Performance variability of radiomics machine learning models for the detection of clinically significant prostate cancer in heterogeneous MRI datasets
Introduction
Prostate cancer (PCa) currently is the second most common malignancy among men worldwide (1). Despite its high prevalence, PCa related mortality is low with a five-year survival rate of around 98% for all PCa stages combined (2). Considering the high PCa prevalence and low mortality rate, accurate differentiation of clinically significant PCa (csPCa) from clinically insignificant PCa (cisPCa) is of high importance to decrease overdiagnosis and overtreatment. Large prospective trials such as the PRECISION-Trial and PROMIS-Trial have concluded that the use of multiparametric MRI (mpMRI) prior to biopsy increases detection of csPCa while decreasing detection of cisPCa compared to transrectal ultrasound guided biopsy (3-5). Whereas mpMRI has been included in the guidelines of the European Association of Urology (EAU) to be performed prior to biopsy (6), also biparametric MRI (bpMRI) without use of a contrast agent was shown to accurately detect and localize PCa (7,8). In order to improve image acquisition and reporting standards, the Prostate Imaging Reporting and Data System (PI-RADS) was introduced in the clinical diagnostic workup of PCa (9-11).
Over the last few years, machine-learning techniques have increasingly been used to evaluate imaging-based biomarkers to support PCa detection as well as personalized therapeutic decision-making. Radiomics has emerged as a promising tool to enhance information attainable from imaging by means of automated high-throughput data extractions and analysis combined with machine learning or deep learning techniques (12,13). Initial studies have reported promising results for radiomics-based characterization of suspicious prostate lesions and significant performance increase in cancer detection in combination with retrospective PI-RADS assessment (14,15). However, increasing evidence suggests that radiomics models’ performance might not be robust and might strongly depend on the underlying data characteristics. Bonekamp et al. find in their analysis no advantage of using radiomics models over classification by mean apparent diffusion coefficient (ADC), while Spohn et al. identify variability of features as potential reason for lack of reproducibility of high classification performances and Twilt et al. describe a gap “between academic results and clinical practice (12,13,16). Furthermore, comparison of radiomics performance with clinical performance on the same dataset is often missing and there is still lack of information on the clinical impact and utility of radiomics models within the clinical diagnostic workup (12,13,17).
This study analyzes a heterogeneous prostate MRI dataset in order to differentiate csPCa with a Gleason score of ≥7 from cisPCa (Gleason score ≤6) and benign lesions (non-csPCa) using imaging-based biomarkers such as PI-RADS, mean ADC (mADC) and prostate-specific antigen density (PSAD) and radiomics-based state-of-the-art machine learning models for the classification of suspicious prostate lesions (PI-RADS score ≥3). We present the following study in accordance with the TRIPOD reporting checklist (available at https://qims.amegroups.com/article/view/10.21037/qims-22-265/rc).
Methods
Study design
The study was conducted in accordance with the Declaration of Helsinki (as revised in 2013). The study was approved by the institutional ethics committee (Ethikkommission bei der LMU) and individual consent for this retrospective analysis was waived. From January 2012 to November 2018, a total of 467 patients consecutively registered with the suspicion for PCa at our hospital with available bpMRI were considered for this study. A total of 189 patients received histopathological correlation within six month and prostate-specific antigen (PSA) within four weeks of the MRI scans. Only patients with suspicious prostate lesions (PI-RADS score ≥3) with complete and accessible MRI datasets [including T1-weighted (T1w), T2-weighted (T2w) and diffusion-weighted imaging (DWI)/ADC images] were included in the study (n=142) (Figure 1). For each patient, clinical parameters were documented including presence of PCa, Gleason score of confirmation biopsy (n=56) or after radical prostatectomy (n=86), TNM classification, Union for International Cancer Control (UICC) stages, PI-RADS scores, PSA level and volume-based PSAD.
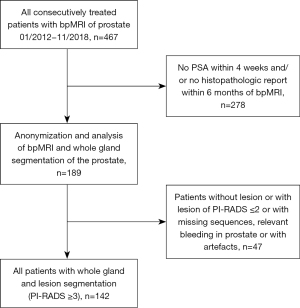
Imaging characteristics and image segmentation
Most of bpMRI scans were obtained at our hospital (n=115) using a 3-Tesla scanner (Magnetom Skyra, Siemens, Germany), whereas some patients had undergone MRI scans externally (n=27) using different 1.5- or 3-Tesla scanners (in total 7 scanner types, 14 institutions). No pelvic coil was used and the bpMRI protocol included T1w, T2w and DWI sequences. ADC maps were extracted from DWI sequences. The acquisition parameters for T2w sequences ranged in echo time from 87 to 182 ms, in repetition time from 1,500 to 8,740 ms, whereas resolutions in x and y direction were between 0.31 and 0.78 mm and slice thickness between 1.0–3.5 mm. Acquisition parameter ranges for DWI sequences used for ADC calculation ranged from 48 ms to 80 ms in echo time, from 1,943 to 5,716 ms in repetition time, between 0.77 and 2.13 mm for resolutions in x and y direction and between 3.0–5.0 in slice thickness.
