Interval changes of histogram-derived diffusion indices predict treatment response to induction chemotherapy in head and neck cancer: a feasibility study
Introduction
The Global Burden of Disease study estimated 8,90,000 new head and neck cancer (HNC) cases worldwide in 2017, representing 5.3% of all cancer cases (1). Although radiation therapy or concurrent chemoradiation therapy (CCRT) remains the mainstay of treatment for HNC, induction chemotherapy (ICT) can reduce loco-regional relapse and distant metastasis. Numerous studies have investigated the role of ICT in HNC, and available evidence of whether ICT is superior to standard care (concurrent chemoradiotherapy) is inconclusive (2). In addition to the controversial role of treatment efficacy, the adverse events of ICT, such as hematological and renal toxicity, are the main concerns. In some studies, ICT treatment-related mortality has been reported to be as high as 6–7% (3,4). Therefore, early reliable predictive biomarkers that can reveal whether HNC patients would benefit from ICT are urgently required.
The apparent diffusion coefficient (ADC) map derived from diffusion-weighted magnetic resonance imaging (DWI) provides physiological information on tumor cellularity (5). Water diffusivity within the tumor can reflect changes in tumor cellularity, which occur after ICT, radiotherapy, or concurrent chemoradiotherapy. Pathophysiological changes revealed through DWI are usually evident before morphologic changes (6), with the potential use of these changes in early response assessments. A systematic review demonstrated that high pretreatment ADC and a low increase in early intratreatment or posttreatment ADC were potential indicators of locoregional failure in patients with HNC receiving chemoradiation therapy (7). Several studies (8-10) have investigated treatment responses to concurrent chemoradiation therapy or radiotherapy at different time points by using the mean or median value of ADC. However, HNC is typically heterogeneous; thus, ADC assessments are insufficient to reveal the diverse conditions of HNC. Recently, histogram analysis (11), which reflects the distribution and variation of all voxels and considers tumor heterogeneity, has been used in ADC studies, showing promising results for the prediction of the treatment response (12-14). Studies evaluating the predictive value of DWI for treatment response early after ICT are limited, and the optimal parameters of ADC analysis for predicting the treatment response have not been well established. Thus, reliable surrogate biomarkers should be identified that can be used for the individualization of treatment strategies.
This study evaluated the usefulness of interval change of ADC (∆ADC; derived from histogram analysis) between pretreatment and posttreatment (i.e., after one cycle of ICT) for the early prediction of treatment response to ICT in patients with HNC and the ability of derived indices to provide prognostic information for the patients. We present the following article in accordance with the STARD reporting checklist (available at https://qims.amegroups.com/article/view/10.21037/qims-22-263/rc).
Methods
Patients
The study was conducted in accordance with the Declaration of Helsinki (as revised in 2013). In our institution, pre- and post-ICT MRI scans were standard of care protocol in our clinical practice for HNC patients. And informed consent was also signed by the patients before the image studies. In this study, we retrospectively analyzed these MRI images to identify the radiomic features and the study was also approved by the local institutional review board of Chung Shan Medical University Hospital (No. CS1-21105).
Twenty-six consecutive patients with histologically confirmed primary HNC undergoing two MR examinations before and 1 week after the first cycle of ICT were enrolled between June 2018 and January 2019. Seven patients who received other treatments before ICT, who had poor-quality MR images due to a low signal-to-noise ratio or artifacts, or who could not complete ICT because of side effects were excluded. Eventually, 19 patients were eligible for inclusion in the present analysis (Figure 1). Patient characteristics are displayed in Table 1. Staging was performed for each patient according to the 8th edition of the American Joint Committee on Cancer (AJCC) Cancer Staging Manual, Head and Neck Section. All participants were followed up for at least 2 years.
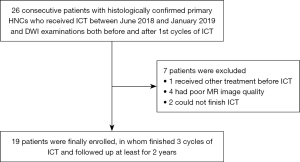
Table 1
Characteristics | All patients (n=19) | Responders (n=15) | Non-responders (n=4) |
---|---|---|---|
Age (years) | 55.9±6.91 [43–75] | 56.5±7.74 [43–75] | 54.0±0.82 [53–55] |
Male/female | 17/2 | 13/2 | 4/0 |
Tumor location | |||
Oral cavity | 10 | 7 | 3 |
Oropharynx | 2 | 2 | – |
Hypopharynx | 1 | 1 | – |
Larynx | 2 | 2 | – |
Nasopharynx | 3 | 3 | – |
Paranasal sinus | 1 | – | 1 |
T stage (AJCC 8th) | |||
T1 | 3 | 2 | 1 |
T2 | 4 | 4 | – |
T3 | 1 | 0 | 1 |
T4 | 11 | 9 | 2 |
N stage (AJCC 8th) | |||
N0 | 2 | 1 | 1 |
N1 | 7 | 6 | 1 |
N2 | 9 | 7 | 2 |
N3 | 1 | 1 | – |
AJCC, American Joint Committee on Cancer.
