Wu J, Lambin P. Diagnosis of Invasive Lung Adenocarcinoma Based on Chest CT Radiomic Features of Part-Solid Pulmonary Nodules: A Multicenter Study. Radiology 2020;297:E282. [Crossref] [PubMed]
- Wu L, Gao C, Xiang P, Zheng S, Pang P, Xu M. CT-Imaging Based Analysis of Invasive Lung Adenocarcinoma Presenting as Ground Glass Nodules Using Peri- and Intra-nodular Radiomic Features. Front Oncol 2020;10:838. [Crossref] [PubMed]
- Xu F, Zhu W, Shen Y, Wang J, Xu R, Qutesh C, Song L, Gan Y, Pu C, Hu H. Radiomic-Based Quantitative CT Analysis of Pure Ground-Glass Nodules to Predict the Invasiveness of Lung Adenocarcinoma. Front Oncol 2020;10:872. [Crossref] [PubMed]
- Wu YJ, Liu YC, Liao CY, Tang EK, Wu FZ. A comparative study to evaluate CT-based semantic and radiomic features in preoperative diagnosis of invasive pulmonary adenocarcinomas manifesting as subsolid nodules. Sci Rep 2021;11:66. [Crossref] [PubMed]
- Wu YJ, Wu FZ, Yang SC, Tang EK, Liang CH. Radiomics in Early Lung Cancer Diagnosis: From Diagnosis to Clinical Decision Support and Education. Diagnostics (Basel) 2022;12:1064. [Crossref] [PubMed]
- Han K, Song K, Choi BW. How to Develop, Validate, and Compare Clinical Prediction Models Involving Radiological Parameters: Study Design and Statistical Methods. Korean J Radiol 2016;17:339-50. [Crossref] [PubMed]
- Naidich DP, Bankier AA, MacMahon H, Schaefer-Prokop CM, Pistolesi M, Goo JM, Macchiarini P, Crapo JD, Herold CJ, Austin JH, Travis WD. Recommendations for the management of subsolid pulmonary nodules detected at CT: a statement from the Fleischner Society. Radiology 2013;266:304-17. [Crossref] [PubMed]
- Wu FZ, Chen PA, Wu CC, Kuo PL, Tsao SP, Chien CC, Tang EK, Wu MT. Semiquantative Visual Assessment of Sub-solid Pulmonary Nodules ≦3 cm in Differentiation of Lung Adenocarcinoma Spectrum. Sci Rep 2017;7:15790. [Crossref] [PubMed]
- Nioche C, Orlhac F, Boughdad S, Reuzé S, Goya-Outi J, Robert C, Pellot-Barakat C, Soussan M, Frouin F, Buvat I. LIFEx: A Freeware for Radiomic Feature Calculation in Multimodality Imaging to Accelerate Advances in the Characterization of Tumor Heterogeneity. Cancer Res 2018;78:4786-9. [Crossref] [PubMed]
- Peduzzi P, Concato J, Feinstein AR, Holford TR. Importance of events per independent variable in proportional hazards regression analysis. II. Accuracy and precision of regression estimates. J Clin Epidemiol 1995;48:1503-10. [Crossref] [PubMed]
- DeLong ER, DeLong DM, Clarke-Pearson DL. Comparing the areas under two or more correlated receiver operating characteristic curves: a nonparametric approach. Biometrics 1988;44:837-45. [Crossref] [PubMed]
- Lemeshow S, Hosmer DW Jr. A review of goodness of fit statistics for use in the development of logistic regression models. Am J Epidemiol 1982;115:92-106. [Crossref] [PubMed]
- Li W, Wang X, Zhang Y, Li X, Li Q, Ye Z. Radiomic analysis of pulmonary ground-glass opacity nodules for distinction of preinvasive lesions, invasive pulmonary adenocarcinoma and minimally invasive adenocarcinoma based on quantitative texture analysis of CT. Chin J Cancer Res 2018;30:415-24. [Crossref] [PubMed]
- Cho HH, Lee G, Lee HY, Park H. Marginal radiomics features as imaging biomarkers for pathological invasion in lung adenocarcinoma. Eur Radiol 2020;30:2984-94. [Crossref] [PubMed]
- Cui X, Heuvelmans MA, Fan S, Han D, Zheng S, Du Y, Zhao Y, Sidorenkov G, Groen HJM, Dorrius MD, Oudkerk M, de Bock GH, Vliegenthart R, Ye Z. A Subsolid Nodules Imaging Reporting System (SSN-IRS) for Classifying 3 Subtypes of Pulmonary Adenocarcinoma. Clin Lung Cancer 2020;21:314-325.e4. [Crossref] [PubMed]
