Radiomics models based on multi-sequence MRI for preoperative evaluation of MUC4 status in pancreatic ductal adenocarcinoma: a preliminary study
Introduction
Pancreatic ductal adenocarcinoma (PDAC) is a malignant digestive system tumor and is the third leading cause of death among cancers (1). Although radical resection is the only possible curative treatment, the addition of neoadjuvant chemotherapy can improve the survival rate of patients with PDAC (2). Due to the heterogeneity of tumors, sensitivities toward chemotherapeutic drugs are diverse among different people. Even though progress has been made in diagnosing and treating pancreatic cancer, the prognosis of PDAC is still poor, with a reported 5-year survival rate of 7.2% (3). Therefore, it is essential to discover molecular markers that can be targeted by chemotherapy and used to predict patient prognosis in PADC patients. Recently, individualized cancer treatment has aroused great interest and is being widely studied. The use of molecular biomarkers helps the optimization of appropriate treatment and evaluation of prognosis in patients with cancer (4).
Mucin 4 (MUC4) is a highly glycosylated, membrane-bound protein. The overexpression of MUC4 promotes tumorigenesis and increases the aggressiveness of PDAC (5). The high expression of MUC4 promotes tumor cell metastasis, regulates the interaction between tumor cells and microenvironmental components, and promotes tumor cell resistance to chemotherapy (6,7). Studies have confirmed that the PDAC group with a high expression of MUC4 is less sensitive to gemcitabine (8,9). The high expression of MUC4 is closely related to the poor prognosis of patients with PDAC (10). Therefore, it is essential to detect the status of MUC4 for optimizing individualized treatment and evaluating the prognosis of patients with PDAC.
Immunohistochemistry (IHC) is a conventional, albeit invasive method used for detecting MUC4 expression status. Magnetic resonance imaging (MRI) is capable of noninvasively detecting pancreatic malignancy. However, MRI is generally not used to describe features other than tumor location, size, and general appearance. Radiomics can obtain a large quantity of potential feature data that is not seen by the naked eye from traditional images in a noninvasive and high throughput way and quantitatively analyze the obtained feature data to provide a basis for treatment decision-making (11). It relies on objective computer measurements rather than a radiologist’s subjective assessment (12). Radiogenomics is an emerging field in which the relationship between radiological features and genetic characteristics are studied. Routine image data are transformed into mineable quantitative data where quantitative features are extracted and linked to specific genomic profiles and outcomes (13). Some progress has been made in various tumors using radiogenomics applications, such as rectal cancer, breast cancer, and brain glioblastoma (14-16). There has been no report, however, about the association between radiomics and MUC4 expression status in PDAC patients.
This study aimed to identify the correlation between radiomics and MUC4 in PDAC. The radiomics features were extracted from multi-sequence MRI, and radiomics models were developed to explore their utility in preoperatively predicting MUC4 expression levels in PDAC. We present the following article in accordance with the TRIPOD reporting checklist (available at https://qims.amegroups.com/article/view/10.21037/qims-22-112/rc).
Methods
Patients
The study was conducted in accordance with the Declaration of Helsinki (as revised in 2013). This retrospective study received ethical approval from the Institutional Review Board (IRB), and the requirement for informed consent was waived. Consecutive patients with pathologically confirmed PDAC and preoperative MRI admitted at our institution from March 2016 to September 2019 were identified. The paraffin sections of each patient were collected for IHC detection of the status of MUC4 expression in PDAC. Our study recruited patients who met the following criteria: (I) total tumor resection and a pathological diagnosis of PDAC; (II) available IHC detection of MUC4 expression status; (III) available preoperative multi-sequence MR scan; and (IV) available complete clinical data sets. Patients were excluded according to the following criteria: (I) absence of paraffin-embedded sections or inability to evaluate MUC4 expression; (II) previous administration of preoperative therapy, such as chemoradiotherapy; (III) incomplete imaging data or poor image quality; or (IV) >30-day interval between surgery and MR examination.
