Development and validation of a prediction model of pneumothorax after CT-guided coaxial core needle lung biopsy
Introduction
With a sharp increase in the incidence of chest tumors and the widespread use of computed tomography (CT) of the chest, more pulmonary nodules are detected (1,2). Hence, there is a mounting demand for CT-guided coaxial core needle biopsy (CCNB), which plays an important role in the pathological and cytological evaluation of pulmonary parenchymal lesions (3,4). Although CCNB has been recognized as a relatively safe and less invasive medical diagnostic workup, it is inevitably accompanied by the risk of procedure-related complications (5,6). Pneumothorax is the most common complication of CT-guided CCNB, with a rate of 2.4–60% (7-9). Chest pain, shortness of breath, and hypoxia caused by pneumothorax may increase the need for chest tube placement and prolong hospitalization (10), and pneumothorax may be life-threatening for patients with respiratory insufficiency (5). It is of great significance to establish a predictive model for pneumothorax after CT-guided CCNB.
The factors associated with pneumothorax have been investigated in several previous studies. Emphysema, lesion size, lesion location, patient position, insertion through the interlobar fissures, needle path length from the pleura to the lesion, skin-to-pleura distance, and age have been predictors of pneumothorax in several studies (11-16). Pleural thickening, lobulation signs (16), lesions not in contact with the pleura (12,15), longer procedure duration, repositioning of a coaxial needle with new insertion through the pleura (17), and the use of a 19-G needle are predictors of pneumothorax (9) in other studies.
A number of studies on predictors of pneumothorax after CT-guided CCNB have recruited about 300 to 600 patients in total and have mainly presented univariate analyses. Biopsy methods vary among studies and even within studies, such as coaxial or non-coaxial, the use of core or fine needle biopsy, and the caliber of the biopsy needle. A few studies have further established prediction models for pneumothorax after CT-guided CCNB (12-16), and some of them have applied nomograms to visualize the results; however, the models have not been validated in their studies. One study further assessed the clinical usefulness of a predictive model using decision curve analysis (DCA), but some risk factors, such as emphysema, were not included in this study (14).
This study aimed to develop and validate a risk prediction model for pneumothorax after CT-guided CCNB. The predictive value of the model was also evaluated. We present the following article in accordance with the TRIPOD reporting checklist (available at https://qims.amegroups.com/article/view/10.21037/qims-22-176/rc).
Methods
Study population
This study was conducted in accordance with the Declaration of Helsinki (revised in 2013). The study was approved by the Ethics Committee of the National Cancer Center/Cancer Hospital, Chinese Academy of Medical Sciences and Peking Union Medical College, and the requirement for individual consent for this retrospective analysis was waived. We analyzed consecutive patients who underwent CT-guided CCNB of lung nodules between January 2017 and December 2019. All patients were aged ≥18 years. Patients were excluded from the study if they underwent CCNB of sites not within the lung parenchyma (ribs, scapula, pleural nodule, etc.), could not tolerate CT-guided CCNB for various reasons (uncontrollable cough, sharp rise in intraoperative blood pressure or decrease in blood oxygen saturation that could not be improved, unknown reasons), had a repeat biopsy, or had inadequate documentation of the biopsy in electronic medical records. This study included 1,193 consecutive patients who were randomly allocated to a training set (690 patients), a validation set (253 patients), and an internal test set (250 patients) at a ratio of 6:2:2 (Figure 1).
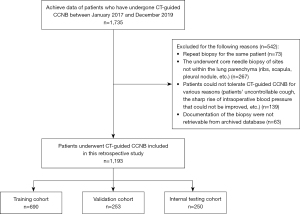
Biopsy technique
Written informed consent for CT-guided CCNB was obtained from all patients during the biopsy procedure. Procedures were performed under CT guidance on a 64-section spiral CT machine (Somatom Definition Edge; Siemens Healthineers, Erlangen, Germany) with a thickness of 5 mm, layer spacing of 5 mm, tube voltage of 120 kV, and automatic tube current. A disposable semi-automatic ejection cutting biopsy needle (NIPRO ELPICK; Plastic Honda Co. Ltd., Tokyo, Japan) with a 17-G coaxial trocar (diameter 1.5 mm, length 10/15 cm) and an 18-G semi-automatic-cut biopsy needle (diameter 1.2 mm, length 15/20 cm) were used for all biopsies.
The position (supine, prone, or lateral) of the biopsy patient was chosen depending on the location of the lesion. An initial non-contrast material-enhanced CT scan with a thickness of 5 mm was routinely performed to determine the location and path of the puncture. A relatively safe needle path was then chosen through the anterior, posterior, or lateral chest wall, depending on the most direct needle path to the lesion, avoiding the rib and lung bullae, if possible. A positioning grid was placed, and a local scan of the lesion was performed at a thickness of 2–5 mm to determine the insertion point on the skin. A subcutaneous injection of 5 mL of 1% lidocaine was used for local anesthesia. According to the predetermined path of puncture, the needle was gradually inserted from the skin, outside the pleura, through the pleura, to inside the lung, until within or around the lesion. Two or more local CT scans were required to determine and adjust the position of the insertion point and direction of the needle. The needle core was pulled out and ejection-cut needle biopsies were performed. Tissue specimens were obtained for pathological and cytological examinations. Three radiologists performed all procedures and were accompanied by the patients’ clinicians.
Patients were advised to avoid coughing postoperatively. Immediate whole-chest CT was performed to observe for pneumothorax, hemorrhage, and other complications, especially the presence of air in the aorta and heart. A chest radiograph was re-examined 2 h later for all patients to determine complications (pneumothorax, hemorrhage, etc.) or whether complications were aggravated (18).