ICT and treatment response evaluation
The ICT regimen, which typically consists of docetaxel plus cisplatin and 5-fluorouracil (TPF), was modified according to the EORTC 24971/TAX 323 study (15). The modified TPF regimen consisted of 20 mg/m2 docetaxel administered as a 1-h infusion on days 1 and 8, 50 mg/m2 cisplatin infusion administered 2 h on day 1, 200 mg/m2 leucovorin infusion administered on day 1, and intravenous bolus 5-fluorouracil at 400 mg/m2 administered for 30 min on day 1, followed by intravenous continuous infusion of 1,200 mg/m2 5-fluorouracil for 48 h on day 1. The regimen was repeated every 2 weeks, and a total of three cycles of modified TPF was administered to patients. The Response Evaluation Criteria in Solid Tumors 1.1 (RECIST 1.1) criteria were used to evaluate the response to the modified TPF regimen (16).
MR examination
All data were acquired on a 3.0T clinical MR scanner (MAGNETOM® Skyra; Siemens Healthcare) with a 20-channel head and neck coil, covering the range from the level of the skull base to the thoracic inlet. Images were obtained with patients in the supine position. After three-plane tripilot imaging, turbo spin echo-based fat-saturated T2-weighted axial images (TR/TE =4,000/86 ms; matrix size =320×320; field of view =220×220 mm2; slice thickness =5 mm; bandwidth =400 Hz/Px; acquisition time =3 min and 6 s) and T1-weighted axial images (TR/TE =602/13 ms; matrix size =320×320; field of view =230×230 mm2; slice thickness =5 mm; bandwidth =240 Hz/Px; and acquisition time =1 min 52 s) were acquired. In addition, readout-segmented echo-planar DWI with two-dimensional navigator-based reacquisition [TR/TE =5,800/63 ms; matrix size =160×160 (zero-filled to 320×320); slice thickness =5 mm; no intersection gap; slice thickness =5 mm; number of slices =32; iPAT =2; bandwidth =919 Hz/Px; readout segments =5; echo spacing =0.34 ms; b value =0 and 800 s/mm2; and acquisition time =2 min 15 s] was performed. The motion-probing gradients were placed along three orthogonal axes with the same strength.
Data analysis
All measurements were performed by a neuroradiologist (with more than 10 years of experience in head and neck CT imaging), who was blinded to clinical and survival data. All primary tumors were evaluated through DWI performed from 1 to 3 days before the start of treatment and performed after the first cycle of ICT. The obtained data were transferred to an offline PC and analyzed using MATLAB 2018b software (MathWorks, Natick, MA, USA). All images in our study were accurately registered first, and then, ADC maps were calculated using signal intensities of the corresponding DWI images. Polygonal regions of interest (ROIs) were manually drawn on the derived ADC maps along the contours of the primary tumor on each slice (whole-lesion measure); the segmentation was aided with side-by-side visualization of T2-weighted and DWI images. ROIs in the lesions were carefully drawn with the inclusion of the solid portions of the lesions and the exclusion of any obviously cystic or necrotic areas with reference to T2WI (6,17). Subsequently, the mean, median, 25th percentile (ADC25), 75th percentile (ADC75), minimum, maximum, skewness, kurtosis, and entropy within the whole volume were derived through histogram analysis. Kurtosis indicates the histogram peakedness (the lower the kurtosis, the more flat the histogram); skewness is related to histogram symmetry (positive skewness indicates a right-tailed histogram); and entropy is a metric positively associated with image heterogeneity. The nth percentile is the point at which n% of the voxel values that form the histogram are found to the left. The interval changes (Δ) of the aforementioned parameters were generated by calculating the difference in pretreatment and posttreatment (after the first cycle of ICT) ADC values divided by the pretreatment ADC value. For example, ∆ADC = (ADC1st − ADCpre)/ADCpre, where ADCpre represents pretreatment ADC value, and ADC1st represents the ADC value after the first cycle of ICT. In addition, ROIs were used to measure the whole-tumor volume. In each primary tumor, the whole-tumor volume was calculated by multiplying each cross-sectional area by the section thickness.
