A nomogram based on 18F-fluorodeoxyglucose PET/CT and clinical features to predict epidermal growth factor receptor mutation status in patients with lung adenocarcinoma
Introduction
As the most common histological subtype of non-small cell lung cancer (NSCLC), lung adenocarcinoma (LADC) accounts for nearly 50% of all primary lung cancers. The reported incidence of LADC is continuously increasing due to multiple factors such as air pollution and exposure to second-hand smoke, as well as improved early detection through low-dose computed tomography (CT) screening (1). With the discovery of an increasing number of driver oncogenes, targeted therapy has greatly extended the progression-free survival of patients with LADC. For patients with EGFR-mutated LADCs, tyrosine kinase inhibitors (TKIs) targeting the epidermal growth factor receptor (EGFR) have become the standard of care (2). Identifying activating mutations of EGFR in cases of LADC is essential for selecting appropriate candidates for EGFR-TKI therapy, and is recommended by the National Comprehensive Cancer Network (NCCN) Guideline Version 2.2021 (3).
In clinical practice, accurate genetic analysis relies on surgical or biopsy samples. However sometimes the quantity or quality of biopsy specimens is insufficient for molecular testing due to the intratumoral heterogeneity of LADCs or because the condition or personal preference of the patient prohibits an invasive biopsy procedure. As a result, there is a lack of information on somatic mutations in almost 30% of NSCLC cases (4,5). Therefore, a noninvasive, simple, and practical means of predicting EGFR mutation status to inform treatment decision-making is needed.
18F-fluorodeoxyglucose positron emission tomography/CT (18F-FDG PET/CT) is a hybrid molecular imaging technique that provides simultaneous metabolic and morphological information, and has great benefit for the diagnosis, staging, and prognostication of patients with LADCs (6-8). The metabolic and morphological characteristics of EGFR-mutated LADCs are distinct from those of EGFR wild-type LADCs (9-13), but the results of previous studies are inconsistent, such as either higher or lower value of those parameters being more indicative of EGFR mutation. Two meta-analyses revealed a moderate ability of PET metabolic parameters alone (14) and CT features alone (15) to discriminate EGFR-mutated and wild-type LADCs. Recently, some researchers have used radiomics or deep learning to enhance the efficacy of predictive methods (16-18), but these technologies require specialized software and are neither practical nor feasible for daily clinical use.
Therefore, in the present study, we aimed to establish a convenient and simple nomogram based on metabolic and volume-based parameters and CT characteristics derived from 18F-FDG PET/CT to predict EGFR mutation status in patients with LADC. We present the following article in accordance with the TRIPOD reporting checklist (available at https://qims.amegroups.com/article/view/10.21037/qims-22-248/rc).
Methods
This retrospective study was conducted in accordance with the Declaration of Helsinki (as revised in 2013). This retrospective research was conducted under the approval of the Ethics Committee of Beijing Hospital, and the requirement to obtain individual consent for this retrospective analysis was waived.
Patients
A total of 394 consecutive patients with histologically confirmed LADCs who underwent diagnostic 18F-FDG PET/CT scanning between October 2012 and December 2019 were retrospectively enrolled. The exclusion criteria were as follows: (I) EGFR test results were not available (n=81); (II) the patient had a history of other malignancies (n=7); (III) the interval between the PET/CT scan and pathological and gene analyses exceeded 4 weeks (n=15); (IV) the patient had undergone chemotherapy or radiotherapy before the PET/CT scan (n=5); and (V) the image quality was poor due to respiratory artefacts or the tumor manifesting as an inflammatory pattern, which made delineation of the region of interest difficult (n=12). Finally, 274 Asian patients were included. All clinical data, including age, sex, smoking status (never or former/current), and tumor-node-metastasis (TNM) stage (according to the eighth edition of the International Association for the Study of Lung Cancer staging system), were collected from the patients’ medical records.
Acquisition of PET/CT images
All PET/CT studies were performed using the same equipment (Biograph mCT PET/CT, Siemens) and scanning protocol. The 18F-FDG was produced and supplied by Beijing Atom High Tech Co., Ltd., with radiochemical purity >95%. Each participant fasted for at least 4 hours and needed to have a controlled blood glucose level <11.1 mmol/L before they received an intravenous injection of 5.18 MBq/kg (0.14 mCi/kg) 18F-FDG. After 60 min had passed, a spiral CT scan from the base of the skull to the proximal thighs in a supine position was conducted with a tube voltage of 120 kV and flexible tube current adjusted by CARE dose technology, followed by a PET scan (2 min/bed, 5–7 beds) acquired in the three-dimensional mode with the same coverage. The PET data were corrected for attenuation by CT images and reconstructed using a Gaussian filter with an ordered subset expectation-maximization algorithm (2 iterations, 20 subsets), incorporating correction with the time-of-flight model. To show the morphological details more clearly, a regional 1.0-mm thin-layer high-resolution lung CT scan under deep inspiration breath-hold conditions was added covering the whole primary lesion.