Measured variables
Patient-, lesion-, and procedure-related variables were recorded. The factors included patient’s clinical characteristics, such as age, sex, and history of treatment; factors related with lesion such as size (shorter dimension of the maximum cross section of the lesion on axial lung-window CT images), lobar location (upper or lower lobe of the left lung; upper, middle, or lower lobe of right lung), lung field (fields of the lung were divided into five sections from top to bottom: upper, upper-middle, middle, lower-middle, and lower on the chest radiograph), positional relationship between lesion and pleura (lesion contact with pleura or not), presence of emphysema adjacent to the biopsy track [based on CT diagnostic criteria (19,20) to confirm the presence or absence of emphysema], primary or metastatic disease confirmed by diagnostic results of biopsy and clinical history; date of biopsy (year), patient position (supine, prone or lateral), crossing of fissures, distance from pleura to lesion (along the needle path), angle of needle insertion (the acute angle between the trajectory of the puncture needle and the perpendicular line drawn to the tangent line of the pleura) (Figure 2), number of pleural punctures, number of core samples taken, duration, and performing radiologist. Baseline CT images were reviewed by two radiologists with more than 10 years of experience in chest imaging, and data collection was performed by three radiologists. Pneumothorax was also noted.
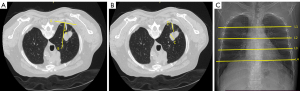
Model development and validation
Univariate logistic regression analysis was used to select the pneumothorax-related factors. Variables with a P value <0.1 on univariate analysis were used as input variables for multivariate logistic regression analysis. We used multivariate logistic regression analysis to select the most useful predictive markers of all the clinical variables identified and constructed a model for predicting pneumothorax in patients undergoing CT-guided CCNB of the lung in the training set. The predictive accuracy of the established model was investigated using receiver operating characteristic (ROC) analysis. To make the outcomes more accessible, we adopted a nomogram as a pictorial representation of the complex mathematical formula. Calibration curves were plotted via bootstrapping with 1,000 resamples to assess the calibration of the prediction model. DCA was used to assess the net benefit of nomogram-assisted decisions at different threshold probabilities compared to the net benefit of decisions made with the assumption that either all patients or none have favorable outcomes.
Patients were classified into high- or low-risk groups according to the model, and the threshold was identified using ROC analysis. Stratified analyses were performed to explore the potential association of the prediction model with pneumothorax using subgroups within clinical risk factors from the entire data set.
Statistical analysis
Categorical variables were compared the χ2 test or Fisher’s exact test. Continuous variables were compared by analysis of variance, the independent-samples t-test, or the Mann–Whitney U test. Statistical analysis was performed using R software (version 4.0.2; R Foundation, Vienna, Austria). A two-sided P value less than 0.1 was considered to indicate statistical significance.
Results
Patient demographics
A total of 1,193 patients, including 702 males (mean age, 61.8 years; age range, 21–88 years) and 491 females (mean age, 59.9 years; age range, 22–85 years), were identified according to the inclusion and exclusion criteria. Pneumothorax occurred in 201 of 690 procedures (29.1%) in the training cohort, 76 of 253 procedures (30.0%) in the validation cohort, and 65 of 250 procedures (26.0%) in the internal testing cohort. Table 1 shows detailed clinical characteristics of the training, validation, and internal test sets. Of the 1,193 patients included in this study, 24 (2.0%) underwent chest tube insertion for a significant amount of pneumothorax (Table S1). This accounted for 7.0% of all patients with pneumothorax.
Table 1
Characteristic | Training cohort (n=690) | Validation cohort (n=253) | Test cohort (n=250) | P value |
---|---|---|---|---|
Age (years)* | 61.26±10.34 | 60.21±9.89 | 61.32±10.52 | 0.496 |
Sex | 0.161 | |||
Male | 422 (61.2) | 140 (55.3) | 140 (56.0) | |
Female | 268 (38.8) | 113 (44.7) | 110 (44.0) | |
History of treatment | 0.112 | |||
Newly diagnosed | 518 (75.1) | 174 (68.8) | 177 (70.8) | |
After treatment | 172 (24.9) | 79 (31.2) | 73 (29.2) | |
Lesion type | 0.660 | |||
Primary | 618 (89.6) | 227 (89.7) | 219 (87.6) | |
Metastatic | 72 (10.4) | 26 (10.3) | 31 (12.4) | |
