Association of deep medullary veins with the neuroimaging burden of cerebral small vessel disease
Introduction
Cerebral small vessel disease (CSVD) comprises a combination of pathological, clinical, and neuroimaging processes, which involve the small arteries, arterioles, venules, and capillaries of the brain (1). CSVD is responsible for 20% to 25% of stroke cases and 45% of dementia cases, especially in older adults (2). Age, in addition to conventional vascular factors, such as hypertension (HTN), diabetes, and hypercholesterolemia, have been reported as the primary triggers of CSVD (3), and the general consensus is that CSVD is mainly caused by arteriolosclerosis. However, the exact pathophysiological mechanisms of CSVD still remain unclear.
There have been recent discoveries of possible multifactorial pathophysiological changes of CSVD, including reduced cerebral blood flow (4), increased blood-brain barrier permeability (5), microglia activation (6), and other pathological processes such as vascular degeneration or inflammation. Previous studies have mostly focused on the role of small arteries in CSVD, while more recently, research on venules has become increasingly popular (7-12). Deep medullary veins (DMVs), as a type of cerebral small veins, are located adjacent to the lateral ventricle and support venous drainage of the surrounding white matter (13,14). Venous collagenosis, which refers to the process of increased thickness of the walls that results in a narrowed lumen due to collagen deposition, is also classified as one of the subtypes of CSVD (1).
The diagnosis of CSVD is highly dependent on brain magnetic resonance imaging (MRI) markers, including recent small subcortical infarct, white matter hyperintensity (WMH), lacune, prominent perivascular space (PVS), cerebral microbleed (CMB), and brain atrophy (15), and the total burden of neuroimaging markers has been introduced as a tool to evaluate the severity of CSVD (16). Despite this, few studies have concentrated on the imaging manifestation of the venous system, which can be clearly visualized on susceptibility-weighted imaging (SWI). Morphological abnormalities of DMVs on SWI have attracted attention in relation to different neurological disorders, such as cerebral venous thrombosis (14), multiple sclerosis (17), and ischemic stroke (18).
Previous studies on the relationship between CSVD and DMV have either focused on a single neuroimaging marker of CSVD or have used a single parameter for the assessment of DMV, indicating the significant role of DMV in CSVD (7-13). On the basis of this previous research, we hypothesized that a higher neuroimaging burden of CSVD is accompanied by more severe disruption and a smaller number of DMVs. Here, we evaluated the visibility and quantity of DMV on 3-T SWI in patients with CSVD, with the aim to determine the association between the visibility and number of DMVs and the comprehensive neuroimaging burden of CSVD. We present the following article in accordance with the STROBE reporting checklist (available at https://qims.amegroups.com/article/view/10.21037/qims-22-264/rc).
Methods
Study design and participants
This study employed a cross-sectional, retrospective, single-institution design. The study was conducted in accordance with the Declaration of Helsinki (as revised in 2013) and approved by the Institutional Review Board of Huashan Hospital (No. KY2020-731), with individual consent waived due to the retrospective nature of the study.
Clinical and imaging data of 1002 patients suspected of cerebrovascular diseases were collected on admission to the Neurology Department of Huashan Hospital between January 2016 and May 2020. Patients mostly complained of gait disturbance with limb weakness, dizziness, memory decline, and headache. Inclusion criteria were as follows: (I) age >40 years; (II) neuroimages meeting the Standards for Reporting Vascular Changes on Neuroimaging (STRIVE) (15), including WMH with Fazekas score 1–3 in periventricular or deep white matter, or at least 1 lacune or 1 CMB on MRI; (III) complete imaging data, including T1-weighted imaging (T1WI), T2-weighted imaging (T2WI), T2 fluid-attenuated inversion recovery (T2-FLAIR), diffusion-weighted imaging (DWI), and SWI; and (IV) complete clinical data, including vascular risk factors. Exclusion criteria were the following: (I) presence of lesions that could have influenced the assessment of DMV, including tumor, infection, trauma, and acute infarction; (II) severe stenosis or occlusion of large cerebral arteries; and (III) hereditary CSVD. The process of enrollment of patients is shown in Figure 1.