All MRI scans were reviewed independently and blinded to the clinical data and were screened for suspicious prostate lesions and segmented by two radiologists with >6 years and >4 years of experience in prostate imaging (blinded). To reduce inter-reader associated differences in segmentation size, all segmentations were controlled and adjusted by a reader with >7 years of experience in urogenital imaging (blinded). Structured reporting included PI-RADS v2.1 score for every lesion. The lesion with the highest PI-RADS score in the peripheral or transitional zone was defined as the target lesion for each patient and included for radiomics analysis. If more than one lesion among the highest PI-RADS score was present, the lesion with the lowest ADC value was selected as the region of interest for subsequent analysis. Whole organ segmentations for calculation of PSA density and separate single lesion segmentations as the regions of interest for feature extraction were performed semi-automatically on T2w- and DWI transversal images using the post-processing software MITK (Medical Imaging Interaction Toolkit) (Figure 2).
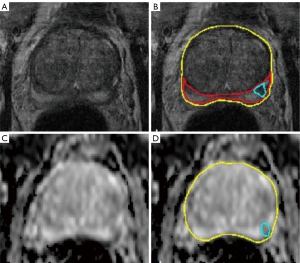
Radiomics feature extraction and selection
All radiomic features were calculated using the PyRadiomics package (version 3.0.1) (18). Image features can be distinguished in three classes: (I) shape features, (II) first order (distribution) features; and (III) texture features. First, the shape of the segmentation was used to determine measurements such as volume and surface, but also more sophisticated variables such as compactness and sphericity. The second category of features was derived from the distribution of intensities in the region of interest. These features include general measures of the distribution, such as the mean, median, interquartile range of the distribution, but also descriptors of shape, such as skewness and information-theoretical measures such as entropy. Third, texture features were derived from discretized gray values in the region of interest. Different matrices were defined to characterize patterns in the discretized gray values, such as gray-level size-zone matrix (GLSZM), gray-level run-length matrix (GLRLM), gray-level co-occurrence matrix (GLCM). Two more matrices analyzed the local neighborhood of pixels [neighboring gray-tone difference matrix (NGTDM) and gray-level dependence matrix (GLDM)]. In order to mitigate the noise inherent to any MR measurement and to extract even more features in addition to the clinically used T2w and ADC images, filters were applied to these images. Wavelet and Laplacian over Gaussian (LoG) were used to sharpen or smooth the images, while the local binary pattern (LBP) filter was used as a special algorithm extracting information on local neighborhoods of pixels. Before calculating the features, T2-weighted images were normalized to a scale of 100 and shifted to a mean of 300 to allow for a correct and equal calculation of features. ADC images were not normalized since ADC is a quantitative measurement. Both sequences were resampled to their respective most common resolution: The T2-weighted images were resampled to 0.55 mm in xy- and 3 mm in z-direction, while the ADC images were resampled to 1.25 mm in xy- and 3 mm in z-direction. The discretization for the features based on pattern analysis was adapted to the filters: On the T2-weighted images a fixed bin width of 10 was used for all filters, except for the LBPs, where a bin width of 1 was used. On ADC images the bin width for original and wavelet transforms was 25, while LoG used 10 and LBP used 1 as in the case of T2w images. In total, 741 radiomic features were extracted for every patient using T2w and ADC images in the following three categories: (I) shape features (n=13), (II) first order features (n=18×8 filters =144) and (III) texture features (n=73×8 filters =584).
Feature selection: minimal-redundancy-maximal-relevance (mRMR)
Since many features are expected to be correlated e.g., due to using several filters on the same image, feature-selection algorithms were considered to reduce the number of features used for training. As a baseline feature selection algorithm, the mRMR criterion was used (19). This criterion simultaneously retains the feature with highest mutual information with respect to the target class (relevant features) and removes correlated (redundant) features. mRMR provides a ranking of features and the number of features selected is a hyperparameter of the classification pipeline. As baseline configuration, a selection of the first 25 features of the ranking was chosen. In order to assess the impact of hyperparameter changes on the classification performance, selections of 50 and 100 features and no feature selection at all were tested. Thereby, the intrinsic robustness of the classifier with respect to correlated and uninformative features was evaluated.
Class imbalance: class weights, synthetic minority oversampling technique (SMOTE)
To cope with the class imbalance of the training sample (unequal incidence of patients with significant (n=93) and insignificant or no prostate carcinoma (n=47) within the study cohort), the following mitigation strategies were considered to avoid a classification bias towards the majority class. A simple class-weight-based approach was chosen as the baseline. To each sample, a weight of the inverse of the frequency of its class was assigned. These weights were taken into account at the calculation of the loss function and increased the importance of minority class examples. As an alternative approach following Fehr et al. (14), the SMOTE was used to artificially add synthetic samples to the minority class (20). The minority class was increased to match the number of training samples of the majority class.
Classifiers: logistic regression, random forest algorithms
Two machine learning approaches were used for classification: logistic regression and random forests. For the logistic regression analysis, the data was preprocessed by scaling each feature to its standard deviation and removing the mean. The logistic regression loss was penalized with elastic-net regularization. On each fold, a cross-validated grid search was run to determine the hyperparameters for the regularization. The main approach used random forests for classification (21). For the baseline classification, the hyperparameters were set to their default values as provided by scikit-learn, except for the number of estimators, which was set to 5,000. The feature selection mRMR with 25 features was chosen as the baseline configuration. The feature selection was varied to zero, 50 and 100 features testing the assumption that random forests should be robust to correlated and uninformative features. Class weights were used as the baseline class-imbalance mitigation strategy with the variations of using no mitigation strategy as well as the SMOTE method.
