Development of a novel nomogram-based model incorporating 3D radiomic signatures and lung CT radiological features for differentiating invasive adenocarcinoma from adenocarcinoma in situ and minimally invasive adenocarcinoma
Introduction
Lung cancer is one of the most common cancers in human society and is directly responsible for the high incidence and mortality rates of cancer worldwide (1). The dominant histological type of lung cancer is adenocarcinoma, whose incidence rate has increased over time (1,2). According to the latest classification, the subtypes of lung adenocarcinoma include atypical adenomatous hyperplasia (AAH), adenocarcinoma in situ (AIS), minimally invasive adenocarcinoma (MIA), and invasive adenocarcinoma (IAC) (3). Owing to the popularization and renewal of computed tomography (CT), the capability to screen pulmonary nodules has developed quickly. Due to the higher risk of disease recurrence for patients with IAC after routine treatment, lobectomy is usually recommended (4,5). However, patients with AIS or MIA have a better prognosis through active monitoring or sublobar resection (6). Nowadays, biopsy or postoperative pathological sections are mainly used in clinical practice to determine the subtypes of adenocarcinoma (7). However, these methods are both invasive and risky, emphasizing the significance of presurgery discrimination for supporting prognostic assessment to optimize clinical decision-making and avoid inappropriate surgery for patients.
Adenocarcinoma frequently presents as pulmonary nodules on CT, including pure ground-glass nodules (pGGNs), mixed ground-glass nodules (mGGNs), and solid nodules (SNs). The radiologist identifies the radiological features of the lesions by observing CT scans. Features such as lobulation, spiculate protuberance, vogue sign, left/right lobe, and lung lobe, are related to the malignancy or tumor histology of GGNs (8-10). 3D radiomic signatures can provide more detailed information extracted from medical images through advanced feature analysis methods (11). Radiomic signatures are calculated by multiple formulae, which can quantify the characteristics of tumor tissue (12). Thus, in a 3D environment, they can provide a comprehensive characterization of the tumor phenotype. Radiomic signatures, such as nodule diameter, CT value, and lesion size, play important roles in the prediction of pathological classification (13-16), pathological staging (17), tumor diagnosis (benign/malignant) (18), treatment response (19), and patient survival (20). Radiomics has been used in the prediction and prognosis of lung cancer (21,22). Some studies use radiomic features and radiological features of multiphase CT to distinguish the subtypes of lung adenocarcinoma (such as the difference between IAC and MIA/AIS, the difference between MIA and AIS). Other studies have focused on radiomic features to explore the feasibility of an auxiliary diagnosis from the perspective of image texture and have yielded exciting results (23,24). Some studies have analyzed the correlation between radiomic features and genetic data, attempting to achieve a breakthrough in radiogenomics (25,26).
In this study, the ability of 3D radiomic signatures combined with radiological features to differentiate IAC from AIS and MIA was investigated, and a novel nomogram was developed to assess the risk of IAC preoperatively. In addition, this study used semiautomatic segmentation software and a nomogram to develop a semiautomatic detection process, thus realizing the standardization of data. The stability of the model was verified by data of different regions and different temporal periods. We present the following article in accordance with the TRIPOD reporting checklist (available at https://qims.amegroups.com/article/view/10.21037/qims-22-491/rc).
Methods
The study was conducted in accordance with the Declaration of Helsinki (as revised in 2013). The study was approved by the ethics board of Shanghai University of Medicine & Health Sciences, and the written informed consent of patients was waived by the ethics committee because the study was a retrospective experiment and did not involve patient privacy.
Patients
The data set was collected from 3 hospitals: Shanghai Public Health Clinical Center (hospital 1), Shanghai Ruijin Hospital (hospital 2), and Ningbo Beilun No. 2 Hospital (hospital 3). In patients who showed pulmonary nodules on chest CT scan, those nodules diagnosed as pulmonary adenocarcinomas based on the pathologic analysis of surgical specimens were selected for analyses. The other inclusion criteria were the following: (I) routine CT examination conducted the month before surgery, (II) maximum tumor diameter of less than 20 mm, and (III) preoperative CT layer thickness of less than 2 mm. Multiple nodules from the same patients were analyzed separately. The exclusion criteria were the following: (I) obvious artifacts around the tumor on CT imaging and (II) the use of contrast medium for CT examination. Meanwhile, the study also collected clinical information from patients (Figure 1).