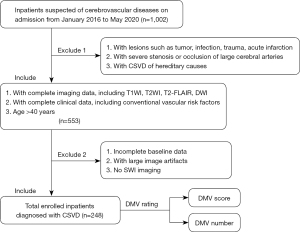
Clinical data
We collected clinical data of the enrolled patients upon admission, including gender, age, body mass index (BMI), diabetes mellitus (DM), hypertension (HTN), current smoking, alcohol use, coronary heart disease (CHD), systolic and diastolic blood pressure (SBP and DBP, respectively), glucose, hemoglobin A1c (HbA1c), total cholesterol (TC), triglyceride (TG), low-density lipoprotein (LDL), high-density lipoprotein (HDL), and homocysteine (Hcy). DM was defined as random blood glucose >11.1 mmol/L or HbA1c >6.5 mmol/L. HTN was defined as SBP ≥140 mmHg or DBP ≥90 mmHg. Current smoking or alcohol drinking was defined as smoking or alcohol intake within the past month.
MRI protocol and parameters
MRI was performed on a GE 3.0-T scanner (Discovery MR750, GE Healthcare, Milwaukee, WI, USA) with a standard 8-channel HRBRAIN coil. The MRI protocol included the following: (I) an axial T1-FLAIR-weighted sequence [repetition time (TR) =1,878 ms, echo time (TE) =23 ms, matrix =288×192, field of view (FOV) =240 mm, slice thickness =6 mm, spacing =2, number of excitations (NEX) =1], (II) an axial T2-PROPELLER–weighted [fast recovery fast spin echo (FRFSE)] sequence (TR =4,526 ms, TE =96 ms, matrix =416×416, FOV =240 mm, slice thickness =6 mm, spacing =2, NEX =1), (III) a T2-FLAIR sequence [TR =8,800 ms, TE = 147 ms, inversion time (TI) =2,100 ms, matrix =256×224, FOV =240 mm, slice thickness =6 mm, spacing =2, NEX =1], (IV) an SWI sequence (TR =76 ms; TE =43 ms; FOV =24 cm; matrix =320×256, slice thickness =3 mm, spacing =1.5, NEX =0.69); and (V) a DWI sequence (TR =3,000 ms; TE =65 ms; matrix =320×256, FOV =240 mm, slice thickness =6 mm, spacing =8, NEX =1).
CSVD assessment
We identified the above-mentioned neuroimaging markers according to STRIVE. The extent of WMH was scored according to the Fazekas scale (periventricular WMH and deep WMH each ranged from 0 to 3), whereby the WMH Fazekas score was the sum of the periventricular WMH and the deep WMH score. PVS was evaluated based on the level of the maximum number of PVSs in the unilateral basal ganglia using 4 levels of severity (19) (0= no PVSs, 1= ≤10 PVSs, 2= 10–20 PVSs, 3= 21–40 PVSs, and 4= ≥40 PVSs). CMB was assessed using both the number of CMBs (20) (degree 0= no CMBs, 1= 1–4 CMBs, 2= 5–9 CMBs, and 3= ≥10 CMBs) and their anatomical location (21) (deep, lobar, and infratentorial CMBs). We also counted the number of lacunes.
The total score of CSVD burden ranged from 0 to 4 (16): a score was tabulated independently for each of high-grade WMH (deep WMH Fazekas 2–3 or periventricular WMH Fazekas 3), moderate-to-severe PVS (degree 2–4), presence of CMB, and presence of lacune.
DMV assessment
We assessed the visibility and quantity of DMVs on 3-T SWI. According to the former semiquantitative visual scoring system (22), DMVs were observed on 5 consecutive periventricular slices (10 mm thick), ranging from the level of the ventricles immediately above the basal ganglia to the level where the ventricles were no longer visible. Similarly, we categorized the distribution of DMVs into 6 anatomical locations: frontal region, parietal region, and occipital region (bilaterally for each region). Each region was given a score ranging from 0 to 3: a score of 0 was given for a continuous and homogenous signal of each DMV, a score of 1 for at least 1 heterogenous but continuous DMV, a score of 2 for at least 1 discontinuous DMV presenting with little homogeneity, and a of score 3 for no visible DMV. Therefore, the total DMV score ranged from 0 to 18 (22). Enrolled patients were divided based on the median DMV score into 2 groups: a low and a high DMV score group. The number of DMVs were also counted on the same levels of both sides. Figure 2 depicts typical images of different DMV scores. Figure 3 shows 2 examples of DMV assessment.
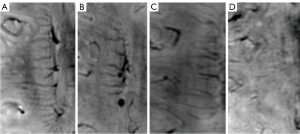
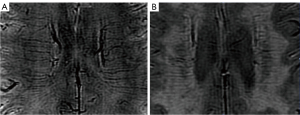
Reliability of the radiological assessments
Two experienced radiologists blinded to the clinical data reviewed all images of each enrolled patient for both the CSVD and DMV assessments. Disagreements were resolved by consensus. The interobserver intraclass correlation coefficients (ICCs) were 0.84 for rating the DMV score, 0.86 for rating the DMV number, and 0.92 for rating the total burden of CSVD.
