Evaluation of a respiratory motion-corrected image reconstruction algorithm in 2-[18F]FDG and [68Ga]Ga-DOTA-NOC PET/CT: impacts on image quality and tumor quantification
Introduction
Patients are breathing freely during positron emission tomography (PET) scans, because 2–3 min per bed position or even 10 min, depending on the applications, are usually needed to obtain diagnostic quality images. As a result, respiratory motions cause image artifacts and blurring (1), which may affect image interpretation and therapy evaluation (2-6).
The importance of respiratory motion correction for PET imaging has prompted a series of technical developments (7). Gated image reconstruction is a basic approach for respiratory motion correction in which PET data are allocated into several gates according to their phase or amplitude in the respiratory waveform. Since 6 to 8 gates are typically used, only 12–17% of total counts are allocated in each gate. Therefore, a longer acquisition time is required to compensate for lower photon-counting statistics. An alternative to this method is quiescent period gating (QPG), which generates a single image in the expiration phase. Since exhalation usually takes more time than does inhalation, QPG can use 30–50% of the counts and alleviate the demand for a longer scan time. Recent studies have shown that nonrigid registration-based algorithms can use all counts in the motion correction process, such as advanced optical flow and deblurring convolution (8-12). Promising phantom and clinical results (13-15) have demonstrated that these algorithms can improve image noise performance without prolonging the acquisition time.
A recent development in this field is the respiratory motion-corrected image reconstruction (MCIR) algorithm. The MCIR includes activity-attenuation matching and nonrigid registration into an image reconstruction process to improve quantitative accuracy and reduce motion artifacts. However, the efficacy of MCIR on respiratory motion correction has not yet been evaluated, and the evaluation of MCIR on tracers beyond 2-deoxy-2-[18F]fluoro-D-glucose {2-[18F]FDG} has not been reported on. Therefore, this study aimed to investigate the impact of the MCIR algorithm on tumor evaluation and image quality using the data of a motion phantom and oncology patients undergoing 2-[18F]FDG and 68Ga-labeled [1,4,7,10-tetraazacyclododecane-1,4,7,10-tetraacetic acid]-1-Nal3-octreotide {[68Ga]Ga-DOTA-NOC} PET/computed tomography (CT). In this study, lesions’ standardized uptake values (SUVs), volumes, and visual image quality scores were compared between reconstructions with and without MCIR. Furthermore, to test the feasibility of a deviceless MCIR solution, we evaluated the images reconstructed with MCIR using an external respiratory monitor device that acquired the respiratory waveform as the input and using deviceless data-driven gating (DDG) technique to derive the respiratory waveform as the input for the MCIR process.
Methods
Respiratory motion-corrected image reconstruction
In this study, we used a fully automated gate-to-gate activity-attenuation-matched elastic respiratory MCIR algorithm that was US Food and Drug Administration-cleared as HYPER Focus by United Imaging Healthcare (UIH; Shanghai, China). In the MCIR process, interim gated PET images are reconstructed using a pseudo attenuation map filled with a soft tissue coefficient. The mutual information of the gated PET and CT image is then calculated, and the gate with the maximal similarity is selected as the best match (16). The selected gate is set as the reference in the motion vector field (MVF) modeling that is based on a B-spline interpolation-based nonrigid registration algorithm (17). This MVF is transposed and applied to the CT image to generate a matched activity-attenuation map for each gate. A second set of gated PET images is reconstructed with the attenuation map transferred by the MVFs. Finally, the gated PET images of the second reconstruction are deformed with the MVF and fused into a single image set as the final output.
Phantom study
A motion phantom was built in-house based on the design shown in previous studies (13,14). The phantom consisted of 5 spheres with 10, 13, 17, 22, and 28 mm diameters placed in the middle of a 15 liter acrylic tank that was filled with 18F solution with a sphere-to-background activity ratio of 5:1. The spheres were connected to a respiratory motion platform (Modus QA, QUASAR, London, ON, Canada) that generated a back-and-forth motion in a head-to-feet direction. The 2 motion amplitudes were 2 and 3 cm, both at a respiratory cycle of 6 s. The respiratory signal was acquired with a vital signal module (VSM; UIH) by fastening a pressure sensor belt to an airbag connected to the motion platform that provided synchronized respiratory motion signals. Figure 1 illustrates the phantom setup.
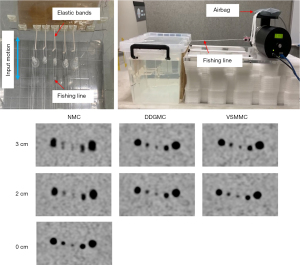
The phantom was scanned on a UIH uMI780 PET/CT. List-mode PET data were acquired in 3 15-min sessions in which the phantom was set at a motionless mode (nonmoving state) and a motion mode with a 2 and 3 cm amplitude. Three frames were cut at the beginning, the middle, and the end of the 15-min list-mode data, allowing us to measure the spheres 3 times. The duration of the frames was 182 to 212 s. These durations considered radioactive decay to be approximate to a clinical situation of image acquisition with a 3 min per bed acquisition time 1-hour postinjection of 3.7 MBq/kg 2-[18F]FDG.
Four groups of PET images were reconstructed for the phantom. The first group was for the phantom in motionless mode (motionless group). The other 3 groups were for the phantom operating in the motion mode: DDG-based motion correction (DDGMC), VSM-based motion correction (VSMMC), and no motion correction (NMC). DDGMC and VSMMC images were reconstructed with UIH’s HYPER Focus algorithm using DDG-derived and VSM-acquired respiratory waveforms as the respective inputs. NMC was a nongated static reconstruction without motion correction. In total, there were 21 reconstructions: 1 for the motionless group; 3 for the DDGMC, VSMMC, and NMC group with a motion amplitude of 2 cm; and 3 for the DDGMC, VSMMC, and NMC group with a motion amplitude of 3 cm. Each was multiplied by 3 frames divided from the list-mode data. All reconstructions were performed under the following parameters: 192×192 matrix, 600 mm field of view (FOV), 2.68 mm slice thickness, ordered subset expectation maximization (OSEM) reconstruction with 2 iterations, 20 subsets, 3 mm post-Gaussian filter, time-of-flight kernel, point-spread-function model, and other vendor’s default reconstruction settings.