The flowchart of patient recruitment is shown in Figure 1. Finally, 52 patients (mean age, 64 years; males =30) with PDAC were included in our study. Clinical data, including age, gender, tumor location, tumor size, tumor differentiation, lymph node status, and serological carbohydrate antigen 19-9 (CA19-9) levels, were recorded from the admission notes. Tumor size was based on the product of the lesion’s length, width, and height measured on surgical specimens. According to the pathological results, lymph node status was divided into positive and negative status. In addition, we followed up on the overall survival (OS) rate of patients for 1 year. The OS was defined as the time interval between the date of operation and the date of death or the last known date of life. Taking the follow-up for 1 year as the boundary, more than 1 year of survival was deemed survival, and less than 1 year of survival was deemed non-survival or dead. We compared the correlation between OS and MUC4 expression levels.
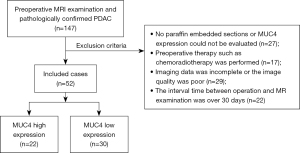
Immunohistochemistry for MUC4
The MUC4 expression level was identified using standard IHC methods. Paraffin sections of PDAC were collected from the Pathology Department of our unit. The department made 5 µm-thick sections and used MUC4-specific antibodies (Abcam, Cambridge, MA, USA) for IHC staining. Two experienced pathologists (with 5 and 8 years of experience, respectively) performed the IHC scoring independently and were blinded to the clinical data of these patients; they discussed the results when their scores were inconsistent. The score was based on the degree of staining and the proportion of positive cells (H-score). The evaluation criteria for the degree of staining were as follows: no color, 0 score; pale yellow, 1 score; claybank, 2 scores; and brown, 3 scores. The evaluation criteria for the proportion of positive cells were as follows: no more than 5%, 0 score; 5–25%, 25 scores; 25–50%, 50 scores; 50–75%, 75 scores; and over 75%, 100 scores. Positive staining over 5% was defined as positive expression. High and low expressions of MUC4 were defined as H-score >100 and H-score ≤100, respectively (8). The IHC and corresponding histopathological results of high and low MUC4 expression in different patients are shown in Figure 2.
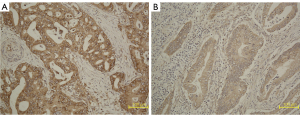
MRI techniques
All patients were scanned with a 3.0-T MR machine (MR 750; GE Medical Systems, Waukesha, WI, USA; Achieva, Philips, the Netherlands) with a 32-channel body phased-array coil. The scan sequence included T2-weighted imaging (T2WI), pre-contrast T1-weighted imaging (T1WI), and the arterial, portal-venous, and delayed phases of dynamic contrast-enhanced MRI (DCE-MRI). The contrast agent for dynamic enhancement was gadopentetate dimeglumine (Magnevist, Bayer Schering, Guangzhou, China); the dose was 0.2 mmol/kg (approximately 20 mL), which was injected intravenously with a pressure syringe (Spectris MR Injection System; MEDRAD, Inc., Pittsburgh, PA, USA) at 2–3 mL/s, followed by flushing with 20 mL of saline. Scanning was performed at 30, 60, and 120 s after the injection of contrast agent to obtain images of the arterial phase, portal vein phase, and delayed phase, respectively. Details of the parameters are shown in Table 1.
Table 1
Sequence | TR (ms) | TE (ms) | Slice thickness (mm) | Slice gap (mm) | Matrix | FOV (cm) |
---|---|---|---|---|---|---|
Axial T2WI | 4,500–6,000 | 90–120 | 6 | 1 | 320×256 | 34×34 |
Axial fat suppression T2WI | 2,500–3,000 | 90–110 | 6 | 1 | 384×384 | 34×34 |
Axial 3D-LAVA | 3.6–4.4 | 1.7–1.9 | 5.2 | 0 | 224×192 | 36×36 |
Dynamic enhancement 3D-LAVA | 3.6–4.4 | 1.7–1.9 | 5.2 | 0 | 224×192 | 36×36 |
MRI, magnetic resonance imaging; TE, echo time; T2WI, T2-weighted image; TR, repetition time; FOV, field of view; LAVA, liver acquisition with volume acceleration.