Interpretation of PET/CT images
The PET/CT images were analyzed by two board-certified physicians, each with >5 years of experience in chest PET and CT imaging, working side by side at the workstation (Syngo MI applications, Volumetric Analysis 6.0.14.4, Siemens Medical Solutions). During image interpretation, both physicians were blinded to the participants’ clinical data and EGFR testing results. When multifocal LADCs were encountered, the largest lesion was selected for analysis. The volume of interest (VOI) was placed to encircle the entire lesion on reconstructed axial/coronal/sagittal PET images, in which metabolic parameters, including the maximum standardized uptake value (SUVmax), mean standardized uptake value (SUVmean), metabolic tumor volume (MTV), and the standard deviation (SD) of the SUV, were measured using a threshold of 40% of the SUVmax, which is used in our daily routine practice and recommended in a previous study (19). CT and PET/CT fusion images were displayed simultaneously to observe the contour of the VOI and, when necessary, manual minor adjustments were made to ensure the accuracy of delineation. The MTV was defined as the total tumor volume inside the outline of the VOI. Total lesion glycolysis (TLG) was calculated as the product of the MTV and the SUVmean of the lesion. The heterogeneity parameters coefficient of variation (COV) and heterogeneity index (HI) were calculated as SD/SUVmean ×100 (20) and SUVmax/SUVmean, respectively (21). CT features were assessed at the lung window (width, 1,500 HU; level, –600 HU). The following characteristics were recorded: (I) size, represented by the longest diameter (in cm) of the tumor on the axial image; (II) the presence or absence of a ground-glass opacity (GGO), in which blood vessels can be observed; (III) the presence or absence of vascular convergence towards the tumor; (IV) the presence or absence of pleural retraction by the tumor; (V) the presence or absence of air bronchogram, indicated by a tube-like or branched air structure within the tumor; (VI) the presence or absence of spiculation, defined as lines or stripes radiating from the tumor margin; (VII) the presence or absence of cavitation/bubble-like lucency, indicating air within the tumor; (VIII) the presence or absence of lobulation, indicated by a tumor margin with a wavy configuration; and (IX) the presence or absence of pleural effusion. The final values of the continuous variables, including the SUVmax, SUVmean, MTV, SD and size, were the mean values measured by the two observers. In the evaluation of categorical variables, such as CT features, consensus was reached by discussion in cases of disagreement.
EGFR mutation testing
Genomic DNA was extracted from tissue specimens obtained by surgery or biopsy. The mutation status of EGFR exons 18, 19, 20, and 21 was identified with a polymerase chain reaction-based amplification refractory mutation system using the AmoyDx Human EGFR Mutation Detection Kit (Amoy Diagnostics, Xiamen, China). The presence of any mutation within exons 18–21 was defined as EGFR positive (EGFR+), while no evidence of gene mutation was defined as EGFR negative (EGFR–).
Establishment and evaluation of the nomogram prediction model
Multivariate logistic regression with backward stepwise selection was applied to determine the independent predictors among the clinical and PET/CT parameters. Then, a nomogram prediction model incorporating the significant factors was constructed. Leave-one-out cross-validation was used to assess the generalization of the model. The discrimination ability of the nomogram was assessed by calculating the area under the curve (AUC) of the receiver operating characteristic (ROC) curve. Calibration curves and the Hosmer-Lemeshow test were used to evaluate the consistency between predicted and observed values. The clinical utility of the nomogram was assessed through decision curve analysis.
Statistical analysis
SPSS 22.0 (IBM Corp., Armonk, NY, USA) and R software (version 4.1.2, Vienna, Austria; URL https://www.R-project.org/) were used for statistical analysis. The normality of continuous variables was evaluated using the Kolmogorov-Smirnov test. Variables with a normal distribution were expressed as the mean ± SD, while others were expressed as the median (interquartile range). Categorical variables were expressed as numbers (percentages). Comparisons of continuous variables were performed using Student’s t-test or the Mann-Whitney U test, and categorical variables were evaluated using Pearson’s chi-squared test. To obtain the optimal cut-off value for dichotomization of the significant parameters, ROC curves were drawn, and the AUC and 95% confidence interval (CI) were calculated to evaluate the performance of the significant parameters for discriminating LADCs with EGFR mutations. Multivariate logistic regression analysis was performed using the “glmnet” package, and odds ratios (ORs) with 95% CIs were calculated. The nomogram prediction model and calibration curves were constructed and plotted using the “rms” package. For model evaluation, leave-one-out cross-validation and ROC curves were computed using the “caret” and “pROC” packages, and the AUC, sensitivity, specificity, and accuracy were calculated. The Hosmer-Lemeshow test was performed using the “ResourceSelection” package. The decision curve analysis was conducted using the “rmda” package. A two-sided P value <0.05 was regarded as a significant difference.