Model configurations
Using different combinations of the above-described methods, seven models were trained for the correct discrimination between csPCa (Gleason score ≥7) and non-csPCa (Gleason score ≤6), one logistic regression approach and six different random-forest algorithms: logistic regression, class weights mRMR [25], class weights mRMR [50], class weights mRMR [100], no weights mRMR [25], class weights only and smote mRMR [25]. The RF model configurations and their respective shorthands can be found in Table 1, a general overview of the applied radiomics workflow and the model selection process in Figure 3.
Table 1
Shorthand | Feature selection method | Imbalance mitigation method |
---|---|---|
cw | mRMR(25) | mRMR with 25 features | RF class weights |
cw | mRMR(50) | mRMR with 50 features | RF class weights |
cw | mRMR(100) | mRMR with 100 features | RF class weights |
no cw | mRMR(25) | mRMR with 25 features | No mitigation method |
mRMR(25) | SMOTE | mRMR with 25 features | SMOTE |
cw | no FS | No feature selection | RF class weights |
“cw | mRMR(25)” is used as baseline setting, all other configurations are variations thereof. cw, class weights; mRMR, minimal redundancy maximal relevance; RF, random forest; SMOTE, synthetic minority oversampling technique; FS, feature selection.
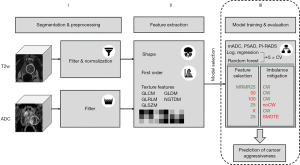
Statistical analysis and machine learning
All statistical analyses were performed in R and Python 3.7. To evaluate the discriminative performance to differentiate csPCa from non-csPCa lesions, for each of the radiomics classifiers as well as for the PI-RADS score, the mADC and the PSAD, a receiver operating curve (ROC) was determined and the area under the curve (AUC) calculated. As single-center studies often are limited by relatively small sample sizes (13), we assessed the performance of the radiomics analyses using cross-validation methods. The dataset was randomly divided into five folds—stratified by the outcome labels csPCa and non-csPCa. Training, including feature selection and imbalance mitigation, was repeated five times, such that each fold was used exactly once for testing and the respective four remaining folds for training the classifier. For each model configuration, the mean AUC over the five test folds was calculated. For the assessment of sensitivity and specificity, the working point of trained classifiers was determined by the Youden’s index.
To investigate the significance in the different model configurations a mixed-model approach was used. The setup of the random effects of the mixed model followed Eugster et al. (22), modeling the variation of the resampling of each cross-validation step as a random effect. The model configurations described above were modeled as fixed effects and the configuration “class weights mRMR(100)”—with the best mean AUC—was set as reference configuration. This allowed us to calculate P values based on the slope parameters for the fixed effects of the mixed model. If the slope parameters differed significantly from zero (using a significance level of 0.05), we could conclude that in case of a positive (negative) slope a significantly better (worse) model configuration was found.
Results
Patient and lesion characteristics
The detailed clinical patient characteristics of the study cohort are shown in Tables 2-4. Median age of patients in the study cohort was 68 [66.5–69.5] years. In 13% of patients, no PCa was detected histologically. Of the 87% patients positive for PCa, 76% exhibited csPCa (GS ≥7) and 24% cisPCa (GS =6). Whereas 14% of lesions were localized in the transitional zone, 86% of index lesions were found in the peripheral zone. The maximum diameter of the lesions ranged from 7.2 up to 64 mm with a mean maximum diameter of 25 mm.
Table 2
Patient characteristics | Study cohort (n=142) |
---|---|
Age (years) | 68 [66.5–69.5] |
PCa-negative | 18 (13%) |
PCa-positive | 124 (87%) |
Biopsy | 56 (39%) |
MRI/TRUS fusion targeted biopsy | 29 (52%) |
Ultrasound-guided punch biopsy | 27 (48%) |
Prostatectomy | 86 (61%) |
Median prostate volume (mL) | 41.5 [29.3–60.0] |
Median lesion volume (mL) | 1.1 [0.44–2.8] |
Median PSA value (ng/mL) | 7.6 [2.4–12.7] |
Median PSA density (ng/mL2) | 8.0 [3.7–17.3] |
Median mADC prostate (mm2/s) | 1,197 [1,129–1,76] |
Median mADC lesion (mm2/s) | 839 [769–979] |
Variables are shown in median [interquartile ranges] or in count (percentage); PCa, prostate cancer; TRUS, transrectal ultrasonography; PSA, prostate specific antigen; mADC, mean apparent diffusion coefficient.
Table 3
MRI index lesion evaluation | Study cohort (n=142) |
---|---|
PI-RADS 3 | 44 (31%) |
PI-RADS 4 | 28 (20%) |
PI-RADS 5 | 70 (49%) |
Index lesion in PZ | 122 (86%) |
Index lesion in TZ | 20 (14%) |
PI-RADS, Prostate Imaging Reporting and Data System; PZ, peripheral zone; TZ, transitional zone.
Table 4
Grading of PCa | PCa-positive cohort (n=124) |
---|---|
ISUP grade 1 (GS =6) = cisPCa | 30 (24%) |
ISUP grade ≥2 (GS ≥7) = csPCa | 94 (76%) |
ISUP grade 2 (GS =3+4) | 37 (39%) |
ISUP grade 3 (GS =4+3) | 25 (27%) |
ISUP grade 4 (GS =8) | 12 (13%) |
ISUP grade 5 (GS =9–10) | 20 (21%) |
PCa, prostate cancer; cisPCa, clinically insignificant PCa; csPCa, clinically significant PCa; ISUP, International Society of Urological Pathology; GS, Gleason score.