Image segmentation, preprocessing, and feature extraction
Two radiologists with experience in abdominal diagnosis (Reader 1 with 4 years and Reader 2 with 7 years, respectively) manually outlined the region of interest (ROI) based on arterial phase and portal phase around the tumor edge, layer by layer, without knowing the clinical and pathological data of the patient. Two corresponding independent feature sets (arterial phase, portal phase) were produced, and radiomics models were built. To reduce the influence of the volume effect on the peripancreatic fat space or normal pancreas, the outlined ROI was slightly smaller than the area of the actual lesion (17). The process was implemented using an open-source software package, Imaging Biomarker Explorer (IBEX, β1.0; University of Texas MD Anderson Cancer Center, Houston, TX, USA; http://bit.ly//), which runs on MATLAB 2016b (The MathWorks Inc., Natick, MA, USA). The radiomics workflow is shown in Figure 3. Four sets of features from IBEX were opted for, including the intensity histogram, the gray-level co-occurrence matrix (GLCM), the gray-level run-length matrix (GLRLM), and the shape. We identified 350 radiomics features in arterial phase and portal phase, each of the independent feature sets, respectively (see Appendix 1, which elucidates the features of information). All patients included in this study had identical MR scan sequences and parameters. Thus, no image preprocessing methods were applied. To eliminate the effect of different dimensions of features and to make the results more reliable, we performed z-score standardization on all data sets (see Appendix 2, which explains the data preprocessing method).
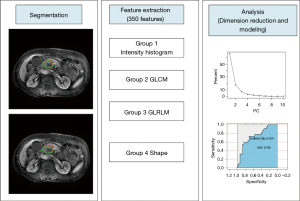
Intraobserver and interobserver agreement
We selected all patients to evaluate the repeatability of radiomics of different feature sets. We drew the ROI contours of the arterial phase and the portal phase from multi-sequenced MRI, thus generating corresponding feature subsets. Intraobserver consistency was checked in the results illustrated by Reader 1, who drew the ROI twice, and the interval time between the two outlines was greater than one week. Inter-observer consistency was checked by examining the outcomes reported by Reader 2, who independently drew the ROI, and comparing them with the consequences of the first outcomes reported by Reader 1. The interclass correlation coefficient (ICC) was used as a measurement index in the intra- and inter-observer consistency evaluation. Intra- and inter-observer consistency were checked for all radiomics features generated from the ROIs extracted by Reader 1 and Reader 2. When the ICC score exceeded 0.75, consistency was considered good (18). Due to the characteristics of voxel size and gray-scale dependence, not all radiomic features reached a satisfactory consistency (19).
Dimensionality reduction and model building
A large number of redundant radiomics features were used in the operation of the classifier, which would cause over-fitting and lead to dimensional disasters. To avoid this, it was necessary to select suitable features through dimensionality reduction. First, univariate analysis, including the independent samples t-test or the Mann-Whiney U test, was applied to identify the features in each data set which exhibited a statistically significant difference between high and low MUC4 expression levels. A false discovery rate (FDR) was used to revise the P-value to decrease a risk type I error. Then, the minimum redundancy maximum relevance (MRMR) algorithm was applied to each data set to select a non-redundant and highly informative set of features. The top 15 features were picked out using MRMR. Then, principal component analysis (PCA) was applied for dimensionality reduction and feature selection. The main component features with the strongest discriminative power and cumulative variance of 90% were selected. The selected features of each dataset were modeled by multivariable logistic regression (see Appendix 3, which explains the process of feature selection and modeling). In addition, the most discriminative features from each dataset and clinical characteristics were combined to generate a joint radiomics model (combined model), and multivariable logistic regression was applied for modeling. A 5-fold, cross-validation method was used to verify the performance of the combined model. Several clinical characteristics, the size, and the differentiation degree of the tumor were selected via univariate analysis for the continuous variables and the Pearson chi-square test for the categorical variables to construct the clinical model. The area under the receiver operating characteristic (ROC) curve (AUC) and other evaluation metrics, such as accuracy, sensitivity, and specificity, were applied to evaluate the performance of these radiomics and clinical models. The DeLong test was used to compare the AUC among the four radiomics and clinical models.
Statistical analysis
Regarding the clinical data, continuous variables were assessed by univariate analysis. Pearson’s chi-square test or Fisher’s exact test were used to assess categorical variables and the data were analyzed using SPSS 23.0 (IBM Corp., Armonk, NY, USA). The dimensionality reduction and model-building processes of the radiomics features, including MRMR, PCA, multivariable logistic regression model, and ROC curve analyses, were implemented in R 3.5.2 (https://www.r-project.org/). Kfoldclass was implemented in Stata 15.0 (Stata Corp., College Station, TX, USA) for the 5-fold cross-validation. P value less than 0.05 was considered statistically significant.
