Tumor metabolism derived from 18F-FDG PET/CT in predicting the macrotrabecular-massive subtype of hepatocellular carcinoma
Introduction
Hepatocellular carcinoma (HCC), which accounts for the majority of primary liver cancers, ranks as the sixth most commonly diagnosed cancer and the third leading cause of cancer death (1). A large proportion of HCC patients are diagnosed at an advanced stage, and the remaining patients who can receive surgical treatment experience high rates of tumor recurrence (2). Indeed, the clinical prognosis of HCC patients is poor and varies widely among individuals. Individualized therapy based solely on the Barcelona Clinic Liver Cancer (BCLC) stage is far from sufficient because HCC is highly heterogeneous in terms of the genome, molecule, and histology (3,4).
Stratification of HCC according to different molecular or pathological features may be beneficial for developing individualized treatments and assessing the prognosis of patients (5,6). The macrotrabecular-massive (MTM) HCC is characterized by a thick trabecula of more than 6–10 cells (7,8). This special histopathological subtype has been newly enrolled in the fifth edition of the World Health Organization (WHO) Classification of Tumors of the Digestive System (9). The MTM subtype is associated with aggressive molecular and biological characteristics, including TP53 mutations, FGF19 amplification, higher alpha-fetoprotein (AFP) levels, larger tumor diameters, and vascular invasion (10). Previous research has indicated that the MTM subtype is an independent risk factor for early recurrence and poor overall survival (8,10). Therefore, preoperative identification of this aggressive subtype of HCC may facilitate decision-making for individualized treatment and prognosis prediction.
In addition to the risk of hemorrhage and tumor cell spread, a histopathological biopsy is not a necessary step for diagnosing HCC. Therefore, noninvasive preoperative identification of aggressive HCC with imaging methods is crucial. Currently, β-2-[18F] fluoro-2-deoxy-D-glucose positron emission tomography with computed tomography (18F-FDG PET/CT) has shown to be a valuable molecular imaging tool when evaluating the biological behavior of tumors (11-13). The FDG uptake in HCC lesions is reportedly associated with more aggressive characteristics, such as a higher histological grade, the presence of microvascular invasion (MVI), early recurrence, and poor overall survival (14-16). Thus, we aimed to evaluate the potential to use tumor FDG metabolism as an imaging indicator to preoperatively predict the MTM subtype in HCC patients. We present the following article in accordance with the STROBE reporting checklist (available at https://qims.amegroups.com/article/view/10.21037/qims-22-523/rc).
Methods
Study design
This is a retrospective study evaluating the potential tumor metabolism obtained by 18F-FDG PET/CT as a preoperative imaging indicator for predicting MTM-HCCs. The study was conducted in accordance with the Declaration of Helsinki (as revised in 2013). This study was approved by the Ethics Committee of The Third Affiliated Hospital, Sun Yat-sen University (No. [2022]02-161-01), and individual consent for this retrospective analysis was waived.
Patients
Patients with liver lesions who underwent surgical resection or liver transplantation and preoperative 18F-FDG PET/CT between June 2015 and June 2021 were identified from the institutional database. Patients were included if they satisfied the following criteria: (I) they had received 18F-FDG PET/CT within 1 month before surgery; (II) they were gross pathologically confirmed to have primary HCC after surgery, including hematoxylin-eosin staining; and (III) they had available clinical data and pathological specimens. Patients were excluded if they had previously received anti-tumoral treatment, had a history of other malignant tumors, and/or had suboptimal radiological or pathological image quality for interpretation. The flowchart of patient selection is displayed in Figure 1. Each patient’s surgical therapy was validated and planned through the hepatobiliary multidisciplinary meeting. Recorded clinical data were age, sex, BCLC stage, hepatitis B virus (HBV) infection, preoperative serum AFP levels (divided into ≤400 and >400 ng/mL) (17,18), prothrombin time (PT), total bilirubin (TB), aspartate transaminase (AST), alanine aminotransferase (ALT), platelet count (PLT), and albumin (ALB). The traditional image feature of substantial necrosis based on CT or magnetic resonance imaging (MRI) was also recorded to compare with the 18F-FDG PET/CT. The detailed criteria for evaluating substantial necrosis are provided in the supplementary materials (Appendix 1).