Prediction of cancer significance using PI-RADS, PSAD and mADC values
Classification performance of csPCa vs. non-csPCa was evaluated on the whole dataset for the three univariate attributes PI-RADS, PSAD and mADC. The performance of PI-RADS was found to achieve an AUC of 0.78 with a maximum Youden’s index of 0.53 (at sensitivity of 0.82 and specificity of 0.71) at the threshold of PI-RADS of at least 4. PSAD scored an AUC of 0.63 with maximum Youden’s index of 0.35 (at sensitivity of 0.72 and specificity of 0.54) at a threshold of PSAD of at least 12.4 ng/mL2. mADC achieved an AUC of 0.71 with maximum Youden’s index of 0.49 (sensitivity of 0.84 and specificity of 0.63) at a threshold of 930 mm2/s. Figure 4 shows the ROC curves for the three discriminators, Figure 5 the AUC in comparison to the other classifiers.
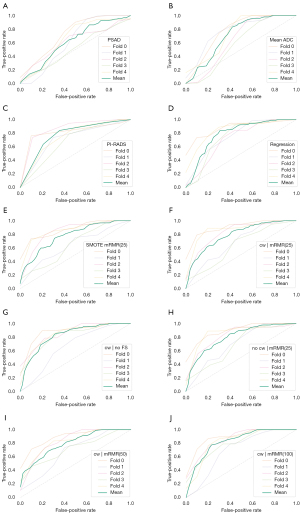
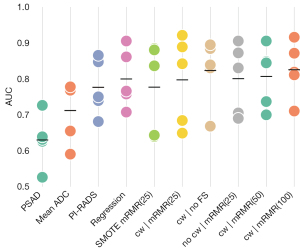
Assessment of cancer significance using radiomics models
Cross-validated training and evaluation of the logistic regression and six random forest classifiers yielded mean AUCs from 0.78 to 0.83 with single fold AUCs in the range of 0.64 and 0.92, with differences between best and worst fold of on average 0.2. Figure 4 shows the ROC curves for each fold of the classifiers individually and the mean ROC curve averaged over all 5 folds of each classifier, precision-recall curves are added to Figure S1. The ROC curves of different folds exhibited significant variation. The variance of the ROC curves was aggregated in the summary plot Figure 5, showing the AUC of each fold and the respective mean AUC of each classifier. Mean AUCs for the cross-validated classifiers ranged from 0.78 to 0.83 (Table 5). Mean Youden’s index ranged from 0.54 to 0.62 (Table S1).
Table 5
PSA density | Mean ADC | PI-RADS | Regression | SMOTE mRMR(25) | cw | mRMR(25) | cw | no FS | no cw | mRMR(25) | cw | mRMR(50) | cw | mRMR(100) | |
---|---|---|---|---|---|---|---|---|---|---|
AUC (mean) | 0.63 | 0.71 | 0.78 | 0.80 | 0.78 | 0.80 | 0.82 | 0.80 | 0.81 | 0.83 |
AUC [1] | 0.73 | 0.77 | 0.85 | 0.91 | 0.88 | 0.92 | 0.88 | 0.91 | 0.91 | 0.92 |
AUC [2] | 0.63 | 0.77 | 0.74 | 0.76 | 0.64 | 0.68 | 0.67 | 0.71 | 0.70 | 0.71 |
AUC [3] | 0.63 | 0.65 | 0.87 | 0.71 | 0.84 | 0.84 | 0.89 | 0.83 | 0.85 | 0.87 |
AUC [4] | 0.53 | 0.59 | 0.68 | 0.77 | 0.64 | 0.65 | 0.83 | 0.69 | 0.74 | 0.82 |
AUC [5] | 0.64 | 0.78 | 0.75 | 0.86 | 0.88 | 0.89 | 0.84 | 0.87 | 0.84 | 0.81 |
P values | <0.001 | 0.003 | 0.185 | 0.478 | 0.186 | 0.436 | 0.953 | 0.496 | 0.610 | 1 |
For each score or configuration, the mean AUC and the result for each CV fold is shown. The bottom row shows the P values yielded by the mixed-model analysis, showing how significantly different the classification performance of each of the model configurations in comparison to the best-performing “cw | mRMR(100)” was. Model configuration shorthands according to Table 1. AUC, area under the curve; PSA, prostate specific antigen; ADC, apparent diffusion coefficient; PI-RADS, Prostate Imaging Reporting and Data System; SMOTE, synthetic minority oversampling technique; mRMR, minimal redundancy maximal relevance; cw, class weights.
Comparison of the classification models
The classification performance of the trained models to correctly distinguish between csPCa and non-csPCa was on average higher than with PI-RADS, mADC or PSAD alone (Figure 5). On average, highest AUC was found for the random forest models using class weights to balance group sizes and using 50 and 100 features selected with mRMR. However, robustness of results was low with high variations of AUC between the five cross-validation performance estimates. In particular, the variation of AUCs for a single classifier over the five cross validation (CV) folds is higher than the variation of the means of the classifiers compared to each other.