Results
Clinical data
A total of 52 patients with PDAC were included in this retrospective study. There were 22 cases with a high expression and 30 cases with a low expression of MUC4. Among the clinical characteristics, only tumor size and tumor differentiation were significantly different between the high- and low-MUC4 expression groups (P<0.05). We compared the OS between high- and low-MUC4 expression groups and demonstrated that the higher the expression level of MUC4, the lower the 1-year survival rate of PDAC patients. The baseline characteristics were recorded in Table 2. The preoperative MRI of the MUC4 expression status of patients with PDAC is shown in Figure 4.
Table 2
Characteristics | MUC4 high expression (n=22) | MUC4 low expression (n=30) | Total | P value |
---|---|---|---|---|
Age (years), medium [P25, P75] | 66 [54, 68] | 64 [49, 66] | 64 [53, 67] | 0.364 |
Gender | 0.249 | |||
Male | 11 | 19 | 30 | |
Female | 11 | 11 | 22 | |
Location | 0.506 | |||
Head or neck | 19 | 27 | 46 | |
Body or tail | 3 | 3 | 6 | |
Differentiation degree | 0.000* | |||
Highly differentiated | 1 | 9 | 10 | |
Moderately differentiated | 11 | 21 | 32 | |
Poorly differentiated | 10 | 0 | 10 | |
Size (cm3), medium (P25, P75) | 13.75 (5.85, 27.19) | 6.38 (2.09, 17.48) | 7.50 (3.05, 18.00) | 0.042* |
Lymph node status | 0.425 | |||
N− | 14 | 21 | 35 | |
N+ | 8 | 9 | 17 | |
OS | ||||
OS+ | 6 | 20 | 26 | 0.005* |
OS− | 16 | 10 | 26 | |
CA19-9 level (U/mL), medium (P25, P75) | 247.67 (55.74, 972.65) | 90.82 (44.37, 282.34) | 149.96 (47.83, 573.35) | 0.175 |
Data are presented as median (interquartile range). *, significant difference. N−, negative status; N+, positive status. PDAC, pancreatic ductal adenocarcinoma; OS, overall survival; MUC4, mucin 4; CA19-9, carbohydrate antigen 19-9.
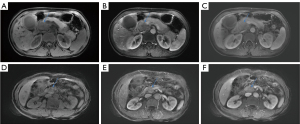
Intra- and inter-observer agreement
The mean values of the interobserver agreement of radiomics features were 0.951 and 0.958 for the arterial phase model and portal phase model feature sets, respectively. For the intraobserver agreement, the mean values were 0.952 and 0.970 for the arterial phase and portal phase feature sets, respectively. Ultimately, through the ICC reliability test, the remaining features of the arterial phase and portal phase datasets were 336 and 334 for the following analysis, respectively.
Dimensionality reduction and model building
After single factor analysis, the selected features with a significant difference were 324 and 307 in the arterial phase and portal phase feature sets. We used the top 15 radiomics features after the MRMR algorithm for PCA analysis. For PCA dimensionality reduction, five principal component features of the arterial phase feature set and four principal component features of the portal phase feature set closely related to the MUC4 expression status of PDAC were selected. Combining these selected features of each feature set and clinical characteristics generated a combined radiomics model, including 11 features. Multivariable logistic regression was performed for the classifier. The AUCs of the arterial phase model, portal phase model, and combined model were 0.732 (0.591–0.872), 0.709 (0.569–0.849), and 0.861 (0.760–0.961), respectively. The sensitivity/specificity of the arterial phase model, portal phase model, and combined model were 0.727/0.700, 0.773/0.600, and 0.909/0.682, respectively. The AUC of the clinical model was 0.666 (0.600–0.682). The sensitivity and specificity of the clinical model were 0.682 and 0.600, respectively (Table 3). The combined model achieved the best performance among these radiomics and clinical models (comparing the AUC of all models: P<0.05), although no statistical significance was observed in the combined model vs. Arterial phase model. These results are shown in Figure 5. We used a 5-fold cross-validation to test the effectiveness of the combined model, and the results are shown in Figure 6.