Results
Patients’ clinical characteristics
The general characteristics of the included patients are summarized in Table 1. The 274 patients had a median age of 65 years (range, 27–88 years). Of the patients, 130 (47.4%) were male and 101 (36.9%) were former or current smokers. There were 114 (41.6%) and 160 (58.4%) patients with stage I–II disease and stage III–IV disease, respectively. Mutations in EGFR were detected in 143 (52.2%) patients, of whom 54 (37.8%) patients had exon 19 deletions, 63 (44.0%) had exon 21 L858R mutations, 7 (4.9%) had exon 21 L861Q mutations, 6 (4.2%) had exon 20 insertions, 2 (1.4%) had exon 18 G719X mutations, and 11 (7.7%) had combined mutations.
Table 1
Variable | Total (n=274) | EGFR mutation-positive n=143 (52.2%) | EGFR mutation-negative n=131 (47.8%) | χ2/Z/t | P value |
---|---|---|---|---|---|
Age (years) | 65.00 [57.75–74.00] | 65.00 [57.00–77.00] | 65.00 [58.00–72.00] | –1.242 | 0.214 |
Sex | 36.214 | <0.001 | |||
Male | 130 (47.4) | 43 (33.1) | 87 (66.9) | ||
Female | 144 (52.5) | 100 (69.4) | 44 (30.6) | ||
Smoking status | 38.378 | <0.001 | |||
Former/current | 101 (36.9) | 28 (27.7) | 73 (72.3) | ||
Never | 173 (63.1) | 115 (66.5) | 58 (33.5) | ||
Staging | 6.642 | 0.010 | |||
I–II | 114 (41.6) | 70 (61.4) | 44 (38.6) | ||
III–IV | 160 (58.4) | 73 (45.6) | 87 (54.4) | ||
GGO component | 7.263 | 0.007 | |||
Presence | 82 (29.9) | 53 (64.6) | 29 (35.4) | ||
Absence | 192 (70.1) | 90 (46.9) | 102 (53.1) | ||
Vascular convergence | 3.948 | 0.047 | |||
Presence | 128 (46.5) | 75 (58.6) | 53 (41.4) | ||
Absence | 146 (53.5) | 68 (46.6) | 78 (53.4) | ||
Pleural retraction | 10.148 | 0.001 | |||
Presence | 163 (59.5) | 98 (60.1) | 65 (39.9) | ||
Absence | 111 (40.5) | 45 (40.5) | 66 (59.5) | ||
Air bronchogram | 6.652 | 0.010 | |||
Presence | 101 (36.9) | 63 (62.4) | 38 (37.6) | ||
Absence | 173 (63.1) | 80 (46.2) | 93 (53.8) | ||
Spiculation | 2.765 | 0.096 | |||
Presence | 193 (70.4) | 107 (55.4) | 86 (44.6) | ||
Absence | 81 (29.6) | 36 (44.4) | 45 (55.6) | ||
Cavitation/bubble-like lucency | 2.512 | 0.113 | |||
Presence | 52 (19.0) | 22 (42.3) | 30 (57.7) | ||
Absence | 222 (81.0) | 121 (54.5) | 101 (45.5) | ||
Lobulation | 5.565 | 0.018 | |||
Presence | 243 (88.7) | 133 (54.7) | 110 (45.3) | ||
Absence | 31 (11.3) | 10 (32.3) | 21 (67.7) | ||
Pleural effusion | 0.446 | 0.504 | |||
Presence | 56 (20.4) | 27 (48.2) | 29 (51.8) | ||
Absence | 218 (79.6) | 116 (53.2) | 102 (46.8) | ||
Size (cm) | 2.92 [2.03–4.08] | 2.76 [1.97–3.60] | 3.30 [2.14–4.75] | −2.869 | 0.004 |
SUVmax | 11.68 [6.33–17.13] | 9.49 [4.70–15.22] | 13.70 [8.95–20.46] | −4.341 | <0.001 |
SUVmean | 6.88 [3.52–10.32] | 5.56 [2.70–9.11] | 8.52 [5.11–12.08] | −4.435 | <0.001 |
MTV (cm3) | 5.71 [2.37–13.99] | 4.91[2.46–10.56] | 7.79 [2.37–23.13] | −2.455 | 0.014 |
TLG (g) | 33.04 [10.09–129.72] | 26.07 [7.28–64.03] | 52.85 [13.96–243.43] | −3.686 | <0.001 |
COV | 23.76±3.47 | 24.00±3.30 | 23.50±3.63 | −1.205 | 0.229 |
HI | 1.69 [1.61–1.76] | 1.70 [1.61–1.77] | 1.68 [1.61–1.75] | −0.449 | 0.653 |
Data are expressed as n (%) or median [interquartile range] or mean ± standard deviation. PET, positron emission tomography; CT, computed tomography; EGFR, epidermal growth factor receptor; GGO, ground-glass opacity; SUVmax, maximum standardized uptake value; SUVmean, mean standardized uptake value; MTV, metabolic tumor volume; TLG, total lesion glycolysis; COV, coefficient of variation; HI, heterogeneity index; IQR, interquartile range.