The analysis of the mixed model showed that none of the RF models had a significant change in performance when considering the model configuration as fixed effect and the CV fold as random effect. The lowest P value was obtained for model configuration using SMOTE as imbalance mitigation technique with a negative slope of fixed effects indicating worse performance, but at a P value of P=0.19 not significant. PI-RADS also showed a trend for worse performance, but at a P value of P=0.19 also not significant. The only significantly worse classification approaches were mADC and PSAD at P values of P=0.003 and P<0.001, respectively. Table 5 shows a summary of the AUC values of all model configurations and folds with their respective P values from the mixed-model analysis.
Discussion
This study investigated whether radiomics-based imaging biomarkers can reliably detect csPCa on a consecutively registered heterogeneous prostate MRI dataset of suspicious prostate lesions (PI-RADS ≥3). We applied state-of-the-art machine learning techniques and systematically trained models using different configurations of methods and hyperparameters, evaluated the models using CV and transparently reported results of all experiments. Radiomics results were compared to the discrimination performance of the clinical scoring parameters PI-RADS, mADC and PSAD. Our trained models yielded a discrimination performance of mean AUCs ranging from 0.78 to 0.83, where the worst-performing fold yielded an AUC of 0.62 and the best performing fold an AUC of 0.95. Radiomics random forest models achieved on average better classification performance than clinical parameters such as PI-RADS (AUC =0.78), mADC (AUC =0.71) and PSAD (AUC =0.63), but did not fully outperform them due to the high variability of the results within the models’ testfolds.
We hypothesize that an important factor for the high variability of performance derives from the heterogeneity of our dataset. We included all consecutively registered patients at the urology department of our hospital which reflects a realistic approach to future usage of radiomics models in clinical workup. The underlying MRI datasets were partially acquired at different institutions using a number of scanner types and protocols, with various T2-sequences and DWI-sequences with several combinations of b-values for ADC calculation were used. This impacts the intensities of the resulting images and consequently changes the derived radiomics features (23-25). Furthermore, we included lesions from peripheral as well as transitional zones which differ due to their distinct anatomical embeddings and their specific tissue structures, which again might change which radiomics features carry discriminative potential (26,27). Whereas the folds were stratified by tumor aggressiveness (csPCa versus non-csPCa), the above-mentioned heterogeneities could not be taken into account when dividing folds, which—considering the small sample size—inevitably led to statistical fluctuations and therefore uneven distribution of heterogeneities over the folds.
In order to optimize classification performance of the models, we investigated hyperparameter settings and used variations of imbalance mitigation techniques on the baseline model in order to investigate the influence of a method within the given setting. In comparison to PI-RADS, all RF-based model configurations scored better in their mean classification performance, while the regression analysis did not add value and even performed worse on average. This is within expectations, since the RF can model more complex relations and interactions of features than a logistic regression. All machine-learning-based models showed a high variability of results over the five folds of cross-validation, which demonstrates that the choice of samples for evaluation has a substantial impact. The models trained in this study were able to achieve high performances of up to an AUC of 0.92 by picking a single test fold, but at the same time showing differences of up to 0.20 within the results of the five folds of the same model. Therefore, the classification performance on each CV fold needs to be reported to show the variability and thereby assess robustness of radiomics models. Moreover, the results of our study indicate that PSAD and mADC have limited discrimination potential and were outperformed by the trained models. The PI-RADS score however, which is routinely used in clinical work-flow, proved to be a good discriminator for csPCa for suspicious prostate lesions. Although mean performance of the radiomics models trained was higher than the PI-RADS score, all models also had folds which performed worse.
The differentiation potential of PCa aggressiveness using radiomics has been intensely discussed in the literature and results published in earlier studies vary substantially (12,13). Whereas some studies have reported remarkably promising radiomics performances to determine csPCa (14,15,28-32), others did not find substantial stratification potential or conclusive results of radiomics to outperform clinical scoring systems (16,33). It is difficult to fully compare our models with earlier research due to different study designs and underlying methods. The underlying patient population differed depending on the hypothesis investigated and endpoints formulated, whereas most concentrated on PCa detection and differentiation of csPCa (12). Previous studies evaluated radiomics-based analyses on homogeneously acquired datasets with respect to scanner type and protocol (14-16,28-34). While the homogeneity might enhance radiomics models’ performance, it fails to address the real-world scenario. When applied to different (external) datasets, model performance decreased in several studies (34-36), suggesting that single-center trained radiomics-based bpMRI models do not seem to sufficiently generalize to multi-center data sets (36), The minority of studies included suspicious lesions from both peripheral and transitional zones (12), limiting the clinical applicability of these models. In addition, established methods that have been shown to increase radiomics performance on homogeneous datasets such as SMOTE (14,37) did not prove valid for our models, confirming that the added value of methods can depend on the underlying dataset. Reporting checklists as well as evaluation criteria for radiomics studies have been proposed to increase transparency in publishing radiomics studies (38-40). Our study, however, also argues for a more transparent handling of variability of radiomic models results and for reporting of all findings to prevent overly optimistic or pessimistic results. This especially refers to the results from all folds and all configurations, which could be reported explicitly or using appropriate summary metrics.
Accurate differentiation of suspicious lesions on prostate MRI (PIRADS ≥3) for the differentiation of csPCa versus cisPCa or benign lesions is of high importance to decrease overdiagnosis and overtreatment. In order to enhance the diagnostic workup based on prostate MRI, radiomics has become a highly active field offering non-invasive imaging biomarkers for an objective characterization of the imaging data. However, its application introduces some major challenges which have to be carefully addressed. Current radiomics models lack generalizability and reproducibility. Transparent reporting of all investigated model configurations and critical evaluation of the variability of results is a prerequisite for reliable clinical application of radiomics.