Table 3
Model | AUC (95% CI) | Sensitivity | Specificity | Accuracy |
---|---|---|---|---|
Arterial phase model | 0.732 (0.591–0.872) | 0.727 | 0.700 | 0.692 |
Portal phase model | 0.709 (0.569–0.849) | 0.773 | 0.600 | 0.596 |
Combined model | 0.861 (0.760–0.961) | 0.909 | 0.682 | 0.933 |
Clinical model | 0.666 (0.600–0.682) | 0.682 | 0.600 | 0.654 |
MUC4, mucin 4; AUC, area under the curve; CI, confidence interval.
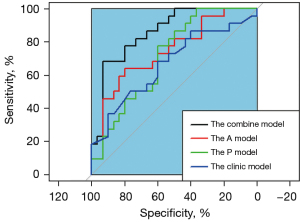
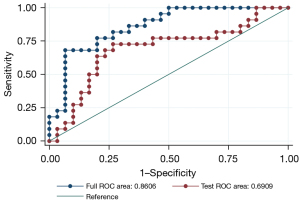
Discussion
In this study, we presented a noninvasive imaging biomarker to preoperatively evaluate the MUC4 expression status of patients with PDAC. Our results suggested that radiomics models based on multi-sequence MRI features have the potential to distinguish between high and low status of MUC4 expression in PDAC.
The microenvironment of the tumor is related to tumorigenesis and its progression. Previous studies (5,10,20) have confirmed that MUC4 increases the aggressiveness and malignancy of PDAC, making the prognosis of patients with PDAC poor. Our study compared the correlation between OS and MUC4 expression levels, and the results are in line with the previous survey. The expression of MUC4 is negatively correlated with the survival rate of patients with PDAC. A series of studies have successfully linked tumor radiomics and biological behavior. For instance, Li et al. (16) used 41 texture features in a logistic regression model that could successfully predict the epidermal growth factor receptor (EGFR) expression status of low-grade glioblastoma. Wang et al. (21) used a radiomics model based on MRI to predict EGFR mutation in patients with lung adenocarcinoma, and achieved good results. However, few studies have established links between radiomics and pancreatic cancer. Permuth et al. (22) demonstrated that radiomics combined with messenger RNA (mRNA) expression could more accurately predict the pathology of intraductal papillary mucinous neoplasms. No study has reported on the application of radiomics in predicting the MUC4 expression status in patients with PDAC.
Our study developed a non-invasive, quantitative, pre-operative radiomics model to predict the level of MUC4 expression in patients with PDAC. Five principal component features of the arterial phase feature set and four principal component features of the portal phase feature set were generated by applying a logistic regression method to each model. The prediction performance of the single sequence radiomics models and the clinical model were poor; the AUC values of the arterial phase model and portal phase model were 0.732 and 0.709, respectively. The AUC values among the arterial phase model, portal phase model, and clinical model showed no statistical significance. This may be associated with the fact that less information was extracted from a single sequence, such that the established model did not work well. In addition, the clinical model established by the size and differentiation degree of a tumor was inherently related to the prognosis of pancreatic cancer, and these clinical indicators were related to the expression of MUC4 in pancreatic cancer. The combined model achieved the best performance with a significantly higher AUC of 0.861 among these models. The same trend was seen in the sensitivity and accuracy; however, specificity was an exception, with that of the specificity of the arterial phase model being higher. These results suggested that combining the arterial phase model, the portal phase model, and the clinical model together would increase the predictive value of the status of MUC4 expression. These results, associated with combining a multi-sequence, could provide more comprehensive information than any individual sequence. Our results were consistent with a previous study (23) which used a multi-sequence radiomics model to evaluate the pathological grade in bladder cancer and demonstrated that the joint model achieved the best performance. In addition, the combined model combined several of the most discriminative principal components selected from every single sequence, giving this model a particular advantage.