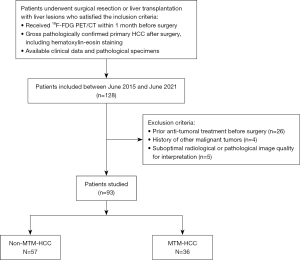
Pathological analysis
All available histological sections were independently evaluated by 2 pathologists with more than 5 years of experience in abdominal pathology. Occasional inconsistencies were discussed to reach a consensus. The presence of the MTM subtype was defined as a predominant macrotrabecular architectural pattern (>6 cells thick) involving more than 50% of the tumor (8,10). Tumor differentiation, the presence of MVI, the presence of cirrhosis, and the expression of nuclear protein Ki-67 were also recorded.
18F-FDG PET/CT acquisition and analysis
All patients were requested to fast for at least 6 hours. Blood glucose levels were measured and were required to be less than 200 mg/dL (11.1 mmol/L) before the patient received the 18F-FDG injection. PET/CT was performed using a Discovery Elite scanner (GE Healthcare, Milwaukee, WI, USA). A diagnostic and non-enhanced CT scan (120 kV, 120 mA, and slice thickness 3.75 mm) was performed 60 minutes after intravenous injection of 3.70–5.55 MBq/kg 18F-FDG. Then, whole-body PET imaging (2 min per bed position; 7–9 beds) from the skull to the thighs was conducted. PET images were reconstructed by iterative reconstruction algorithms using CT images for attenuation correction [ordered subset expectation maximization (OSEM) algorithm and a VUE Point FX module (GE healthcare, 3 iterations, 24 subsets)].
For the semiquantitative analysis, the maximum normalized uptake value (SUVmax) of the primary tumor was measured using a spherical volume of interest on the primary tumor. In the instance of FDG non- or low-avid tumors, the location and extent of the primary tumor on the PET images were determined by the correlation of CT or MR images. For patients with multiple lesions, only the lesion with the highest FDG uptake (SUVmax) was enrolled for analysis, which was matched with the gross pathological specimen based on location and size. The mean SUV of the normal liver was measured by averaging the 3 regions of interest (ROIs; two in the right lobe and one in the left lobe) of 1 cm diameter placed at the location of normal liver tissue, avoiding areas such as focal changes of fatty liver, major vessels and bile ducts, and liver surface margins. The tumor FDG metabolism measured by the tumor-to-normal liver standardized uptake value ratio (TLR) was obtained with the following equation: TLR = SUVmax of the tumor/mean SUV of the normal liver.
Statistical analysis
Continuous variables were represented by the mean with the standard deviation or by the median with the interquartile range (IQR) if they were not normally distributed. Categoric variables were represented by numbers with percentages. Either the Student’s t-test or Mann-Whitney U test was performed for continuous variables. The chi-square test or Fisher’s exact test was used to compare frequencies of categoric variables between MTM-HCCs and non-MTM-HCCs. Receiver operating characteristic (ROC) curves were used to identify the threshold of continuous variables in predicting MTM-HCCs. Variables with statistical significance in the univariate analyses were included in multivariate binary logistic regression to screen for independent risk factors for MTM-HCCs. A nomogram was established for the regression model. The DeLong test was used to compare the AUCs of image features based on 18F-FDG PET/CT and traditional imaging to assess their diagnostic efficacy (19). A 2-sided P value <0.05 was considered indicative of a statistically significant difference. The software SPSS 25.0 (IBM Corp., Armonk, NY, USA), R software (version 4.1.3; The R Foundation for Statistical Computing, Vienna, Austria), and MedCalc (version 20.0; MedCalc, Ostend, Belgium) were used for statistical analysis.
Results
Clinical and pathological characteristics
Ninety-three patients (81 males, 12 females), including 36 MTM-HCCs and 57 non-MTM-HCCs were finally enrolled in this study. HBV infection was observed in 78 patients (83.9%). Fifty-six (60.2%) patients were in BCLC stage 0 or A and 37 (39.8%) patients were in BCLC stage B or C. The pathologic findings showed the presence of cirrhosis in 69 patients (74.2%) in total. The majority of MTM-HCCs were moderately-differentiated (32/36, 88.9%), and none of MTM-HCCs were well-differentiated. MTM-HCCs showed higher AFP levels, worse tumor differentiation, and higher expression of Ki-67 than non-MTM-HCCs. MVI was more common in MTM-HCCs than in non-MTM-HCCs. Other characteristics were not statistically significantly different between MTM-HCCs and non-MTM-HCCs (Table 1). Representative images of MTM-HCCs are shown in Figure 2.