Statistical analysis
The histogram indices of the interval change of ADC (including ∆ADCmin, ∆ADCmax, ∆ADCmean, ∆ADCmedian, ∆ADCkurtosis, ∆ADCskewness, ∆ADCentropy, ∆ADC25 and ∆ADC75) and the interval change of the primary tumor volume (∆TV) as well as other clinical variables such as age, T-stage (T1–2 versus T3–4) and N-stage (N0–1 versus N2–3) were compared between responders and non-responders by using a Mann–Whitney U test. Overall survival (OS) was defined as the time from the start of ICT to the occurrence of any cause of death; the data of patients who were alive at the end of follow-up were censored on that date. The survival curve for OS was generated using the Kaplan–Meier method, and differences in survival curves between responders and non-responders were tested for significance by using the log-rank test. A receiver operating characteristic (ROC) analysis with the area under the curve was used to investigate the discriminatory capability of the significant predictive values of the responders. To calculate the sensitivity, specificity, and accuracy of the significant predictive value of the responders, the optimal threshold was determined by giving equal weighting to sensitivity and specificity on the ROC curve. Statistical calculations were performed using statistical analysis software (Statistical Package for the Social Sciences, Version 18.0; IBM, Armonk, New York, USA), and a P value of <0.05 was considered statistically significant after correction for multiple comparisons.
Results
Patient characteristics
In this study, the data of 19 patients were included in analysis (17 men and 2 women; mean age, 56 years; range, 43–75 years), with the majority having oral cavity cancers (10/19). All patients had completed three cycles of ICT, with a median follow-up of 25 months (range, 4–31 months). According to the RECIST 1.1 criteria, HNC patients showing at least a partial response to ICT were identified as responders (n=15), and those showing stable or progressive disease were classified as non-responders (n=4). The detailed demographic characteristics are displayed in Table 1.
Analysis of histogram indices
Figures 2,3 show two representative cases of responders and non-responders, including the T2-weighted image, DWI image, ADC map, and ADC histogram acquired before (Figure 2A-2D) and after one-cycle (Figure 2E-2H) of ICT, respectively. Compared with non-responders, a decreased tumor volume was obtained on the T2-weighted and DWI images of responders, as well as an elevation of tumor water diffusion after ICT. Comparisons of histogram indices between responders and non-responders are summarized in Table 2. Although no significant differences were found in ∆ADCmean, ∆ADCmedian, ∆ADC75, ∆ADCmax, ∆ADCskewness, ∆ADCkurtosis, ∆ADCentrophy, ∆TV, age, T-stage, and N-stage between responders and non-responders (P>0.05), significant differences were found in ∆ADCmin (1.78±2.98 vs. −0.73±0.36, P=0.009) and ∆ADC25 (0.19±0.23 vs. −0.09±0.15, P=0.035) between the responders and non-responders.
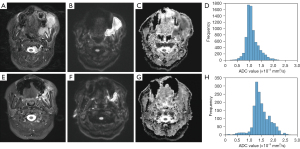
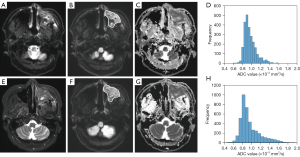
Table 2
Variables | All patients (n=19) | Responders (n=15) | Non-responders (n=4) | P value |
---|---|---|---|---|
ΔADCmean | 0.09±0.20 | 0.12±0.21 | −0.04±0.09 | 0.271 |
ΔADCmedian | 0.11±0.19 | 0.15±0.20 | −0.04±0.08 | 0.089 |
ΔADC25 | 0.13±0.24 | 0.19±0.23 | −0.09±0.15 | 0.035* |
ΔADC75 | 0.08±0.20 | 0.11±0.21 | 0.00±0.05 | 0.317 |
ΔADCkurtosis | 0.05±0.48 | 0.07±0.53 | 0.02±0.30 | 0.764 |
ΔADCskewness | −0.16±1.09 | −0.05±1.16 | −0.55±0.73 | 0.484 |
ΔADCentropy | 0.01±0.07 | 0.02±0.07 | −0.04±0.05 | 0.089 |
ΔADCmin | 1.25±2.83 | 1.78±2.98 | −0.73±0.36 | 0.009* |
ΔADCmax | −0.03±0.24 | −0.08±0.19 | 0.17±0.31 | 0.110 |
∆TV | −0.28±0.31 | −0.34±0.23 | −0.04±0.48 | 0.271 |
T stage (T1–2 vs. T3–4) | 7/12 | 6/9 | 1/3 | 0.591 |
N stage (N0–1 vs. N2–3) | 9/10 | 7/8 | 2/2 | 0.908 |
Age (years) | 55.9±6.91 | 56.5±7.74 | 54.0±0.82 | 0.315 |
*, statistically significant after Mann–Whitney U test. ΔADC, interval changes of ADC values were generated by calculating the difference in pretreatment and posttreatment (after one cycle of induction chemotherapy) ADC values divided by the pretreatment ADC value; ∆TV, interval changes of primary tumor volume were generated by calculating the difference in pretreatment and posttreatment (after one cycle of induction chemotherapy) primary tumor volume divided by the pretreatment primary tumor volume; ADC, apparent diffusion coefficient; TV, primary tumor volume.