Our study also evaluated the relationship between MUC4 expression level and gender, age, tumor location, tumor size, tumor differentiation, lymph node status, and CA19-9 level. Only the degree of tumor differentiation and tumor size significantly differed between the two groups. This may have been caused by the degree of tumor differentiation reflecting the tumor’s biological behavior; the lower the degree of differentiation, the more malignant the tumor. The tumor size was related to the clinical stage and reflected the clinical progress of the tumor (24). In general, the later the tumor stage, the higher the degree of malignancy. A meta-analysis conducted by Huang et al. (25) confirmed that MUC4 expression was related to tumor stage, malignancy, and lymph node status. However, there was no significant difference in the lymph nodes between the high- and low-MUC4 expression groups; this may have been related to their small sample size. In addition, several studies (26,27) have reported that the positive lymph node rate is positively correlated with the number of lymph nodes detected. Thus, the relatively few lymph node examinations conducted by our institution potentially led to a lower detection rate, which may have skewed the result. The clinical model was built by combining tumor size and tumor stage. Compared with the combined model in terms of performance, the combined model performed better. The expression of MUC4 reflected the microenvironment of pancreatic cancer. Radiomics can capture much information which is invisible to the naked eye and can thus reveal the tumor microenvironment.
This study had some limitations. Our research was conducted retrospectively. Prospective studies are needed to assess MUC4 expression status. Furthermore, the small sample size does not support external validation, which is the most critical validation strategy (12). Thus, we used a five-fold cross-validation, which is the most frequently used internal validation approach (28). Multicenter and large-scale research needs to be performed to support our findings. Focusing on the arterial phase and portal phase sequences was another limitation of the study; however, a previous study (29) suggested that pancreatic cancer is better visualized in the arterial and portal phases.
Conclusions
Our study confirmed that the radiomics model based on a multi-sequence MRI has the potentiality to predict MUC4 expression levels in PDAC. Our results provide the foundation for developing a non-invasive diagnostic method and better management of patients with PDAC.
Acknowledgments
We would like to acknowledge all those who helped us during our thesis writing. We want to thank the teachers in our Departments of Pathology and Radiology for their help with the experiments.
Funding: This work was supported by the science and technology strategic cooperation project of Nanchong Municipal (No. 19SXHZ0269), the Science and Technology Strategic Cooperation Project of Nanchong Municipal (No. 20SXQT0250), and the Science and Technology Strategic Project of North Sichuan Medical College (No. CBY20-QA-Z03).
Footnote
Reporting Checklist: The authors have completed the TRIPOD reporting checklist. Available at https://qims.amegroups.com/article/view/10.21037/qims-22-112/rc
Conflicts of Interest: All authors have completed the ICMJE uniform disclosure form (available at https://qims.amegroups.com/article/view/10.21037/qims-22-112/coif). All authors report that this study was supported by the Science and Technology Strategic Cooperation Project of Nanchong Municipal (No. 19SXHZ0269), the Science and Technology Strategic Cooperation Project of Nanchong Municipal (No. 20SXQT0250), and the Science and Technology Strategic Project of North Sichuan Medical College (No. CBY20-QA-Z03). The authors have no other conflicts of interest to declare.
Ethical Statement: The authors are accountable for all aspects of the work in ensuring that questions related to the accuracy or integrity of any part of the work are appropriately investigated and resolved. The study was conducted in accordance with the Declaration of Helsinki (as revised in 2013). This retrospective study received ethical approval from the Institutional Review Board (IRB). Written informed consent was waived from each patient.
Open Access Statement: This is an Open Access article distributed in accordance with the Creative Commons Attribution-NonCommercial-NoDerivs 4.0 International License (CC BY-NC-ND 4.0), which permits the non-commercial replication and distribution of the article with the strict proviso that no changes or edits are made and the original work is properly cited (including links to both the formal publication through the relevant DOI and the license). See: https://creativecommons.org/licenses/by-nc-nd/4.0/.
