Iodine map histogram metrics in early-stage breast cancer: prediction of axillary lymph node metastasis status
Introduction
Breast cancer has overtaken lung cancer as the leading malignant tumor worldwide, and is now the main cause of cancer-related deaths in women (1). The presence of axillary lymph node (ALN) metastases in primary breast cancer plays an important role in predicting prognosis and can be used to guide local-regional and systemic treatment. Patients with ALN metastases are candidates for neoadjuvant chemotherapy (NAC) (2). At present, ALN status of patients with breast cancer is usually assessed using sentinel lymph node biopsy (SLNB) or fine needle aspiration cytology (FNAC). Although the accuracy of SLNB has been reported as being high for the detection of ALN metastasis (3), it is an invasive procedure, and patients often report symptoms such as pain, numbness, and restricted movement following the procedure (4,5). Ultrasound (US)-guided fine-needle biopsy may be capable of replacing SLNB when planning the breast surgical approach; however, it is also an invasive procedure, and its diagnostic performance is not always satisfactory (6,7). Thus, a noninvasive imaging assessment that provides preoperative information on ALN metastasis would be beneficial in clinical practice.
Although various imaging techniques have been proposed to improve the diagnostic accuracy of positron emission tomography-computed tomography (PET-CT), US, and magnetic resonance imaging (MRI) in the evaluation of ALN metastasis, no definitive criteria have been established, and the diagnostic performance of these imaging techniques is insufficient as an alternative to SLNB (8). Computed tomography (CT) is widely used in clinical practice for the staging of breast cancer due to its high specificity and wide applicability. However, traditional CT mainly provides morphological information, which cannot be used to accurately predict the status of lymph nodes (9). Therefore, it is necessary to develop an imaging method to accurately detect ALN metastasis.
The development of spectral CT is considered a milestone in CT diagnosis. It involves the scanning mode of fast kilovolt switching technology alternating between low- and high-energy data sets (10,11). It has been increasingly used in clinical practice, such as during iodine-based material decomposition imaging. The latter quantifies iodine concentration as an imaging marker for tissue vascularization, thus providing a wide range of additional applications (10). In parallel, histogram analysis is an objective and reproducible method based on tissue volume and provides more extensive information on tumor characteristics, especially when the intensity distribution is not normal (12,13). The changes of histogram shape, asymmetry, and variation reflect underlying molecular processes such as proliferation and aggressiveness (14). Recent research has shown that radiomics signatures obtained from iodine maps of spectral CT can predict cancer spread to other organs or tissues (15). However, the value of histogram metrics from iodine maps of preoperatively evaluated ALN status in early-stage breast cancer remains elusive.
In the current study, we hypothesized that variations in the metastatic potential of ALNs between different breast cancers would be influenced by microscopic changes in tumor perfusion heterogeneity, and that these in turn could be captured by iodine map-based histogram metrics. Therefore, our aim was to investigate the usefulness of histogram metrics from iodine maps in the preoperative diagnosis of metastatic ALNs in patients with early-stage breast cancer. We present the following article in accordance with the Standards for Reporting Diagnostic accuracy studies (STARD) reporting checklist (available at https://qims.amegroups.com/article/view/10.21037/qims-22-253/rc).
Methods
The study was conducted in accordance with the Declaration of Helsinki (as revised in 2013). This retrospective single-center study was approved by the Ethics Committee of Fujian Medical University Union Hospital, and individual consent for this retrospective analysis was waived.
Patient characteristics
Between October 2020 and November 2021, women with suspected breast cancer based on mammography screening or ultrasonography who required spectral CT examination to evaluate potential lung or mediastinal lymph node or bone metastases were enrolled. The inclusion criteria were as follows: women (I) who were diagnosed with invasive ductal carcinoma or invasive lobular carcinoma for the first time; (II) whose T stage was pathologically T2 or less (tumor size less than 5 cm); (III) who had not undergone biopsy of the mass or ALNs before the initial CT examination; (IV) who had no history of chemotherapy or radiation therapy in the chest; and (V) whose lymph node pathological status was clear. The exclusion criteria were as follows: (I) presence of a lesion with nonmass enhancement; (II) multiple lesions; (III) primary tumor not visible on CT imaging; and (IV) negative or no fine-needle aspiration results. Thus, based on these criteria, a total of 113 consecutive patients were included in this study.
CT imaging acquisition and postprocessing
Imaging acquisition was performed using a 256 multidetector row CT scanner (Revolution CT; GE Healthcare, Chicago, IL, USA) in dual-energy mode. Each patient underwent nonenhanced and double-phase contrast-enhanced scanning. The scanning range included the entire chest from the superior aperture of the thorax to the diaphragm, thus covering the entire breast and armpit area. Spectral CT scanning parameters were as follows: voltages between 80 and 140 kVp (rapid switching), tube current of 280 mA, helical pitch of 0.992, reconstructed layer thickness of 1.25 mm, and rotation speed of 0.28 seconds. Patients were intravenously injected with a total dose of 50–60 mL nonionic iodinated contrast agent (iopamidol 400 mgI/mL, Shanghai Bracco Sine Pharmaceutical, Shanghai, China) at a rate of 2–3 mL/s, followed by administration of 30 mL saline solution. When the attenuation value of the descending aortic level reached the trigger threshold [up to 100 Hounsfield unit (HU)], an arterial phase (AP) enhancement scan was performed automatically, and the venous phase (VP) was performed 30 seconds after the AP scans. The following sets of images were transferred to a workstation (AW 4.6; GE Healthcare): a 1.25 mm-thick spectral CT data file containing sets of monochromatic images ranging from 40 to 70 keV, iodine- and water-based material decomposition images, and effective atomic number images.