Table 1
Characteristics | Non-MTM-HCCs (n=57) | MTM-HCCs (n=36) | P value |
---|---|---|---|
Clinical | |||
Age (years) | 54.8±10.2 | 50.7±12.6 | 0.138 |
Sex | 0.682 | ||
Male | 49 (86.0) | 32 (88.9) | |
Female | 8 (14.0) | 4 (11.1) | |
BCLC stage | 0.110 | ||
0–A | 38 (66.7) | 18 (50.0) | |
B–C | 19 (33.3) | 18 (50.0) | |
HBV infection | 0.911 | ||
Present | 48 (84.2) | 30 (83.3) | |
Absent | 9 (15.7) | 6 (16.7) | |
AFP (ng/mL) | 0.007 | ||
≤400 | 44 (77.2) | 18 (50.0) | |
>400 | 13 (22.8) | 18 (50.0) | |
PT (second) | 14.6 (13.3–17.5) | 14.1 (13.2–15.5) | 0.132 |
TB (μmol/L) | 16.2 (8.3–29.8) | 10.4 (7.6–35.9) | 0.513 |
AST (IU/L) | 37.0 (28.0–64.0) | 56.5 (30.0–94.3) | 0.049 |
ALT (IU/L) | 32.0 (21.0–54.0) | 32.5 (21.3–62.3) | 0.776 |
ALB (g/L) | 38.1 (32.4–41.4) | 38.3 (33.8–41.2) | 0.622 |
PLT (×103/μL) | 115 [56–192] | 165 [121–244] | 0.002 |
Pathological | |||
Tumor differentiation | 0.025 | ||
Well | 10 (17.5) | 0 | |
Moderately | 40 (70.2) | 32 (88.9) | |
Poorly | 7 (12.3) | 4 (11.1) | |
MVI | 0.024 | ||
Present | 26 (45.6) | 25 (69.4) | |
Absent | 31 (54.4) | 11 (30.6) | |
Cirrhosis | 0.730 | ||
Present | 43 (75.4) | 26 (72.2) | |
Absent | 14 (24.6) | 10 (27.8) | |
Ki-67 | 15 [10–30] | 30 [20–40] | 0.001 |
Imaging | |||
Substantial necrosis | 7 (12.3) | 19 (52.8) | <0.001 |
Number of tumors | 0.279 | ||
1 | 35 (61.4) | 18 (50.0) | |
≥2 | 22 (38.6) | 18 (50.0) | |
Tumor size (mm) | 37.0 (27.5–62.0) | 56.0 (35.3–109.8) | 0.003 |
TLR | 1.66 (1.41–2.20) | 2.63 (2.08–3.64) | <0.001 |
Normally distributed continuous variables are represented as mean ± standard deviation; abnormally distributed continuous variables are represented as median (interquartile range); categorical variables are represented as the number of cases (percentage). MTM, macrotrabecular massive; HCC, hepatocellular carcinoma; BCLC, Barcelona Clinic Liver Cancer; HBV, hepatitis B virus; AFP, alpha, fetoprotein; PT, prothrombin time; TB, total bilirubin; AST, aspartate transaminase; ALT, alanine aminotransferase; ALB, albumin; PLT, platelet count; MVI, microvascular invasion; TLR, the tumor-to-normal liver standardized uptake value ratio.
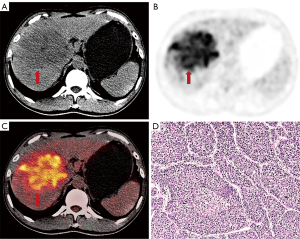
Of the 93 patients enrolled in this study, 31 received enhanced CT only, 45 received MRI examinations only, and 17 received both enhanced CT and MRI before surgery. Substantial necrosis based on traditional imaging was more frequently observed in MTM-HCCs (19/36, 52.8%) than in non-MTM-HCCs (7/57, 12.3%; Table 1).
Association between PET/CT characteristics and MTM-HCCs
Qualitative analyses showed no statistical difference in the number of tumors between MTM-HCCs and non-MTM-HCCs patients (χ2=1.171; P=0.279; Table 1). In the quantitative analysis, MTM-HCCs were with larger tumor size [median (IQR), 56.0 (35.3–109.8) vs. 37.0 (27.5–62.0); Z=–2.951; P=0.003] and larger TLR [median (IQR), 1.66 (1.41–2.20) vs. 2.63 (2.08–3.64); Z=–4.540; P<0.001; Table 1]. We performed a stratified analysis of HCC patients according to the degree of tumor differentiation. The results showed that there was a statistical difference in TLR between MTM-HCCs and non-MTM-HCCs in both moderately and poorly differentiated subgroups (Table S1). TLR of MTM-HCCs was higher than that of non-MTM-HCCs in both subgroups.