Statistical analysis
Continuous variables are shown as mean ± standard deviation or median (interquartile range), and categorical variables are described as frequencies and proportions. Differences in numerical variables were analyzed using the Student’s t-test, while differences in categorical variables were analyzed using the chi-square test. Multivariable regression analysis with correction for confounders was used to identify the correlation of DMV number and DMV score with each neuroimaging marker of CSVD. Statistical significance was defined as P<0.05 (two-sided). Data were analyzed using SPSS 23.0 (IBM Corp., Armonk, NY, USA).
Results
A total of 248 patients with CSVD were enrolled, with a mean age of 69.5±14.8 years, and male patients accounted for 73.8% of total patients. High-grade WMH, presence of CMB, moderate-to-severe PVS, and presence of lacune were observed in 118 (47.6%), 193 (77.8%), 96 (38.7%), and 183 (73.8%) patients, respectively. The median DMV score was 8.00.
Univariable analysis of clinical and imaging characteristics of study population
The basic characteristics of the low DMV score group (DMV score ≤8, interquartile range, 2–6) and high DMV score group (DMV score >8, interquartile range, 12–16) are listed in Table 1. Patients with a high DMV score had a significantly higher age (P<0.001) and lower BMI (P=0.007). In addition, the high DMV score group had a higher total CSVD score (P<0.001), more severe deep WMH (P=0.017) and periventricular WMH (P=0.012), more frequent CMBs in different locations (P<0.001 in total, P=0.006 for infratentorial CMB, P=0.024 for deep CMB, P<0.001 for lobar CMB), and a greater number of lacunes (P=0.047). However, there was no significant difference in PVS between the 2 groups.
Table 1
Clinical and imaging characters | DMV score ≤8, n=135 | DMV score >8, n=113 | P |
---|---|---|---|
Vascular risk factors | |||
Age (years) | 66.51±14.97 | 73.12±13.77 | <0.001 |
Male | 96 (71.11%) | 87 (76.99%) | 0.295 |
DM | 40 (29.63%) | 35 (30.97%) | 0.819 |
HTN | 101 (74.81%) | 79 (69.91%) | 0.390 |
Current smoking | 26 (19.26%) | 23 (20.35%) | 0.830 |
Alcohol use | 14 (10.37%) | 12 (10.62%) | 0.949 |
CHD | 19 (14.07%) | 20 (17.70%) | 0.436 |
SBP (mmHg) | 146.84±25.34 | 145.63±24.15 | 0.829 |
DBP (mmHg) | 82.21±13.16 | 79.88±13.87 | 0.200 |
GLU (mmol/L) | 6.52±2.81 | 6.23±2.31 | 0.631 |
HbA1c (%) | 6.53±1.67 | 6.59±1.59 | 0.712 |
TC (mmol/L) | 4.00±0.86 | 3.83±0.94 | 0.146 |
TG (mmol/L) | 1.45±0.83 | 1.46±0.98 | 0.647 |
LDL (mmol/L) | 2.29±0.76 | 2.18±0.76 | 0.319 |
HDL (mmol/L) | 1.08±0.31 | 1.06±0.32 | 0.174 |
BMI (kg/m2) | 25.10±3.23 | 23.81±2.73 | 0.007 |
Hcy (μmol/L) | 13.58±6.90 | 13.32±5.79 | 0.601 |
Neuroimaging markers | |||
Total CSVD score | 2.00 (1.00, 3.00) | 3.00 (2.00, 4.00) | <0.001 |
WMH Fazekas-PV | 2.00 (1.00, 3.00) | 2.00 (1.00, 3.00) | 0.012 |
WMH Fazekas-D | 1.00 (1.00, 2.00) | 2.00 (1.00, 2.00) | 0.017 |
WMH Fazekas | 3.00 (2.00, 5.00) | 4.00 (2.00, 5.00) | 0.011 |
PVS | 1.00 (1.00, 2.00) | 1.00 (1.00, 2.00) | 0.063 |
CMB degree | 1.00 (0.00, 2.00) | 1.00 (1.00, 2.00) | <0.001 |
CMB (infraten) | 0.00 (0.00, 1.00) | 0.00 (0.00, 1.00) | 0.006 |
CMB (deep) | 1.00 (0.00, 2.00) | 1.00 (0.00, 3.00) | 0.024 |
CMB (lobe) | 1.00 (0.00, 2.00) | 1.00 (0.00, 2.00) | <0.001 |
Lacune | 1.00 (0.00, 2.00) | 1.00 (1.00, 2.00) | 0.047 |
Data are presented as mean ± standard deviation, median (interquartile range), or number (frequency) for each variable. DMV, deep medullary vein; DM, diabetes mellitus; HTN, hypertension; CHD, coronary heart disease; SBP and DBP, systolic and diastolic blood pressure; GLU, glucose; HbA1c, hemoglobin A1c; TC, total cholesterol; TG, triglyceride; LDL, low-density lipoprotein; HDL, high-density lipoprotein; BMI, body mass index; Hcy, homocysteine; CSVD, cerebral small vessel disease; WMH, white matter hyperintensity; PVS, perivascular space; CMB, cerebral microbleed.