Quantitative spectral CT parameters of breast masses
Two experienced radiologists (LL and FZ) with 8 years and 15 years of experience in breast and chest radiology, respectively, who were blinded to the correlative clinical data and ALN pathologic status, independently performed the measurements using viewer software on an AW 4.6 workstation. Spectral parameters were measured by placing a region of interest (ROI), which encompassed the entire mass on VPs, including CT values of monochromatic images (40, 50, 60, and 70 keV), the iodine concentration, and the effective atomic number of tumors. The normalized iodine concentration was defined as the iodine concentration of the mass divided by the iodine concentration of the aorta. The slope of the spectral HU curve (λHU, in HU per keV) was calculated as follows: λHU = (HU40 keV − HU70 keV)/30 keV, where HU40 keV represents the CT value measured on 40 keV images, and HU70 keV stands for the CT value measured on 70 keV images. The maximum diameter of the tumor was measured using an image of 40 keV. The location of primary tumors in the breast was also recorded.
Histogram metrics analysis
The iodine map images data were evaluated with the open-source software package FeAture Explorer (FAE; https://github.com/salan668/FAE). The same 2 senior radiologists, who were blinded to axillary area findings on CT, analyzed the histogram metrics of the primary breast tumors. For each lesion, the ROI was semiautomatically delineated using the ITK-SNAP program (version 4.6.1; University of Pennsylvania, Philadelphia, PA, USA; www.itksnap.org), and then manually delineated by an operator and verified by an expert radiologist. The ROIs were drawn on the solid portion of tumors, using 40 keV images as a reference, excluding areas with necrosis, cysts, calcifications, and hemorrhage as much as possible. All imaging slices including tumors were obtained to visualize the whole lesion and were then automatically projected onto an iodine map. Only 40 keV images were used for drawing ROIs, and iodine maps were used for histogram metrics analysis. The final 3D information from all voxels was calculated to generate the corresponding histograms. The following metrics were obtained from each histogram: (I) mean, median, maximum, minimum, and percentiles (10th, 90th); and (II) kurtosis, skewness, energy, range, and variance.
Clinicopathological data
Clinicopathological data, including patient age, menopausal status, family history, estrogen receptor (ER) status, progesterone receptor (PR) status, human epidermal growth factor receptor 2 (HER2) status, Ki-67 index, and lymph node status, were obtained from patients’ electronic medical records. The cutoff point for an ER- and PR-positive expression level was 1% based on the immunohistochemical (IHC) results. The intensity of HER2 staining was semiquantitatively scored as 0, 1+, 2+, or 3+. Tumors with a 3+ score were defined as HER2-positive, and tumors with a 0 or 1+ score were defined as HER2-negative. The fluorescence in-situ hybridization (FISH) technique was used to determine HER2 status for tumors with a 2+ score. According to the IHC results of ER, PR, HER2, and Ki-67, breast cancer molecular subtypes were classified into four types, including luminal A type, luminal B type, HER2-enriched type, and triple-negative type.
Statistical analyses
All statistical analyses were carried out using the software SPSS 23 (IBM Corp., Armonk, NY, USA). Graphs were drawn using GraphPad Prism 8.0.2 (GraphPad Software, San Diego, CA, USA), and MedCalc 20.106 (MedCalc Software Ltd., Ostend, Belgium). Consistency and Bland-Altman tests were performed to assess the agreement of quantitative parameters between the two readers. An intraclass correlation coefficient (ICC) of <0.4 showed poor consistency of the diagnostic test, and a diagnostic test with an ICC value of >0.75 was considered to indicate good consistency. Categorical variables including tumor location, menopausal status, family history, and molecular subtypes of breast cancers were compared between tumors with and without ALN metastasis using the χ2 test or Fisher’s exact test. The Kolmogorov-Smirnov test was used to test normal distribution of the continuous parameters. Mean ± standard deviation and the median and interquartile range (IQR) were used to express normally and nonnormally distributed data, respectively. Quantitative spectral CT parameters and histogram metrics were compared between metastatic and nonmetastatic ALNs using the Student’s t-test or Mann-Whitney U test for normally and nonnormally distributed data, respectively. If a significant difference was obtained for more than two parameters among the clinical, quantitative spectral CT, or histogram parameters, these parameters were analyzed using multivariate logistic regression models to determine whether they had independent predictive value. Odds ratios (OR) and corresponding 95% confidence intervals (CI) were calculated as part of these logistic regressions. The diagnostic ability of the model to predict ALN metastasis status was also assessed using receiver operating characteristic (ROC) curves to calculate the area under the curve (AUC). The optimal threshold was determined according to the Youden index. A P value <0.05 was considered statistically significant.