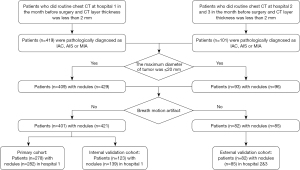
A total of 401 patients with 421 pulmonary nodules (MIA/AIS: n=323, 76.7%; IAC: n=98, 23.3%) from hospital 1 met the inclusion requirements. Moreover, a total of 82 patients with 85 pulmonary nodules (MIA/AIS: n=42, 49.4%; IAC: n=43, 50.6%) nodules from hospital 2 and hospital 3 were selected for inclusion in the study.
CT image acquisition
All the patients in the study underwent an unenhanced chest CT examination. In hospital 1, the CT images were obtained on a UCT 760 scanner (United-Imaging; Shanghai, China; scanning conditions: 42–126 mA, 120 kV, 1-mm slice thickness) and a Siemens Emotion 16 CT scanner (Siemens Healthineers; Erlangen, Germany; scanning conditions: 34–123 mA, 130 kV, 1-mm slice thickness) with a 512×512 resolution. In hospitals 2 and 3, a Philips iCT 256 scanner (Philips Healthcare, Cleveland, OH, USA; scanning conditions: 161 mA, 120 kV, 1-mm slice thickness) and a Philips Brilliance 16 CT scanner (Philips Healthcare; scanning conditions: 219 mA, 120 kV, 1-mm slice thickness) were used for obtaining CT images. All patients performed full inspiration to eliminate artifacts of respiratory movement in the chest CT examination.
3D radiomic signatures and radiological features extraction
All the nodules in selected CT images were segmented by in-house, semiautomatic software (27). All segmentation results were manually examined by a radiologist with 6 years of experience and confirmed by another radiologist with 20 years of experience. After that, a total of 1,600 3D radiomic signatures, including tumor size, shape, first-order statistics of descriptor values (histogram features), and high-order texture features [gray-level co-occurrence matrix (GLCM) and gray-level run length] were extracted from the 3D region of interest (ROI) using open-source software (PyRadiomics v. 3.0.1; Appendix 1) (28).
The radiographic features were assessed independently by the 2 experienced radiologists mentioned above. Any discrepancies in interpretation between the observers were resolved by consensus. The radiological features recorded and analyzed for each lesion were the following: (I) margin (clear, blurred), (II) lobulation (absent, present), (III) spiculation (absent, present), (IV) pleural attachment including pleural tag and indentation (absent, present), (V) air bronchogram (absent, present), (VI) vessel change (absent, present), (VII) bubble lucency (absent, present), (VIII) nodule location (left/right lobe; right upper lobe, right middle lobe, right lower lobe, left upper lobe, left lower lobe), and (IX) major/minor axis (calculated from the ROI area with PyRadiomics software).
Feature selection
A total of 1,611 features of the 3D lesion areas in the CT images were identified, including 1,600 radiomic signatures and 11 radiological features. First, all radiomic signatures were normalized by the scale function. Then, the variance of each feature was calculated and the one close to 0 was excluded. Meanwhile, Spearman pairwise correlation analysis was used to calculate the correlation strength between features. Features whose absolute correlation value was above 0.9 were identified as strong interference features and were removed. After that, the top 100 features were selected using the minimum redundancy maximum relevance (mRMR) method. Then, those features were fed into least absolute shrinkage and selection operator (LASSO) models to select the optimal subsets for evaluating IAC and MIA/AIS. Given the possible randomness, the feature subset was calculated 1,000 times to determine the best result.
Construction and validation of the prediction nomogram
In this study, the predictive factors were screened by analyzing the training set. Multiple logistic regression models were then used to forecast IAC, followed by a combined nomogram-based model. The diagnostic performance of the established predictive nomogram-based model was confirmed by the validation set. The calibration curve and receiver operating characteristic (ROC) curve were plotted, and the area under the curve (AUC) values of the predictive nomogram in the training and validation sets were calculated. To test the diagnostic performance and clinical utility of the combined nomogram, we compared the diagnostic accuracy of (I) the nomogram and the model with radiomic signatures only and (II) the nomogram and the model with radiological features only.
Statistical analysis
Quantitative data are described as mean ± standard deviation or median (25th–75th); qualitative data are described as counts (n). The comparisons between groups for qualitative variables were performed by the Fisher exact test, and comparisons between groups for quantitative variables were performed using the t-test or Wilcoxon test. A 2-sided P value <0.05 was considered statistically significant.