Phantom data evaluation
The volume of interest (VOI) of the sphere was delineated on the PET images using an adaptive threshold-based segmentation tool on a commercial workstation (uWS-MI, UIH). The segmentation threshold was set at 50% of the maximum standardized uptake value (SUV). The maximum SUV (SUVmax), mean SUV (SUVmean), and volume were measured at each sphere VOI delineated in DDGMC, VSMMC, and NMC images. The differences in SUVmax, SUVmean, and volume (ΔSUVmax, ΔSUVmean, and Δvolume) were calculated by subtracting these values from 2 reconstruction groups denoted as DDGMC-NMC, VSMMC-NMC, and DDGMC-VSMMC. Relative changes of SUVmax, SUVmean, and volume (relative ΔSUVmax, relative ΔSUVmean, and relative Δvolume) of the spheres were calculated by normalizing ΔSUVmax, ΔSUVmean, and Δvolume to their corresponding SUVmax, SUVmean, and volume values in NMC, respectively. The relative changes are presented in percentages. In addition, the SUV and the volume of DDGMC, VSMMC, and NMC measured under the motion modes were compared with those from the motionless group.
Patient study
Patients were referred to whole-body 2-[18F]FDG or [68Ga]Ga-DOTA-NOC PET/CT examination for tumor staging or follow-up. Patients were enrolled if 2-[18F]FDG or [68Ga]Ga-DOTA-NOC avid lesions were found in the regions subject to respiratory motion artifacts, such as lesions in the vicinity of the diaphragm, and if they could cooperate with having an external breathing monitor device tied around their chests. The study was conducted in accordance with the Declaration of Helsinki (as revised in 2013). The study was approved by the Ethics Committee of the Nanjing First Hospital, and informed consent was taken from all individual participants.
The patient study used the same PET/CT scanner as the phantom study. Patients undergoing 2-[18F]FDG PET/CT fasted for at least 6 h and had a plasma glucose level of <11 mmol/L before the examination. Ultimately, 42 patients underwent PET/CT scans 60±17 min postinjection of 277±67 MBq 2-[18F]FDG, and 17 patients underwent PET/CT scans 76±26 min postinjection of 110±17 MBq [68Ga]Ga-DOTA-NOC. The respiratory waveform was acquired using a VSM device by fastening a belt around the upper abdomen below the top of the xiphoid process. After completion of a helix CT scan using 120 kVp tube voltage and 200 mAs tube current with automatic modulation, a PET scan was performed from the mid-thighs to the eyes with an emission time of 3 min per bed position at the chest and upper abdomen with 2 min per bed position in the rest of body regions. The patient was asked to keep quiet and breath normally during the whole scanning process. The 3 reconstructions, DDGMC, VSMMC, and NMC, were performed using the same reconstruction parameters used in the phantom study.
Patient data evaluation
The quantitative evaluation and visual image assessment were performed on the same workstation used in the phantom study. A nuclear radiologist with 5 years of experience in PET/CT performed the quantitative evaluation and was blinded to patient identifications and motion correction-related reconstruction settings. A 3 cm diameter circular region of interest (ROI) was placed on a homogeneous area of healthy tissue in the liver. This ROI was first placed on NMC images and propagated to DDGMC and VSMMC. The mean (SUVmean) and standard deviation (SD) of SUVs were measured from the liver ROI. The coefficient of variation (COV%) was calculated by dividing the SD by SUVmean of the liver and was presented as the percentage.
From each patient, the radiologist selected 1–2 lesions to evaluate. The lesion was selected if the motion artifact was visually identified. Otherwise, it was selected from the regions that were most likely to be vulnerable to respiratory motion. If there were multiple candidate lesions, up to 2 smaller and segmentable lesions were selected. The lesions were segmented with the same segmentation method used in the phantom study. The SUVmax, SUVmean, and volume were measured in the DDGMC, VSMMC, and NMC groups. The ΔSUVmax, ΔSUVmean, Δvolume, and their relative changes were also calculated.
Visual image quality assessment was performed by 2 nuclear radiologists with 5 and 7 years of experience in PET/CT. The information related to motion correction settings was removed, and the image reading order was randomized. The respiratory motion artifacts were assessed using the diaphragm and lesion motion score that focused on the motion artifacts on the diaphragm and the lesions, respectively. Both motion scores used a 3-point scale: 1, nondetectable or minor motion; 2, mild motion; and 3, severe motion artifacts. In another review session, the radiologists were asked to compare the images of each of the 2 reconstruction groups in a pairwise fashion. This comparison used a setup similar to that used in a previous study (18). With a side-by-side display mode, the images were displayed in pairs: DDGMC vs. NMC, VSMMC vs. NMC, and DDGMC vs. VSMMC. The displayed side of a reconstruction group in the image pairs was assigned randomly such that raters could not associate the displaying side with a reconstruction group. The raters scored the image pairs using a 5-point scale. For the assessment of motion artifacts, lesion blurring, and overall image quality, scores of −2, −1, 0, 1, or 2 were given when the image displayed on the left side was obviously better, slightly better, not different, slightly worse, or obviously worse compared with the right-side image, respectively. After the assessment, a data scientist reviewed the scores and the displayed side randomization records to note the association between scores and reconstruction groups.