The performance of the predictive model
The best cutoff value to predict MTM-HCCs was estimated to be ≥52 IU/L for AST, ≥118.5×103/µL for PLT, ≥2.2 for TLR, and ≥47.5 mm for tumor size according to corresponding ROC analyses. Clinical and PET/CT characteristics with statistical significance in univariate analyses were included in multivariate binary logistic regression. Multivariate binary logistic regression analyses identified AST ≥52 IU/L [odds ratio (OR) =4.15; 95% confidence interval (CI), 1.34–14.33; P=0.017], PLT ≥118.5×103/µL (OR =3.63; 95% CI: 1.13–12.87; P=0.035), and TLR ≥2.2 (OR =5.55; 95% CI: 1.90–17.56; P=0.002) as independent predictors of MTM-HCCs.
Diagnostic performances of each independent indicator in the multivariate binary logistic regression analysis, the combination of all three indicators, and the regression-based model in predicting MTM-HCCs are shown in Table 2. When TLR was greater than or equal to 2.2, 72.2% of the MTM-HCCs were identified (sensitivity) with a specificity of 75.4%. The AUC for TLR ≥2.2 to identify MTM-HCCs was 0.738 (95% CI: 0.631–0.845). A higher PLT (≥118.5×103/µL) enabled the identification of MTM-HCCs with a sensitivity of 77.8% and a specificity of 54.4%. The AUC for PLT ≥118.5×103/µL to identify MTM-HCCs was 0.661 (95% CI: 0.548–0.773). When AST was greater than or equal to 52 IU/L, 61.1% of the MTM-HCCs were identified (sensitivity) with a specificity and AUC of 68.4% and 0.648 (95% CI: 0.531–0.764), respectively. When all three indicators were satisfied, the specificity reached 98.2%, with sensitivity and AUC of 36.1% and 0.672 (95% CI: 0.552–0.792), respectively. The regression-based model demonstrated a higher AUC (0.835; 95% CI: 0.746–0.923) than single indicators and straightforward combinations of indicators. The nomogram of the regression-based model is shown in Figure 3.
Table 2
Indicator/models | Sensitivity | Specificity | PPV | NPV | Accuracy | AUC |
---|---|---|---|---|---|---|
AST ≥52 IU/L | 0.611 | 0.684 | 0.550 | 0.736 | 0.656 | 0.648 (0.531–0.764) |
PLT ≥118.5×103/μL | 0.778 | 0.544 | 0.519 | 0.795 | 0.634 | 0.661 (0.548–0.773) |
TLR ≥2.2 | 0.722 | 0.754 | 0.650 | 0.811 | 0.742 | 0.738 (0.631–0.845) |
All three indicators | 0.361 | 0.982 | 0.929 | 0.709 | 0.742 | 0.672 (0.552–0.792) |
Regression-based model | 0.806 | 0.789 | 0.707 | 0.865 | 0.796 | 0.835 (0.746–0.923) |
Numbers in parentheses are 95% confidence intervals. MTM, macrotrabecular massive; HCC, hepatocellular carcinoma; PPV, positive predictive value; NPV, negative predictive value; AUC, area under the receiver operating characteristic curve; AST, aspartate transaminase; PLT, platelet count; TLR, the tumor-to-normal liver standardized uptake value ratio.
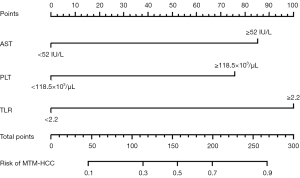
Compared with the diagnostic performance of TLR ≥2.2, substantial necrosis based on traditional images enabled the identification of MTM-HCCs with a sensitivity and a specificity of 52.8% and 87.7%, respectively, with a slightly lower AUC of 0.702 (95% CI: 0.588–0.817). The positive predictive value (PPV), negative predictive value (NPV), and accuracy of substantial necrosis in predicting MTM-HCCs were 73.1%, 74.6%, and 74.2%, respectively. There was no statistical difference between TLR and substantial necrosis in predicting MTM-HCCs using the DeLong test (P>0.05).