Associations of clinical, PET, and CT features with EGFR mutation status
As shown in Table 1, EGFR mutations were observed more frequently in female patients than in male patients (69.4% vs. 33.1%, χ2=36.214, P<0.001), in never-smokers than in former/current smokers (66.5% vs. 27.7%, χ2=38.378, P<0.001), and in stage I–II disease than in stage III–IV disease (61.4% vs. 45.6%, χ2=6.642, P=0.01). The median age showed no difference between the EGFR+ and EGFR– groups (Z=–1.242, P=0.214).
Concerning the PET parameters, the SUVmax (P<0.001), SUVmean (P<0.001), MTV (P=0.014), and TLG (P<0.001) in the EGFR+ group were significantly lower than those in the EGFR– group, while the heterogeneity parameters COV and HI were not associated with EGFR mutation status (P=0.229 and 0.653, respectively).
Regarding CT features, the LADCs in the EGFR+ group were significantly smaller than those in the EGFR– group (Z=–2.869, P=0.004). Vascular convergence (P=0.047), GGO component (P=0.007), pleural retraction (P=0.001), air bronchogram (P=0.01), and lobulation (P=0.018) were significantly more common in the EGFR+ group than in the EGFR– group. However, spiculation (P=0.096), cavitation/bubble-like lucency (P=0.113), and pleural effusion (P=0.504) demonstrated no correlation with EGFR mutation status.
The AUC values of the significant variables ranged from 0.545–0.682, which indicated a moderate predictive efficacy for EGFR mutation status (Figure 1A-1C). The AUC (95% CI), sensitivity, specificity, and optimal cut-off values are detailed in Table 2.
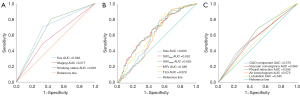
Table 2
Variable | Optimal cut-off value | AUC (95% CI) | P value | Sensitivity | Specificity |
---|---|---|---|---|---|
Sex | – | 0.682 (0.618–0.746) | <0.001 | 0.699 | 0.664 |
Smoking status | – | 0.681 (0.617–0.745) | <0.001 | 0.804 | 0.557 |
Staging | – | 0.577 (0.509–0.644) | 0.028 | 0.49 | 0.664 |
GGO component | – | 0.575 (0.507–0.642) | 0.035 | 0.371 | 0.777 |
Vascular convergence | – | 0.560 (0.492–0.628) | 0.087 | 0.524 | 0.595 |
Pleural retraction | – | 0.595 (0.527–0.662) | 0.006 | 0.685 | 0.508 |
Air bronchogram | – | 0.575 (0.508–0.643) | 0.034 | 0.441 | 0.708 |
Lobulation | – | 0.545 (0.477–0.614) | 0.191 | 0.93 | 0.162 |
Size (cm) | 4.18 | 0.600 (0.532–0.669) | 0.004 | 0.888 | 0.359 |
SUVmax | 12.82 | 0.652 (0.587–0.717) | <0.001 | 0.671 | 0.565 |
SUVmean | 9.23 | 0.655 (0.590–0.720) | <0.001 | 0.783 | 0.473 |
MTV (cm3) | 17.72 | 0.586 (0.517–0.655) | 0.014 | 0.923 | 0.321 |
TLG (g) | 76.93 | 0.629 (0.562–0.696) | <0.001 | 0.832 | 0.443 |
PET/CT, positron emission tomography/computed tomography; EGFR, epidermal growth factor receptor; LADC, lung adenocarcinoma; GGO, ground-glass opacity; SUVmax, maximum standardized uptake value; SUVmean, mean standardized uptake value; MTV, metabolic tumor volume; TLG, total lesion glycolysis; AUC, area under the curve; CI, confidence interval.
Multivariable analysis of independent predictive factors for EGFR mutation status and the establishment and evaluation of the nomogram prediction model
As shown in Table 3, female sex (OR: 2.64; 95% CI: 1.29–5.45), never smoking (OR: 2.78; 95% CI: 1.30–5.88), an SUVmean ≤9.23 (OR: 2.44; 95% CI: 1.35–4.55), MTV ≤17.72 cm3 (OR: 5.00; 95% CI: 2.38–12.50), and pleural retraction (OR: 1.88; 95% CI: 1.05–3.40) were demonstrated to be independent predictors of EGFR mutations in patients with LADCs. A nomogram to predict the probability of EGFR mutation was established by combining these variables (Figure 2). Of the predictors in the nomogram, MTV had the highest score, corresponding to 100 points on the point scale axis, followed by smoking history, sex, SUVmean, and pleural retraction with scores of 62.5, 60, 55, and 38.8, respectively. The probability of EGFR mutation could be easily estimated by projecting the cumulative point score of the five variables to the probability axis. Leave-one-out cross-validation of the nomogram prediction model showed an accuracy of 73.0% and a Kappa value of 0.457. The ROC curve of the nomogram (Figure 3) revealed a good diagnostic performance, with an AUC value of 0.805 (95% CI: 0.753–0.857), a sensitivity of 90.2%, and a specificity of 59.5%, which were higher than those for any single variable. The calibration curve (Figure 4) and Hosmer-Lemeshow test showed that the nomogram had good calibration (χ2=9.752, P=0.283). The decision curve (Figure 5) of the nomogram presented a good net benefit when the threshold probability ranged from 0.1 to 0.85.