Statistical analysis
Data analyses were performed using R statistical package version 4.1.2 (The R Foundation of Statistical Computing) and Microsoft Excel version 2016. The numerical data were tested with the Shapiro-Wilks normality test. Because the data did not follow a normal distribution, the Wilcoxon signed-rank test was used to compare numeric data between reconstruction groups. The difference in visual image quality scores was compared using Fisher exact test due to the small data size. P values were adjusted with false discovery rate correction for multiple comparisons. The interreader agreement of visual image assessment was tested with Cohen’s kappa. A P value of <0.05 was considered statistically significant.
Results
Phantom study
Respiratory motions distorted the outline of larger spheres and caused blurring in smaller spheres in the NMC groups, and those artifacts were corrected in the DDGMC and VSMMC groups (Figure 1). Without motion correction, the SUVmax, SUVmean, and volume had a dependency on the motion amplitudes in NMC. The SUVs were lower and the volumes were larger for spheres with a 3 cm motion amplitude compared to those with a 2 cm motion amplitude (Figure 2). Compared with the NMC group, the SUVmax increased by 28% and 36%, the SUVmean increased by 26% and 32%, and the volume reduced by 35% and 38% in the DDGMC and VSMMC groups, respectively. Figure 2 shows that the curves of SUVmax, SUVmean, and volumes were close or largely overlapped for DDGMC and VSMMC, and the dependency on motion amplitudes was minimal compared with NMC. In comparison with the motionless group, the SUVmax decreased 32%, 15%, and 10%, the SUVmean decreased 31%, 13%, and 9%, and the volume increased 100%, 19%, and 14% in the NMC, DDGMC, and VSMMC groups, respectively.
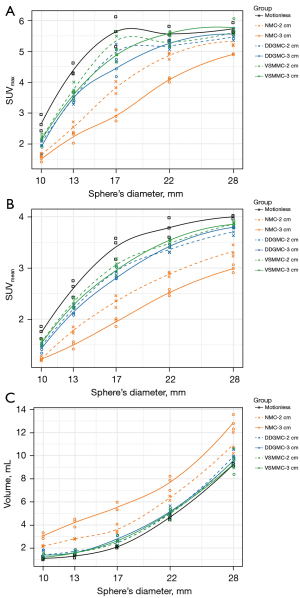
Patient study
A total of 58 patients were enrolled (age: 61±13 years; weight: 60±14 kg; height: 1.65±0.07 m), of whom 42 and 16 patients underwent 2-[18F]FDG and [68Ga]Ga-DOTA-NOC PET/CT, respectively. There were 74 2-[18F]FDG avid lesions and 30 [68Ga]Ga-DOTA-NOC avid lesions included in the quantitative analysis. The lesions’ diameters were 1.46±0.44 (range, 0.83–2.91) and 1.38±0.46 (range, 0.91–3.13) cm in the 2-[18F]FDG and [68Ga]Ga-DOTA-NOC groups, respectively, as measured on DDGMC images.
Quantitative measurements of the patient data
The image noise metrics were measured by the SD and COV in the liver. They were not statistically different between the DDGMC, VSMMC, and NMC groups (Tables 1,2 for the 2 tracers). There was no difference in SUVmean of the liver between the 3 reconstruction groups in [68Ga]Ga-DOTA-NOC PET. In contrast, in the 2-[18F]FDG group, the liver SUVmean in DDGMC was less than that in VSMMC and NMC (both P values =0.04). However, this difference was small (the median SUV difference was −0.02 and −0.04), which explained why no difference was found in the COV.
Table 1
Metrics | Difference median | P value | |||||
---|---|---|---|---|---|---|---|
DDGMC-NMC | VSMMC-NMC | DDGMC-VSMMC | DDGMC vs. NMC | VSMMC vs. NMC | DDGMC vs. VSMMC | ||
Liver ΔSUVmean | −0.04 (–0.06, −0.01) | −0.01 (−0.04, 0.02) | −0.02 (−0.03, −0.01) | 0.04 | 0.46 | 0.04 | |
Liver ΔSD | 0.02 (−3.44e-05, 0.03) | 0.02 (−2.54e-05, 0.04) | −0.01 (−0.02, 0.01) | 0.15 | 0.15 | 0.38 | |
Liver ΔCOV (%) | 0.57 (−0.04, 1.34) | 0.70 (−0.06, 1.40) | −0.11 (−0.44, 0.23) | 0.11 | 0.11 | 0.47 | |
Lesion ΔSUVmax | 0.90 (0.60, 1.30) | 0.91 (0.59, 1.34) | 0.00 (−0.08, 0.07) | <0.001 | <0.001 | 0.97 | |
Relative lesion ΔSUVmax (%)* | 11.08 (7.47, 14.86) | 10.87 (7.24, 15.69) | 0.07 (−0.75, 0.76) | <0.001 | <0.001 | 0.86 | |
Lesion ΔSUVmean | 0.62 (0.43, 0.90) | 0.69 (0.46, 1.01) | −0.02 (−0.07, 0.03) | <0.001 | <0.001 | 0.44 | |
Relative lesion ΔSUVmean (%)* | 11.68 (8.18, 15.03) | 12.65 (8.63, 16.49) | −0.15 (−1.04, 0.56) | <0.001 | <0.001 | 0.66 | |
Lesion Δvolume | −0.30 (−0.40, −0.15) | −0.25 (−0.40, −0.15) | 0.00 (−0.10, 0.05) | <0.001 | <0.001 | 0.4 | |
Relative lesion Δvolume (%)* | −15.18 (−22.12, −9.17) | −14.88 (−22.50, −9.07) | 1.04 (−3.60, 5.55) | <0.001 | <0.001 | 0.66 |
Numbers in parentheses are 95% confidence intervals. *, the values are normalized by NMC and are presented as percentages. SUV, standardized uptake value; 2-[18F]FDG PET/CT, 2-deoxy-2-[18F]fluoro-D-glucose positron emission tomography/computed tomography; DDGMC, data-driven gating-based motion correction; NMC, no motion correction; VSMMC, vital signal module-based motion correction; SD, standard deviation; COV, coefficient of variation.