With regard to sample size, a previous study (16) showed that the AUC of the VP normalized iodine concentration was 0.74 for the differentiation between metastatic and nonmetastatic ALNs. The ratio between the nonmetastatic ALNs group and the metastatic ALNs group was 1.00, and thus a sample size of at least 56 patients was required. Type I error was 5%, and Type II error was 10%.
Results
Patient characteristics
Among the 216 patients enrolled, 103 participants were excluded because the primary tumor was not visible on CT imaging (n=3) or due to the presence of benign breast lesions (n=22), breast cancer types other than invasive ductal carcinoma or invasive lobular carcinoma (n=2), lesions with nonmass enhancement (n=44), multiple lesions (n=14), or a tumor size exceeding 5 cm (n=11); those with negative or no fine-needle aspiration results (n=7) were also excluded. Finally, 113 participants were included in our study (Figure 1). Of these, 51 patients had ALN metastasis, and 62 patients had no ALNs metastasis. The clinical characteristics of the 113 women are summarized in Figure 1. Of these, tumor size, molecular subtypes, and tumor location differed significantly between the ALN-negative and ALN-positive groups (P<0.05). No significant differences were observed between the two sets in terms of age, menopausal status, and family history of breast cancer (Table 1).
Table 1
Characteristics | Metastatic ALNs | Nonmetastatic ALNs | P value |
---|---|---|---|
Numbers | 51 | 62 | |
Age (years)a | 53.14±1.28 | 55.03±1.41 | 0.332 |
Menopausal (n) | 0.800 | ||
Yes | 30 | 35 | |
No | 21 | 27 | |
Family history of breast cancer (n) | 0.677 | ||
Yes | 1 | 2 | |
No | 50 | 60 | |
Tumor size (cm)b | 2.27 (1.91–2.93) | 1.82 (1.57–2.16) | <0.001 |
Affected side | 0.146 | ||
Left | 25 | 22 | |
Right | 26 | 40 | |
Tumor position (n) | 0.004 | ||
SIQ | 2 | 20 | |
LIQ | 9 | 10 | |
SLQ | 25 | 18 | |
LLQ | 10 | 11 | |
Others | 5 | 3 | |
Molecular subtypes (n) | 0.013 | ||
Luminal A | 11 | 29 | |
Luminal B | 25 | 15 | |
HER-2 enriched | 12 | 12 | |
Triple negative | 3 | 6 |
a, mean ± standard deviation; b, data in parentheses refer to IQR. ALN, axillary lymph node; SIQ, superior inner quadrant; LIQ, lower inner quadrant; SLQ, superior lateral quadrant; LLQ, lower lateral quadrant; HER-2, human epidermal growth factor receptor 2; IQR, interquartile range.
Results of consistency analysis
Quantitative spectral CT parameters were consistent between the two radiologists. The ICCs for all the parameters were >0.75, indicating good consistency (Figure 2).
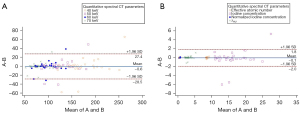
Comparison of quantitative spectral CT parameters between breast cancers with metastatic and nonmetastatic ALNs
Quantitative spectral CT parameters of tumors of metastatic and nonmetastatic ALNs groups are listed in Table 2. There were no significant differences between the two groups (P>0.05) for any of the quantitative tumor parameters.
Table 2
Quantitative spectral CT parameters of tumor | Metastatic ALN group | Nonmetastatic ALN group | P value |
---|---|---|---|
CT values of monochromatic images (HU)a | |||
40 keV | 172.71±46.04 | 168.45±44.57 | 0.619 |
50 keV | 135.53±31.12 | 123.39±31.58 | 0.720 |
60 keV | 96.49±22.12 | 95.65±23.83 | 0.849 |
70 keV | 78.42±16.64 | 78.39±19.14 | 0.995 |
λHUb | 2.88 (2.51–3.75) | 3.00 (2.31–3.49) | 0.457 |
Iodine concentration (100 μg/cm3)a | 16.28±5.29 | 16.94±4.67 | 0.426 |
Normalized iodine concentrationb | 0.27 (0.32–0.46) | 0.38 (0.32–0.46) | 0.940 |
Effective atomic numbera | 8.58±0.28 | 8.54±0.25 | 0.430 |
a, mean ± standard deviation; b, data in parentheses refer to IQR. λHU, the slope of energy spectrum curve. CT, computed tomography; ALN, axillary lymph node; IQR, interquartile range.
Comparisons of iodine map histogram parameters between breast cancers with metastatic and nonmetastatic ALNs
The histogram parameters of iodine maps of breast cancers with metastatic and nonmetastatic ALNs are shown in Table 3. The maximum, 10th percentile, range, and energy were significantly higher in the metastatic ALN group than in the nonmetastatic ALN group (P<0.05), in particular the energy. Parametric maps of representative cases of the metastatic and nonmetastatic ALNs groups are shown in Figure 3.