Analyses of ROC and OS
Furthermore, ROC analyses were used to investigate the feasibility of using interval changes of ADC to predict HNC patients’ responses to ICT. The area under the ROC curve of ΔADCmin and ΔADC25 was 0.933 [95% confidence interval (CI): 0.720–0.997] (Figure 4A) and 0.850 (95% CI: 0.614–0.970) (Figure 4B), respectively. The highest sensitivity (80.0%, 73.3%) and specificity (100%, 100%) was obtained using the cutoff probability based on the Youden index when ΔADCmin and ∆ADC25 were selected. OS did not show significantly statistically differences between responders and non-responders (Figure 5).
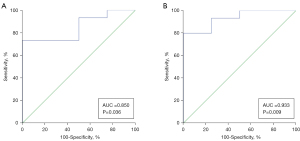
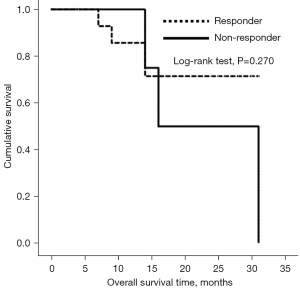
Discussion
The present study demonstrated the feasibility of interval change assessments in ADC through whole-tumor histogram analysis to predict the response to ICT in patients with HNC, contributing to the individualization of treatment strategies. Significantly higher ∆ADCmin and ∆ADC25 values were found in patients responding positively to ICT than in non-responders, probably because of elevated water diffusion resulting from low cellularity in the responsive tumor regions. Specifically, the results of ROC analysis revealed high AUC (0.933, 0.850) for ∆ADCmin and ∆ADC25, yielding high diagnostic sensitivity (80.0%, 73.3%) and specificity (100%, 100%), which suggests the superior discrimination ability of ∆ADCmin and ∆ADC25 in predicting therapeutic responses to ICT.
Several studies have indicated that the ADC change between pre- and intratreatment could be useful in predicting the treatment response to ICT, radiation therapy alone, or chemoradiotherapy in HNC. Better responses were revealed for tumors showing significant increases in ADC in the early phase of treatment compared with those with little or no ADC increase (6,7,18-21). Wong et al. found that early ADC change was the most powerful biomarker relative to other parameters derived from PET-CT and dynamic contrast-enhanced MRI (18). King et al. (21) further demonstrated that a serial change in ADC was a stronger marker than single ADC measurement at pre- or intratreatment for the prediction of the treatment response in patients with locally advanced HNC after CRT. However, another report demonstrated no significant difference in ADC values between responders and non-responders after two cycles of ICT (19), implying the possible variation of mean ADC during the therapeutic period. Our finding is consistent with the findings of the study; no significant differences were obtained in the interval changes of the mean ADC and tumor volume between the two groups, supporting the possible limitation of conducting conventional comparisons of the mean ADC values.
Several investigators have used the mean (9,10) or median (8,22) ADC to predict the treatment response to ICT or chemoradiotherapy in HNC. However, HNC is typically heterogeneous, and this heterogeneity may be due to areas with different cellularity, necrosis, and stroma and areas with increased or decreased vascularity. The mean and median ADC may be suboptimal, and they are not always significantly sensitive to small changes or treatment effects, because when areas with different ADC values are included in ROI, the effects of heterogeneity are smoothed out. The histogram analysis of ADC values within the primary tumor has been frequently used to adaptively evaluate the heterogeneity of the primary tumor relative to the complex tumor microenvironment (11). Such analysis has proven helpful in predicting the histologic grade (23), HPV status (24), and treatment response (25,26) in patients with HNC. In the present study, although primary tumor ∆ADCmean and ∆ADCmedian values were higher in responders than in non-responders, which is compatible with the results of previous studies (18,20), this finding did not reach statistical significance. By contrast, ∆ADCmin and ∆ADC25 in whole-primary-tumor assessments showed significant differences between responders and non-responders, indicating the potential of using ADC histogram analysis to extract effective surrogate biomarkers for prognosis prediction.