Lesion size (mm)* | 28.51±16.96 | 27.46±16.37 | 28.09±16.26 | >0.999 |
Lobar location | 0.302 | |||
Left upper | 186 (27.0) | 80 (31.6) | 64 (25.6) | |
Left lower | 139 (20.1) | 36 (14.2) | 46 (18.4) | |
Right upper | 198 (28.7) | 75 (29.6) | 67 (26.8) | |
Right middle | 32 (4.6) | 10 (4.0) | 18 (7.2) | |
Right lower | 135 (19.6) | 52 (20.6) | 55 (22.0) | |
Lung field | 0.404 | |||
Upper | 97 (14.1) | 40 (15.8) | 37 (14.8) | |
Upper-middle | 211 (30.6) | 87 (34.4) | 65 (26.0) | |
Middle | 109 (15.8) | 41 (16.2) | 42 (16.8) | |
Lower-middle | 152 (22.0) | 52 (20.6) | 67 (26.8) | |
Lower | 121 (17.5) | 33 (13.0) | 39 (15.6) | |
Contact with pleura | 0.244 | |||
Yes | 243 (35.2) | 93 (36.8) | 103 (41.2) | |
No | 447 (64.8) | 160 (63.2) | 147 (58.8) | |
Emphysema | 0.079 | |||
Yes | 167 (24.2) | 44 (17.4) | 59 (23.6) | |
No | 523 (75.8) | 209 (82.6) | 191 (76.4) | |
Date of biopsy (year) | 0.092 | |||
2017 | 158 (22.9) | 55 (21.7) | 75 (30.0) | |
2018 | 287 (41.6) | 118 (46.6) | 98 (39.2) | |
2019 | 245 (35.5) | 80 (31.6) | 77 (30.8) | |
Patient position | 0.231 | |||
Supine | 186 (27.0) | 86 (34.0) | 74 (29.6) | |
Prone | 435 (63.0) | 147 (58.1) | 148 (59.2) | |
Lateral | 69 (10.0) | 20 (7.9) | 28 (11.2) | |
Crossing of fissures | 0.201 | |||
Yes | 92 (13.3) | 23 (9.1) | 29 (11.6) | |
No | 598 (87.7) | 230 (90.9) | 221 (88.4) | |
Distance from pleura to lesion (mm)* | 15.64±14.51 | 15.89±14.31 | 14.42±14.88 | 0.771 |
Angle of needle insertion (°)* | 13.79±12.11 | 13.00±11.55 | 13.16±11.65 | >0.999 |
Number of pleural punctures | 0.384 | |||
Single | 687 (99.6) | 253 (100.0) | 249 (99.6) | |
Multiple | 3 (0.4) | 0 | 1 (0.4) | |
Number of core samples taken | 0.578 | |||
Single | 291 (42.2) | 109 (43.1) | 115 (46.0) | |
Multiple | 399 (57.8) | 144 (56.9) | 135 (54.0) | |
Duration (min)* | 17.68±5.63 | 18.04±6.10 | 18.34±6.63 | 0.412 |
Operator | 0.102 | |||
A | 129 (18.7) | 62 (24.5) | 55 (22.0) | |
B | 325 (47.1) | 121 (47.8) | 126 (50.4) | |
C | 236 (34.2) | 70 (27.7) | 69 (27.6) | |
Pneumothorax | 0.556 | |||
Yes | 201 (29.1) | 76 (30.0) | 65 (26.0) | |
No | 489 (70.9) | 177 (70.0) | 185 (74.0) |
*, data are mean ± standard deviation; data in parentheses are percentages. The P values are results of ANOVA for continuous variable and χ2 test for categorized variables. P >0.05 suggests no significant difference between the subjects in the three cohorts.
Univariate and multivariate analysis
Univariate logistic regression analysis was performed to ascertain the effects of various demographic, lesion, and procedural variables on the likelihood of pneumothorax development in the training cohort (Table 2). Sex (P=0.025), date of biopsy (P=0.054), patient position (P<0.001), lung field (P=0.002), lesion contact with the pleura (P=0.002), lesion size (P=0.003), distance from the pleura to the lesion (P<0.001), number of core samples taken (P=0.098), duration (P=0.021), presence of emphysema adjacent to the biopsy track (P=0.005), and crossing fissures (P=0.001) were included in the multivariate logistic regression analysis.
Table 2
Variable | β | SE | z | P | OR | 95% CI | |
---|---|---|---|---|---|---|---|
Lower | Upper | ||||||
Age | −0.007 | 0.008 | 0.894 | 0.371 | 0.993 | 0.977 | 1.009 |
Sex | −0.395 | 0.177 | 2.240 | 0.025* | 0.673 | 0.327 | 1.019 |
History of treatment | −0.196 | 0.198 | 0.988 | 0.323 | 0.822 | 0.434 | 1.211 |
Lesion type | 0.22 | 0.265 | 0.828 | 0.408 | 1.246 | 0.726 | 1.766 |
Lesion size | −0.016 | 0.005 | 2.964 | 0.003* | 0.984 | 0.973 | 0.995 |
Lobar location | 0.024 | 0.059 | 0.406 | 0.685 | 1.024 | 0.909 | 1.14 |
Lung field | 0.194 | 0.063 | 3.066 | 0.002* | 1.214 | 1.09 | 1.338 |
Contact with pleura or not | −0.574 | 0.185 | 3.100 | 0.002* | 0.564 | 0.201 | 0.926 |
Emphysema | 0.525 | 0.188 | 2.792 | 0.005* | 1.69 | 1.322 | 2.058 |
Date of biopsy | −0.214 | 0.111 | 1.924 | 0.054* | 0.808 | 0.59 | 1.025 |
Patient position | 0.547 | 0.148 | 3.703 | <0.001* | 1.728 | 1.438 | 2.017 |
Crossing of fissures | 0.736 | 0.23 | 3.206 | 0.001* | 2.088 | 1.638 | 2.538 |
Distance from pleura to lesion | 0.022 | 0.006 | 3.936 | <0.001* | 1.023 | 1.012 | 1.034 |
Angle of needle insertion | 0.009 | 0.007 | 1.256 | 0.209 | 1.009 | 0.995 | 1.022 |
Number of pleural punctures | −13.683 | 509.652 | 0.032 | 0.979 | <0.001 | −998.918 | 998.918 |
Number of core samples taken | 0.285 | 0.172 | 1.655 | 0.098* | 1.329 | 0.992 | 1.666 |
Duration | 0.033 | 0.014 | 2.316 | 0.021* | 1.034 | 1.006 | 1.062 |
Operator | −0.119 | 0.074 | 1.603 | 0.109 | 0.888 | 0.742 | 1.033 |
*, risk factors included in the multivariate logistic regression analysis. CI, confidence interval; OR, odds ratio; SE, standard error.