Discussion
The recently described pathological subtype of MTM represents the aggressive behavior of HCC and was enrolled into the fifth edition of the WHO classification of digestive tumors (8-10). Recent studies show that MTM-HCCs exhibited strong prognostic significance, which was associated with early recurrence and poor survival in HCC patients undergoing resection or radiofrequency ablation (8,10). Pretreatment identification of this special histopathological subtype may have important therapeutic and prognostic implications. The present study confirms the ability of preoperative 18F-FDG PET/CT to identify the MTM-HCC subtype. Tumor FDG metabolism measured by TLR, PLT, and AST were identified to be independent predictors of MTM-HCCs. TLR sensitively identified 72.2% of MTM-HCCs with a specificity of 75.4%. When these 3 indicators were combined into a regression model, MTM-HCCs could be identified with high sensitivity and good predictive performance.
The expression rate of the MTM subtype (38.7%, 36/93) among all patients with HCCs in this study was similar to that reported by Zhu et al. (36.4%, 32/88) (20) but was higher than in previous studies (16–22.3%) (8,21). As reported, MTM-HCCs were more frequent in HBV-infected patients (22). The patient population included in our study had a relatively higher prevalence of HBV infection than previously reported (21) (83.9% vs. 22.4%).
We found significant correlations between MTM-HCCs and the expression of Ki-67 (P=0.001). MTM-HCCs showed a higher expression of Ki-67, which is one of the most widely used proliferation-associated markers of tumor cells. A previous study indicated that proliferative activity was closely correlated with the glucose metabolism evaluated by FDG-PET (23). Gene expression profiling showed activation of angiogenesis in MTM-HCCs, accompanied by overexpression of angiopoietin 2, vascular endothelial growth factor A (VEGFA), and endothelial-specific molecule 1 (8,10,24). Reports show that the specific neovascularization in MTM-HCCs is associated with a peculiar microvascular pattern named vessels that encapsulate tumor clusters (VETC) with a sinusoid-like microvascular pattern (25,26). Itoh et al. (27) reported that the vascular formation of the VETC pattern was associated with metabolic activity measured by SUVmax. Taken together, we speculated that MTM-HCCs might affect glucose metabolism through cell proliferation and specific angiogenesis and thus can be assessed by FDG PET.
Several studies have found that intratumor necrosis assessed by enhanced CT or MRI images was significantly associated with the MTM subtype, which was also confirmed in our study (21,26,28). Although TLR ≥2.2 in predicting MTM-HCCs was not statistically significant compared with substantial necrosis based on traditional images, our findings suggest that tumor FDG metabolism measured by TLR can be used as an assistant method for predicting MTM-HCCs with relatively high sensitivity and good predictive performance.
In this study, we identified high platelet count as an independent predictor of MTM-HCCs, which was consistent with the recent report by Chen et al. (28). Similar to the conjecture of Calderaro et al. (29), we believe that the high platelet count in MTM-HCCs may be related to its activation of angiogenesis. As a potent multifunctional cytokine that can induce angiogenesis and increase microvascular permeability, VEGF, is mainly derived from platelets and released by physiological stimulation during platelet aggregation (30). Although there is no consensus on the role of platelets in the development and growth of HCC, several studies have demonstrated the contribution of PLT to the angiogenesis and invasiveness of malignant tumors (31,32).
As an indicator of impaired liver function, our results found that the AST level was an independent predictor of MTM-HCCs. Although the AST levels between MTM-HCCs and non-MTM-HCCs in a previous study by Chen et al. (28) did not show a statistical difference (P=0.064), MTM-HCCs showed higher AST levels than non-MTM-HCCs [median (IQR), 37.00 (28.00–50.25) vs. 43.00 (30.50–71.00)], which was consistent with the trend in our study. AST mainly exists in the mitochondria of hepatocytes, and its elevated levels usually indicate that the organelles of hepatocytes have been damaged, which may be associated with the invasion of hepatic carcinoma cells. However, the exact mechanisms underlying increased AST levels in MTM-HCCs are poorly understood.