The relevant statistical analysis in the study was performed in the environment of R software v. 4.0.3 (R Foundation for Statistical Computing, Vienna, Austria). The Spearman correlation analysis was performed using the “caret” package. LASSO regression was performed using the “glmnet” package. The “rms” software package was used to complete logistic regression, nomogram construction, and chart calibration. The “rmda” package was used to plot the decision curve.
Results
Patients
A total of 483 patients with 506 pulmonary nodules were divided into the training cohort (278 patients with 282 nodules from April 2015 to February 2017), the testing cohort (123 patients with 139 nodules from February 2015 to December 2016), and the validation cohort (82 patients with 85 nodules from September 2017 to February 2018; Table S1). There were no significant differences in gender or age among the 3 cohorts. There were no statistical differences observed between the IAC group and the MIA/AIS group in segment, margin, spiculation, vessel change, air bronchogram, or bubble lucency. However, differences in lobulation, pleural attachment, and the average major axis were found to be statistically significant. mGGNs were more common and the average major/minor axis was larger in the IAC group than in the MIA/AIS group in the 3 cohorts (Table 1).
Table 1
Characteristics | Training cohort (n=282) | Testing cohort (n=139) | Validation cohort (n=85) | ||||||||
---|---|---|---|---|---|---|---|---|---|---|---|
MIA/AIS (n=216) | IAC (n=66) | P value | MIA/AIS (n=107) | IAC (n=32) | P value | MIA/AIS (n=42) | IAC (n=43) | P value | |||
Age (years) | 50.74±11.19 | 54.60±11.66 | <0.05 | 51.43±11.31 | 53.28±11.34 | <0.05 | 54.02±13.39 | 62.74±11.36 | <0.05 | ||
Gender | 0.211 | 0.1148 | |||||||||
Male | 55 | 22 | 35 | 12 | 28 | 23 | |||||
Female | 161 | 44 | 72 | 20 | 14 | 20 | |||||
Nodule type | <0.05 | <0.05 | <0.05 | ||||||||
pGGN | 152 | 29 | 76 | 10 | 29 | 20 | |||||
mGGN | 64 | 37 | 31 | 22 | 13 | 23 | |||||
Segment | 0.7653 | 0.562 | 0.07993 | ||||||||
Left upper lobe | 62 | 18 | 34 | 7 | 14 | 7 | |||||
Left lower lobe | 35 | 7 | 18 | 4 | 3 | 7 | |||||
Right upper lobe | 73 | 26 | 33 | 15 | 11 | 21 | |||||
Right middle lobe | 16 | 4 | 10 | 2 | 3 | 2 | |||||
Right lower lobe | 30 | 11 | 12 | 4 | 11 | 6 | |||||
Margin | 0.8258 | 0.99 | 0.493 | ||||||||
Blurred | 192 | 58 | 92 | 28 | 33 | 36 | |||||
Clear | 24 | 8 | 15 | 4 | 9 | 7 | |||||
Lobulation | 0.0313 | 0.1693 | 0.0891 | ||||||||
Present | 158 | 57 | 76 | 27 | 3 | 7 | |||||
Absent | 58 | 9 | 31 | 5 | 39 | 36 | |||||
Spiculation | 0.4384 | 0.6686 | 0.2648 | ||||||||
Present | 152 | 50 | 71 | 23 | 34 | 39 | |||||
Absent | 64 | 16 | 36 | 9 | 8 | 4 | |||||
Pleural attachment | <0.05 | <0.05 | 0.3273 | ||||||||
Present | 38 | 31 | 20 | 15 | 19 | 24 | |||||
Absent | 178 | 35 | 87 | 17 | 23 | 19 | |||||
Air bronchogram | 0.0923 | <0.05 | 0.1278 | ||||||||
Present | 95 | 37 | 45 | 21 | 7 | 16 | |||||
Absent | 121 | 29 | 62 | 11 | 35 | 27 | |||||
Vessel change | 0.5801 | 0.6003 | 0.3988 | ||||||||
Present | 180 | 53 | 89 | 25 | 29 | 37 | |||||
Absent | 36 | 13 | 18 | 7 | 13 | 6 | |||||
Bubble lucency | 0.1767 | 0.1877 | 0.09175 | ||||||||
Present | 44 | 19 | 29 | 13 | 3 | 10 | |||||
Absent | 172 | 47 | 78 | 19 | 39 | 33 | |||||
Average major axis (mm) | 8.59±2.31 | 11.02±2.47 | <0.05 | 8.73±2.55 | 11.19±2.80 | <0.05 | 10.80±4.26 | 18.58±8.52 | <0.05 | ||
Average minor axis (mm) | 7.15±2.00 | 8.61±1.70 | <0.05 | 7.31±2.21 | 8.53±1.91 | <0.05 | 8.67±3.38 | 14.18±5.95 | <0.05 |
Data are shown as number or mean ± standard deviation. MIA, minimally invasive adenocarcinoma; AIS, adenocarcinoma in situ; IAC, invasive adenocarcinoma; pGGN, pure ground-glass nodule; mGGN, mixed ground-glass nodule.