Multivariable logistic regression demonstrated that sex (P=0.016), lesion size (P=0.002), lung field (P=0.001), positional relationship with the pleura (P=0.020), presence of emphysema adjacent to the biopsy track (P=0.002), patient position (P=0.002), crossing fissures (P<0.001), and distance from the pleura to the lesion (P=0.077) were independent risk factors for pneumothorax development (Table 3).
Table 3
Variable | β | SE | Z | P | OR | 95% CI | |
---|---|---|---|---|---|---|---|
Lower | Upper | ||||||
Sex | −0.492 | 0.204 | 2.413 | 0.016* | 0.611 | 0.409 | 0.91 |
Lesion size | −0.02 | 0.006 | 3.16 | 0.002* | 0.98 | 0.968 | 0.992 |
Lung field | 0.224 | 0.07 | 3.216 | 0.001* | 1.251 | 1.092 | 1.435 |
Contact with pleura or not | −0.568 | 0.245 | 2.319 | 0.02* | 0.567 | 0.349 | 0.912 |
Emphysema | 0.687 | 0.223 | 3.079 | 0.002* | 1.987 | 1.284 | 3.083 |
Date of biopsy | −0.204 | 0.144 | 1.423 | 0.155 | 0.815 | 0.615 | 1.081 |
Patient position | 0.513 | 0.165 | 3.106 | 0.002* | 1.67 | 1.211 | 2.316 |
Crossing of fissures | 0.932 | 0.268 | 3.484 | <0.001* | 2.54 | 1.503 | 4.301 |
Distance from pleura to lesion | 0.013 | 0.007 | 1.766 | 0.077* | 1.013 | 0.999 | 1.028 |
Number of core samples taken | 0.153 | 0.205 | 0.745 | 0.456 | 1.165 | 0.781 | 1.743 |
Duration | 0.012 | 0.018 | 0.649 | 0.516 | 1.012 | 0.976 | 1.048 |
*, indicates independent risk factors. CI, confidence interval; OR, odds ratio; SE, standard error.
Risk prediction model building and validation
A prediction model including eight variables for pneumothorax in patients undergoing CT-guided CCNB of the lung was built based on the results of multivariable logistic regression analysis in the training set (Table 4). The model indicated a favorable prediction accuracy of pneumothorax with an area under the curve (AUC) of 0.71 [95% confidence interval (CI): 0.67–0.75] in the training cohort, 0.71 (95% CI: 0.64–0.78) in the validation cohort, and 0.68 (95% CI: 0.60–0.75) in the internal test cohort (Figure 3).
Table 4
Variable | β | SE | Z | P | OR | 95% CI | |
---|---|---|---|---|---|---|---|
Lower | Upper | ||||||
Sex | −0.519 | 0.204 | 2.537 | 0.011 | 0.595 | 0.397 | 0.886 |
Lesion size | −0.02 | 0.006 | 3.185 | 0.001 | 0.98 | 0.968 | 0.992 |
Emphysema | 0.591 | 0.219 | 2.700 | 0.007 | 1.806 | 1.176 | 2.776 |
Crossing of fissures | 1.056 | 0.263 | 4.020 | <0.001 | 2.874 | 1.718 | 4.823 |
Distance from pleura to lesion | 0.017 | 0.007 | 2.413 | 0.016 | 1.017 | 1.003 | 1.031 |
Contact with pleura or not | −0.44 | 0.232 | 1.895 | 0.058 | 0.644 | 0.406 | 1.011 |
Lung field | |||||||
Upper-middle | 0.091 | 0.311 | 0.292 | 0.771 | 1.095 | 0.601 | 2.043 |
Middle | 0.477 | 0.341 | 1.399 | 0.162 | 1.611 | 0.831 | 3.175 |
Middle-lower | 0.315 | 0.325 | 0.970 | 0.332 | 1.37 | 0.731 | 2.62 |
Lower | 1.086 | 0.332 | 3.273 | 0.001 | 2.964 | 1.563 | 5.763 |
Patient position | |||||||
Prone | 0.319 | 0.233 | 1.365 | 0.172 | 1.375 | 0.875 | 2.189 |
Lateral | 1.085 | 0.332 | 3.267 | 0.001 | 2.959 | 1.544 | 5.692 |
The total number of cases in the cohort for the multivariable analysis was 690, with 201 pneumothorax. Biopsy position is for the prone or lateral group compared with the supine group; gender is for female compared with male patients; lesion lung field location (upper-middle, middle, middle-lower, lower) is compared with upper lung field. CI, confidence interval; OR, odds ratio; SE, standard error.
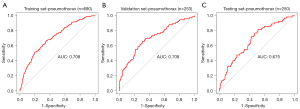
To provide clinicians with a quantitative method to predict a patient’s probability of pneumothorax after CT-guided CCNB of the lung, we constructed a nomogram integrated with eight clinical risk factors (Figure 4A). Each risk variable was listed separately on the nomogram with a corresponding number of points assigned to a given variable magnitude. Then, the cumulative point score for all variables matched the “diagnostic possibility,” which was the pneumothorax probability of the patient. The calibration curve of the prediction model demonstrated that the estimated risks were in agreement with the observed risks of pneumothorax in both the training and validation sets, as well as in the internal test set (Figure 4B-4D).