References
- Siegel RL, Miller KD, Jemal A. Cancer statistics, 2018. CA Cancer J Clin 2018;68:7-30. [Crossref] [PubMed]
- Oettle H, Neuhaus P, Hochhaus A, Hartmann JT, Gellert K, Ridwelski K, Niedergethmann M, Zülke C, Fahlke J, Arning MB, Sinn M, Hinke A, Riess H. Adjuvant chemotherapy with gemcitabine and long-term outcomes among patients with resected pancreatic cancer: the CONKO-001 randomized trial. JAMA 2013;310:1473-81. [Crossref] [PubMed]
- Ko AH. Pancreatic Cancer and the Possibility of Long-term Survival: A Glimmer of Hope?. JAMA Oncol 2016;2:380-1. [Crossref] [PubMed]
- Birnbaum DJ, Bertucci F, Finetti P, Birnbaum D, Mamessier E. Molecular classification as prognostic factor and guide for treatment decision of pancreatic cancer. Biochim Biophys Acta Rev Cancer 2018;1869:248-55. [Crossref] [PubMed]
- Gautam SK, Kumar S, Cannon A, Hall B, Bhatia R, Nasser MW, Mahapatra S, Batra SK, Jain M. MUC4 mucin- a therapeutic target for pancreatic ductal adenocarcinoma. Expert Opin Ther Targets 2017;21:657-69. [Crossref] [PubMed]
- Mimeault M, Johansson SL, Senapati S, Momi N, Chakraborty S, Batra SK. MUC4 down-regulation reverses chemoresistance of pancreatic cancer stem/progenitor cells and their progenies. Cancer Lett 2010;295:69-84. [Crossref] [PubMed]
- Chaturvedi P, Singh AP, Moniaux N, Senapati S, Chakraborty S, Meza JL, Batra SK. MUC4 mucin potentiates pancreatic tumor cell proliferation, survival, and invasive properties and interferes with its interaction to extracellular matrix proteins. Mol Cancer Res 2007;5:309-20. [Crossref] [PubMed]
- Urey C, Andersson B, Ansari D, Sasor A, Said-Hilmersson K, Nilsson J, Andersson R. Low MUC4 expression is associated with survival benefit in patients with resectable pancreatic cancer receiving adjuvant gemcitabine. Scand J Gastroenterol 2017;52:595-600. [Crossref] [PubMed]
- Skrypek N, Duchêne B, Hebbar M, Leteurtre E, van Seuningen I, Jonckheere N. The MUC4 mucin mediates gemcitabine resistance of human pancreatic cancer cells via the Concentrative Nucleoside Transporter family. Oncogene 2013;32:1714-23. [Crossref] [PubMed]
- Saitou M, Goto M, Horinouchi M, Tamada S, Nagata K, Hamada T, Osako M, Takao S, Batra SK, Aikou T, Imai K, Yonezawa S. MUC4 expression is a novel prognostic factor in patients with invasive ductal carcinoma of the pancreas. J Clin Pathol 2005;58:845-52. [Crossref] [PubMed]
- Chang N, Cui L, Luo Y, Chang Z, Yu B, Liu Z. Development and multicenter validation of a CT-based radiomics signature for discriminating histological grades of pancreatic ductal adenocarcinoma. Quant Imaging Med Surg 2020;10:692-702. [Crossref] [PubMed]
- Gillies RJ, Kinahan PE, Hricak H. Radiomics: Images Are More than Pictures, They Are Data. Radiology 2016;278:563-77. [Crossref] [PubMed]
- Bai HX, Lee AM, Yang L, Zhang P, Davatzikos C, Maris JM, Diskin SJ. Imaging genomics in cancer research: limitations and promises. Br J Radiol 2016;89:20151030. [Crossref] [PubMed]
- Oh JE, Kim MJ, Lee J, Hur BY, Kim B, Kim DY, Baek JY, Chang HJ, Park SC, Oh JH, Cho SA, Sohn DK. Magnetic Resonance-Based Texture Analysis Differentiating KRAS Mutation Status in Rectal Cancer. Cancer Res Treat 2020;52:51-9. [Crossref] [PubMed]
- Xie T, Zhao Q, Fu C, Bai Q, Zhou X, Li L, Grimm R, Liu L, Gu Y, Peng W. Differentiation of triple-negative breast cancer from other subtypes through whole-tumor histogram analysis on multiparametric MR imaging. Eur Radiol 2019;29:2535-44. [Crossref] [PubMed]