Table 3
Variable (reference) | Univariate analysis | Multivariate analysis | |||
---|---|---|---|---|---|
OR (95% CI) | P value | OR (95% CI) | P value | ||
Sex (female) | 2.77 (1.32–5.90) | 0.007 | 2.64 (1.29–5.45) | 0.008 | |
Smoking status (never) | 2.78 (1.30–5.88) | 0.009 | 2.78 (1.30–5.88) | 0.008 | |
Staging (I–II) | 1.49 (0.75–2.94) | 0.256 | – | – | |
GGO component (presence) | 0.70 (0.31–1.57) | 0.393 | – | – | |
Vascular convergence (presence) | 1.16 (0.61–2.20) | 0.644 | – | – | |
Pleural retraction (presence) | 1.73 (0.87–3.43) | 0.109 | 1.88 (1.05–3.40) | 0.034 | |
Air bronchogram (presence) | 1.13 (0.55–2.30) | 0.738 | – | – | |
Lobulation (presence) | 1.16 (0.43–3.17) | 0.775 | – | – | |
Size (≤4.18 cm) | 1.54 (0.52–4.55) | 0.432 | – | – | |
SUVmax (≤12.82) | 1.29 (0.49–3.60) | 0.613 | – | – | |
SUVmean (≤9.23) | 2.78 (1.01–8.33) | 0.050 | 2.44 (1.35–4.55) | 0.004 | |
MTV (≤17.72 cm3) | 4.00 (1.27–12.50) | 0.019 | 5.00 (2.38–12.50) | <0.001 | |
TLG (≤76.93 g) | 1.04 (0.40–2.77) | 0.940 | – | – |
EGFR, epidermal growth factor receptor; LADC, lung adenocarcinoma; GGO, ground-glass opacity; SUVmax, maximum standardized uptake value; SUVmean, mean standardized uptake value; MTV, metabolic tumor volume; TLG, total lesion glycolysis; OR, odds ratio; CI, confidence interval.
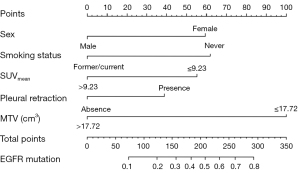
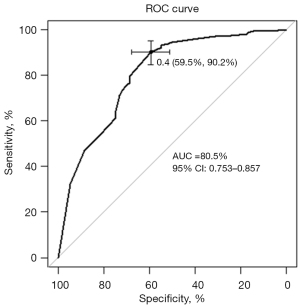
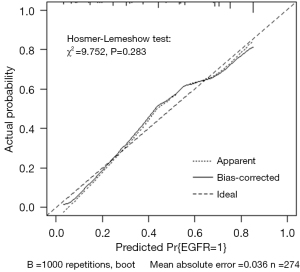
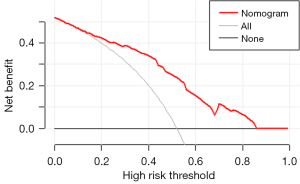
Discussion
In this research, we explored the relationship of clinical, PET, and CT features with EGFR mutation status in patients with LADC. In our cohort of 274 Asian patients, the EGFR mutation rate was as high as 52.2%, which is in accordance with rates reported in previous studies (9,13) and indicates an ethnic tendency of EGFR mutations in LADC. It has been reported that EGFR mutations occur in 30–40% of Asian patients with LADC, compared to only 15% of Caucasian patients (22). We also found that the EGFR mutation rate was significantly higher in never-smokers and in females, with rates of 66.5% and 69.4%, respectively. Epidemiological data from East Asian patients demonstrated that the morbidity of lung cancer in never-smokers was high and that almost 90% of never-smokers with LADCs had single oncogenic mutations, with the majority of these being EGFR mutations (23). According to another epidemiological study, the incidence rate of NSCLC among never-smokers was 53% in women and only 15% in men, with LADC being the most common histological type (24). According to these data and our results, Asian ethnicity, female sex, and never smoking are strong predictors of EGFR mutations in patients with LADC.
Several studies (9-13) have reported connections between EGFR gene phenotypes and PET metabolic or volumetric parameters of LADCs, such as the SUVmax, SUVmean, MTV, and TLG, among which the SUVmax is the most studied. Most of the previous studies reported that these parameters were significantly lower in EGFR-mutated LADCs than in EGFR wild-type LADCs, which is consistent with our results; however, contrasting results still exist. For example, Ko et al. (12), Kanmaz et al. (25), and Huang et al. (26) all found that a higher SUVmax served as a predictor of the presence of EGFR mutation, but Liao et al. (19), Liu et al. (27), and Ishimura et al. (28) concluded that the SUVmax was not significantly associated with EGFR mutation status. The deviation in these results may stem from differences in the study sample sizes and compositions, such as differences in the proportions of patients with various TNM stages or in the target disease (NSCLC vs. LADC). Recent research from Chen et al. (29) validated the underlying mechanism of how EGFR mutations affect FDG uptake; they found that the expression of NADPH oxidase 4 downregulated reactive oxygen species activity in EGFR-mutant lung cancer cells, leading to a decrease in total glucose transporter 1 expression and a subsequent decline in FDG uptake. The heterogeneity parameters COV and HI are measures of the gray-level dispersion within the VOI, which represents the global heterogeneity. In patients with locally advanced NSCLC, Dong et al. (30) reported that the COV change during concurrent cisplatin-based chemotherapy could be a valuable parameter of treatment response and survival. Another study from Pahk et al. (20) demonstrated that the diagnostic performance of COV was superior to that of other metabolic parameters in predicting pathological N2 lymph node metastasis in patients with NSCLC. However, few studies have investigated the correlation between intratumoral heterogeneity measured by COV or HI and EGFR mutations in LADC, and the results of our research revealed no relationship between them. In future studies, more dedicated and sophisticated parameters that can reflect metabolic heterogeneity should be explored and validated.