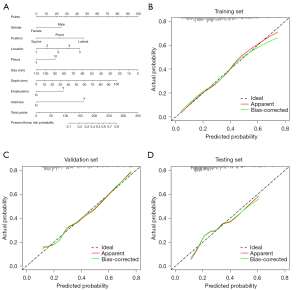
DCA was used to estimate the clinical utility of the prediction model based on threshold probability. Threshold probability is used to derive the net benefit. Based on the decision curves in the validation set (Figure 5A), if a physician’s threshold probability of undergoing CT-guided CCNB of the lung after a comprehensive assessment of the patient’s physical condition is a <25% risk of pneumothorax, nomogram-assisted decisions at these threshold probabilities are irrelevant, as the net benefit is equal to assuming that all or no patients have pneumothorax. In the test set (Figure 5B), the threshold probability was a <25% or >78% risk of pneumothorax. In this figure, the red line corresponding to the prediction model has the highest benefit across a wide range of values of threshold probability both in the validation and test sets. Hence, we can conclude that, except for a small range of threshold probability, intervening with patients based on the prediction model leads to higher benefits than the alternative strategies of treating all patients or treating no patients. In our study, we concluded that using this model to predict the risk of pneumothorax after CT-guided CCNB of the lung would lead to improved clinical outcomes.
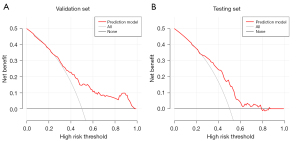
By using ROC analysis to generate the optimum cutoff score, we included patients with a risk score of 0.364 or higher in the group of patients at high risk of pneumothorax (high-risk group) and those with a risk score lower than 0.364 in the group at low risk of pneumothorax (low-risk group). When assessing the distribution of risk values and pneumothorax status, patients with lower risk values generally had a lower probability of pneumothorax than those with higher risk values (Figure 6). When patients were stratified based on clinical factors, a favorable predictive performance of the prediction model was found in all subgroups. The performance of the model for patients within different clinical subgroups is shown in Figure 7.
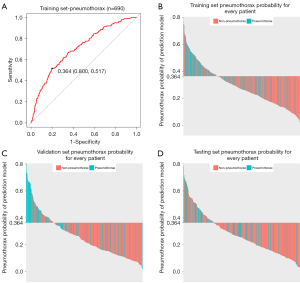
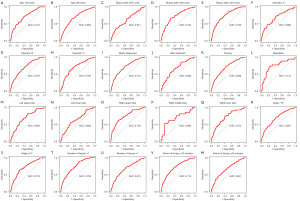
Discussion
In our study, the pneumothorax rate was 28.67%, which approximates the reported incidence. We established a risk prediction model for pneumothorax in patients undergoing CT-guided CCNB, including eight risk factors: sex, patient position, lung field, positional relationship with the pleura, lesion size, distance from the pleura to the lesion, presence of emphysema adjacent to the biopsy tract, and crossing fissures. The model showed good predictive ability in the training cohort (AUC, 0.71), validation cohort (AUC, 0.71), and internal test cohort (AUC, 0.68). A nomogram integrated with the aforementioned predictors provides clinicians with a quantitative method for predicting the probability of pneumothorax.
The presence of emphysema adjacent to the biopsy track is associated with a higher likelihood of associated pneumothorax, which has been widely accepted (16,21-23). This may be related to hyperinflation, hyperexpansion of the thoracic cavity, and destruction of the alveoli in emphysema (11). Reduction in the integrity of the adjacent alveolar interstitial structure and decreased lung elasticity. This reduction in tensile strength may result in poor retraction of the lung after withdrawal of the core needle, and easily leads to the formation of lung bullae. Therefore, patients are more prone to developing pneumothorax.
We also demonstrated that lesion size is a significant risk factor for pneumothorax development. Given that approaching smaller lesions may result in prolonged and more difficult operations (thus increasing the risk of complications), most studies have reached an agreement on small lesion size as a risk factor (16,24-27). However, the threshold lesion size for assessing biopsy risk remains controversial (11,28). In a recent study on complications of CT-guided core needle biopsies, nodules with a diameter <3 cm were reported to have the highest risk of pneumothorax development (11).
No contact between the lesion and pleura and distance from the pleura to the lesion were found to be independent risk factors for pneumothorax in this study. The lesions in contact with the pleura had a depth of 0 cm. The results of the multivariable logistic regression analysis in this study showed that contact with the pleura [P=0.020, odds ratio (OR) =0.567] and distance from the pleura to the lesion (P=0.077, OR =1.013) were independent risk factors for pneumothorax development. In terms of the statistical results, the impact of contact with the pleura was stronger. However, in terms of clinical cognition, value of clinical application, and data recording, calculation, and processing, the distance from the lesion to the pleura has a greater impact. Therefore, both contact with the pleura and the distance from the pleura to the lesion are important in different ways in our study, and they are still included in the prediction model. With regard to lesion depth as a risk factor for pneumothorax, some researchers have also shown that the pneumothorax rate is significantly higher in lesions without contact with the pleura (12,29). It would be reasonable to hypothesize that a longer needle path tends to tear the pleura and normal lung tissue when patients breathe during the procedure, which may cause an increase in the amount of air leakage.
Biopsies in which the needle crossed a fissure had a significant increase in the risk of pneumothorax (79.5% vs. 20.5%, P<0.001; OR =2.702; 95% CI: 1.895–3.853). This may be explained by the multiple pleural punctures, leaving multiple opportunities for air leakage (30-32). The mechanism may also be partly related to shearing at the punctures along a fissure, as each lobe slides somewhat independently from the other with breathing and the needle is fixed within each of them. During a pleural puncture along the chest wall, the needle can move freely with the lung if it is in just one lobe, fixed with the chest wall as its pivot point, which might allow for less pleural tearing than occurs at the interface between the two lobes for a trans-fissural puncture (30).