Table 2
Metrics | Difference median | P value | |||||
---|---|---|---|---|---|---|---|
DDGMC-NMC | VSMMC-NMC | DDGMC-VSMMC | DDGMC vs. NMC | VSMMC vs. NMC | DDGMC vs. VSMMC | ||
Liver ΔSUVmean | 0.06 (−0.12, 0.23) | 0.06 (−0.13, 0.25) | −0.01 (−0.06, 0.05) | 0.59 | 0.59 | 0.59 | |
Liver ΔSD | 0.04 (−0.03, 0.11) | 0.06 (−0.01, 0.12) | 0.04 (−0.03, 0.11) | 0.28 | 0.25 | 0.28 | |
Liver ΔCOV (%) | 0.55 (−0.44, 1.27) | 0.83 (−0.30, 1.86) | −0.33 (−0.83, 0.16) | 0.40 | 0.32 | 0.38 | |
Lesion ΔSUVmax | 5.16 (2.90, 7.40) | 5.49 (3.25, 7.76) | 0.16 (−0.11, 0.44) | <0.001 | <0.001 | 0.3 | |
Relative lesion ΔSUVmax (%)* | 14.23 (7.99, 25.83) | 14.77 (9.04, 25.71) | 0.73 (−0.23, 2.27) | <0.001 | <0.001 | 0.14 | |
Lesion ΔSUVmean | 3.75 (2.05, 5.54) | 3.88 (2.29, 5.63) | 0.11 (−0.03, 0.29) | <0.001 | <0.001 | 0.11 | |
Relative lesion ΔSUVmean (%)* | 15.30 (8.75, 26.86) | 15.45 (10.27, 26.75) | 0.78 (−0.17, 1.99) | <0.001 | <0.001 | 0.13 | |
Lesion Δvolume | −0.30 (−0.60, −0.10) | −0.35 (−0.75, −0.15) | 0.00 (−0.15, 0.20) | <0.01 | <0.001 | 0.85 | |
Relative lesion Δvolume (%)* | −19.11 (−35.28, −8.14) | −20.94 (−34.72, −9.52) | −1.39 (−7.70, 6.67) | <0.01 | <0.001 | 0.66 |
Numbers in parentheses are 95% confidence intervals. *, the values are normalized to NMC and are presented as percentages. SUV, standardized uptake value; [68Ga]Ga-DOTA-NOC PET/CT, 68Ga-labeled [1,4,7,10-tetraazacyclododecane-1,4,7,10-tetraacetic acid]-1-Nal3-octreotide positron emission tomography/computed tomography; DDGMC, data-driven gating-based motion correction; NMC, no motion correction; VSMMC, vital signal module-based motion correction; SD, standard deviation; COV, coefficient of variation.
The lesions’ SUVmax and SUVmean increased and the volumes reduced with DDGMC and VSMMC compared with NMC reconstruction (all P<0.01). In the 2-[18F]FDG group, SUVmax and SUVmean had a median increase of 0.62–0.91 in DDGMC and VSMMC compared with NMC. In the [68Ga]Ga-DOTA-NOC group, SUVmax and SUVmean had a median increase of 3.75–5.49 in DDGMC and VSMMC compared with NMC. The ΔSUVs were larger in the [68Ga]Ga-DOTA-NOC than in the 2-[18F]FDG group, which might have been due to the higher uptake values in the [68Ga]Ga-DOTA-NOC group than in the 2-[18F]FDG group (Figure 3A-3D). After normalization to NMC, the relative increases of SUVmax and SUVmean exhibited a much less pronounced difference (10.87–12.65% vs. 14.23–15.45%) between the 2 tracer groups. There was no difference in SUVs and volumes between DDGMC and VSMMC. The volumes reduced by 0.30 and 0.25 mL (15.18% and 14.88%) in 2-[18F]FDG DDGMC and VSMMC compared with 2-[18F]FDG NMC reconstruction. In contrast, the volumes reduced by 0.30 and 0.35 mL (19.11% and 20.94%) in [68Ga]Ga-DOTA-NOC DDGMC and VSMMC reconstructions. The difference in relative volume change between 2-[18F]FDG and [68Ga]Ga-DOTA-NOC might have occurred because there were more smaller lesions in the latter group than in the former (Figure 3E,3F). The details of ΔSUVmax, ΔSUVmean, and Δvolume, and P values of the statistical analysis are listed in Tables 1,2 for the 2-[18F]FDG and [68Ga]Ga-DOTA-NOC groups, respectively.
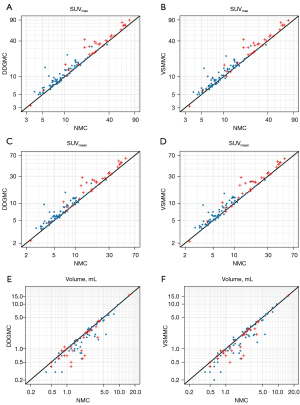
Visual motion assessment of the patient data
During the visual diaphragm motion assessment, radiologists rated more cases with mild or severe motions in NMC compared with DDGMC and VSMMC (25.9% vs. 1.7% vs. 1.7% in NMC, DDGMC, and VSMMC, respectively, after 2 tracer groups were combined; P<0.05). During the lesion-based motion assessment, radiologists identified a larger number of cases with at least 1 lesion with mild to severe motions in NMC compared to DDGMC and VSMMC (53.4% vs. 0% vs. 0%; P<0.01). No difference was found between DDGMC and VSMMC. Itemized visual motion scores for the 2-[18F]FDG and [68Ga]Ga-DOTA-NOC groups are listed in Table 3. An example of a [68Ga]Ga-DOTA-NOC case with severe diaphragm motion is shown in Figure 4, and an example of a 2-[18F]FDG case with motion artifacts on the lesions in the lung and the liver without apparent diaphragm motion artifact is shown in Figure 5. The interrater agreement of the motion score was substantial (kappa =0.71).