The advances in integrating CT equipment within PET/CT scanners have led to the possibility of identifying CT features more clearly, which would aid the diagnostic accuracy for patients with LADC. Several reports have shown an association between CT imaging features and EGFR mutations. Liu et al. (9) quantified 30 detailed features from the CT images of 385 patients with LADCs and discovered that tumors with a smaller size, spiculation, a GGO component, an air bronchogram, bubble-like lucency, vascular convergence, thickened adjacent bronchovascular bundles, and pleural retraction tended to be EGFR mutated. Another study from Rizzo et al. (31) reported that EGFR mutations were associated with air bronchogram, pleural retraction, small lesion size, and the absence of fibrosis in NSCLC. The results of a meta-analysis (15) of 17 original articles showed that the risk factors for EGFR mutations in NSCLC were GGOs, air bronchogram, pleural retraction, and vascular convergence. In the current study, we extracted nine CT features and found that a smaller tumor size, the presence of GGOs, air bronchogram, pleural retraction, lobulation, and vascular convergence were associated with EGFR mutations in LADC, which essentially concords with the findings of previous studies.
In our study, the ROC curves showed a modest prognostic performance in differentiating EGFR-mutant from wild-type LADCs for each significant variable alone, with AUC values ranging from 0.546 to 0.682. Our findings are similar to the results of the aforementioned meta-analysis (14), which reported that the summary AUCs of the SUVmax and SUVmean were 0.69 (95% CI: 0.65–0.73) and 0.68 (95% CI: 0.64–0.72), respectively. To promote the application of PET/CT and enhance predictive ability for patients with LADCs, we developed a logistic regression model and visualized it as a nomogram. The risk-score model consisting of five independent risk factors (MTV, smoking history, sex, SUVmean, and pleural retraction) showed a better predictive performance than any of the other variables alone, with an AUC of 0.805 (95% CI: 0.753–0.857). This nomogram, as a simple and user-friendly medical tool, could generate the probability of a given patient with LADC having an EGFR mutation on the basis of individualized clinical and PET/CT imaging features. For patients with LADC who do not have results of gene analysis, our nomogram could serve as a pretreatment tool for predicting EGFR mutation status and thus guide the use of TKI therapy.
Recently, radiomics and deep-learning methods based on PET/CT imaging have been used to evaluate EGFR mutation status. In a study by Zhang et al. (17), two PET and four CT radiomic features were selected to build a predictive model that had an AUC of 0.769. Chang et al. (16) constructed a combined PET/CT radiomics-clinical model with an AUC of 0.84. In another study conducted by Yin et al. (18), a PET/CT model based on a deep-learning system had the potential to help select suitable patients for EGFR-TKIs, with an AUC of 0.84. Compared to artificial intelligence-based models, our model is more accessible and convenient for use in clinical practice and has a comparable performance in the prediction of EGFR-mutated LADCs.
The current study had some limitations. First, it was a retrospective study with a relatively small sample size in a single center, which made selection bias somewhat unavoidable. Second, some clinical parameters, such as tumor markers, were not included because some patient information was not available. Third, the metabolic parameters of the SUVmean and MTV were delineated according to the threshold of 40% of the SUVmax. However, other methods were also used, such as a fixed SUV threshold of 2.5 (32) or 25–75% (33) of the SUVmax, which might have resulted in different conclusions. Fourth, intratumoral heterogeneity could be better identified quantitatively with the addition of continuous tone image texture features. Finally, due to the small sample size, we only performed internal validation for the diagnostic efficacy of the model. In further research, a testing cohort should be enrolled for external validation to obtain a more convincing result.
In conclusion, based on the results of our study, the clinical characteristics of female sex and never-smoking status and the PET/CT imaging parameters/features of the SUVmean, the MTV, and the presence of pleural retraction are predictive factors for LADCs harboring EGFR mutations. Our nomogram prediction model combining these variables could be a noninvasive, easy-to-use, and practical method of selecting patients with LADC who are suitable for EGFR-TKI treatment.
Acknowledgments
The authors express enormous appreciation and gratitude to all the participants from the Department of Nuclear Medicine, Pathology at Beijing Hospital.