Certain limitations in this study should be acknowledged. First, our study had a retrospective design, which may have contributed to selection bias. The results of this study might not be representative of the entire clinical spectrum with the involvement of only surgery resected lesions. Second, the sample size was relatively small, and the samples were derived from a single institution, potentially limiting the external validation of our results. Although we had strict criteria for evaluating substantial necrosis, this could be a bias because substantial necrosis was assessed by 2 different traditional imaging (CT or MRI). Therefore, the comparison between CT or MRI images alone and 18F-FDG PET/CT needs to be verified by in-depth studies based on larger sample sizes. Finally, the pathophysiological mechanism of the development of MTM-HCCs has not been explored in depth, and the relationship between the tumor FDG avidity and the MTM subtype should be further evaluated.
This is the first known study to explore the correlation between tumor glucose metabolism and the MTM-HCC subtype. This study revealed that FDG avidity measured by TLR was a valuable radiographic quantitative indicator of MTM-HCCs. Combining regression models enables noninvasive prediction of MTM-HCCs with good sensitivity and excellent predictive performance.
Acknowledgments
The authors sincerely thank all participants and the technicians for FDG radiolabeling and PET/CT scanning.
Funding: None.
Footnote
Reporting Checklist: The authors have completed the STROBE reporting checklist. Available at https://qims.amegroups.com/article/view/10.21037/qims-22-523/rc
Conflicts of Interest: All authors have completed the ICMJE uniform disclosure form (available at https://qims.amegroups.com/article/view/10.21037/qims-22-523/coif). The authors have no conflicts of interest to declare.
Ethical Statement: The authors are accountable for all aspects of the work in ensuring that questions related to the accuracy or integrity of any part of the work are appropriately investigated and resolved. This study was conducted following the Declaration of Helsinki (as revised in 2013). It was approved by the Institutional Review Board at The Third Affiliated Hospital of Sun Yat-sen University (No. [2022]02-161-01). Owing to the retrospective nature of the study, individual consent for this analysis was waived.
Open Access Statement: This is an Open Access article distributed in accordance with the Creative Commons Attribution-NonCommercial-NoDerivs 4.0 International License (CC BY-NC-ND 4.0), which permits the non-commercial replication and distribution of the article with the strict proviso that no changes or edits are made and the original work is properly cited (including links to both the formal publication through the relevant DOI and the license). See: https://creativecommons.org/licenses/by-nc-nd/4.0/.
References
- Forner A, Reig M, Bruix J. Hepatocellular carcinoma. Lancet 2018;391:1301-14. [Crossref] [PubMed]
- Kumari R, Sahu MK, Tripathy A, Uthansingh K, Behera M. Hepatocellular carcinoma treatment: hurdles, advances and prospects. Hepat Oncol 2018;5:HEP08. [Crossref] [PubMed]
- Calderaro J, Ziol M, Paradis V, Zucman-Rossi J. Molecular and histological correlations in liver cancer. J Hepatol 2019;71:616-30. [Crossref] [PubMed]
- Nault JC, Martin Y, Caruso S, Hirsch TZ, Bayard Q, Calderaro J, et al. Clinical Impact of Genomic Diversity From Early to Advanced Hepatocellular Carcinoma. Hepatology 2020;71:164-82. [Crossref] [PubMed]
- Uenishi T, Kubo S, Yamamoto T, Shuto T, Ogawa M, Tanaka H, Tanaka S, Kaneda K, Hirohashi K. Cytokeratin 19 expression in hepatocellular carcinoma predicts early postoperative recurrence. Cancer Sci 2003;94:851-7. [Crossref] [PubMed]
- Guan DX, Shi J, Zhang Y, Zhao JS, Long LY, Chen TW, Zhang EB, Feng YY, Bao WD, Deng YZ, Qiu L, Zhang XL, Koeffler HP, Cheng SQ, Li JJ, Xie D. Sorafenib enriches epithelial cell adhesion molecule-positive tumor initiating cells and exacerbates a subtype of hepatocellular carcinoma through TSC2-AKT cascade. Hepatology 2015;62:1791-803. [Crossref] [PubMed]
- Tan PS, Nakagawa S, Goossens N, Venkatesh A, Huang T, Ward SC, Sun X, Song WM, Koh A, Canasto-Chibuque C, Deshmukh M, Nair V, Mahajan M, Zhang B, Fiel MI, Kobayashi M, Kumada H, Hoshida Y. Clinicopathological indices to predict hepatocellular carcinoma molecular classification. Liver Int 2016;36:108-18. [Crossref] [PubMed]
- Ziol M, Poté N, Amaddeo G, Laurent A, Nault JC, Oberti F, et al. Macrotrabecular-massive hepatocellular carcinoma: A distinctive histological subtype with clinical relevance. Hepatology 2018;68:103-12. [Crossref] [PubMed]
- Torbenson M, Ng I, YN P, et al. Hepatocellular Carcinoma. In: WHO Classification of Tumours. Digestive System Tumours. 5th edition. Geneva: WHO, 2019:229-39.