Table 3
Score | Reconstruction | Tracer | Motion | ||
---|---|---|---|---|---|
Non/minor | Mild | Severe | |||
Diaphragm motion score | DDGMC | 2-[18F]FDG | 41 (97.6%) | 0 | 1 (2.4%) |
[68Ga]Ga-DOTA-NOC | 16 (100.0%) | 0 | 0 | ||
VSMMC | 2-[18F]FDG | 41 (97.6%) | 0 | 1 (2.4%) | |
[68Ga]Ga-DOTA-NOC | 16 (100.0%) | 0 | 0 | ||
NMC | 2-[18F]FDG | 32 (76.2%) | 4 (9.5%) | 6 (14.3%) | |
[68Ga]Ga-DOTA-NOC | 11 (68.8%) | 2 (12.5%) | 3 (18.8%) | ||
Lesion motion score | DDGMC | 2-[18F]FDG | 42 (100.0%) | 0 | 0 |
[68Ga]Ga-DOTA-NOC | 16 (100.0%) | 0 | 0 | ||
VSMMC | 2-[18F]FDG | 42 (100.0%) | 0 | 0 | |
[68Ga]Ga-DOTA-NOC | 16 (100.0%) | 0 | 0 | ||
NMC | 2-[18F]FDG | 20 (47.6%) | 5 (11.9%) | 17 (40.5%) | |
[68Ga]Ga-DOTA-NOC | 7 (43.8%) | 2 (12.5%) | 7 (43.8%) |
Numbers are counts of patient cases. DDGMC, data-driven gating-based motion correction; 2-[18F]FDG, 2-deoxy-2-[18F]fluoro-D-glucose; [68Ga]Ga-DOTA-NOC, 68Ga-labeled [1,4,7,10-tetraazacyclododecane-1,4,7,10-tetraacetic acid]-1-Nal3-octreotide; VSMMC, vital signal module-based motion correction; NMC, no motion correction.
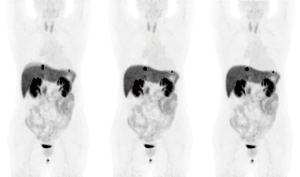
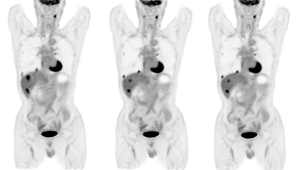
In the pairwise comparisons, the raters gave favorable scores to DDGMC and VSMMC in 45.3% and 43.8% of 2-[18F]FDG and [68Ga]Ga-DOTA-NOC cases, respectively. No preference was found in the comparison of DDGMC and VSMMC. Table 4 shows the results of the pairwise visual image quality comparison scores. The interrater agreement was very good (kappa =0.80).
Table 4
Group pairs | Tracer | Score* | ||||
---|---|---|---|---|---|---|
−2 | −1 | 0 | +1 | +2 | ||
DDGMC vs. NMC | 2-[18F]FDG | 0 | 0 | 23 (54.8%) | 12 (28.6%) | 7 (16.7%) |
[68Ga]Ga-DOTA-NOC | 0 | 0 | 9 (56.3%) | 2 (12.5%) | 5 (31.3%) | |
VSMMC vs. NMC | 2-[18F]FDG | 0 | 0 | 23 (54.8%) | 12 (28.6%) | 7 (16.7%) |
[68Ga]Ga-DOTA-NOC | 0 | 0 | 9 (56.3%) | 2 (12.5%) | 5 (31.3%) | |
DDGMC vs. VSMMC | 2-[18F]FDG | 0 | 0 | 42 (100.0%) | 0 | 0 |
[68Ga]Ga-DOTA-NOC | 0 | 0 | 16 (100.0%) | 0 | 0 |
Numbers are counts of patient cases. *, a score of 0 means no preference, and positive scores represent a better image quality of the former over the later images in an image pair shown in the group pairs column. DDGMC, data-driven gating-based motion correction; NMC, no motion correction; 2-[18F]FDG, 2-deoxy-2-[18F]fluoro-D-glucose; [68Ga]Ga-DOTA-NOC, 68Ga-labeled [1,4,7,10-tetraazacyclododecane-1,4,7,10-tetraacetic acid]-1-Nal3-octreotide; VSMMC, vital signal module-based motion correction.
Discussion
We investigated the impacts of MCIR on tumor quantification and visual image quality. In our phantom study, the SUVs increased by 26–36% while the volumes decreased by 35–38% in DDGMC and VSMMC compared with NMC reconstructions. The SUVs and the volume in the DDGMC and VSMMC groups were much closer to the measurements in the motionless group compared those in the NMC group. In our patient study, the lesions’ SUVs had a median increase of 10.87–15.45%, and the volumes had a median decrease of 14.88–20.94% in DDGMC and VSMMC compared with NMC, while the image noise metrics were not different. In about 45% of patient cases, improvements in respiratory motion correction were appreciated by human observers. Furthermore, no statistically significant differences in SUVs, volume, or visual scores were found between DDGMC and VSMMC reconstructions.
Our results showed that MCIR reduced motion artifacts on tumors in the regions vulnerable to respiratory motions. Respiratory motion is one of the major factors causing motion artifacts in PET/CT (7,19-21). In this study, the observers identified lesion motions in 52.4–56.3% of patients, suggesting respiratory motion correction may potentially bring benefits to many patients. This finding is in line with other studies (5,6,18). However, the impacts of respiratory motions on tumor quantification are case-dependent (2,3). Previous clinical studies found a large variance in respiratory amplitude at the diaphragm that can be from 0.4 to 3.8 cm in a superior-inferior direction depending on breathing patterns (2,22). The average motion extent of a lung tumor is usually in the order of 1 cm (23-25). Our phantom study also showed that the SUV and volume change caused by motion correction were associated with motion amplitudes (Figure 2). This may explain the gaps and variances of relative SUV and volume changes in our phantom and patient study. Therefore, a respiratory motion correction solution with the flexibility to be turned on and off retrospectively will be helpful because the amplitudes of respiratory motions and the benefits of correction efforts vary from patient to patient. Our results suggest that DDG has equivalent efficacy to an external device as the input for MCIR and unimpaired image noise performance as static imaging and uses a relatively short acquisition time. Therefore, it provides a robust deviceless respiratory motion correction solution that can be adopted easily in real-world practice.