- Zhang Y, Shen Y, Qiang JW, Ye JD, Zhang J, Zhao RY. HRCT features distinguishing pre-invasive from invasive pulmonary adenocarcinomas appearing as ground-glass nodules. Eur Radiol 2016;26:2921-8. [Crossref] [PubMed]
- Yue X, Liu S, Liu S, Yang G, Li Z, Wang B, Zhou Q. HRCT morphological characteristics distinguishing minimally invasive pulmonary adenocarcinoma from invasive pulmonary adenocarcinoma appearing as subsolid nodules with a diameter of ≤3 cm. Clin Radiol 2018;73:411.e7-411.e15. [Crossref] [PubMed]
- Zhan Y, Peng X, Shan F, Feng M, Shi Y, Liu L, Zhang Z. Attenuation and Morphologic Characteristics Distinguishing a Ground-Glass Nodule Measuring 5-10 mm in Diameter as Invasive Lung Adenocarcinoma on Thin-Slice CT. AJR Am J Roentgenol 2019;213:W162-70. [Crossref] [PubMed]
- Tamura M, Matsumoto I, Saito D, Yoshida S, Kakegawa S, Takemura H. Mean Computed Tomography Value to Predict the Tumor Invasiveness in Clinical Stage IA Lung Cancer. Ann Thorac Surg 2017;104:261-6. [Crossref] [PubMed]
- Chae HD, Park CM, Park SJ, Lee SM, Kim KG, Goo JM. Computerized texture analysis of persistent part-solid ground-glass nodules: differentiation of preinvasive lesions from invasive pulmonary adenocarcinomas. Radiology 2014;273:285-93. [Crossref] [PubMed]
- Jeon KN, Goo JM, Lee CH, Lee Y, Choo JY, Lee NK, Shim MS, Lee IS, Kim KG, Gierada DS, Bae KT. Computer-aided nodule detection and volumetry to reduce variability between radiologists in the interpretation of lung nodules at low-dose screening computed tomography. Invest Radiol 2012;47:457-61. [Crossref] [PubMed]
- van Riel SJ, Sánchez CI, Bankier AA, Naidich DP, Verschakelen J, Scholten ET, de Jong PA, Jacobs C, van Rikxoort E, Peters-Bax L, Snoeren M, Prokop M, van Ginneken B, Schaefer-Prokop C. Observer Variability for Classification of Pulmonary Nodules on Low-Dose CT Images and Its Effect on Nodule Management. Radiology 2015;277:863-71. [Crossref] [PubMed]
- Varghese BA, Hwang D, Cen SY, Lei X, Levy J, Desai B, Goodenough DJ, Duddalwar VA. Identification of robust and reproducible CT-texture metrics using a customized 3D-printed texture phantom. J Appl Clin Med Phys 2021;22:98-107. [Crossref] [PubMed]
- Lee SH, Cho HH, Lee HY, Park H. Clinical impact of variability on CT radiomics and suggestions for suitable feature selection: a focus on lung cancer. Cancer Imaging 2019;19:54. [Crossref] [PubMed]
- Zhang Y, Ma X, Shen X, Wang S, Li Y, Hu H, Chen H. Surgery for pre- and minimally invasive lung adenocarcinoma. J Thorac Cardiovasc Surg 2022;163:456-64. [Crossref] [PubMed]
- Saji H, Okada M, Tsuboi M, Nakajima R, Suzuki K, Aokage K, et al. Segmentectomy versus lobectomy in small-sized peripheral non-small-cell lung cancer (JCOG0802/WJOG4607L): a multicentre, open-label, phase 3, randomised, controlled, non-inferiority trial. Lancet 2022;399:1607-17. [Crossref] [PubMed]
- van Velden FH, Kramer GM, Frings V, Nissen IA, Mulder ER, de Langen AJ, Hoekstra OS, Smit EF, Boellaard R. Repeatability of Radiomic Features in Non-Small-Cell Lung Cancer (18)FFDG-PET/CT Studies: Impact of Reconstruction and Delineation. Mol Imaging Biol 2016;18:788-95. [Crossref] [PubMed]
- Zwanenburg A. Radiomics in nuclear medicine: robustness, reproducibility, standardization, and how to avoid data analysis traps and replication crisis. Eur J Nucl Med Mol Imaging 2019;46:2638-55. [Crossref] [PubMed]
- Orlhac F, Boughdad S, Philippe C, Stalla-Bourdillon H, Nioche C, Champion L, Soussan M, Frouin F, Frouin V, Buvat I. A Postreconstruction Harmonization Method for Multicenter Radiomic Studies in PET. J Nucl Med 2018;59:1321-8. [Crossref] [PubMed]