Feature selection
All radiomic signatures were normalized. A total of 127 features with variance close to 0 were deleted. Then, Spearman correlation analysis was used to delete the features with the absolute value of correlation greater than 0.9, which resulted in 237 remaining features. The mRMR method was used to sort the remaining features and select the top 100. After that, LASSO regression analysis with a cross-validation method was conducted on the training cohort with the 100 remaining features to find the optimal feature subset (Figure 2). Finally, the optimal 10 features were selected.
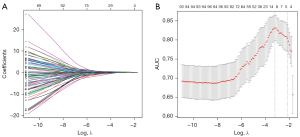
Nomogram construction, validation, and performance
The features related to IAC prediction were extracted from the training set by multivariate logistic regression analysis. The predictors selected were pleural attachment, margin, major axis length, and wavelet.LLH_firstorder_Maximum, original_firstorder_90Percentile, original_firstorder_10Percentile, lbp.3D.k_glcm_Imc2, original_glcm_JointEntropy, wavelet.LLL_glszm_LargeAreaHighGrayLevelEmphasis, lbp.3D.k_glrlm_LongRunEmphasis.
The predictive multivariate logistic regression equation is shown below.
The nomogram (Figure 3) incorporating selected features based on multivariate logistic regression analysis was constructed in the training cohort and confirmed in the testing cohort and the validation cohort. The model performed well in the 3 cohorts (Figure 4). The AUC of the training cohort was 0.877 (95% CI: 0.829–0.925) with 77.8% specificity and 86.4% sensitivity, as shown in Figure 4A. In the testing cohort, the value of the AUC was 0.864 (95% CI: 0.789–0.940) with 80.4% specificity and 81.2% sensitivity, as shown in Figure 4B. In the validation cohort, the AUC value was 0.836 (95% CI: 0.749–0.924) with 78.6% specificity and 86.0% sensitivity, as shown in Figure 4C. Compared with the training cohort, the predictive ability of the model in the testing cohort and validation cohort was not significantly reduced. The calibration curve (Figure 5) of the nomogram showed that the nomogram demonstrated good calibration ability for the training cohort and also showed similar ability in the testing cohort and validation cohort. Meanwhile, the decision curve (Figure 6) analysis in the 3 cohorts proved that the overall net benefit of the nomogram was high compared with the “treat-none” and “treat-all” strategies, and the nomogram model’s prediction showed greater benefit with good clinical application value.
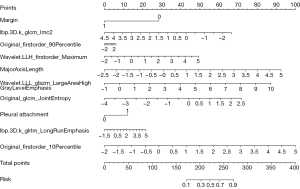
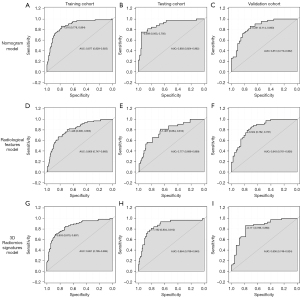
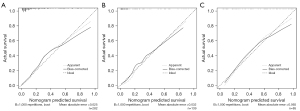
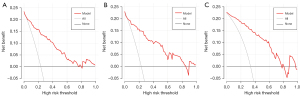
Combining the calibration curve, the ROC curve, and the decision curve of the model’s performance indicated that the nomogram performed well in the 3 cohorts and had good and stable application value in predicting IAC.
Comparison with 3D radiomic signatures and radiological features
The 3 cohorts were also analyzed using multiple logistic regression based on the 3D radiomic signatures subset and the radiological features subset. In the training cohort, the AUC of the 3D radiomic signatures subset model was 0.851 (95% CI: 0.795–0.906) with 87.0% specificity and 69.7% sensitivity, as shown in Figure 4G. In the testing cohort, the evaluation values were AUC 0.893 (95% CI: 0.824–0.962), 95.3% specificity, and 75.0% sensitivity, as shown in Figure 4H. In the validation cohort, the evaluation values were AUC 0.836 (95% CI: 0.749–0.924), 86.0% specificity, and 78.6% sensitivity, as shown in Figure 4I. In the subset based on radiological features, the values were as follows: AUC 0.806 (95% CI: 0.747–0.865), 69.0% specificity, and 78.6% sensitivity; AUC 0.777 (95% CI: 0.686–0.869), 66.4% specificity, and 81.2% sensitivity; and AUC 0.843 (95% CI: 0.761–0.926), 76.2% specificity, and 79.1% sensitivity (Figure 4D-4F).