- Calderaro J, Couchy G, Imbeaud S, Amaddeo G, Letouzé E, Blanc JF, Laurent C, Hajji Y, Azoulay D, Bioulac-Sage P, Nault JC, Zucman-Rossi J. Histological subtypes of hepatocellular carcinoma are related to gene mutations and molecular tumour classification. J Hepatol 2017;67:727-38. [Crossref] [PubMed]
- Takada Y, Kaido T, Shirabe K, Nagano H, Egawa H, Sugawara Y, et al. Significance of preoperative fluorodeoxyglucose-positron emission tomography in prediction of tumor recurrence after liver transplantation for hepatocellular carcinoma patients: a Japanese multicenter study. J Hepatobiliary Pancreat Sci 2017;24:49-57. [Crossref] [PubMed]
- Hsu CC, Chen CL, Wang CC, Lin CC, Yong CC, Wang SH, Liu YW, Lin TL, Lee WF, Lin YH, Chan YC, Wu YJ, Eng HL, Cheng YF. Combination of FDG-PET and UCSF Criteria for Predicting HCC Recurrence After Living Donor Liver Transplantation. Transplantation 2016;100:1925-32. [Crossref] [PubMed]
- Liu S, Feng Z, Zhang J, Ge H, Wu X, Song S. A novel 2-deoxy-2-fluorodeoxyglucose (18F-FDG) positron emission tomography/computed tomography (PET/CT)-based nomogram to predict lymph node metastasis in early stage uterine cervical squamous cell cancer. Quant Imaging Med Surg 2021;11:240-8. [Crossref] [PubMed]
- Hyun SH, Eo JS, Song BI, Lee JW, Na SJ, Hong IK, Oh JK, Chung YA, Kim TS, Yun M. Preoperative prediction of microvascular invasion of hepatocellular carcinoma using 18F-FDG PET/CT: a multicenter retrospective cohort study. Eur J Nucl Med Mol Imaging 2018;45:720-6. [Crossref] [PubMed]
- Lim C, Salloum C, Chalaye J, Lahat E, Costentin CE, Osseis M, Itti E, Feray C, Azoulay D. 18F-FDG PET/CT predicts microvascular invasion and early recurrence after liver resection for hepatocellular carcinoma: A prospective observational study. HPB (Oxford) 2019;21:739-47. [Crossref] [PubMed]
- Sabaté-Llobera A, Mestres-Martí J, Reynés-Llompart G, Lladó L, Mils K, Serrano T, Cortés-Romera M, Bertran E, Fabregat I, Ramos E. 2-[18F]FDG PET/CT as a Predictor of Microvascular Invasion and High Histological Grade in Patients with Hepatocellular Carcinoma. Cancers (Basel) 2021;13:2554. [Crossref] [PubMed]
- Toso C, Meeberg G, Hernandez-Alejandro R, Dufour JF, Marotta P, Majno P, Kneteman NM. Total tumor volume and alpha-fetoprotein for selection of transplant candidates with hepatocellular carcinoma: A prospective validation. Hepatology 2015;62:158-65. [Crossref] [PubMed]
- Rhee H, Cho ES, Nahm JH, Jang M, Chung YE, Baek SE, Lee S, Kim MJ, Park MS, Han DH, Choi JY, Park YN. Gadoxetic acid-enhanced MRI of macrotrabecular-massive hepatocellular carcinoma and its prognostic implications. J Hepatol 2021;74:109-21. [Crossref] [PubMed]
- DeLong ER, DeLong DM, Clarke-Pearson DL. Comparing the areas under two or more correlated receiver operating characteristic curves: a nonparametric approach. Biometrics 1988;44:837-45. [Crossref] [PubMed]
- Zhu Y, Weng S, Li Y, Yan C, Ye R, Wen L, Zhou L, Gao L. A radiomics nomogram based on contrast-enhanced MRI for preoperative prediction of macrotrabecular-massive hepatocellular carcinoma. Abdom Radiol (NY) 2021;46:3139-48. [Crossref] [PubMed]