Table 3
Parameters | Metastatic ALNs | Nonmetastatic ALNs | P value |
---|---|---|---|
Mean (100 μg/cm3)a | 14.59±3.59 | 13.37±3.52 | 0.072 |
Median (100 μg/cm3) | 14.00 (13.00, 18.00) | 13.00 (11.75, 16.00) | 0.057 |
Minimum (100 μg/cm3) | 1.00 (1.00, 1.00) | 1.00 (1.00, 2.25) | 0.604 |
Maximum (100 μg/cm3) | 30.00 (26.00, 34.00) | 27.00 (23.75, 30.25) | 0.009 |
Variance | 19.20 (15.63, 26.39) | 17.85 (13.09, 21.44) | 0.080 |
Skewness | −0.07 (−0.23, 0.75) | −0.06 (−0.26, 0.17) | 0.760 |
Kurtosis | 2.82 (2.60, 3.00) | 2.81 (2.58, 3.03) | 0.791 |
Energy (×105) | 18.48 (9.00, 35.13) | 6.34 (3.50, 10.69) | <0.001 |
Range | 28.00 (24.00, 32.00) | 24.00 (22.00, 29.00) | 0.004 |
10th percentile (100 μg/cm3) | 9.00 (7.00, 10.00) | 7.00 (6.00, 9.00) | 0.050 |
90th percentile (100 μg/cm3) | 19.00 (18.00, 23.00) | 18.00 (15.75, 21.00) | 0.059 |
Unless otherwise indicated, data in parentheses refer to IQR. a, mean ± standard deviation. ALN, axillary lymph node; IQR, interquartile range.
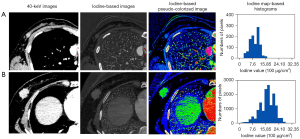
Multivariate analysis and diagnostic performance
Multivariate logistic regression was used to examine the independent prognostic value of each variable after adjusting for potential confounding effects of other variables, and the results suggested that tumor location and energy were independent predictors of metastatic ALNs in breast cancers (Table 4, Figure 4). The combination of tumor location and energy yielded an AUC of 0.824 for the differentiation between metastatic and nonmetastatic ALN groups. The ROC curve showed that the optimal threshold for distinguishing metastatic ALNs from nonmetastatic ALNs was 0.438, and the sensitivity and specificity were 72.5% (37 of 51) and 74.2% (46 of 62), respectively (Figure 5, Table 5).
Table 4
Variables | OR | 95% CI | P value |
---|---|---|---|
Location of tumors | 9.482 | 1.046–85.983 | 0.046 |
Tumor size (cm) | 0.618 | 0.179–1.141 | 0.448 |
Molecular subtypes | 3.417 | 0.472–24.725 | 0.224 |
Maximum (100 μg/cm3) | 1.111 | 0.826–1.494 | 0.486 |
Energy (×105) | 1.097 | 1.044–1.152 | <0.001 |
Range | 0.978 | 0.894–1.069 | 0.621 |
10th percentile (100 μg/cm3) | 0.921 | 0.749–1.133 | 0.436 |
ALN, axillary lymph node; OR, odds ratio; CI, confidence interval.
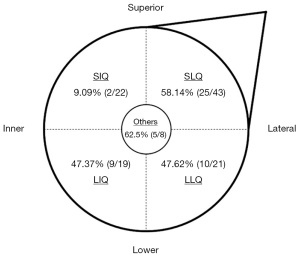
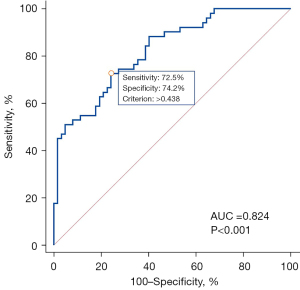
Table 5
Diagnostic test | Pathological findings | Total | |
---|---|---|---|
Positive | Negative | ||
Positive | 37 | 16 | 53 |
Negative | 14 | 46 | 60 |
Total | 51 | 62 | 113 |
ALN, axillary lymph node.
Discussion
In this study, we demonstrated that iodine maps are a clinically feasible and available method, which can aid in the preoperative identification of ALN metastasis in early-stage breast cancers. To our knowledge, this is the first study to evaluate the effectiveness of histogram parameters obtained from iodine maps to predict ALN pathological status in early-stage breast cancers. Using whole-lesion histogram analyses, iodine map-derived features combined with tumor locations showed favorable and effective differentiating functions for ALN with and without metastasis in early-stage breast cancer, and the AUC were over 0.80. The results suggested that iodine map histogram features of primary breast tumors carry potentially helpful information, which can be used as a noninvasive method to predict ALN metastasis status.