∆ADCmin or ∆ADC25 derived from histogram analysis has been applied for assessing chemotherapy responses in malignant bone tumors (27,28), pancreatic cancer (29), or gynecological cancer (30). Saleh et al. (27) and Oka et al. (28) have used ∆ADCmin to monitor responses to chemotherapy in osteosarcoma or Ewing’s sarcoma, demonstrating that ∆ADCmin values were significantly higher in patients with good responses than in those with poor responses. Kyriazi et al. (30) also found that changes in all ADC histogram parameters after the first and third cycles of chemotherapy were higher in responders than in non-responders, indicating the superior discrimination ability of ∆ADC25 in predicting responses to chemotherapy in patients with metastatic ovarian or primary peritoneal cancer. For responders, due to cell shrinkage and death after treatment, the increase in ADC would occur as a result of an increase in the fractional volume and diffusion of water molecules in the extracellular space. A strong negative correlation exists between ADC values and tumor cellularity (5), indicating that the tumor regions with the lowest or lower diffusivity (i.e., the highest or higher cellularity) may be more sensitive to chemotherapy or radiotherapy. This might explain why ∆ADCmin or ∆ADC25 is the most significant predictor of treatment response in cancer patients. The present study demonstrated the superior performance of ∆ADCmin and ∆ADC25 in predicting the response to ICT, which is in agreement with the previous report.
Approximately 20–30% of patients do not respond to ICT (31-33), and our results showed similar results in that four of our enrolled patients (4/19, 21%) did not show responses to ICT. All of these four patients received concurrent chemoradiotherapy, but three died before the study endpoint due to disease progression, which implies that a poor response to ICT might be a poor prognostic factor of OS. Two (patient 1 and 2 in Table 3) patients received additional target therapy with cetuximab after ICT failure and showed longer survival than those without therapy. Several studies (34,35) have shown that cetuximab can improve survival outcomes in advanced HNC. Taken together, these findings indicate that the early identification of potential poor responders to ICT and an early shift in treatment regimens to target therapy or immune therapy may improve survival outcomes in HNC.
Table 3
Patient No. | Age, years | Gender | TNM stage | Tumor location | Survival time (months) | Treatment regimens | Final status |
---|---|---|---|---|---|---|---|
1 | 55 | Male | T4bN2bM0 | Buccal | 33 | ICT + target therapy + CRT | Alive |
2 | 54 | Male | T3N0M0 | Maxillary sinus | 31 | ICT + OP + target therapy + CRT | Dead |
3 | 54 | Male | T2N1M0 | Tongue | 16 | ICT + CRT | Dead |
4 | 53 | Male | T4aN2bM0 | Gingival | 14 | ICT + CRT | Dead |
ICT, induction chemotherapy; CRT, concurrent chemoradiotherapy; OP, operation.
Our study has some limitations. First, this was a retrospective study with a small patient population with varying tumor locations. Although non-responders tended to have shorter survival times than responders, no significant difference was found, possibly resulting from the small and heterogeneous patient population in this study. The main purpose of this study was to identify early predictors of treatment response to avoid ineffective ICT. A multicenter prospective study with a larger sample size should be conducted to validate the clinical effectiveness of our findings. Second, ROIs were manually drawn by one observer, which limits reproducibility. A comprehensive investigation of interobserver, intraobserver, or intersoftware variances is required. Third, the second DWI was performed after one cycle of ICT, which would not reveal the evolution of the ADC pattern during ICT. A longitudinal study with serial ADC measurements would be useful. Fourth, performing DW imaging in the regions of the head and neck is still challenging. Although the use of the segmented-readout EPI scheme could alleviate susceptibility artifacts, problems with patient movement from swallowing cannot be effectively resolved. Further reduction of the total acquisition time by using advanced imaging sequences would be helpful.
In conclusion, our findings demonstrated that ADC histogram analysis can be used to extract potential surrogate biomarkers for the early prediction of the treatment response to ICT. ∆ADCmin and ∆ADC25 before and after one cycle of ICT outperformed other histogram indices, showing promising diagnostic efficacy for predicting responsiveness to ICT. These early predictive biomarkers may help avoid ineffective treatments and unnecessary toxicity, enabling further individualization of treatment strategies.
Acknowledgments
This manuscript was edited by Wallace Academic Editing.
Funding: This work was supported by a grant (#MOST 108-2221-E-040-007-MY3) at the Ministry of Science and Technology, Taiwan.