Multivariable analysis of neuroimaging markers associated with DMV score
The multivariable analysis of the association between neuroimaging markers and DMV score is presented in Table 2. After adjustment for age and BMI, a higher DMV score independently correlated with a higher total CSVD score (β coefficient =1.39; P<0.001), a greater number of CMBs (β coefficient =1.60, P<0.001 in total, β coefficient =0.31, P=0.007 for infratentorial CMB, β coefficient =0.16, P=0.018 for deep CMB), and a greater number of lacunes (P=0.026). However, the severity of deep or periventricular WMH did not exhibit a significant linear association with DMV scores.
Table 2
Neuroimaging markers | B (95% CI) | P |
---|---|---|
Total CSVD score | 1.39 (0.75, 2.23) | <0.001 |
WMH Fazekas-PV | 0.19 (−0.65, 1.04) | 0.651 |
WMH Fazekas-D | 0.57 (−0.21, 1.36) | 0.150 |
WMH Fazekas | 0.21 (−0.21, 0.63) | 0.238 |
PVS | 0.57 (−0.23, 1.37) | 0.163 |
CMB degree | 1.60 (0.86, 2.33) | <0.001 |
CMB (infraten) | 0.31 (0.09, 0.54) | 0.007 |
CMB (deep) | 0.16 (0.03, 0.28) | 0.018 |
CMB (lobe) | 0.04 (−0.01, 0.09) | 0.107 |
Lacune | 0.97 (0.12, 1.82) | 0.026 |
†, adjusted for age and BMI. CSVD, cerebral small vessel disease; WMH, white matter hyperintensity; PVS, perivascular spaces; CMB, cerebral microbleed; BMI, body mass index; CI, confidence interval.
Multivariable analysis of neuroimaging markers associated with DMV number
The multivariable analysis of the association between neuroimaging markers and DMV number is presented in Table 3. Similar to the results obtained for the DMV score, after adjusting for age and BMI, a higher DMV number was independently associated with a higher total CSVD score (β coefficient =–2.55; P=0.001), more frequent total CMBs (β coefficient =−2.27, P=0.009), and a greater number of infratentorial CMBs (β coefficient =–0.51; P=0.048). WMH or presence of lacunes showed no significant linear association with DMV number.
Table 3
Neuroimaging markers | B (95% CI) | P |
---|---|---|
Total CSVD score | −2.55 (−4.00, −1.09) | 0.001 |
WMH Fazekas-PV | 0.17 (−1.72, 2.06) | 0.858 |
WMH Fazekas-D | −0.96 (−2.73, 0.80) | 0.284 |
WMH Fazekas | −0.20 (−1.14, 0.75) | 0.682 |
PVS | −1.24 (−3.03, 0.56) | 0.176 |
CMB degree | −2.27 (−3.98, 0.57) | 0.009 |
CMB (infraten) | −0.51 (−1.02, −0.01) | 0.048 |
CMB (deep) | −0.20 (−0.49, 0.09) | 0.184 |
CMB (lobe) | −0.10 (−0.21, 0.00) | 0.054 |
Lacune | −1.10 (−3.03, 0.83) | 0.263 |
†, adjusted for age and BMI. CSVD, cerebral small vessel disease; WMH, white matter hyperintensity; CMB, cerebral microbleed; PVS, perivascular space; DMV, deep medullary vein; BMI, body mass index; CI, confidence interval.
Discussion
The main finding of our study is that both the DMV score and DMV number independently correlated with the total CSVD score. In other words, reduced homogeneity and continuity as well as a reduced number of DMVs may indicate the severity of CSVD on a whole-brain level, suggesting that DMV may be a novel imaging indicator of CSVD.