Lateral positioning during biopsy may increase the risk of developing severe pneumothorax. This has been previously demonstrated by Ruud et al. (17) and may be related to the serious separation of the parietal and visceral pleura in the lateral decubitus position with the biopsied lung up (ante-dependent) than in the supine and prone positions; therefore, air is more likely to enter the pleural cavity as the needle is removed (33). The prone position is thought to be preferable during the biopsy procedure in some studies (34-36) with several advantages. The thickness of the thoracic wall is greater posteriorly than anteriorly, the posterior intercostal spaces are wider than the anterior ones, and the biopsy needle tends to be more stable when inserted because the posterior ribs move less with respiratory motion than when inserted into the anterior ribs (34).
In this study, lesions of the lower lung field were regarded as a risk factor for pneumothorax compared to those of the upper, upper-middle, middle, and middle-lower lung fields, in large part to greater respiratory motion of the lower lung field (5,21). Five lung fields were defined as five equal sections from the top to the bottom on the chest radiograph. The lobe location (25,37) was not shown to be an independent risk factor in univariate analysis; a possible contributor may be collinearity between the two factors.
Sex has not been reported to correlate with the incidence of pneumothorax in previous studies. Female sex was found to be a protective factor in the current study. A possible explanation for this finding is that the prevalence of lung tumors in men is higher than that in women, and more men smoke than women (38), leading to more damage to the lungs in men. Another possible explanation for the protective effect of the female sex is the higher incidence of emphysema in males (female:male =26:244, P<0.001), which is a risk factor for pneumothorax in CT-guided CCNB, as explained earlier. Many factors related to the patient or lesion, such as age and lesion type, could not be changed and were not independent risk factors in this study. Close attention should be paid to the factors influencing the biopsy procedure and to post-biopsy preventive measures (5).
Accurately predicting the probability of pneumothorax after CT-guided CCNB is important. However, only a few predictive models are currently available for this purpose. A risk prediction model was established based on 8 predictors and showed favorable predictive performance in our research, with AUCs of 0.71 and 0.68 in the validation and internal test sets, respectively. This nomogram can provide clinicians with a quantitative method (39) to predict a patient’s probability of pneumothorax after CT-guided CCNB of the lung. This prediction model exhibited good discrimination and calibration. Based on the DCA in the validation and test sets, except for a small range of threshold probability, intervening for patients based on the prediction model leads to higher benefits than the alternative strategies of treating all patients or treating no patients (40). In addition, to justify the clinical usefulness, patients were stratified based on clinical factors, and a favorable predictive performance of the prediction model was found in all subgroups.
This study had some limitations. First, an external validation was not performed. Although strengthened by a large consecutive number of patients and supported by an internal test cohort, this was a single-center retrospective study with an unknown bias. Further verification in multiple centers is needed to confirm the generalization and external validity of the findings. Second, some risk factors, such as pulmonary function, needle size, and patient positioning after biopsy, were not included in this study. Further investigations are warranted to validate the risk factors for pneumothorax. Finally, although the development of a significant amount of pneumothorax requiring chest tube insertion was important, we were unable to establish a prediction model for patients requiring chest tube insertion due to the small number of patients in this retrospective study. In this study, no differences were found in most characteristics between the two groups of patients with pneumothorax requiring chest tube insertion, except for lesion size and the presence of emphysema adjacent to the biopsy tract.
Conclusions
In summary, we proposed a convenient predictive model to facilitate preoperative evaluation of the risk of pneumothorax in patients undergoing CT-guided CCNB.
Acknowledgments
Funding: This work was supported by Beijing Hope Run Special Fund of Cancer Foundation of China (No. LC2021A25).
Footnote
Reporting Checklist: The authors have completed the TRIPOD reporting checklist. Available at https://qims.amegroups.com/article/view/10.21037/qims-22-176/rc
Conflicts of Interest: All authors have completed the ICMJE uniform disclosure form (available at https://qims.amegroups.com/article/view/10.21037/qims-22-176/coif). All authors reported that this work was supported by Beijing Hope Run Special Fund of Cancer Foundation of China (No. LC2021A25). The authors have no other conflicts of interest to declare.
Ethical Statement: The authors are accountable for all aspects of the work in ensuring that questions related to the accuracy or integrity of any part of the work are appropriately investigated and resolved. This study was conducted in accordance with the Declaration of Helsinki (revised in 2013). The study was approved by the Ethics Committee of the National Cancer Center/Cancer Hospital, Chinese Academy of Medical Sciences and Peking Union Medical College, and the requirement for individual consent for this retrospective analysis was waived.
Open Access Statement: This is an Open Access article distributed in accordance with the Creative Commons Attribution-NonCommercial-NoDerivs 4.0 International License (CC BY-NC-ND 4.0), which permits the non-commercial replication and distribution of the article with the strict proviso that no changes or edits are made and the original work is properly cited (including links to both the formal publication through the relevant DOI and the license). See: https://creativecommons.org/licenses/by-nc-nd/4.0/.