Footnote
Reporting Checklist: The authors have completed the STARD reporting checklist. Available at https://qims.amegroups.com/article/view/10.21037/qims-22-263/rc
Conflicts of Interest: All authors have completed the ICMJE uniform disclosure form (available at https://qims.amegroups.com/article/view/10.21037/qims-22-263/coif). PHT reports that this work was supported by a grant (#MOST 108-2221-E-040-007-MY3) at the Ministry of Science and Technology, Taiwan. The other authors have no conflicts of interest to declare.
Ethical Statement: The authors are accountable for all aspects of the work in ensuring that questions related to the accuracy or integrity of any part of the work are appropriately investigated and resolved. The study was conducted in accordance with the Declaration of Helsinki (as revised in 2013), The study was approved by the local institutional review board of Chung Shan Medical University Hospital (No. CS1-21105) and informed consent was signed by the patients before the image studies.
Open Access Statement: This is an Open Access article distributed in accordance with the Creative Commons Attribution-NonCommercial-NoDerivs 4.0 International License (CC BY-NC-ND 4.0), which permits the non-commercial replication and distribution of the article with the strict proviso that no changes or edits are made and the original work is properly cited (including links to both the formal publication through the relevant DOI and the license). See: https://creativecommons.org/licenses/by-nc-nd/4.0/.
References
- Aupérin A. Epidemiology of head and neck cancers: an update. Curr Opin Oncol 2020;32:178-86. [Crossref] [PubMed]
- Haddad RI, Posner M, Hitt R, Cohen EEW, Schulten J, Lefebvre JL, Vermorken JB. Induction chemotherapy in locally advanced squamous cell carcinoma of the head and neck: role, controversy, and future directions. Ann Oncol 2018;29:1130-40. [Crossref] [PubMed]
- Gau M, Karabajakian A, Reverdy T, Neidhardt EM, Fayette J. Induction chemotherapy in head and neck cancers: Results and controversies. Oral Oncol 2019;95:164-9. [Crossref] [PubMed]
- Ferrari D, Ghi MG, Franzese C, Codecà C, Gau M, Fayette J. The Slippery Role of Induction Chemotherapy in Head and Neck Cancer: Myth and Reality. Front Oncol 2020;10:7. [Crossref] [PubMed]
- Chen L, Liu M, Bao J, Xia Y, Zhang J, Zhang L, Huang X, Wang J. The correlation between apparent diffusion coefficient and tumor cellularity in patients: a meta-analysis. PLoS One 2013;8:e79008. [Crossref] [PubMed]
- Matoba M, Tuji H, Shimode Y, Toyoda I, Kuginuki Y, Miwa K, Tonami H. Fractional change in apparent diffusion coefficient as an imaging biomarker for predicting treatment response in head and neck cancer treated with chemoradiotherapy. AJNR Am J Neuroradiol 2014;35:379-85. [Crossref] [PubMed]
- Chung SR, Choi YJ, Suh CH, Lee JH, Baek JH. Diffusion-weighted Magnetic Resonance Imaging for Predicting Response to Chemoradiation Therapy for Head and Neck Squamous Cell Carcinoma: A Systematic Review. Korean J Radiol 2019;20:649-61. [Crossref] [PubMed]
- Kim S, Loevner L, Quon H, Sherman E, Weinstein G, Kilger A, Poptani H. Diffusion-weighted magnetic resonance imaging for predicting and detecting early response to chemoradiation therapy of squamous cell carcinomas of the head and neck. Clin Cancer Res 2009;15:986-94. [Crossref] [PubMed]
- Lambrecht M, Van Calster B, Vandecaveye V, De Keyzer F, Roebben I, Hermans R, Nuyts S. Integrating pretreatment diffusion weighted MRI into a multivariable prognostic model for head and neck squamous cell carcinoma. Radiother Oncol 2014;110:429-34. [Crossref] [PubMed]
- Schouten CS, de Bree R, van der Putten L, Noij DP, Hoekstra OS, Comans EF, Witte BI, Doornaert PA, Leemans CR, Castelijns JA. Diffusion-weighted EPI- and HASTE-MRI and 18F-FDG-PET-CT early during chemoradiotherapy in advanced head and neck cancer. Quant Imaging Med Surg 2014;4:239-50. [PubMed]