Our results showed that MCIR improved the quantification accuracy of lesions’ SUVs and volumes caused by respiratory motion artifacts, which agrees with previous findings in other studies that used elastic respiratory motion correction algorithms (13,14,23,26). Pösse et al. (23) reported that compared with uncorrected images, a gate-to-gate elastic motion compensation (G2G-MC) and an elastic motion deblurring (EMDB) algorithm yielded a 12% and 11% increase in lesion SUVs and an 18% and 28% decrease in lesion volume in 28 patients, respectively. In this study, we found that the SUVs increased by 11–15% while lesion volume decreased by 15–20% in a larger population using MCIR. Our results are more consistent with those of the G2G-MC (23). A plausible explanation may be that MCIR shares more similarities with G2G-MC in algorithm architecture than it does with EMDB. In another study, Meier et al. (14) reported that SUVmax increased by 19.3% in 65 lesions that were less than 3 cm in diameter using EMDB, and the liver noise increased by 15% over static imaging. Compared with their results, we found less SUVmax increase in 104 lesions with a mean diameter of 1.37 cm without degraded liver noise levels compared with static images. We postulate that the SUVmax measured on the MCIR image is less influenced by the image noise while EMDB is subjected to the image noise in the process of forming blurring kernels (14). This may explain the reason for the superior liver noise performance of MCIR. Bouyeure-Petit et al. (13) reported a nonstatistically significant increase in SUVs using the reconstruct-register-averaged (RRA) method compared with nongated images. They suggested that RRA is more meaningful for smaller lesions with a displacement larger than 1.2 cm. In this study, we found a significant increase in SUVs in small lesions. This discrepancy might be the result of the mismatched attenuation correction that arises in RRA approaches (7), but that can be rectified in the MCIR algorithm. Nevertheless, both studies support respiratory motion corrections being applied to small lesions in organs subject to larger respiratory motions.
We investigated the effects of MCIR elastic respiratory motion correction in both 2-[18F]FDG and [68Ga]Ga-DOTA-NOC images. Consistent with the studies using an elastic respiratory motion correction algorithm on 2-[18F]FDG (13,14,23,26), our study found that MCIR improved tumor quantification accuracy and visual image quality without degraded image noise or prolonged acquisition time. However, there is a lack of studies on elastic motion correction using tracers beyond 2-[18F]FDG. Sigfridsson et al. (27) found that QPG with 50% of total coincidences resulted in higher SUVs and smaller tumor volumes compared with nongated images in 68Ga-DOTATOC PET/CT when the acquisition time was doubled from 2 to 4 min per bed. Similar to these results, ours showed that MCIR-corrected [68Ga]Ga-DOTA-NOC PET images had increased SUVs due to reduced motion artifacts. Compared with QPG, MCIR used all counts; therefore, MCIR had better noise performance without needing to increase the acquisition time. Liberini et al. (28) showed that joint QPG and Bayesian penalized likelihood (BPL) reconstruction could increase SUVmax and improve the differential diagnostic performance of neuroendocrine tumors and accessory spleens using 68Ga-DOTATATE PET. BPL reconstruction can increase SUVs and lower image noise compared with OSEM reconstruction. The gains of adding BPL to MCIR should be investigated in further studies. Furthermore, our study showed a larger increase of SUVs in the [68Ga]Ga-DOTA-NOC group than that in the 2-[18F]FDG group. Taken together, these results support the notion that respiratory motion correction may play an important role in PET imaging with tracers besides 2-[18F]FDG. This may be more helpful for tumors that need multi-tracer PET studies, such as neuroendocrine tumors that may need [68Ga]Ga-DOTA-NOC as well as 2-[18F]FDG PET in patient management.
Our results showed that the effects of DDGMC and VSMMC on tumor quantification and visual image quality were not statistically different. Although external devices for respiratory monitoring are commonly provided by vendors, using these devices is not widely accepted in clinical practice due to the complexity associated with the hardware setup. Previous studies (18,29,30) demonstrated that DDG could robustly estimate respiratory motions and even outperform device-based gating (31). Consistent with these studies, our study shows that DDG provides a reliable estimation of the respiratory waveform for gated image reconstruction in MCIR. Additionally, we found a discrepancy in the motion inspection in the per-diaphragm and per-lesion assessment. This result suggests that some motion artifacts may be identified afterward in the image interpretation session by radiologists, which highlights the merit of DDGMC since it can be applied retrospectively on demand by reading physicians. Therefore, we recommend the DDG as the respiratory signal feed-in for MCIR in future studies to investigate the clinical impact of MCIR.
Our study has several limitations. In the phantom study, only the 18F solution, not the 68Ga, was tested. As 68Ga and 18F performed similarly in terms of the image quality in a phantom study using a clinical PET/CT and a PET/magnetic resonance (MR) scanner (32), we postulated that the difference between the two radionuclides would be minimal in the performance of MCIR. Nevertheless, a dedicated phantom study using non-18F radionuclides is warranted, due to the growing use of new radiopharmaceuticals. In the patient study, the patients were enrolled with lesions in the regions prone to respiratory motions. The lesions chosen for the quantitative evaluation tended to be smaller in size and subject to motion artifacts. Further studies with unselective cohorts or lesions are needed to evaluate the clinical impact of MCIR on tumor detection, quantification, and diagnosis. Furthermore, we used OSEM reconstruction with an acquisition time of 3 min per bed position in the chest and upper abdomen according to the vendor’s default settings. The influence of acquisition time and advanced reconstruction algorithms, such as BPL (28) or deep learning techniques (33,34), on MCIR should be studied in the future.