Discussion
A nomogram incorporating 3D radiomic signatures and radiological features was developed and validated in our study. This nomogram can help to accurately describe and classify non-IAC and IAC, thus helping to improve clinical decisions. In addition, the process designed in this study (beginning with the semiautomatic software segmentation of lesions, having these checked and confirmed by radiologists, using the PyRadiomics package to calculate features, and finally, using the nomogram to make an evaluation) has strong reusability and can play a better auxiliary role in the diagnostic process of radiologists. Because of the high risk of recurrence, patients with IAC usually choose lobectomy for treatment. For MIA/AIS, because of its good prognosis, the treatment is usually regular monitoring or limited resection. Therefore, the accurate distinction between IAC and MIA/AIS before surgery can help clinicians effectively develop a prognosis and thus program appropriate treatment plans.
There were three radiological features and seven 3D radiomic signatures used in the nomogram model. The margin (whether the interface of the tumor-lung is clean or blurred) was an important factor in identifying IAC. No significant difference in boundary clearness between IAC and MIA/AIS was found in this study (relatively more common in IAC), which was similar to the findings of previous studies (29,30). The study showed that pleural attachment was more common in IAC than in MIA/AIS, and it was also one of the important factors in the model. In addition, in clinical diagnosis, attachment of the lesion to the pleura is an important basis for differentiating benign from malignant lesions. The long and short axis of nodules has always been considered one of the important indexes for identifying the subtypes of lung adenocarcinoma, and our model also identified the long axis as an important factor. The bubble lucency sign was found to be one of the important criteria for identifying IAC in some studies (31,32), but it was not used in the model of this study. Other studies have established models to predict nodule invasiveness through radiomics and average CT values (24), which were also not used in this study. In a subsequent study, we should increase the sample size according to different signs in order to determine the relationship between radiological features and the invasiveness of lung adenocarcinoma. We also found that the high-dimensional features of multiple radiological features could be integrated to further reduce the workload of identification by radiologists. In addition, we compared the results with other studies (32-34), and the results showed that the evaluation effect of our nomogram was more accurate and robust in the verification set (Table S2).
The “original_firstorder_90Percentile” and “original_firstorder_10Percentile” mean the 90th and 10th percentile of the lesion CT value, respectively. The “original_glcm_JointEntropy” is a measure of the randomness/variability in neighborhood intensity values in the GLCM features. The “wavelet.LLH_firstorder_Maximum” is the feature of the CT maximum value of the lesion area after the wavelet transform. All these features not only reflect the CT value distribution within the lesion area but also further analyze the trend of CT value changes. This probably explains why the average CT value is not used as a factor in the model. The “wavelet.LLL_glszm_LargeAreaHighGrayLevelEmphasis” is the proportion in the image of the joint distribution of larger size zones with higher gray-level values in the gray-level size zone (GLSZM) quantifies gray level zones with wavelet transform. This feature shows the proportion of connected areas with higher CT values within the range of the lesion, and also reflects the size of the suspected solid area. The “lbp.3D.k_glrlm_LongRunEmphasis” and “lbp.3D.k_glcm_Imc2” indicate the changing state of the texture and also quantify the complexity of the texture in the 3D environment. These features describe the changes in CT value and texture of the lesion area, respectively, which also explains why the bubble lucency sign, the vessel change sign, and other features are not used by the model. This also makes the model trained on the 3D radiomic signatures subset more accurate than the radiological features subset. It can be seen that high-dimensional radiomic signatures provide a more comprehensive description of the changes in the characteristics of the lesion area and can replace some traditional radiological features.
This study had some limitations. First, all samples were collected based on actual clinical conditions, and there was no balancing of the samples from the IAC group and the MIA/AIS group. Therefore, the nomogram model might have been affected by the proportion of sample subsets. In subsequent research, we will expand the sample size and sample distribution to verify the performance of the model. Second, the 3D radiomic signatures under study were calculated using only 1 publicly available software package, so there may be a certain degree of inclination. In future research, we will look for more software packages to calculate high-dimensional image features and aim to develop features that contain higher levels of information. Third, the CT images used in this study were scanned by 4 different types of CT equipment, which might also have had an impact on the performance of the model.