- Just N. Improving tumour heterogeneity MRI assessment with histograms. Br J Cancer 2014;111:2205-13. [Crossref] [PubMed]
- King AD, Chow KK, Yu KH, Mo FK, Yeung DK, Yuan J, Bhatia KS, Vlantis AC, Ahuja AT. Head and neck squamous cell carcinoma: diagnostic performance of diffusion-weighted MR imaging for the prediction of treatment response. Radiology 2013;266:531-8. [Crossref] [PubMed]
- Ren JL, Yuan Y, Li XX, Shi YQ, Tao XF. Histogram analysis of apparent diffusion coefficient maps in the prognosis of patients with locally advanced head and neck squamous cell carcinoma: Comparison of different region of interest selection methods. Eur J Radiol 2018;106:7-13. [Crossref] [PubMed]
- Ryoo I, Kim JH, Choi SH, Sohn CH, Kim SC. Squamous Cell Carcinoma of the Head and Neck: Comparison of Diffusion-weighted MRI at b-values of 1,000 and 2,000 s/mm(2) to Predict Response to Induction Chemotherapy. Magn Reson Med Sci 2015;14:337-45. [Crossref] [PubMed]
- Vermorken JB, Remenar E, van Herpen C, Gorlia T, Mesia R, Degardin M, Stewart JS, Jelic S, Betka J, Preiss JH, van den Weyngaert D, Awada A, Cupissol D, Kienzer HR, Rey A, Desaunois I, Bernier J, Lefebvre JL. EORTC 24971/TAX 323 Study Group. Cisplatin, fluorouracil, and docetaxel in unresectable head and neck cancer. N Engl J Med 2007;357:1695-704. [Crossref] [PubMed]
- Nishino M, Jagannathan JP, Ramaiya NH, Van den Abbeele AD. Revised RECIST guideline version 1.1: What oncologists want to know and what radiologists need to know. AJR Am J Roentgenol 2010;195:281-9. [Crossref] [PubMed]
- Fujima N, Kudo K, Yoshida D, Homma A, Sakashita T, Tsukahara A. Khin Khin Tha, Yuri Zaitsu, Satoshi Terae, Hiroki Shirato. Arterial spin labeling to determine tumor viability in head and neck cancer before and after treatment. J Magn Reson Imaging 2014;40:920-8. [Crossref] [PubMed]
- Wong KH, Panek R, Dunlop A, Mcquaid D, Riddell A, Welsh LC, Murray I, Koh DM, Leach MO, Bhide SA, Nutting CM, Oyen WJ, Harrington KJ, Newbold KL. Changes in multimodality functional imaging parameters early during chemoradiation predict treatment response in patients with locally advanced head and neck cancer. Eur J Nucl Med Mol Imaging 2018;45:759-67. [Crossref] [PubMed]
- Powell C, Schmidt M, Borri M, Koh DM, Partridge M, Riddell A, Cook G, Bhide SA, Nutting CM, Harrington KJ, Newbold KL. Changes in functional imaging parameters following induction chemotherapy have important implications for individualised patient-based treatment regimens for advanced head and neck cancer. Radiother Oncol 2013;106:112-7. [Crossref] [PubMed]
- Vandecaveye V, Dirix P, De Keyzer F, de Beeck KO, Vander Poorten V, Roebben I, Nuyts S, Hermans R. Predictive value of diffusion-weighted magnetic resonance imaging during chemoradiotherapy for head and neck squamous cell carcinoma. Eur Radiol 2010;20:1703-14. [Crossref] [PubMed]
- King AD, Mo FK, Yu KH, Yeung DK, Zhou H, Bhatia KS, Tse GM, Vlantis AC, Wong JK, Ahuja AT. Squamous cell carcinoma of the head and neck: diffusion-weighted MR imaging for prediction and monitoring of treatment response. Eur Radiol 2010;20:2213-20. [Crossref] [PubMed]
- Wong KH, Panek R, Welsh L, Mcquaid D, Dunlop A, Riddell A, Murray I, Du Y, Chua S, Koh DM, Bhide S, Nutting C, Oyen WJ, Harrington K, Newbold KL. The Predictive Value of Early Assessment After 1 Cycle of Induction Chemotherapy with 18F-FDG PET/CT and Diffusion-Weighted MRI for Response to Radical Chemoradiotherapy in Head and Neck Squamous Cell Carcinoma. J Nucl Med 2016;57:1843-50. [Crossref] [PubMed]
- Meyer HJ, Leifels L, Hamerla G, Höhn AK, Surov A. ADC-histogram analysis in head and neck squamous cell carcinoma. Associations with different histopathological features including expression of EGFR, VEGF, HIF-1α, Her 2 and p53. A preliminary study. Magn Reson Imaging 2018;54:214-7. [Crossref] [PubMed]