Conclusions
The MCIR reduced respiratory motion artifacts and improved tumor quantification accuracy and visual image quality in 2-[18F]FDG and [68Ga]Ga-DOTA-NOC PET/CT tumor imaging with unimpaired image noise performance compared to nongated images. DDG provides a deviceless solution for MCIR that is as accurate as an external device solution. Therefore, MCIR with DDG has the potential to be adopted in routine practice.
Acknowledgments
Funding: This work was partially supported by the Nanjing Municipal Health Science and Technology Development Fund (No. YKK20104 to L Xu), the Nanjing Municipal Medical and Technology Development Fund (No. QRX11033 to H Jiang), and the NHC Key Laboratory of Nuclear Technology Medical Transformation (No. 2021HYX026 to Z Chen).
Footnote
Conflicts of Interest: All authors have completed the ICMJE uniform disclosure form (available at https://qims.amegroups.com/article/view/10.21037/qims-22-557/coif). RZW, HL, GY, and YD are employees of United Imaging Healthcare. The other authors have no conflicts of interest to declare.
Ethical Statement: The authors are accountable for all aspects of the work in ensuring that questions related to the accuracy or integrity of any part of the work are appropriately investigated and resolved. The study was conducted in accordance with the Declaration of Helsinki (as revised in 2013). The study was approved by the Ethics Committee of the Nanjing First Hospital, and informed consent was obtained from all individual participants.
Open Access Statement: This is an Open Access article distributed in accordance with the Creative Commons Attribution-NonCommercial-NoDerivs 4.0 International License (CC BY-NC-ND 4.0), which permits the non-commercial replication and distribution of the article with the strict proviso that no changes or edits are made and the original work is properly cited (including links to both the formal publication through the relevant DOI and the license). See: https://creativecommons.org/licenses/by-nc-nd/4.0/.
References
- Nehmeh SA, Erdi YE. Respiratory motion in positron emission tomography/computed tomography: a review. Semin Nucl Med 2008;38:167-76. [Crossref] [PubMed]
- Callahan J, Kron T, Schneider-Kolsky M, Hicks RJ. The clinical significance and management of lesion motion due to respiration during PET/CT scanning. Cancer Imaging 2011;11:224-36. [PubMed]
- Liu C, Pierce LA 2nd, Alessio AM, Kinahan PE. The impact of respiratory motion on tumor quantification and delineation in static PET/CT imaging. Phys Med Biol 2009;54:7345-62. [Crossref] [PubMed]
- Frood R, McDermott G, Scarsbrook A. Respiratory-gated PET/CT for pulmonary lesion haracterization-promises and problems. Br J Radiol 2018;91:20170640. [Crossref] [PubMed]
- Guerra L, De Ponti E, Elisei F, Bettinardi V, Landoni C, Picchio M, Gilardi MC, Versari A, Fioroni F, Dziuk M, Koza M, Ahond-Vionnet R, Collin B, Messa C. Respiratory gated PET/CT in a European multicentre retrospective study: added diagnostic value in detection and characterization of lung lesions. Eur J Nucl Med Mol Imaging 2012;39:1381-90. [Crossref] [PubMed]
- Crivellaro C, De Ponti E, Elisei F, Morzenti S, Picchio M, Bettinardi V, Versari A, Fioroni F, Dziuk M, Tkaczewski K, Ahond-Vionnet R, Nodari G, Todde S, Landoni C, Guerra L. Added diagnostic value of respiratory-gated 4D 18F-FDG PET/CT in the detection of liver lesions: a multicenter study. Eur J Nucl Med Mol Imaging 2018;45:102-9. [Crossref] [PubMed]
- Lamare F, Bousse A, Thielemans K, Liu C, Merlin T, Fayad H, Visvikis D. PET respiratory motion correction: quo vadis? Phys Med Biol 2022;67: [Crossref] [PubMed]
- Dawood M, Buther F, Jiang X, Schafers KP. Respiratory motion correction in 3-D PET data with advanced optical flow algorithms. IEEE Trans Med Imaging 2008;27:1164-75. [Crossref] [PubMed]
- Dawood M, Gigengack F, Jiang X, Schafers KP. A mass conservation-based optical flow method for cardiac motion correction in 3D-PET. Med Phys 2013;40:012505. [Crossref] [PubMed]
- Menke M, Atkins MS, Buckley KR. Compensation methods for head motion detected during PET imaging. IEEE Trans Nucl Sci 1996;43:310-7. [Crossref]
- Hong I, Jones J, Casey M. Ultrafast Elastic Motion Correction via Motion Deblurring. In: 2014 IEEE Nuclear Science Symposium and Medical Imaging Conference (NSS/MIC). Seattle, WA, USA: IEEE, 2014.
- Karakatsanis NA, Tsoumpas C, Zaidi H. Quantitative PET image reconstruction employing nested expectation-maximization deconvolution for motion compensation. Comput Med Imaging Graph 2017;60:11-21. [Crossref] [PubMed]
- Bouyeure-Petit AC, Chastan M, Edet-Sanson A, Becker S, Thureau S, Houivet E, Vera P, Hapdey S. Clinical respiratory motion correction software (reconstruct, register and averaged-RRA), for 18F-FDG-PET-CT: phantom validation, practical implications and patient evaluation. Br J Radiol 2017;90:20160549. [Crossref] [PubMed]
- Meier JG, Wu CC, Betancourt Cuellar SL, Truong MT, Erasmus JR, Einstein S, Mawlawi O. Evaluation of a novel elastic respiratory motion correction algorithm on quantification and image quality in abdomino-thoracic PET/CT. J Nucl Med 2018; Epub ahead of print. [Crossref] [PubMed]
- Huang TC, Chou KT, Wang YC, Zhang G. Motion freeze for respiration motion correction in PET/CT: a preliminary investigation with lung cancer patient data. Biomed Res Int 2014;2014:167491. [Crossref] [PubMed]
- Wang J, Feng T, Dong Y, Li H. Automatic Mismatch Correction and Motion Compensation for Free-breathing PET/CT. In: 2018 IEEE Nuclear Science Symposium and Medical Imaging Conference Proceedings (NSS/MIC). Sydney: IEEE, 2018.