Although imaging methods have greatly facilitated the direct assessment of ALN status in breast cancers, for instance, by visualizing incrassated cortexes, loss of fatty hilum, unclear margins, or irregular shapes as features of metastatic ALN, only visualized nodes can be analyzed. Moreover, most early-stage breast cancers with clinically negative ALN have no suspicious signs on imaging. Some MRI features of primary breast tumor were closely associated with ALN metastases, such as irregular shapes, larger tumors, equal or slightly elevated T2WI signal, heterogeneous enhancement, and washout time intensity curve (TIC) (17). Xu et al. (18) showed that primary tumor biopsy whole-slide images (WSIs) as a complementary imaging tool has the potential for ALN metastasis prediction. Zhou et al. (19) demonstrated that a deep learning algorithm from US images of primary breast cancer has high accuracy in predicting ALN metastasis. The findings from this study are consistent with the results of these previous studies, which demonstrated that imaging features of primary breast cancers are associated with ALN metastasis.
Regarding clinical characteristics, we found that tumor size, molecular subtypes, and location were significantly associated with ALN metastases, and tumor location was an independent risk factor. Larger tumor volume indicated rapid tumor growth with higher malignancy. The present study showed that tumor size was significantly larger in the metastatic ALNs group than in the nonmetastatic ALNs group, consistent with previous results (17). The relationship between ALN status and molecular subtype remains controversial. In our study, luminal B type (62.5%) was associated with a statistically higher risk of metastatic ALNs than luminal A type (27.5%) and triple-negative subtypes (33.3%), but we failed to identify molecular subtype as an independent predictor of ALN metastasis. The results were the same as those of a previous study (20); however, another recent single-center study demonstrated that luminal B type and HER2 overexpression were independent and statistically significant predictors of nonsentinel lymph node metastasis, in contrast to luminal A type (21). Our data demonstrate that tumor location is an independent predictive factor for ALN metastases, and superior-inner-quadrant tumors had a lower frequency of ALN metastases than other quadrant tumors. This finding is supported by other published studies (22-24). These results are consistent with the anatomy of mammary lymphatic drainage, in that inner quadrant tumors are more likely to have alternative drainage to the internal mammary chain (24).
In our study, histogram metrics including energy, range, maximum, minimum, and 10th percentiles were significant in univariate analysis in terms of predicting ALN metastasis status. The feature of histogram metrics, including maximum, minimum, and 10th percentiles, can estimate the distribution character of a continuous variable, besides, energy and range can reflect texture feature. These features reflect the heterogeneity of a tumor. Previous studies (25-27) have demonstrated heterogeneity of tumor perfusion, especially for necrosis and microvessel density (MVD), which are related to aggressive tumor behavior and decreased survival rate, which in turn are prognostic factors for breast cancers. Also, Lenga et al. (15) found that iodine map radiomics can serve as an additional tool for assessing the risk of metastasis in breast cancers. Different perfusion and permeability exist in the intratumorally regions of well-oxygenated tissue, hypoxia, or necrosis, which may be reflected by the heterogeneity of iodine maps. Angiogenesis is the key mechanism for cancer cell invasion and metastasis (28,29). Contrast-enhanced CT allows assessment of tumor angiogenesis (30) and can also be used to detect necrosis within tumors (31). However, spectral CT technology allows selective visualization and quantification of iodine-related attenuation and thus enables more accurate quantification of tumor blood supply than routine enhanced CT (32). The iodine map of spectral CT, which depicts the distribution of iodine in tissues, is strongly related to blood vessel density and blood volume of the lesion, and it can be used as an imaging substitute for tumor angiogenesis assessment (33). Histogram analysis of iodine maps estimates the probability distribution of iodine and evaluates the spatial distribution of gray values, which can yield more comprehensive information about tumor heterogeneity (34). Therefore, based on our results, we believe that histogram analysis of iodine maps can capture the status of blood supply within the tumor and may provide predictive metastases information.
In our study, quantitative spectral CT parameters of breast tumors included CT values of monochromatic images, λHU, iodine concentration, normalized iodine concentration, and effective atomic number, which could not be used to identify ALN metastasis in patients with early-stage breast cancer. Quantitative spectral CT parameters of breast tumor depend on the selected ROI, which was placed on a representative section within the tumor. It may be limited by the inaccuracies caused by the variations in ROI size and position. Whole-tumor volume analysis includes the sampling of the entire tumor, which may minimize sampling bias. In addition, it may better capture inherent intratumoral heterogeneity and improve the accuracy of tumor measurements (35). Also, histogram metrics can not only contribute median values but also other distribution parameters for the assessment of ALN metastasis status. Thus, our results suggest that whole-tumor-derived histogram metrics might be the preferred approach for the assessment of ALN metastasis status in early-stage breast cancer.
Due to its ease of use, US is the first-choice preoperative imaging modality for breast cancers; however, it is somewhat affected by the operator’s skills and approach to manipulation. One systematic review of the literature reported that the sensitivity and specificity of US depicting metastatic lymph nodes ranges from 49% to 87% and 55% to 97%, respectively (36). As such, US will lead to unstable results. In our study, the sensitivity and specificity of our method were comparable with those of axillar US. Here, we used a semiautomatic segmentation technique to segment the whole lesion, which was independent of the physician’s skill. Hence, our method might be more reliable and stable. Furthermore, chest CT can evaluate not only ALN status but also lung and bone metastases. Spectral CT at low keV virtual monochromatic images (VMIs) has superior ability in discriminating between breast carcinomas (37). In the current study, the ROIs were drawn on the solid portion of tumors based on 40 keV images, which can improve the accuracy of the segmentation. Although spectral CT selective quantification has been evaluated in other body regions (38), it has not been used in breast cancer for this particular purpose but instead for prediction of locoregional stage (39), nature of breast masses (40), and distant metastases (15).