- de Perrot T, Lenoir V, Domingo Ayllón M, Dulguerov N, Pusztaszeri M, Becker M. Apparent Diffusion Coefficient Histograms of Human Papillomavirus-Positive and Human Papillomavirus-Negative Head and Neck Squamous Cell Carcinoma: Assessment of Tumor Heterogeneity and Comparison with Histopathology. AJNR Am J Neuroradiol 2017;38:2153-60. [Crossref] [PubMed]
- Martens RM, Noij DP, Koopman T, Zwezerijnen B, Heymans M, de Jong MC, Hoekstra OS, Vergeer MR, de Bree R, Leemans CR, de Graaf P, Boellaard R, Castelijns JA. Predictive value of quantitative diffusion-weighted imaging and 18-F-FDG-PET in head and neck squamous cell carcinoma treated by (chemo)radiotherapy. Eur J Radiol 2019;113:39-50. [Crossref] [PubMed]
- Lee MK, Choi Y, Jung SL. Diffusion-weighted MRI for predicting treatment response in patients with nasopharyngeal carcinoma: a systematic review and meta-analysis. Sci Rep 2021;11:18986. [Crossref] [PubMed]
- Saleh MM, Abdelrahman TM, Madney Y, Mohamed G, Shokry AM, Moustafa AF. Multiparametric MRI with diffusion-weighted imaging in predicting response to chemotherapy in cases of osteosarcoma and Ewing's sarcoma. Br J Radiol 2020;93:20200257. [Crossref] [PubMed]
- Oka K, Yakushiji T, Sato H, Hirai T, Yamashita Y, Mizuta H. The value of diffusion-weighted imaging for monitoring the chemotherapeutic response of osteosarcoma: a comparison between average apparent diffusion coefficient and minimum apparent diffusion coefficient. Skeletal Radiol 2010;39:141-6. [Crossref] [PubMed]
- Nishiofuku H, Tanaka T, Marugami N, Sho M, Akahori T, Nakajima Y, Kichikawa K. Increased tumour ADC value during chemotherapy predicts improved survival in unresectable pancreatic cancer. Eur Radiol 2016;26:1835-42. [Crossref] [PubMed]
- Kyriazi S, Collins DJ, Messiou C, Pennert K, Davidson RL, Giles SL, Kaye SB, Desouza NM. Metastatic ovarian and primary peritoneal cancer: assessing chemotherapy response with diffusion-weighted MR imaging--value of histogram analysis of apparent diffusion coefficients. Radiology 2011;261:182-92. [Crossref] [PubMed]
- Posner MR, Hershock DM, Blajman CR, Mickiewicz E, Winquist E, Gorbounova V, et al. Cisplatin and fluorouracil alone or with docetaxel in head and neck cancer. N Engl J Med 2007;357:1705-15. [Crossref] [PubMed]
- Hitt R, Grau JJ, López-Pousa A, Berrocal A, García-Girón C, Irigoyen A, Sastre J, Martínez-Trufero J, Brandariz Castelo JA, Verger E, Cruz-Hernández JJSpanish Head and Neck Cancer Cooperative Group (TTCC). A randomized phase III trial comparing induction chemotherapy followed by chemoradiotherapy versus chemoradiotherapy alone as treatment of unresectable head and neck cancer. Ann Oncol 2014;25:216-25. [Crossref] [PubMed]
- Bernadach M, Lapeyre M, Dillies AF, Miroir J, Casile M, Moreau J, Molnar I, Ginzac A, Pham-Dang N, Saroul N, Durando X, Biau J. Predictive factors of toxicity of TPF induction chemotherapy for locally advanced head and neck cancers. BMC Cancer 2021;21:360. [Crossref] [PubMed]
- Lee KW, Koh Y, Kim SB, Shin SW, Kang JH, Wu HG, et al. A Randomized, Multicenter, Phase II Study of Cetuximab With Docetaxel and Cisplatin as Induction Chemotherapy in Unresectable, Locally Advanced Head and Neck Cancer. Oncologist 2015;20:1119-20. [Crossref] [PubMed]
- Hecht M, Hahn D, Wolber P, Hautmann MG, Reichert D, Weniger S, Belka C, Bergmann T, Göhler T, Welslau M, Große-Thie C, Guntinas-Lichius O, von der Grün J, Balermpas P, Orlowski K, Messinger D, Stenzel KG, Fietkau R. A Prospective Real-World Multi-Center Study to Evaluate Progression-Free and Overall Survival of Radiotherapy with Cetuximab and Platinum-Based Chemotherapy with Cetuximab in Locally Recurrent Head and Neck Cancer. Cancers (Basel) 2021;13:3413. [Crossref] [PubMed]