There is controversy regarding the radiological changes of DMV in related disorders, and the methods for assessing DMVs show discrepancies. The number of DMVs has been evaluated for several cerebrovascular diseases, and an increased number of DMVs in response to ischemia is known as brush sign on SWI (18). However, there have been studies demonstrating that pathological changes of DMVs are considered to be a dynamic process (22), and both morphological and metabolic changes should be considered when evaluating DMVs. The DMV visual scoring system, which evaluates the continuity and homogeneity of DMV, has been introduced and applied in CSVD over recent years (9-12,22). Meanwhile, the association between DMV number and CSVD markers has also been investigated recently in a population-based cohort (8,23). Given that DMV number and DMV score may indicate different pathological mechanisms (24), we used 2 different methods for the comprehensive evaluation of DMV using 3-T SWI in patients with CSVD.
Our results revealed that the higher DMV score group had more severe periventricular and deep WMHs, which is a finding consistent with those of previous studies (7,9,22). However, neither DMV score nor DMV number was associated with WMHs in multivariable analysis, suggesting possible synchronous changes of DMVs and WMHs with aging. Most studies on DMVs have emphasized the role of WMH (13,25,26). Collagen deposition in DMVs may disrupt the drainage system in the periventricular white matter, lead to increased interstitial fluid, and thus result in vasogenic edema manifesting as WMH on neuroimaging (13,24,26). Nevertheless, a prospective study revealed the increased visibility of DMVs in patients with WMH (25). DMV number was found to have no significant association with WMH in a large community sample (8). As WMH is generally considered to result from ischemic injuries related to small arteries (27), we speculate that DMVs may be a relatively subordinate contributor to WMH. In addition, as the enrolled patients with CSVD had specific clinical symptoms with a relatively high burden of WMH, the insufficient representation of patients with CSVD with a low burden of WMH might have underpowered the analysis. Therefore, the relationship between DMVs and WMH should be verified in further longitudinal radiological and pathological studies.
The severity of CMB was an independent risk factor for both DMV score and DMV number. Furthermore, the number of CMBs in the different distributions showed significant correlations with DMV score and DMV number. Zhang et al. reported that a high DMV score was related to the presence of nonstrict lobar CMBs (10), while our finding extended this to the specific quantification and severity. To date, histopathological research related to CMB has focused on arterioles and small arteries (28), whereas CMBs of venous origin have received little attention, especially for DMVs. Blood-brain barrier failure due to venous collagenosis and leakage of venules has been considered one of the mechanisms of CMB (26,29). A recent study focused on detecting CMBs of venous connections using quantitative susceptibility mapping (QSM) with 7-T MRI (30), which could be applied to explore CMBs of DMV origin.
Prescence of lacunes was independently associated with DMV score, while there was no association with DMV number; this result is consistent with those of previous research (7-9). According to longitudinal observations of DMVs in patients with lacunes, both venular blood-brain barrier disruption resulting from obstruction of the periventricular veins and venous ischemia due to venous outflow obstruction may be possible contributors to the pathogenesis of lacunes (12). Regarding PVS, a recent study demonstrated that presence of PVS in the basal ganglia was related to DMV score (11), which is not consistent with our study. There is evidence for the different distribution and infrequent overlap between venules and PVSs, indicating that most venules and MRI-visible PVSs are not spatially related (31). Comprehensive analysis of PVSs according to anatomical location may be of great value for further investigation of its association with DMV.
Further, we observed a significant difference in age between the low DMV score group and the high DMV score group. After adjusting for age, WMH was no longer associated with DMV score. It is well established that age is a primary risk factor for CSVD, while few studies have focused on veins or venules with regard to age as a risk factor. From the morphological perspective, venous stenosis and occlusion caused by collagen deposition are common pathological processes in older adults (24,26). Higher tortuosity of DMVs in normal aging populations have also been detected by in vivo radiological studies. The remodeling of small veins may be due to the damage of the microvasculature caused by apolipoprotein E4 (32). From the perspective of hemodynamics, impairment of adaptive mechanisms and increases in vascular resistance with aging can result in low blood flow in the brain (4), which disrupts the visibility of DMVs. However, conventional vascular risk factors have not been shown to apply to DMVs, which is likely due to them having different hemodynamics compared with arteries. Interestingly, a higher BMI was found in the lower DMV score group. It is worth noting that most of our enrolled patients were not categorized as obese, and very little research has investigated the influence of obesity on the cerebral venous system. Additionally, the significant difference was based on univariable analysis in our study, which might have been accompanied by possible confounders.