Conclusions
In summary, this study proposes a nomogram combining 3D image features and radiological features to detect the risk of IAC in patients. The results confirm that the nomogram can assist clinicians in the diagnosis and optimization of treatment and, thus, has clinical application value.
Acknowledgments
Funding: The study was supported by Chinese Stomatological Association, Clinical Research Fund for Youth (No. CSA-A2021-03).
Footnote
Reporting Checklist: The authors have completed the TRIPOD reporting checklist. Available at https://qims.amegroups.com/article/view/10.21037/qims-22-491/rc
Conflicts of Interest: All authors have completed the ICMJE uniform disclosure form (available at https://qims.amegroups.com/article/view/10.21037/qims-22-491/coif). The authors have no conflicts of interest to declare.
Ethical Statement: The authors are accountable for all aspects of the work in ensuring that questions related to the accuracy or integrity of any part of the work are appropriately investigated and resolved. The study was conducted in accordance with the Declaration of Helsinki (as revised in 2013). The study was approved by the ethics board of Shanghai University of Medicine & Health Sciences, and the patients’ written informed consent was waived by the ethics committee because it was a retrospective experiment and did not involve patients’ privacy.
Open Access Statement: This is an Open Access article distributed in accordance with the Creative Commons Attribution-NonCommercial-NoDerivs 4.0 International License (CC BY-NC-ND 4.0), which permits the non-commercial replication and distribution of the article with the strict proviso that no changes or edits are made and the original work is properly cited (including links to both the formal publication through the relevant DOI and the license). See: https://creativecommons.org/licenses/by-nc-nd/4.0/.
References
- Kuhn E, Morbini P, Cancellieri A, Damiani S, Cavazza A, Comin CE. Adenocarcinoma classification: patterns and prognosis. Pathologica 2018;110:5-11. [PubMed]
- Casal-Mouriño A, Valdés L, Barros-Dios JM, Ruano-Ravina A. Lung cancer survival among never smokers. Cancer Lett 2019;451:142-9. [Crossref] [PubMed]
- Travis WD, Brambilla E, Nicholson AG, Yatabe Y, Austin JHM, Beasley MB, Chirieac LR, Dacic S, Duhig E, Flieder DB, Geisinger K, Hirsch FR, Ishikawa Y, Kerr KM, Noguchi M, Pelosi G, Powell CA, Tsao MS, Wistuba I, Panel WHO. The 2015 World Health Organization Classification of Lung Tumors: Impact of Genetic, Clinical and Radiologic Advances Since the 2004 Classification. J Thorac Oncol 2015;10:1243-60. [Crossref] [PubMed]
- Wen Z, Zhao Y, Fu F, Hu H, Sun Y, Zhang Y, Chen H. Comparison of outcomes following segmentectomy or lobectomy for patients with clinical N0 invasive lung adenocarcinoma of 2 cm or less in diameter. J Cancer Res Clin Oncol 2020;146:1603-13. [Crossref] [PubMed]
- Liu S, Wang R, Zhang Y, Li Y, Cheng C, Pan Y, Xiang J, Zhang Y, Chen H, Sun Y. Precise Diagnosis of Intraoperative Frozen Section Is an Effective Method to Guide Resection Strategy for Peripheral Small-Sized Lung Adenocarcinoma. J Clin Oncol 2016;34:307-13. [Crossref] [PubMed]
- Behera M, Owonikoko TK, Gal AA, Steuer CE, Kim S, Pillai RN, Khuri FR, Ramalingam SS, Sica GL. Lung Adenocarcinoma Staging Using the 2011 IASLC/ATS/ERS Classification: A Pooled Analysis of Adenocarcinoma In Situ and Minimally Invasive Adenocarcinoma. Clin Lung Cancer 2016;17:e57-64. [Crossref] [PubMed]