- Mulé S, Galletto Pregliasco A, Tenenhaus A, Kharrat R, Amaddeo G, Baranes L, Laurent A, Regnault H, Sommacale D, Djabbari M, Pigneur F, Tacher V, Kobeiter H, Calderaro J, Luciani A. Multiphase Liver MRI for Identifying the Macrotrabecular-Massive Subtype of Hepatocellular Carcinoma. Radiology 2020;295:562-71. [Crossref] [PubMed]
- Jeon Y, Benedict M, Taddei T, Jain D, Zhang X. Macrotrabecular Hepatocellular Carcinoma: An Aggressive Subtype of Hepatocellular Carcinoma. Am J Surg Pathol 2019;43:943-8. [Crossref] [PubMed]
- Kitamura K, Hatano E, Higashi T, Narita M, Seo S, Nakamoto Y, Yamanaka K, Nagata H, Taura K, Yasuchika K, Nitta T, Uemoto S. Proliferative activity in hepatocellular carcinoma is closely correlated with glucose metabolism but not angiogenesis. J Hepatol 2011;55:846-57. [Crossref] [PubMed]
- Villa E, Critelli R, Lei B, Marzocchi G, Cammà C, Giannelli G, et al. Neoangiogenesis-related genes are hallmarks of fast-growing hepatocellular carcinomas and worst survival. Results from a prospective study. Gut 2016;65:861-9. [Crossref] [PubMed]
- Renne SL, Woo HY, Allegra S, Rudini N, Yano H, Donadon M, Viganò L, Akiba J, Lee HS, Rhee H, Park YN, Roncalli M, Di Tommaso L. Vessels Encapsulating Tumor Clusters (VETC) Is a Powerful Predictor of Aggressive Hepatocellular Carcinoma. Hepatology 2020;71:183-95. [Crossref] [PubMed]
- Feng Z, Li H, Zhao H, Jiang Y, Liu Q, Chen Q, Wang W, Rong P. Preoperative CT for Characterization of Aggressive Macrotrabecular-Massive Subtype and Vessels That Encapsulate Tumor Clusters Pattern in Hepatocellular Carcinoma. Radiology 2021;300:219-29. [Crossref] [PubMed]
- Itoh S, Yoshizumi T, Kitamura Y, Yugawa K, Iseda N, Shimagaki T, Nagao Y, Toshima T, Harada N, Kohashi K, Baba S, Ishigami K, Oda Y, Mori M. Impact of Metabolic Activity in Hepatocellular Carcinoma: Association With Immune Status and Vascular Formation. Hepatol Commun 2021;5:1278-89. [Crossref] [PubMed]
- Chen J, Xia C, Duan T, Cao L, Jiang H, Liu X, Zhang Z, Ye Z, Wu Z, Gao R, Shi Y, Song B. Macrotrabecular-massive hepatocellular carcinoma: imaging identification and prediction based on gadoxetic acid-enhanced magnetic resonance imaging. Eur Radiol 2021;31:7696-704. [Crossref] [PubMed]
- Calderaro J, Meunier L, Nguyen CT, Boubaya M, Caruso S, Luciani A, et al. ESM1 as a Marker of Macrotrabecular-Massive Hepatocellular Carcinoma. Clin Cancer Res 2019;25:5859-65. [Crossref] [PubMed]
- Hashiguchi T, Arimura K, Matsumuro K, Otsuka R, Watanabe O, Jonosono M, Maruyama Y, Maruyama I, Osame M. Highly concentrated vascular endothelial growth factor in platelets in Crow-Fukase syndrome. Muscle Nerve 2000;23:1051-6. [Crossref] [PubMed]
- Di Vito C, Navone SE, Marfia G, Abdel Hadi L, Mancuso ME, Pecci A, Crisà FM, Berno V, Rampini P, Campanella R, Riboni L. Platelets from glioblastoma patients promote angiogenesis of tumor endothelial cells and exhibit increased VEGF content and release. Platelets 2017;28:585-94. [Crossref] [PubMed]
- Campanella R, Guarnaccia L, Cordiglieri C, Trombetta E, Caroli M, Carrabba G, La Verde N, Rampini P, Gaudino C, Costa A, Luzzi S, Mantovani G, Locatelli M, Riboni L, Navone SE, Marfia G. Tumor-Educated Platelets and Angiogenesis in Glioblastoma: Another Brick in the Wall for Novel Prognostic and Targetable Biomarkers, Changing the Vision from a Localized Tumor to a Systemic Pathology. Cells 2020;9:294. [Crossref] [PubMed]