- Bai W, Brady M. Regularized B-spline deformable registration for respiratory motion correction in PET images. Phys Med Biol 2009;54:2719-36. [Crossref] [PubMed]
- Kesner AL, Chung JH, Lind KE, Kwak JJ, Lynch D, Burckhardt D, Koo PJ. Validation of Software Gating: A Practical Technology for Respiratory Motion Correction in PET. Radiology 2016;281:239-48. [Crossref] [PubMed]
- Beyer T, Antoch G, Blodgett T, Freudenberg LF, Akhurst T, Mueller S. Dual-modality PET/CT imaging: the effect of respiratory motion on combined image quality in clinical oncology. Eur J Nucl Med Mol Imaging 2003;30:588-96. [Crossref] [PubMed]
- Kang SY, Moon BS, Kim HO, Yoon HJ, Kim BS. The impact of data-driven respiratory gating in clinical F-18 FDG PET/CT: comparison of free breathing and deep-expiration breath-hold CT protocol. Ann Nucl Med 2021;35:328-37. [Crossref] [PubMed]
- Sun T, Mok GS. Techniques for respiration-induced artifacts reductions in thoracic PET/CT. Quant Imaging Med Surg 2012;2:46-52. [PubMed]
- Vedam SS, Kini VR, Keall PJ, Ramakrishnan V, Mostafavi H, Mohan R. Quantifying the predictability of diaphragm motion during respiration with a noninvasive external marker. Med Phys 2003;30:505-13. [Crossref] [PubMed]
- Pösse S, Büther F, Mannweiler D, Hong I, Jones J, Schäfers M, Schäfers KP. Comparison of two elastic motion correction approaches for whole-body PET/CT: motion deblurring vs gate-to-gate motion correction. EJNMMI Phys 2020;7:19. [Crossref] [PubMed]
- Knybel L, Cvek J, Molenda L, Stieberova N, Feltl D. Analysis of Lung Tumor Motion in a Large Sample: Patterns and Factors Influencing Precise Delineation of Internal Target Volume. Int J Radiat Oncol Biol Phys 2016;96:751-8. [Crossref] [PubMed]
- Tahari AK, Lodge MA, Wahl RL. Respiratory-gated PET/CT versus delayed images for the quantitative evaluation of lower pulmonary and hepatic lesions. J Med Imaging Radiat Oncol 2014;58:277-82. [Crossref] [PubMed]
- Minamimoto R, Mitsumoto T, Miyata Y, Sunaoka F, Morooka M, Okasaki M, Iagaru A, Kubota K. Evaluation of a new motion correction algorithm in PET/CT: combining the entire acquired PET data to create a single three-dimensional motion-corrected PET/CT image. Nucl Med Commun 2016;37:162-70. [Crossref] [PubMed]
- Sigfridsson J, Lindström E, Iyer V, Holstensson M, Velikyan I, Sundin A, Lubberink M. Prospective data-driven respiratory gating of [68Ga]Ga-DOTATOC PET/CT. EJNMMI Res 2021;11:33. [Crossref] [PubMed]
- Liberini V, Kotasidis F, Treyer V, Messerli M, Orita E, Engel-Bicik I, Siebenhüner A, Huellner MW. Impact of PET data driven respiratory motion correction and BSREM reconstruction of 68Ga-DOTATATE PET/CT for differentiating neuroendocrine tumors (NET) and intrapancreatic accessory spleens (IPAS). Sci Rep 2021;11:2273. [Crossref] [PubMed]
- Walker MD, Morgan AJ, Bradley KM, McGowan DR. Evaluation of data-driven respiratory gating waveforms for clinical PET imaging. EJNMMI Res 2019;9:1. [Crossref] [PubMed]
- Feng T, Wang J, Sun Y, Zhu W, Dong Y, Li H. Self-Gating: An Adaptive Center-of-Mass Approach for Respiratory Gating in PET. IEEE Trans Med Imaging 2018;37:1140-8. [Crossref] [PubMed]
- Walker MD, Morgan AJ, Bradley KM, McGowan DR. Data-Driven Respiratory Gating Outperforms Device-Based Gating for Clinical 18F-FDG PET/CT. J Nucl Med 2020;61:1678-83. [Crossref] [PubMed]
- Soderlund AT, Chaal J, Tjio G, Totman JJ, Conti M, Townsend DW. Beyond 18F-FDG: Characterization of PET/CT and PET/MR Scanners for a Comprehensive Set of Positron Emitters of Growing Application—18F, 11C, 89Zr, 124I, 68Ga, and 90Y. J Nucl Med 2015;56:1285-91. [Crossref] [PubMed]
- Xue H, Zhang Q, Zou S, Zhang W, Zhou C, Tie C, Wan Q, Teng Y, Li Y, Liang D, Liu X, Yang Y, Zheng H, Zhu X, Hu Z. LCPR-Net: low-count PET image reconstruction using the domain transform and cycle-consistent generative adversarial networks. Quant Imaging Med Surg 2021;11:749-62. [Crossref] [PubMed]
- Teng X, Chen Y, Zhang Y, Ren L. Respiratory deformation registration in 4D-CT/cone beam CT using deep learning. Quant Imaging Med Surg 2021;11:737-48. [Crossref] [PubMed]