Our study had several limitations. First, as a retrospective study, spectral CT was used mainly for locally advanced breast cancers, which led to a limited number of cases for analysis. Moreover, we excluded patients who had undergone biopsies before CT scans, which can maximize inclusion of inherent intratumoral heterogeneity and improve tumor assessment, but may also have led to selection bias. In future study, we will further explore the diagnostic value of our method in patients who had biopsies before the CT scan. Second, our protocol was established for chest examination, and the delay in scanning after contrast medium administration may be too short for proper enhancement of breast cancer. Third, the sensitivity of this preliminary method needs to be further improved. Additional studies, including image radiomic features of tumor, peritumoral areas, and lymph nodes shown in iodine maps to predict ALN status, are required. Also, it is important to assess whether it is possible to use spectral CT scanning as an alternative to SLNB in the appropriate population. Finally, no external validation through other institutions took place. External validation and multicenter clinical trials are, therefore, needed to demonstrate generalizability and provide more convincing evidence.
Conclusions
In conclusion, our preliminary results indicated that whole-lesion histogram parameters derived from iodine maps may be used as a complementary noninvasive method to preoperatively identify ALN metastasis in early-stage breast cancers.
Acknowledgments
Funding: This work was supported by the China International Medical Foundation and Nature Science Foundation of Fujian Province (Nos. Z-2014-07-2003-15 and 2021J01773).
Footnote
Reporting Checklist: The authors have completed the STARD reporting checklist. Available at https://qims.amegroups.com/article/view/10.21037/qims-22-253/rc
Conflicts of Interest: All authors have completed the ICMJE uniform disclosure form (available at https://qims.amegroups.com/article/view/10.21037/qims-22-253/coif). The authors have no conflicts of interest to declare.
Ethical Statement: The authors are accountable for all aspects of the work in ensuring that questions related to the accuracy or integrity of any part of the work are appropriately investigated and resolved. The study was conducted in accordance with the Declaration of Helsinki (as revised in 2013). This retrospective single-center study was approved by the Ethics Committee of Fujian Medical University Union Hospital, and individual consent for this retrospective analysis was waived.
Open Access Statement: This is an Open Access article distributed in accordance with the Creative Commons Attribution-NonCommercial-NoDerivs 4.0 International License (CC BY-NC-ND 4.0), which permits the non-commercial replication and distribution of the article with the strict proviso that no changes or edits are made and the original work is properly cited (including links to both the formal publication through the relevant DOI and the license). See: https://creativecommons.org/licenses/by-nc-nd/4.0/.
References
- Sung H, Ferlay J, Siegel RL, Laversanne M, Soerjomataram I, Jemal A, Bray F. Global Cancer Statistics 2020: GLOBOCAN Estimates of Incidence and Mortality Worldwide for 36 Cancers in 185 Countries. CA Cancer J Clin 2021;71:209-49. [Crossref] [PubMed]
- Imbriaco M. Use of Pretreatment Breast MRI to Predict Failed Sentinel Lymph Node Identification after Neoadjuvant Chemotherapy. Radiology 2020;295:283-4. [Crossref] [PubMed]
- Veronesi U, Paganelli G, Viale G, Luini A, Zurrida S, Galimberti V, Intra M, Veronesi P, Robertson C, Maisonneuve P, Renne G, De Cicco C, De Lucia F, Gennari R. A randomized comparison of sentinel-node biopsy with routine axillary dissection in breast cancer. N Engl J Med 2003;349:546-53. [Crossref] [PubMed]
- Esposito E, Di Micco R, Gentilini OD. Sentinel node biopsy in early breast cancer. A review on recent and ongoing randomized trials. Breast 2017;36:14-9. [Crossref] [PubMed]
- Wilke LG, McCall LM, Posther KE, Whitworth PW, Reintgen DS, Leitch AM, Gabram SG, Lucci A, Cox CE, Hunt KK, Herndon JE 2nd, Giuliano AE. Surgical complications associated with sentinel lymph node biopsy: results from a prospective international cooperative group trial. Ann Surg Oncol 2006;13:491-500. [Crossref] [PubMed]