- He P, Yao G, Guan Y, Lin Y, He J. Diagnosis of lung adenocarcinoma in situ and minimally invasive adenocarcinoma from intraoperative frozen sections: an analysis of 136 cases. J Clin Pathol 2016;69:1076-80. [Crossref] [PubMed]
- Yang W, Sun Y, Fang W, Qian F, Ye J, Chen Q, Jiang Y, Yu K, Han B. High-resolution Computed Tomography Features Distinguishing Benign and Malignant Lesions Manifesting as Persistent Solitary Subsolid Nodules. Clin Lung Cancer 2018;19:e75-83. [Crossref] [PubMed]
- Liang J, Xu XQ, Xu H, Yuan M, Zhang W, Shi ZF, Yu TF. Using the CT features to differentiate invasive pulmonary adenocarcinoma from pre-invasive lesion appearing as pure or mixed ground-glass nodules. Br J Radiol 2015;88:20140811. [Crossref] [PubMed]
- Jiang B, Takashima S, Miyake C, Hakucho T, Takahashi Y, Morimoto D, Numasaki H, Nakanishi K, Tomita Y, Higashiyama M. Thin-section CT findings in peripheral lung cancer of 3 cm or smaller: are there any characteristic features for predicting tumor histology or do they depend only on tumor size? Acta Radiol 2014;55:302-8. [Crossref] [PubMed]
- Lambin P, Rios-Velazquez E, Leijenaar R, Carvalho S, van Stiphout RG, Granton P, Zegers CM, Gillies R, Boellard R, Dekker A, Aerts HJ. Radiomics: extracting more information from medical images using advanced feature analysis. Eur J Cancer 2012;48:441-6. [Crossref] [PubMed]
- Gillies RJ, Kinahan PE, Hricak H. Radiomics: Images Are More than Pictures, They Are Data. Radiology 2016;278:563-77. [Crossref] [PubMed]
- Xue C, Yuan J, Lo GG, Chang ATY, Poon DMC, Wong OL, Zhou Y, Chu WCW. Radiomics feature reliability assessed by intraclass correlation coefficient: a systematic review. Quant Imaging Med Surg 2021;11:4431-60. [Crossref] [PubMed]
- Zhang YP, Heuvelmans MA, Zhang H, Oudkerk M, Zhang GX, Xie XQ. Changes in quantitative CT image features of ground-glass nodules in differentiating invasive pulmonary adenocarcinoma from benign and in situ lesions: histopathological comparisons. Clin Radiol 2018;73:504.e9-504.e16. [Crossref] [PubMed]
- Shen H, Chen L, Liu K, Zhao K, Li J, Yu L, Ye H, Zhu W. A subregion-based positron emission tomography/computed tomography (PET/CT) radiomics model for the classification of non-small cell lung cancer histopathological subtypes. Quant Imaging Med Surg 2021;11:2918-32. [Crossref] [PubMed]
- Lim HJ, Ahn S, Lee KS, Han J, Shim YM, Woo S, Kim JH, Yie M, Lee HY, Yi CA. Persistent pure ground-glass opacity lung nodules ≥ 10 mm in diameter at CT scan: histopathologic comparisons and prognostic implications. Chest 2013;144:1291-9. [Crossref] [PubMed]
- Zhu E, Xie H, Dai C, Zhang L, Huang Y, Dong Z, Guo J, Su H, Ren Y, Shi P, Fu R, Qin S, Wu C, Chen C. Intraoperatively measured tumor size and frozen section results should be considered jointly to predict the final pathology for lung adenocarcinoma. Mod Pathol 2018;31:1391-9. [Crossref] [PubMed]
- Hawkins S, Wang H, Liu Y, Garcia A, Stringfield O, Krewer H, Li Q, Cherezov D, Gatenby RA, Balagurunathan Y, Goldgof D, Schabath MB, Hall L, Gillies RJ. Predicting Malignant Nodules from Screening CT Scans. J Thorac Oncol 2016;11:2120-8. [Crossref] [PubMed]
- Coroller TP, Agrawal V, Huynh E, Narayan V, Lee SW, Mak RH, Aerts HJWL. Radiomic-Based Pathological Response Prediction from Primary Tumors and Lymph Nodes in NSCLC. J Thorac Oncol 2017;12:467-76. [Crossref] [PubMed]
- Parmar C, Leijenaar RT, Grossmann P, Rios Velazquez E, Bussink J, Rietveld D, Rietbergen MM, Haibe-Kains B, Lambin P, Aerts HJ. Radiomic feature clusters and prognostic signatures specific for Lung and Head & Neck cancer. Sci Rep 2015;5:11044. [Crossref] [PubMed]