References
- Bellolio MF, Heien HC, Sangaralingham LR, Jeffery MM, Campbell RL, Cabrera D, Shah ND, Hess EP. Increased Computed Tomography Utilization in the Emergency Department and Its Association with Hospital Admission. West J Emerg Med 2017;18:835-45. [Crossref] [PubMed]
- Barta JA, Powell CA, Wisnivesky JP. Global Epidemiology of Lung Cancer. Ann Glob Health 2019;85:8. [Crossref] [PubMed]
- Manhire A, Charig M, Clelland C, Gleeson F, Miller R, Moss H, Pointon K, Richardson C, Sawicka E. BTS. Guidelines for radiologically guided lung biopsy. Thorax 2003;58:920-36. [Crossref] [PubMed]
- Winokur RS, Pua BB, Sullivan BW, Madoff DC. Percutaneous lung biopsy: technique, efficacy, and complications. Semin Intervent Radiol 2013;30:121-7. [Crossref] [PubMed]
- Zeng L, Liao H, Ren F, Zhang Y, Wang Q, Xie M. Pneumothorax Induced by Computed Tomography Guided Transthoracic Needle Biopsy: A Review for the Clinician. Int J Gen Med 2021;14:1013-22. [Crossref] [PubMed]
- Fang X, Li J, Sun B, Liu M, Tang Z. Underestimated pulmonary hemorrhage-a fatal complication combined with systemic air embolism after CT-guided lung biopsy: a case description. Quant Imaging Med Surg 2021;11:4661-6. [Crossref] [PubMed]
- Richardson CM, Pointon KS, Manhire AR, Macfarlane JT. Percutaneous lung biopsies: a survey of UK practice based on 5444 biopsies. Br J Radiol 2002;75:731-5. [Crossref] [PubMed]
- Wiener RS, Schwartz LM, Woloshin S, Welch HG. Population-based risk for complications after transthoracic needle lung biopsy of a pulmonary nodule: an analysis of discharge records. Ann Intern Med 2011;155:137-44. [Crossref] [PubMed]
- Kuban JD, Tam AL, Huang SY, Ensor JE, Philip AS, Chen GJ, Ahrar J, Murthy R, Avritscher R, Madoff DC, Mahvash A, Ahrar K, Wallace MJ, Nachiappan AC, Gupta S. The Effect of Needle Gauge on the Risk of Pneumothorax and Chest Tube Placement After Percutaneous Computed Tomographic (CT)-Guided Lung Biopsy. Cardiovasc Intervent Radiol 2015;38:1595-602. [Crossref] [PubMed]
- Brown KT, Brody LA, Getrajdman GI, Napp TE. Outpatient treatment of iatrogenic pneumothorax after needle biopsy. Radiology 1997;205:249-52. [Crossref] [PubMed]
- Ozturk K, Soylu E, Gokalp G, Topal U. Risk factors of pneumothorax and chest tube placement after computed tomography-guided core needle biopsy of lung lesions: a single-centre experience with 822 biopsies. Pol J Radiol 2018;83:e407-14. [Crossref] [PubMed]
- Zhao Y, Wang X, Wang Y, Zhu Z. Logistic regression analysis and a risk prediction model of pneumothorax after CT-guided needle biopsy. J Thorac Dis 2017;9:4750-7. [Crossref] [PubMed]
- Anzidei M, Sacconi B, Fraioli F, Saba L, Lucatelli P, Napoli A, Longo F, Vitolo D, Venuta F, Anile M, Diso D, Bezzi M, Catalano C. Development of a prediction model and risk score for procedure-related complications in patients undergoing percutaneous computed tomography-guided lung biopsy. Eur J Cardiothorac Surg 2015;48:e1-6. [Crossref] [PubMed]
- Wang S, Tu J, Chen W. Development and Validation of a Prediction Pneumothorax Model in CT-Guided Transthoracic Needle Biopsy for Solitary Pulmonary Nodule. Biomed Res Int 2019;2019:7857310. [Crossref] [PubMed]
- Weon J, Robson S, Chan R, Ussher S. Development of a risk prediction model of pneumothorax in percutaneous computed tomography guided transthoracic needle lung biopsy. J Med Imaging Radiat Oncol 2021;65:686-93. [Crossref] [PubMed]
- Yang L, Liang T, Du Y, Guo C, Shang J, Pokharel S, Wang R, Niu G. Nomogram model to predict pneumothorax after computed tomography-guided coaxial core needle lung biopsy. Eur J Radiol 2021;140:109749. [Crossref] [PubMed]
- Ruud EA, Stavem K, Geitung JT, Borthne A, Søyseth V, Ashraf H. Predictors of pneumothorax and chest drainage after percutaneous CT-guided lung biopsy: A prospective study. Eur Radiol 2021;31:4243-52. [Crossref] [PubMed]
- Guo Z, Shi H, Li W, Lin D, Wang C, Liu C, et al. Chinese multidisciplinary expert consensus: Guidelines on percutaneous transthoracic needle biopsy. Thorac Cancer 2018;9:1530-43. [Crossref] [PubMed]
- Lynch DA, Austin JH, Hogg JC, Grenier PA, Kauczor HU, Bankier AA, Barr RG, Colby TV, Galvin JR, Gevenois PA, Coxson HO, Hoffman EA, Newell JD Jr, Pistolesi M, Silverman EK, Crapo JD. CT-Definable Subtypes of Chronic Obstructive Pulmonary Disease: A Statement of the Fleischner Society. Radiology 2015;277:192-205. [Crossref] [PubMed]
- Barr RG, Berkowitz EA, Bigazzi F, Bode F, Bon J, et al. A combined pulmonary-radiology workshop for visual evaluation of COPD: study design, chest CT findings and concordance with quantitative evaluation. COPD 2012;9:151-9. [Crossref] [PubMed]