- Leenders MW, Broeders M, Croese C, Richir MC, Go HL, Langenhorst BL, Meijer S, Schreurs WH. Ultrasound and fine needle aspiration cytology of axillary lymph nodes in breast cancer. To do or not to do? Breast 2012;21:578-83. [Crossref] [PubMed]
- Diepstraten SC, Sever AR, Buckens CF, Veldhuis WB, van Dalen T, van den Bosch MA, Mali WP, Verkooijen HM. Value of preoperative ultrasound-guided axillary lymph node biopsy for preventing completion axillary lymph node dissection in breast cancer: a systematic review and meta-analysis. Ann Surg Oncol 2014;21:51-9. [Crossref] [PubMed]
- Ecanow JS, Abe H, Newstead GM, Ecanow DB, Jeske JM. Axillary staging of breast cancer: what the radiologist should know. Radiographics 2013;33:1589-612. [Crossref] [PubMed]
- Uematsu T, Sano M, Homma K. In vitro high-resolution helical CT of small axillary lymph nodes in patients with breast cancer: correlation of CT and histology. AJR Am J Roentgenol 2001;176:1069-74. [Crossref] [PubMed]
- Grillet F, Busse-Coté A, Calame P, Behr J, Delabrousse E, Aubry S. COVID-19 pneumonia: microvascular disease revealed on pulmonary dual-energy computed tomography angiography. Quant Imaging Med Surg 2020;10:1852-62. [Crossref] [PubMed]
- Weidman EK, Plodkowski AJ, Halpenny DF, Hayes SA, Perez-Johnston R, Zheng J, Moskowitz C, Ginsberg MS, Dual-Energy CT. Angiography for Detection of Pulmonary Emboli: Incremental Benefit of Iodine Maps. Radiology 2018;289:546-53. [Crossref] [PubMed]
- Atalay B, Ediz SS, Ozbay NO. Apparent Diffusion Coefficient in Predicting the Preoperative Grade of Meningiomas. J Coll Physicians Surg Pak 2020;30:1126-32. [Crossref] [PubMed]
- Liu HL, Zong M, Wei H, Lou JJ, Wang SQ, Zou QG, Shi HB, Jiang YN. Preoperative predicting malignancy in breast mass-like lesions: value of adding histogram analysis of apparent diffusion coefficient maps to dynamic contrast-enhanced magnetic resonance imaging for improving confidence level. Br J Radiol 2017;90:20170394. [Crossref] [PubMed]
- Kim JY, Kim JJ, Hwangbo L, Lee JW, Lee NK, Nam KJ, Choo KS, Kang T, Park H, Son Y, Grimm R. Diffusion-weighted MRI of estrogen receptor-positive, HER2-negative, node-negative breast cancer: association between intratumoral heterogeneity and recurrence risk. Eur Radiol 2020;30:66-76. [Crossref] [PubMed]
- Lenga L, Bernatz S, Martin SS, Booz C, Solbach C, Mulert-Ernst R, Vogl TJ, Leithner D. Iodine Map Radiomics in Breast Cancer: Prediction of Metastatic Status. Cancers (Basel) 2021;13:2431. [Crossref] [PubMed]
- Zhang X, Zheng C, Yang Z, Cheng Z, Deng H, Chen M, Duan X, Mao J, Shen J. Axillary Sentinel Lymph Nodes in Breast Cancer: Quantitative Evaluation at Dual-Energy CT. Radiology 2018;289:337-46. [Crossref] [PubMed]
- Zhao M, Wu Q, Guo L, Zhou L, Fu K. Magnetic resonance imaging features for predicting axillary lymph node metastasis in patients with breast cancer. Eur J Radiol 2020;129:109093. [Crossref] [PubMed]
- Xu F, Zhu C, Tang W, Wang Y, Zhang Y, Li J, Jiang H, Shi Z, Liu J, Jin M. Predicting Axillary Lymph Node Metastasis in Early Breast Cancer Using Deep Learning on Primary Tumor Biopsy Slides. Front Oncol 2021;11:759007. [Crossref] [PubMed]
- Zhou LQ, Wu XL, Huang SY, Wu GG, Ye HR, Wei Q, Bao LY, Deng YB, Li XR, Cui XW, Dietrich CF. Lymph Node Metastasis Prediction from Primary Breast Cancer US Images Using Deep Learning. Radiology 2020;294:19-28. [Crossref] [PubMed]
- Nowikiewicz T, Wnuk P, Małkowski B, Kurylcio A, Kowalewski J, Zegarski W. Application of artificial neural networks for predicting presence of non-sentinel lymph node metastases in breast cancer patients with positive sentinel lymph node biopsies. Arch Med Sci 2017;13:1399-407. [Crossref] [PubMed]
- Wang NN, Yang ZJ, Wang X, Chen LX, Zhao HM, Cao WF, Zhang B. A mathematical prediction model incorporating molecular subtype for risk of non-sentinel lymph node metastasis in sentinel lymph node-positive breast cancer patients: a retrospective analysis and nomogram development. Breast Cancer 2018;25:629-38. [Crossref] [PubMed]
- Gann PH, Colilla SA, Gapstur SM, Winchester DJ, Winchester DP. Factors associated with axillary lymph node metastasis from breast carcinoma: descriptive and predictive analyses. Cancer 1999;86:1511-9. [Crossref] [PubMed]
- Maibenco DC, Weiss LK, Pawlish KS, Severson RK. Axillary lymph node metastases associated with small invasive breast carcinomas. Cancer 1999;85:1530-6. [Crossref] [PubMed]