This study had several limitations. First, while intra- and interrater reliability was good, the observation of DMVs was still restricted by a relatively low field strength and direct semiquantitative visual measurement. Three-dimensional venous density maps and an automatic segmentation technique have been applied to visualize venous quantification and distribution in deep white matter (33), which could accurately identify and evaluate the DMVs. Second, this was a cross-sectional study, and hence the longitudinal relationship between DMV and CSVD could not be elucidated. Observing the dynamic changes to DMVs may be useful in determining the pathophysiology of CSVD. Third, the neuroimaging burden of our enrolled patients with CSVD was relatively high, which requires further studies concerning the comprehensive representation of CSVD. Fourth, the correlation between DMV score and DMV number was not investigated, as we aimed to explore the separate associations of these 2 markers with other indicators of the conventional neuroimaging burden of CSVD.
In conclusion, our study focused on the association between DMVs and CSVD from the perspective of neuroimaging. By applying different methods of DMV assessment, we demonstrated that both the DMV score and DMV number are potential imaging indicators of CSVD. Hence, further research concerning DMVs may be of critical importance in evaluating the presence and severity of CSVD and clarifying its potential mechanisms.
Acknowledgments
Funding: This work was supported by the National Natural Science Foundation of China (Nos. 81971598 and 82071456), the Shanghai Academic Research Leader Program (No. 21XD1420900), the “Shuguang Program” supported by the Shanghai Education Development Foundation and Shanghai Municipal Education Commission (No. 19SG06), the Research Startup Fund of Huashan Hospital Fudan University (No. 2021QD035), and the Shanghai Sailing Program (No. 22YF1405000).
Footnote
Reporting Checklist: The authors have completed the STROBE reporting checklist. Available at https://qims.amegroups.com/article/view/10.21037/qims-22-264/rc
Conflicts of Interest: All authors have completed the ICMJE uniform disclosure form (available at https://qims.amegroups.com/article/view/10.21037/qims-22-264/coif). The authors have no conflicts of interest to declare.
Ethical Statement: The authors are accountable for all aspects of the work in ensuring that questions related to the accuracy or integrity of any part of the work are appropriately investigated and resolved. The study was conducted in accordance with the Declaration of Helsinki (as revised in 2013). The study was approved by the Institutional Review Board of Huashan Hospital (No. KY2020-731), and individual consent for this retrospective analysis was waived.
Open Access Statement: This is an Open Access article distributed in accordance with the Creative Commons Attribution-NonCommercial-NoDerivs 4.0 International License (CC BY-NC-ND 4.0), which permits the non-commercial replication and distribution of the article with the strict proviso that no changes or edits are made and the original work is properly cited (including links to both the formal publication through the relevant DOI and the license). See: https://creativecommons.org/licenses/by-nc-nd/4.0/.
References
- Pantoni L. Cerebral small vessel disease: from pathogenesis and clinical characteristics to therapeutic challenges. Lancet Neurol 2010;9:689-701. [Crossref] [PubMed]
- Backhouse EV, Shenkin SD, McIntosh AM, Bastin ME, Whalley HC, Valdez Hernandez M, Muñoz Maniega S, Harris MA, Stolicyn A, Campbell A, Steele D, Waiter GD, Sandu AL, Waymont JMJ, Murray AD, Cox SR, de Rooij SR, Roseboom TJ, Wardlaw JM. Early life predictors of late life cerebral small vessel disease in four prospective cohort studies. Brain 2021;144:3769-78. [Crossref] [PubMed]