- Nour-Eldin NE, Alsubhi M, Emam A, Lehnert T, Beeres M, Jacobi V, Gruber-Rouh T, Scholtz JE, Vogl TJ, Naguib NN. Pneumothorax Complicating Coaxial and Non-coaxial CT-Guided Lung Biopsy: Comparative Analysis of Determining Risk Factors and Management of Pneumothorax in a Retrospective Review of 650 Patients. Cardiovasc Intervent Radiol 2016;39:261-70. [Crossref] [PubMed]
- Drumm O, Joyce EA, de Blacam C, Gleeson T, Kavanagh J, McCarthy E, McDermott R, Beddy P. CT-guided Lung Biopsy: Effect of Biopsy-side Down Position on Pneumothorax and Chest Tube Placement. Radiology 2019;292:190-6. [Crossref] [PubMed]
- Hiraki T, Mimura H, Gobara H, Iguchi T, Fujiwara H, Sakurai J, Matsui Y, Inoue D, Toyooka S, Sano Y, Kanazawa S. CT fluoroscopy-guided biopsy of 1,000 pulmonary lesions performed with 20-gauge coaxial cutting needles: diagnostic yield and risk factors for diagnostic failure. Chest 2009;136:1612-7. [Crossref] [PubMed]
- Hajjar WM, Fetyani IM, Alqarni RM, Alajlan FA, Bahgat FF, Alharbi SR. Complications and Risk Factors of Patients Undergoing Computed Tomography-Guided Core Needle Lung Biopsy: A Single-Center Experience. Cureus 2021;13:e16907. [Crossref] [PubMed]
- Huang MD, Weng HH, Hsu SL, Hsu LS, Lin WM, Chen CW, Tsai YH. Accuracy and complications of CT-guided pulmonary core biopsy in small nodules: a single-center experience. Cancer Imaging 2019;19:51. [Crossref] [PubMed]
- Heerink WJ, de Bock GH, de Jonge GJ, Groen HJ, Vliegenthart R, Oudkerk M. Complication rates of CT-guided transthoracic lung biopsy: meta-analysis. Eur Radiol 2017;27:138-48. [Crossref] [PubMed]
- Zhu J, Qu Y, Wang X, Jiang C, Mo J, Xi J, Wen Z. Risk factors associated with pulmonary hemorrhage and hemoptysis following percutaneous CT-guided transthoracic lung core needle biopsy: a retrospective study of 1,090 cases. Quant Imaging Med Surg 2020;10:1008-20. [Crossref] [PubMed]
- Yeow KM, Su IH, Pan KT, Tsay PK, Lui KW, Cheung YC, Chou AS. Risk factors of pneumothorax and bleeding: multivariate analysis of 660 CT-guided coaxial cutting needle lung biopsies. Chest 2004;126:748-54. [Crossref] [PubMed]
- Cox JE, Chiles C, McManus CM, Aquino SL, Choplin RH. Transthoracic needle aspiration biopsy: variables that affect risk of pneumothorax. Radiology 1999;212:165-8. [Crossref] [PubMed]
- Moreland A, Novogrodsky E, Brody L, Durack J, Erinjeri J, Getrajdman G, Solomon S, Yarmohammadi H, Maybody M. Pneumothorax with prolonged chest tube requirement after CT-guided percutaneous lung biopsy: incidence and risk factors. Eur Radiol 2016;26:3483-91. [Crossref] [PubMed]
- Lim CS, Tan LE, Wang JY, Lee CH, Chang HC, Lan CC, Yang MC, Chang-Yao Tsao T, Wu YK. Risk factors of pneumothorax after CT-guided coaxial cutting needle lung biopsy through aerated versus nonaerated lung. J Vasc Interv Radiol 2014;25:1209-17. [Crossref] [PubMed]
- Shiekh Y, Haseeb WA, Feroz I, Shaheen FA, Gojwari TA, Choh NA. Evaluation of various patient-, lesion-, and procedure-related factors on the occurrence of pneumothorax as a complication of CT-guided percutaneous transthoracic needle biopsy. Pol J Radiol 2019;84:e73-9. [Crossref] [PubMed]
- Huo YR, Chan MV, Habib AR, Lui I, Ridley L. Pneumothorax rates in CT-Guided lung biopsies: a comprehensive systematic review and meta-analysis of risk factors. Br J Radiol 2020;93:20190866. [Crossref] [PubMed]
- Nakamura M, Yoshizako T, Koyama S, Kitagaki H. Risk factors influencing chest tube placement among patients with pneumothorax because of CT-guided needle biopsy of the lung. J Med Imaging Radiat Oncol 2011;55:474-8. [Crossref] [PubMed]
- Ko JP, Shepard JO, Drucker EA, Aquino SL, Sharma A, Sabloff B, Halpern E, McLoud TC. Factors influencing pneumothorax rate at lung biopsy: are dwell time and angle of pleural puncture contributing factors? Radiology 2001;218:491-6. [Crossref] [PubMed]
- Miller KS, Fish GB, Stanley JH, Schabel SI. Prediction of pneumothorax rate in percutaneous needle aspiration of the lung. Chest 1988;93:742-5. [Crossref] [PubMed]
- Chami HA, Faraj W, Yehia ZA, Badour SA, Sawan P, Rebeiz K, Safa R, Saade C, Ghandour B, Shamseddine A, Mukherji D, Haydar AA. Predictors of pneumothorax after CT-guided transthoracic needle lung biopsy: the role of quantitative CT. Clin Radiol 2015;70:1382-7. [Crossref] [PubMed]
- GBD 2015 Tobacco Collaborators. Smoking prevalence and attributable disease burden in 195 countries and territories, 1990-2015: a systematic analysis from the Global Burden of Disease Study 2015. Lancet 2017;389:1885-906. [Crossref] [PubMed]
- Balachandran VP, Gonen M, Smith JJ, DeMatteo RP. Nomograms in oncology: more than meets the eye. Lancet Oncol 2015;16:e173-80. [Crossref] [PubMed]
- Vickers AJ, Elkin EB. Decision curve analysis: a novel method for evaluating prediction models. Med Decis Making 2006;26:565-74. [Crossref] [PubMed]