- Ishida H, Shimizu Y, Sakaguchi H, Nitanda H, Kaneko K, Yamazaki N, Yanagihara A, Taguchi R, Sakai F, Yasuda M, Kobayashi K. Distinctive clinicopathological features of adenocarcinoma in situ and minimally invasive adenocarcinoma of the lung: A retrospective study. Lung Cancer 2019;129:16-21. [Crossref] [PubMed]
- Le VH, Kha QH, Hung TNK, Le NQK. Risk Score Generated from CT-Based Radiomics Signatures for Overall Survival Prediction in Non-Small Cell Lung Cancer. Cancers (Basel) 2021;13:3616. [Crossref] [PubMed]
- Fan L, Fang M, Tu W, Zhang D, Wang Y, Zhou X, Xia Y, Li Z, Liu S. Radiomics Signature: A Biomarker for the Preoperative Distant Metastatic Prediction of Stage I Nonsmall Cell Lung Cancer. Acad Radiol 2019;26:1253-61. [Crossref] [PubMed]
- She Y, Zhang L, Zhu H, Dai C, Xie D, Xie H, Zhang W, Zhao L, Zou L, Fei K, Sun X, Chen C. The predictive value of CT-based radiomics in differentiating indolent from invasive lung adenocarcinoma in patients with pulmonary nodules. Eur Radiol 2018;28:5121-8. [Crossref] [PubMed]
- Yotsukura M, Asamura H, Motoi N, Kashima J, Yoshida Y, Nakagawa K, Shiraishi K, Kohno T, Yatabe Y, Watanabe SI. Long-Term Prognosis of Patients With Resected Adenocarcinoma In Situ and Minimally Invasive Adenocarcinoma of the Lung. J Thorac Oncol 2021;16:1312-20. [Crossref] [PubMed]
- Le NQK, Kha QH, Nguyen VH, Chen YC, Cheng SJ, Chen CY. Machine Learning-Based Radiomics Signatures for EGFR and KRAS Mutations Prediction in Non-Small-Cell Lung Cancer. Int J Mol Sci 2021;22:9254. [Crossref] [PubMed]
- Ren H, Zhou L, Liu G, Peng X, Shi W, Xu H, Shan F, Liu L. An unsupervised semi-automated pulmonary nodule segmentation method based on enhanced region growing. Quant Imaging Med Surg 2020;10:233-42. [Crossref] [PubMed]
- van Griethuysen JJM, Fedorov A, Parmar C, Hosny A, Aucoin N, Narayan V, Beets-Tan RGH, Fillion-Robin JC, Pieper S, Aerts HJWL. Computational Radiomics System to Decode the Radiographic Phenotype. Cancer Res 2017;77:e104-7. [Crossref] [PubMed]
- Wu F, Tian SP, Jin X, Jing R, Yang YQ, Jin M, Zhao SH. CT and histopathologic characteristics of lung adenocarcinoma with pure ground-glass nodules 10 mm or less in diameter. Eur Radiol 2017;27:4037-43. [Crossref] [PubMed]
- Jin X, Zhao SH, Gao J, Wang DJ, Wu J, Wu CC, Chang RP, Ju HY. CT characteristics and pathological implications of early stage (T1N0M0) lung adenocarcinoma with pure ground-glass opacity. Eur Radiol 2015;25:2532-40. [Crossref] [PubMed]
- Zhou QJ, Zheng ZC, Zhu YQ, Lu PJ, Huang J, Ye JD, Zhang J, Lu S, Luo QQ. Tumor invasiveness defined by IASLC/ATS/ERS classification of ground-glass nodules can be predicted by quantitative CT parameters. J Thorac Dis 2017;9:1190-200. [Crossref] [PubMed]
- Shi L, Shi W, Peng X, Zhan Y, Zhou L, Wang Y, Feng M, Zhao J, Shan F, Liu L. Development and Validation a Nomogram Incorporating CT Radiomics Signatures and Radiological Features for Differentiating Invasive Adenocarcinoma From Adenocarcinoma In Situ and Minimally Invasive Adenocarcinoma Presenting as Ground-Glass Nodules Measuring 5-10mm in Diameter. Front Oncol 2021;11:618677. [Crossref] [PubMed]
- Das SK, Fang KW, Xu L, Li B, Zhang X, Yang HF. Integrative nomogram of intratumoral, peritumoral, and lymph node radiomic features for prediction of lymph node metastasis in cT1N0M0 lung adenocarcinomas. Sci Rep 2021;11:10829. [Crossref] [PubMed]
- Chen X, Feng B, Chen Y, Liu K, Li K, Duan X, Hao Y, Cui E, Liu Z, Zhang C, Long W, Liu X. A CT-based radiomics nomogram for prediction of lung adenocarcinomas and granulomatous lesions in patient with solitary sub-centimeter solid nodules. Cancer Imaging 2020;20:45. [Crossref] [PubMed]