Funding: This work was supported by National High Level Hospital Clinical Research Funding (No. BJ-2021-186).
Footnote
Reporting Checklist: The authors have completed the TRIPOD reporting checklist. Available at https://qims.amegroups.com/article/view/10.21037/qims-22-248/rc
Conflicts of Interest: All authors have completed the ICMJE uniform disclosure form (available at https://qims.amegroups.com/article/view/10.21037/qims-22-248/coif). The authors have no conflicts of interest to declare.
Ethical Statement: The authors are accountable for all aspects of the work in ensuring that questions related to the accuracy or integrity of any part of the work are appropriately investigated and resolved. The study was conducted in accordance with the Declaration of Helsinki (as revised in 2013). This retrospective research was conducted under the approval of the Ethics Committee of Beijing Hospital, and the requirement to obtain individual consent for this retrospective analysis was waived.
Open Access Statement: This is an Open Access article distributed in accordance with the Creative Commons Attribution-NonCommercial-NoDerivs 4.0 International License (CC BY-NC-ND 4.0), which permits the non-commercial replication and distribution of the article with the strict proviso that no changes or edits are made and the original work is properly cited (including links to both the formal publication through the relevant DOI and the license). See: https://creativecommons.org/licenses/by-nc-nd/4.0/.
References
- Succony L, Rassl DM, Barker AP, McCaughan FM, Rintoul RC. Adenocarcinoma spectrum lesions of the lung: Detection, pathology and treatment strategies. Cancer Treat Rev 2021;99:102237. [Crossref] [PubMed]
- Khaddour K, Jonna S, Deneka A, Patel JD, Abazeed ME, Golemis E, Borghaei H, Boumber Y. Targeting the Epidermal Growth Factor Receptor in EGFR-Mutated Lung Cancer: Current and Emerging Therapies. Cancers (Basel) 2021;13:3164. [Crossref] [PubMed]
- Ettinger DS, Wood DE, Aisner DL, Akerley W, Bauman JR, Bharat A, et al. NCCN Guidelines Insights: Non-Small Cell Lung Cancer, Version 2.2021. J Natl Compr Canc Netw 2021;19:254-66. [Crossref] [PubMed]
- Davies RS, Smith C, Edwards G, Butler R, Parry D, Lester JF. Impact of Cytological Sampling on EGFR Mutation Testing in Stage III-IV Lung Adenocarcinoma. Lung Cancer Int 2017;2017:9614938. [Crossref] [PubMed]
- Soria JC, Ohe Y, Vansteenkiste J, Reungwetwattana T, Chewaskulyong B, Lee KH, et al. Osimertinib in Untreated EGFR-Mutated Advanced Non-Small-Cell Lung Cancer. N Engl J Med 2018;378:113-25. [Crossref] [PubMed]
- Liam CK, Andarini S, Lee P, Ho JC, Chau NQ, Tscheikuna J. Lung cancer staging now and in the future. Respirology 2015;20:526-34. [Crossref] [PubMed]
- Shao X, Shao X, Niu R, Jiang Z, Xu M, Wang Y. Investigating the association between ground-glass nodules glucose metabolism and the invasive growth pattern of early lung adenocarcinoma. Quant Imaging Med Surg 2021;11:3506-17. [Crossref] [PubMed]
- Wang L, Li T, Hong J, Zhang M, Ouyang M, Zheng X, Tang K. 18F-FDG PET-based radiomics model for predicting occult lymph node metastasis in clinical N0 solid lung adenocarcinoma. Quant Imaging Med Surg 2021;11:215-25. [Crossref] [PubMed]
- Liu Y, Kim J, Qu F, Liu S, Wang H, Balagurunathan Y, Ye Z, Gillies RJ. CT Features Associated with Epidermal Growth Factor Receptor Mutation Status in Patients with Lung Adenocarcinoma. Radiology 2016;280:271-80. [Crossref] [PubMed]
- Qiu X, Yuan H, Sima B. Relationship between EGFR mutation and computed tomography characteristics of the lung in patients with lung adenocarcinoma. Thorac Cancer 2019;10:170-4. [Crossref] [PubMed]
- Hong IK, Lee JM, Hwang IK, Paik SS, Kim C, Lee SH. Diagnostic and Predictive Values of 18F-FDG PET/CT Metabolic Parameters in EGFR-Mutated Advanced Lung Adenocarcinoma. Cancer Manag Res 2020;12:6453-65. [Crossref] [PubMed]
- Ko KH, Hsu HH, Huang TW, Gao HW, Shen DH, Chang WC, Hsu YC, Chang TH, Chu CM, Ho CL, Chang H. Value of 18F-FDG uptake on PET/CT and CEA level to predict epidermal growth factor receptor mutations in pulmonary adenocarcinoma. Eur J Nucl Med Mol Imaging 2014;41:1889-97. [Crossref] [PubMed]