Limitations
Our study has several limitations. Firstly, it consists of a relatively small sample size (n=142), which however lies within the average of earlier presented studies (12,13). Our MRI dataset did not follow a standardized imaging protocol as we included datasets from multiple institutions, it was however intended for the assessment of the generalizability of the models. An external validation cohort was not available, but strict separation of training and test data was achieved by means of CV. As only a limited number of configurations and preprocessing approaches could be tested, we chose a set of state-of-the-art machine learning methods that have been utilized in earlier radiomics studies.
Another limitation can be seen in the fact that some patients underwent radical prostatectomy, while the others underwent MRI-guided prostate biopsy. In principle the MRI-guided biopsy might miss the cancer hotspot within the targeted lesion, earlier studies have however found reliable correlation of index lesions on MRI with their histopathologic result (41).
Conclusions
In this study, we showed that the clinical applicability of radiomics models on suspicious prostate lesions (PI-RADS ≥3) in a heterogeneous, real-world dataset is limited due to low robustness and high variations of results. We presented an approach to critically evaluate and transparently report the variability of classification performance on all CV folds across a number of model configurations. In our study, the trained models did not reliably improve lesion discrimination compared to conventional imaging biomarkers. Performance variability, robustness and reproducibility of radiomics-based measures should be addressed more transparently in future research to enable broad clinical application. Further investigations on reducing variability and enhancing robustness are required to facilitate clinical application.
Acknowledgments
Funding: None.
Footnote
Reporting Checklist: The authors have completed the TRIPOD reporting checklist. Available at https://qims.amegroups.com/article/view/10.21037/qims-22-265/rc
Conflicts of Interest: All authors have completed the ICMJE uniform disclosure form (available at https://qims.amegroups.com/article/view/10.21037/qims-22-265/coif). DN and TH are medical consultants for Smart Reporting GmbH, which is not related to the current study. The other authors have no conflicts of interest to declare.
Ethical Statement:
Open Access Statement: This is an Open Access article distributed in accordance with the Creative Commons Attribution-NonCommercial-NoDerivs 4.0 International License (CC BY-NC-ND 4.0), which permits the non-commercial replication and distribution of the article with the strict proviso that no changes or edits are made and the original work is properly cited (including links to both the formal publication through the relevant DOI and the license). See: https://creativecommons.org/licenses/by-nc-nd/4.0/.
References
- Sung H, Ferlay J, Siegel RL, Laversanne M, Soerjomataram I, Jemal A, Bray F. Global Cancer Statistics 2020: GLOBOCAN Estimates of Incidence and Mortality Worldwide for 36 Cancers in 185 Countries. CA Cancer J Clin 2021;71:209-49. [Crossref] [PubMed]
- Siegel RL, Miller KD, Jemal A. Cancer statistics, 2020. CA Cancer J Clin 2020;70:7-30. [Crossref] [PubMed]
- Kasivisvanathan V, Rannikko AS, Borghi M, Panebianco V, Mynderse LA, Vaarala MH, et al. MRI-Targeted or Standard Biopsy for Prostate-Cancer Diagnosis. N Engl J Med 2018;378:1767-77. [Crossref] [PubMed]
- Drost FH, Osses DF, Nieboer D, Steyerberg EW, Bangma CH, Roobol MJ, Schoots IG. Prostate MRI, with or without MRI-targeted biopsy, and systematic biopsy for detecting prostate cancer. Cochrane Database Syst Rev 2019;4:CD012663. [Crossref] [PubMed]
- Rouvière O, Puech P, Renard-Penna R, Claudon M, Roy C, Mège-Lechevallier F, Decaussin-Petrucci M, Dubreuil-Chambardel M, Magaud L, Remontet L, Ruffion A, Colombel M, Crouzet S, Schott AM, Lemaitre L, Rabilloud M, Grenier N. MRI-FIRST Investigators. Use of prostate systematic and targeted biopsy on the basis of multiparametric MRI in biopsy-naive patients (MRI-FIRST): a prospective, multicentre, paired diagnostic study. Lancet Oncol 2019;20:100-9. [Crossref] [PubMed]