- Hamilton OKL, Backhouse EV, Janssen E, Jochems ACC, Maher C, Ritakari TE, Stevenson AJ, Xia L, Deary IJ, Wardlaw JM. Cognitive impairment in sporadic cerebral small vessel disease: A systematic review and meta-analysis. Alzheimers Dement 2021;17:665-85. [Crossref] [PubMed]
- De Silva TM, Faraci FM. Contributions of Aging to Cerebral Small Vessel Disease. Annu Rev Physiol 2020;82:275-95. [Crossref] [PubMed]
- Walsh J, Tozer DJ, Sari H, Hong YT, Drazyk A, Williams G, Shah NJ, O'Brien JT, Aigbirhio FI, Rosenberg G, Fryer TD, Markus HS. Microglial activation and blood-brain barrier permeability in cerebral small vessel disease. Brain 2021;144:1361-71. [Crossref] [PubMed]
- Low A, Mak E, Malpetti M, Passamonti L, Nicastro N, Stefaniak JD, Savulich G, Chouliaras L, Su L, Rowe JB, Markus HS, O'Brien JT. In vivo neuroinflammation and cerebral small vessel disease in mild cognitive impairment and Alzheimer's disease. J Neurol Neurosurg Psychiatry 2020;jnnp-2020-323894. Epub ahead of print. [Crossref] [PubMed]
- Chen X, Wei L, Wang J, Shan Y, Cai W, Men X, Liu S, Kang Z, Lu Z, Mok VCT, Wu A. Decreased visible deep medullary veins is a novel imaging marker for cerebral small vessel disease. Neurol Sci 2020;41:1497-506. [Crossref] [PubMed]
- Ao DH, Zhang DD, Zhai FF, Zhang JT, Han F, Li ML, Ni J, Yao M, Zhang SY, Cui LY, Jin ZY, Zhou LX, Zhu YC. Brain deep medullary veins on 3-T MRI in a population-based cohort. J Cereb Blood Flow Metab 2021;41:561-8. [Crossref] [PubMed]
- Xu Z, Li F, Wang B, Xing D, Pei Y, Yang B, Duan Y. New Insights in Addressing Cerebral Small Vessel Disease: Association With the Deep Medullary Veins. Front Aging Neurosci 2020;12:597799. [Crossref] [PubMed]
- Zhang R, Li Q, Zhou Y, Yan S, Zhang M, Lou M. The relationship between deep medullary veins score and the severity and distribution of intracranial microbleeds. Neuroimage Clin 2019;23:101830. [Crossref] [PubMed]
- Zhang K, Zhou Y, Zhang W, Li Q, Sun J, Lou M. MRI-visible perivascular spaces in basal ganglia but not centrum semiovale or hippocampus were related to deep medullary veins changes. J Cereb Blood Flow Metab 2022;42:136-44. [Crossref] [PubMed]
- Zhou Y, Li Q, Zhang R, Zhang W, Yan S, Xu J, Wang S, Zhang M, Lou M. Role of deep medullary veins in pathogenesis of lacunes: Longitudinal observations from the CIRCLE study. J Cereb Blood Flow Metab 2020;40:1797-805. [Crossref] [PubMed]
- Zhang R, Huang P, Jiaerken Y, Wang S, Hong H, Luo X, Xu X, Yu X, Li K, Zeng Q, Wu X, Lou M, Zhang M. Venous disruption affects white matter integrity through increased interstitial fluid in cerebral small vessel disease. J Cereb Blood Flow Metab 2021;41:157-65. [Crossref] [PubMed]
- Aguiar de Sousa D, Lucas Neto L, Jung S, Penas S, Panos L, Heldner MR, Fischer U, Arnold M, Canhão P, El-Koussy M, Ferro JM, Hakim A. Brush Sign Is Associated With Increased Severity in Cerebral Venous Thrombosis. Stroke 2019;50:1574-7. [Crossref] [PubMed]
- Wardlaw JM, Smith EE, Biessels GJ, Cordonnier C, Fazekas F, Frayne R, et al. Neuroimaging standards for research into small vessel disease and its contribution to ageing and neurodegeneration. Lancet Neurol 2013;12:822-38. [Crossref] [PubMed]
- Huijts M, Duits A, van Oostenbrugge RJ, Kroon AA, de Leeuw PW, Staals J. Accumulation of MRI Markers of Cerebral Small Vessel Disease is Associated with Decreased Cognitive Function. A Study in First-Ever Lacunar Stroke and Hypertensive Patients. Front Aging Neurosci 2013;5:72. [Crossref] [PubMed]
- Taoka T, Fukusumi A, Miyasaka T, Kawai H, Nakane T, Kichikawa K, Naganawa S. Structure of the Medullary Veins of the Cerebral Hemisphere and Related Disorders. Radiographics 2017;37:281-97. [Crossref] [PubMed]
- Mucke J, Möhlenbruch M, Kickingereder P, Kieslich PJ, Bäumer P, Gumbinger C, Purrucker J, Mundiyanapurath S, Schlemmer HP, Bendszus M, Radbruch A. Asymmetry of deep medullary veins on susceptibility weighted MRI in patients with acute MCA stroke is associated with poor outcome. PLoS One 2015;10:e0120801. [Crossref] [PubMed]