- Li Y, Liu X, Xu K, Qian Z, Wang K, Fan X, Li S, Wang Y, Jiang T. MRI features can predict EGFR expression in lower grade gliomas: A voxel-based radiomic analysis. Eur Radiol 2018;28:356-62. [Crossref] [PubMed]
- Hodgdon T, McInnes MD, Schieda N, Flood TA, Lamb L, Thornhill RE. Can Quantitative CT Texture Analysis be Used to Differentiate Fat-poor Renal Angiomyolipoma from Renal Cell Carcinoma on Unenhanced CT Images? Radiology 2015;276:787-96. [Crossref] [PubMed]
- Chen Y, Chen TW, Wu CQ, Lin Q, Hu R, Xie CL, Zuo HD, Wu JL, Mu QW, Fu QS, Yang GQ, Zhang XM. Radiomics model of contrast-enhanced computed tomography for predicting the recurrence of acute pancreatitis. Eur Radiol 2019;29:4408-17. [Crossref] [PubMed]
- Shafiq-Ul-Hassan M, Zhang GG, Latifi K, Ullah G, Hunt DC, Balagurunathan Y, Abdalah MA, Schabath MB, Goldgof DG, Mackin D, Court LE, Gillies RJ, Moros EG. Intrinsic dependencies of CT radiomic features on voxel size and number of gray levels. Med Phys 2017;44:1050-62. [Crossref] [PubMed]
- Andrianifahanana M, Moniaux N, Schmied BM, Ringel J, Friess H, Hollingsworth MA, Büchler MW, Aubert JP, Batra SK. Mucin (MUC) gene expression in human pancreatic adenocarcinoma and chronic pancreatitis: a potential role of MUC4 as a tumor marker of diagnostic significance. Clin Cancer Res 2001;7:4033-40. [PubMed]
- Wang Y, Wan Q, Xia X, Hu J, Liao Y, Wang P, Peng Y, Liu H, Li X. Value of radiomics model based on multi-parametric magnetic resonance imaging in predicting epidermal growth factor receptor mutation status in patients with lung adenocarcinoma. J Thorac Dis 2021;13:3497-508. [Crossref] [PubMed]
- Permuth JB, Choi J, Balarunathan Y, Kim J, Chen DT, Chen L, et al. Combining radiomic features with a miRNA classifier may improve prediction of malignant pathology for pancreatic intraductal papillary mucinous neoplasms. Oncotarget 2016;7:85785-97. [Crossref] [PubMed]
- Wang H, Hu D, Yao H, Chen M, Li S, Chen H, Luo J, Feng Y, Guo Y. Radiomics analysis of multiparametric MRI for the preoperative evaluation of pathological grade in bladder cancer tumors. Eur Radiol 2019;29:6182-90. [Crossref] [PubMed]
- Chun YS, Pawlik TM, Vauthey JN. 8th Edition of the AJCC Cancer Staging Manual: Pancreas and Hepatobiliary Cancers. Ann Surg Oncol 2018;25:845-7.
- Huang X, Wang X, Lu SM, Chen C, Wang J, Zheng YY, Ren BH, Xu L. Clinicopathological and prognostic significance of MUC4 expression in cancers: evidence from meta-analysis. Int J Clin Exp Med 2015;8:10274-83. [PubMed]
- Huebner M, Kendrick M, Reid-Lombardo KM, Que F, Therneau T, Qin R, Donohue J, Nagorney D, Farnell M, Sarr M. Number of lymph nodes evaluated: prognostic value in pancreatic adenocarcinoma. J Gastrointest Surg 2012;16:920-6. [Crossref] [PubMed]
- Slidell MB, Chang DC, Cameron JL, Wolfgang C, Herman JM, Schulick RD, Choti MA, Pawlik TM. Impact of total lymph node count and lymph node ratio on staging and survival after pancreatectomy for pancreatic adenocarcinoma: a large, population-based analysis. Ann Surg Oncol 2008;15:165-74. [Crossref] [PubMed]
- Kocak B, Durmaz ES, Erdim C, Ates E, Kaya OK, Kilickesmez O. Radiomics of Renal Masses: Systematic Review of Reproducibility and Validation Strategies. AJR Am J Roentgenol 2020;214:129-36. [Crossref] [PubMed]
- Lu DS, Vedantham S, Krasny RM, Kadell B, Berger WL, Reber HA. Two-phase helical CT for pancreatic tumors: pancreatic versus hepatic phase enhancement of tumor, pancreas, and vascular structures. Radiology 1996;199:697-701. [Crossref] [PubMed]