- Mottet N, Bellmunt J, Bolla M, Briers E, Cumberbatch MG, De Santis M, et al. EAU-ESTRO-SIOG Guidelines on Prostate Cancer. Part 1: Screening, Diagnosis, and Local Treatment with Curative Intent. Eur Urol 2017;71:618-29. [Crossref] [PubMed]
- Scialpi M, D'Andrea A, Martorana E, Malaspina CM, Aisa MC, Napoletano M, Orlandi E, Rondoni V, Scialpi P, Pacchiarini D, Palladino D, Dragone M, Di Renzo G, Simeone A, Bianchi G, Brunese L. Biparametric MRI of the prostate. Turk J Urol 2017;43:401-9. [Crossref] [PubMed]
- Xu L, Zhang G, Shi B, Liu Y, Zou T, Yan W, Xiao Y, Xue H, Feng F, Lei J, Jin Z, Sun H. Comparison of biparametric and multiparametric MRI in the diagnosis of prostate cancer. Cancer Imaging 2019;19:90. [Crossref] [PubMed]
- Weinreb JC, Barentsz JO, Choyke PL, Cornud F, Haider MA, Macura KJ, Margolis D, Schnall MD, Shtern F, Tempany CM, Thoeny HC, Verma S. PI-RADS Prostate Imaging - Reporting and Data System: 2015, Version 2. Eur Urol 2016;69:16-40. [Crossref] [PubMed]
- Turkbey B, Rosenkrantz AB, Haider MA, Padhani AR, Villeirs G, Macura KJ, Tempany CM, Choyke PL, Cornud F, Margolis DJ, Thoeny HC, Verma S, Barentsz J, Weinreb JC. Prostate Imaging Reporting and Data System Version 2.1: 2019 Update of Prostate Imaging Reporting and Data System Version 2. Eur Urol 2019;76:340-51. [Crossref] [PubMed]
- Park KJ, Choi SH, Lee JS, Kim JK, Kim MH. Interreader Agreement with Prostate Imaging Reporting and Data System Version 2 for Prostate Cancer Detection: A Systematic Review and Meta-Analysis. J Urol 2020;204:661-70. [Crossref] [PubMed]
- Spohn SKB, Bettermann AS, Bamberg F, Benndorf M, Mix M, Nicolay NH, Fechter T, Hölscher T, Grosu R, Chiti A, Grosu AL, Zamboglou C. Radiomics in prostate cancer imaging for a personalized treatment approach - current aspects of methodology and a systematic review on validated studies. Theranostics 2021;11:8027-42. [Crossref] [PubMed]
- Twilt JJ, van Leeuwen KG, Huisman HJ, Fütterer JJ, de Rooij M. Artificial Intelligence Based Algorithms for Prostate Cancer Classification and Detection on Magnetic Resonance Imaging: A Narrative Review. Diagnostics (Basel) 2021;11:959. [Crossref] [PubMed]
- Fehr D, Veeraraghavan H, Wibmer A, Gondo T, Matsumoto K, Vargas HA, Sala E, Hricak H, Deasy JO. Automatic classification of prostate cancer Gleason scores from multiparametric magnetic resonance images. Proc Natl Acad Sci U S A 2015;112:E6265-73. [Crossref] [PubMed]
- Wang J, Wu CJ, Bao ML, Zhang J, Wang XN, Zhang YD. Machine learning-based analysis of MR radiomics can help to improve the diagnostic performance of PI-RADS v2 in clinically relevant prostate cancer. Eur Radiol 2017;27:4082-90. [Crossref] [PubMed]
- Bonekamp D, Kohl S, Wiesenfarth M, Schelb P, Radtke JP, Götz M, Kickingereder P, Yaqubi K, Hitthaler B, Gählert N, Kuder TA, Deister F, Freitag M, Hohenfellner M, Hadaschik BA, Schlemmer HP, Maier-Hein KH. Radiomic Machine Learning for Characterization of Prostate Lesions with MRI: Comparison to ADC Values. Radiology 2018;289:128-37. [Crossref] [PubMed]
- T JMC. Arif M, Niessen WJ, Schoots IG, Veenland JF. Automated Classification of Significant Prostate Cancer on MRI: A Systematic Review on the Performance of Machine Learning Applications. Cancers (Basel) 2020;12:1606. [Crossref]
- van Griethuysen JJM, Fedorov A, Parmar C, Hosny A, Aucoin N, Narayan V, Beets-Tan RGH, Fillion-Robin JC, Pieper S, Aerts HJWL. Computational Radiomics System to Decode the Radiographic Phenotype. Cancer Res 2017;77:e104-7. [Crossref] [PubMed]
- Peng H, Long F, Ding C. Feature selection based on mutual information: criteria of max-dependency, max-relevance, and min-redundancy. IEEE Trans Pattern Anal Mach Intell 2005;27:1226-38. [Crossref] [PubMed]
- Chawla NV, Bowyer KW, Hall LO, Kegelmeyer WP. SMOTE: Synthetic Minority Over-sampling Technique. J Artif Intell Res 2002;16:321-57. [Crossref]
- Breiman L. Random Forests. Mach Learn 2001;45:5-32. [Crossref]
- Eugster MJA, Hothorn T, Leisch F. Exploratory and Inferential Analysis of Benchmark Experiments. Department of Statistics, University of Munich; Technical Report Number 030, 2008.