- Bevilacqua J, Cody H 3rd, MacDonald KA, Tan LK, Borgen PI, Van Zee KJ. A prospective validated model for predicting axillary node metastases based on 2,000 sentinel node procedures: the role of tumour location corrected. Eur J Surg Oncol 2002;28:490-500. [Crossref] [PubMed]
- Weidner N, Folkman J, Pozza F, Bevilacqua P, Allred EN, Moore DH, Meli S, Gasparini G. Tumor angiogenesis: a new significant and independent prognostic indicator in early-stage breast carcinoma. J Natl Cancer Inst 1992;84:1875-87. [Crossref] [PubMed]
- Li J, Zhang Y, Zhang W, Gao Y, Jia S, Guo J. Contrast enhanced computed tomography is indicative for angiogenesis pattern and display prognostic significance in breast cancer. BMC Cancer 2014;14:672. [Crossref] [PubMed]
- Ma ZB, Ge FQ, Wang WX, Sun ZQ. A clinical application of dynamic contrast-enhanced CT (DCE-CT) in patients with variously differentiated breast ductal carcinoma. J Xray Sci Technol 2018;26:977-86. [Crossref] [PubMed]
- Lorusso G, Rüegg C. The tumor microenvironment and its contribution to tumor evolution toward metastasis. Histochem Cell Biol 2008;130:1091-103. [Crossref] [PubMed]
- McDonnell CO, Hill AD, McNamara DA, Walsh TN, Bouchier-Hayes DJ. Tumour micrometastases: the influence of angiogenesis. Eur J Surg Oncol 2000;26:105-15. [Crossref] [PubMed]
- Hattori Y, Gabata T, Matsui O, Mochizuki K, Kitagawa H, Kayahara M, Ohta T, Nakanuma Y. Enhancement patterns of pancreatic adenocarcinoma on conventional dynamic multi-detector row CT: correlation with angiogenesis and fibrosis. World J Gastroenterol 2009;15:3114-21. [Crossref] [PubMed]
- Shan X, Wang D, Chen J, Xiao X, Jiang Y, Wang Y, Fan Y. Necrosis degree displayed in computed tomography images correlated with hypoxia and angiogenesis in breast cancer. J Comput Assist Tomogr 2013;37:22-8. [Crossref] [PubMed]
- Choe J, Lee SM, Do KH, Lee JB, Lee SM, Lee JG, Seo JB. Prognostic value of radiomic analysis of iodine overlay maps from dual-energy computed tomography in patients with resectable lung cancer. Eur Radiol 2019;29:915-23. [Crossref] [PubMed]
- Son JY, Lee HY, Kim JH, Han J, Jeong JY, Lee KS, Kwon OJ, Shim YM. Quantitative CT analysis of pulmonary ground-glass opacity nodules for distinguishing invasive adenocarcinoma from non-invasive or minimally invasive adenocarcinoma: the added value of using iodine mapping. Eur Radiol 2016;26:43-54. [Crossref] [PubMed]
- Li Z, Ai T, Hu Y, Yan X, Nickel MD, Xu X, Xia L. Application of whole-lesion histogram analysis of pharmacokinetic parameters in dynamic contrast-enhanced MRI of breast lesions with the CAIPIRINHA-Dixon-TWIST-VIBE technique. J Magn Reson Imaging 2018;47:91-6. [Crossref] [PubMed]
- Nougaret S, Vargas HA, Lakhman Y, Sudre R, Do RK, Bibeau F, Azria D, Assenat E, Molinari N, Pierredon MA, Rouanet P, Guiu B. Intravoxel Incoherent Motion-derived Histogram Metrics for Assessment of Response after Combined Chemotherapy and Radiation Therapy in Rectal Cancer: Initial Experience and Comparison between Single-Section and Volumetric Analyses. Radiology 2016;280:446-54. [Crossref] [PubMed]
- Marino MA, Avendano D, Zapata P, Riedl CC, Pinker K. Lymph Node Imaging in Patients with Primary Breast Cancer: Concurrent Diagnostic Tools. Oncologist 2020;25:e231-42. [Crossref] [PubMed]
- Metin Y, Metin NO, Özdemir O, Taşçı F, Kul S. The role of low keV virtual monochromatic imaging in increasing the conspicuity of primary breast cancer in dual-energy spectral thoracic CT examination for staging purposes. Acta Radiol 2020;61:168-74. [Crossref] [PubMed]
- Zou Y, Zheng M, Qi Z, Guo Y, Ji X, Huang L, Gong Y, Lu X, Ma G, Xia S. Dual-energy computed tomography could reliably differentiate metastatic from non-metastatic lymph nodes of less than 0.5 cm in patients with papillary thyroid carcinoma. Quant Imaging Med Surg 2021;11:1354-67. [Crossref] [PubMed]
- Volterrani L, Gentili F, Fausto A, Pelini V, Megha T, Sardanelli F, Mazzei MA. Dual-Energy CT for Locoregional Staging of Breast Cancer: Preliminary Results. AJR Am J Roentgenol 2020;214:707-14. [Crossref] [PubMed]
- Demirler Şimşir B, Krug KB, Burke C, Hellmich M, Maintz D, Coche E. Possibility to discriminate benign from malignant breast lesions detected on dual-layer spectral CT-evaluation. Eur J Radiol 2021;142:109832. [Crossref] [PubMed]