- Maclullich AM, Wardlaw JM, Ferguson KJ, Starr JM, Seckl JR, Deary IJ. Enlarged perivascular spaces are associated with cognitive function in healthy elderly men. J Neurol Neurosurg Psychiatry 2004;75:1519-23. [Crossref] [PubMed]
- Yang Q, Yang Y, Li C, Li J, Liu X, Wang A, Zhao J, Wang M, Zeng X, Fan D. Quantitative assessment and correlation analysis of cerebral microbleed distribution and leukoaraiosis in stroke outpatients. Neurol Res 2015;37:403-9. [Crossref] [PubMed]
- Gregoire SM, Chaudhary UJ, Brown MM, Yousry TA, Kallis C, Jäger HR, Werring DJ. The Microbleed Anatomical Rating Scale (MARS): reliability of a tool to map brain microbleeds. Neurology 2009;73:1759-66. [Crossref] [PubMed]
- Zhang R, Zhou Y, Yan S, Zhong G, Liu C, Jiaerken Y, Song R, Yu X, Zhang M, Lou M. A Brain Region-Based Deep Medullary Veins Visual Score on Susceptibility Weighted Imaging. Front Aging Neurosci 2017;9:269. [Crossref] [PubMed]
- Liu ZY, Zhai FF, Ao DH, Han F, Li ML, Zhou L, Ni J, Yao M, Zhang SY, Cui LY, Jin ZY, Zhu YC. Deep medullary veins are associated with widespread brain structural abnormalities. J Cereb Blood Flow Metab 2022;42:997-1006. [Crossref] [PubMed]
- Nan D, Cheng Y, Feng L, Zhao M, Ma D, Feng J. Potential Mechanism of Venous System for Leukoaraiosis: From post-mortem to in vivo Research. Neurodegener Dis 2019;19:101-8. [Crossref] [PubMed]
- Yan S, Wan J, Zhang X, Tong L, Zhao S, Sun J, Lin Y, Shen C, Lou M. Increased visibility of deep medullary veins in leukoaraiosis: a 3-T MRI study. Front Aging Neurosci 2014;6:144. [Crossref] [PubMed]
- Keith J, Gao FQ, Noor R, Kiss A, Balasubramaniam G, Au K, Rogaeva E, Masellis M, Black SE. Collagenosis of the Deep Medullary Veins: An Underrecognized Pathologic Correlate of White Matter Hyperintensities and Periventricular Infarction? J Neuropathol Exp Neurol 2017;76:299-312. [Crossref] [PubMed]
- Han H, Ning Z, Yang D, Yu M, Qiao H, Chen S, Chen Z, Li D, Zhang R, Liu G, Zhao X. Associations between cerebral blood flow and progression of white matter hyperintensity in community-dwelling adults: a longitudinal cohort study. Quant Imaging Med Surg 2022;12:4151-65. [Crossref] [PubMed]
- Liang C, Wang J, Feng M, Zhang N, Guo L. White matter changes, duration of hypertension, and age are associated with cerebral microbleeds in patients with different stages of hypertension. Quant Imaging Med Surg 2022;12:119-30. [Crossref] [PubMed]
- Wardlaw JM, Makin SJ, Valdés Hernández MC, Armitage PA, Heye AK, Chappell FM, Muñoz-Maniega S, Sakka E, Shuler K, Dennis MS, Thrippleton MJ. Blood-brain barrier failure as a core mechanism in cerebral small vessel disease and dementia: evidence from a cohort study. Alzheimers Dement 2017;13:634-43. [Crossref]
- Rotta J, Perosa V, Yakupov R, Kuijf HJ, Schreiber F, Dobisch L, Oltmer J, Assmann A, Speck O, Heinze HJ, Acosta-Cabronero J, Duzel E, Schreiber S. Detection of Cerebral Microbleeds With Venous Connection at 7-Tesla MRI. Neurology 2021;96:e2048-57. [Crossref] [PubMed]
- Jochems ACC, Blair GW, Stringer MS, Thrippleton MJ, Clancy U, Chappell FM, Brown R, Jaime Garcia D, Hamilton OKL, Morgan AG, Marshall I, Hetherington K, Wiseman S, MacGillivray T, Valdés-Hernández MC, Doubal FN, Wardlaw JM. Relationship Between Venules and Perivascular Spaces in Sporadic Small Vessel Diseases. Stroke 2020;51:1503-6. [Crossref] [PubMed]
- Shaaban CE, Aizenstein HJ, Jorgensen DR, MacCloud RL, Meckes NA, Erickson KI, Glynn NW, Mettenburg J, Guralnik J, Newman AB, Ibrahim TS, Laurienti PJ, Vallejo AN, Rosano CLIFE Study Group. In Vivo Imaging of Venous Side Cerebral Small-Vessel Disease in Older Adults: An MRI Method at 7T. AJNR Am J Neuroradiol 2017;38:1923-8. [Crossref] [PubMed]
- Kuijf HJ, Bouvy WH, Zwanenburg JJ, Razoux Schultz TB, Viergever MA, Vincken KL, Biessels GJ. Quantification of deep medullary veins at 7 T brain MRI. Eur Radiol 2016;26:3412-8. [Crossref] [PubMed]