- Peerlings J, Woodruff HC, Winfield JM, Ibrahim A, Van Beers BE, Heerschap A, Jackson A, Wildberger JE, Mottaghy FM, DeSouza NM, Lambin P. Stability of radiomics features in apparent diffusion coefficient maps from a multi-centre test-retest trial. Sci Rep 2019;9:4800. [Crossref] [PubMed]
- Yip SS, Aerts HJ. Applications and limitations of radiomics. Phys Med Biol 2016;61:R150-66. [Crossref] [PubMed]
- Schwier M, van Griethuysen J, Vangel MG, Pieper S, Peled S, Tempany C, Aerts HJWL, Kikinis R, Fennessy FM, Fedorov A. Repeatability of Multiparametric Prostate MRI Radiomics Features. Sci Rep 2019;9:9441. [Crossref] [PubMed]
- Ginsburg SB, Algohary A, Pahwa S, Gulani V, Ponsky L, Aronen HJ, Boström PJ, Böhm M, Haynes AM, Brenner P, Delprado W, Thompson J, Pulbrock M, Taimen P, Villani R, Stricker P, Rastinehad AR, Jambor I, Madabhushi A. Radiomic features for prostate cancer detection on MRI differ between the transition and peripheral zones: Preliminary findings from a multi-institutional study. J Magn Reson Imaging 2017;46:184-93. [Crossref] [PubMed]
- Sakai I, Harada K, Hara I, Eto H, Miyake H. A comparison of the biological features between prostate cancers arising in the transition and peripheral zones. BJU Int 2005;96:528-32. [Crossref] [PubMed]
- Liu B, Cheng J, Guo DJ, He XJ, Luo YD, Zeng Y, Li CM. Prediction of prostate cancer aggressiveness with a combination of radiomics and machine learning-based analysis of dynamic contrast-enhanced MRI. Clin Radiol 2019;74:896.e1-8. [Crossref] [PubMed]
- Hou Y, Bao ML, Wu CJ, Zhang J, Zhang YD, Shi HB. A radiomics machine learning-based redefining score robustly identifies clinically significant prostate cancer in equivocal PI-RADS score 3 lesions. Abdom Radiol (NY) 2020;45:4223-34. [Crossref] [PubMed]
- Gong L, Xu M, Fang M, Zou J, Yang S, Yu X, Xu D, Zhou L, Li H, He B, Wang Y, Fang X, Dong D, Tian J. Noninvasive Prediction of High-Grade Prostate Cancer via Biparametric MRI Radiomics. J Magn Reson Imaging 2020;52:1102-9. [Crossref] [PubMed]
- Li M, Chen T, Zhao W, Wei C, Li X, Duan S, Ji L, Lu Z, Shen J. Radiomics prediction model for the improved diagnosis of clinically significant prostate cancer on biparametric MRI. Quant Imaging Med Surg 2020;10:368-79. [Crossref] [PubMed]
- Chen T, Li M, Gu Y, Zhang Y, Yang S, Wei C, Wu J, Li X, Zhao W, Shen J. Prostate Cancer Differentiation and Aggressiveness: Assessment With a Radiomic-Based Model vs. PI-RADS v2. J Magn Reson Imaging 2019;49:875-84. [Crossref] [PubMed]
- Bernatz S, Ackermann J, Mandel P, Kaltenbach B, Zhdanovich Y, Harter PN, et al. Comparison of machine learning algorithms to predict clinically significant prostate cancer of the peripheral zone with multiparametric MRI using clinical assessment categories and radiomic features. Eur Radiol 2020;30:6757-69. [Crossref] [PubMed]
- Zhang Y, Chen W, Yue X, Shen J, Gao C, Pang P, Cui F, Xu M. Development of a Novel, Multi-Parametric, MRI-Based Radiomic Nomogram for Differentiating Between Clinically Significant and Insignificant Prostate Cancer. Front Oncol 2020;10:888. [Crossref] [PubMed]
- Kan Y, Zhang Q, Hao J, Wang W, Zhuang J, Gao J, Huang H, Liang J, Marra G, Calleris G, Oderda M, Zhao X, Gontero P, Guo H. Clinico-radiological characteristic-based machine learning in reducing unnecessary prostate biopsies of PI-RADS 3 lesions with dual validation. Eur Radiol 2020;30:6274-84. [Crossref] [PubMed]
- Bleker J, Kwee TC, Dierckx RAJO, de Jong IJ, Huisman H, Yakar D. Multiparametric MRI and auto-fixed volume of interest-based radiomics signature for clinically significant peripheral zone prostate cancer. Eur Radiol 2020;30:1313-24. [Crossref] [PubMed]
- Min X, Li M, Dong D, Feng Z, Zhang P, Ke Z, You H, Han F, Ma H, Tian J, Wang L. Multi-parametric MRI-based radiomics signature for discriminating between clinically significant and insignificant prostate cancer: Cross-validation of a machine learning method. Eur J Radiol 2019;115:16-21. [Crossref] [PubMed]
- Lambin P, Leijenaar RTH, Deist TM, Peerlings J, de Jong EEC, van Timmeren J, Sanduleanu S, Larue RTHM, Even AJG, Jochems A, van Wijk Y, Woodruff H, van Soest J, Lustberg T, Roelofs E, van Elmpt W, Dekker A, Mottaghy FM, Wildberger JE, Walsh S. Radiomics: the bridge between medical imaging and personalized medicine. Nat Rev Clin Oncol 2017;14:749-62. [Crossref] [PubMed]
- Mongan J, Moy L, Kahn CE Jr. Checklist for Artificial Intelligence in Medical Imaging (CLAIM): A Guide for Authors and Reviewers. Radiol Artif Intell 2020;2:e200029. [Crossref] [PubMed]
- Park JE, Kim D, Kim HS, Park SY, Kim JY, Cho SJ, Shin JH, Kim JH. Quality of science and reporting of radiomics in oncologic studies: room for improvement according to radiomics quality score and TRIPOD statement. Eur Radiol 2020;30:523-36. [Crossref] [PubMed]
- Radtke JP, Schwab C, Wolf MB, Freitag MT, Alt CD, Kesch C, Popeneciu IV, Huettenbrink C, Gasch C, Klein T, Bonekamp D, Duensing S, Roth W, Schueler S, Stock C, Schlemmer HP, Roethke M, Hohenfellner M, Hadaschik BA. Multiparametric Magnetic Resonance Imaging (MRI) and MRI-Transrectal Ultrasound Fusion Biopsy for Index Tumor Detection: Correlation with Radical Prostatectomy Specimen. Eur Urol 2016;70:846-53. [Crossref] [PubMed]