- Yang B, Wang QG, Lu M, Ge Y, Zheng YJ, Zhu H, Lu G. Correlations Study Between 18F-FDG PET/CT Metabolic Parameters Predicting Epidermal Growth Factor Receptor Mutation Status and Prognosis in Lung Adenocarcinoma. Front Oncol 2019;9:589. [Crossref] [PubMed]
- Guo Y, Zhu H, Yao Z, Liu F, Yang D. The diagnostic and predictive efficacy of 18F-FDG PET/CT metabolic parameters for EGFR mutation status in non-small-cell lung cancer: A meta-analysis. Eur J Radiol 2021;141:109792. [Crossref] [PubMed]
- Zhang H, Cai W, Wang Y, Liao M, Tian S. CT and clinical characteristics that predict risk of EGFR mutation in non-small cell lung cancer: a systematic review and meta-analysis. Int J Clin Oncol 2019;24:649-59. [Crossref] [PubMed]
- Chang C, Zhou S, Yu H, Zhao W, Ge Y, Duan S, Wang R, Qian X, Lei B, Wang L, Liu L, Ruan M, Yan H, Sun X, Xie W. A clinically practical radiomics-clinical combined model based on PET/CT data and nomogram predicts EGFR mutation in lung adenocarcinoma. Eur Radiol 2021;31:6259-68. [Crossref] [PubMed]
- Zhang M, Bao Y, Rui W, Shangguan C, Liu J, Xu J, Lin X, Zhang M, Huang X, Zhou Y, Qu Q, Meng H, Qian D, Li B. Performance of 18F-FDG PET/CT Radiomics for Predicting EGFR Mutation Status in Patients With Non-Small Cell Lung Cancer. Front Oncol 2020;10:568857. [Crossref] [PubMed]
- Yin G, Wang Z, Song Y, Li X, Chen Y, Zhu L, Su Q, Dai D, Xu W. Prediction of EGFR Mutation Status Based on 18F-FDG PET/CT Imaging Using Deep Learning-Based Model in Lung Adenocarcinoma. Front Oncol 2021;11:709137. [Crossref] [PubMed]
- Liao X, Cui Y, Chen X, Di L, Tong Z, Liu M, Wang R. Primary metabolic tumor volume from 18F-FDG PET/CT associated with epidermal growth factor receptor mutation in lung adenocarcinoma patients. Nucl Med Commun 2020;41:1210-7. [Crossref] [PubMed]
- Pahk K, Chung JH, Yi E, Kim S, Lee SH. Metabolic tumor heterogeneity analysis by F-18 FDG PET/CT predicts mediastinal lymph node metastasis in non-small cell lung cancer patients with clinically suspected N2. Eur J Radiol 2018;106:145-9. [Crossref] [PubMed]
- Yang Z, Sun Y, Xu X, Zhang Y, Zhang J, Xue J, Wang M, Yuan H, Hu S, Shi W, Zhu B, Zhang Y. The Assessment of Estrogen Receptor Status and Its Intratumoral Heterogeneity in Patients With Breast Cancer by Using 18F-Fluoroestradiol PET/CT. Clin Nucl Med 2017;42:421-7. [Crossref] [PubMed]
- Shi Y, Au JS, Thongprasert S, Srinivasan S, Tsai CM, Khoa MT, Heeroma K, Itoh Y, Cornelio G, Yang PC. A prospective, molecular epidemiology study of EGFR mutations in Asian patients with advanced non-small-cell lung cancer of adenocarcinoma histology (PIONEER). J Thorac Oncol 2014;9:154-62. [Crossref] [PubMed]
- Zhou F, Zhou C. Lung cancer in never smokers-the East Asian experience. Transl Lung Cancer Res 2018;7:450-63. [Crossref] [PubMed]
- Wakelee HA, Chang ET, Gomez SL, Keegan TH, Feskanich D, Clarke CA, Holmberg L, Yong LC, Kolonel LN, Gould MK, West DW. Lung cancer incidence in never smokers. J Clin Oncol 2007;25:472-8. [Crossref] [PubMed]
- Kanmaz ZD, Aras G, Tuncay E, Bahadır A, Kocatürk C, Yaşar ZA, Öz B, Özkurt CÜ, Gündoğan C, Çermik TF. Contribution of 18Fluorodeoxyglucose positron emission tomography uptake and TTF-1 expression in the evaluation of the EGFR mutation in patients with lung adenocarcinoma. Cancer Biomark 2016;16:489-98. [Crossref] [PubMed]
- Huang CT, Yen RF, Cheng MF, Hsu YC, Wei PF, Tsai YJ, Tsai MF, Shih JY, Yang CH, Yang PC. Correlation of F-18 fluorodeoxyglucose-positron emission tomography maximal standardized uptake value and EGFR mutations in advanced lung adenocarcinoma. Med Oncol 2010;27:9-15. [Crossref] [PubMed]
- Liu A, Han A, Zhu H, Ma L, Huang Y, Li M, Jin F, Yang Q, Yu J. The role of metabolic tumor volume (MTV) measured by 18F FDG PET/CT in predicting EGFR gene mutation status in non-small cell lung cancer. Oncotarget 2017;8:33736-44. [Crossref] [PubMed]
- Ishimura M, Norikane T, Mitamura K, Yamamoto Y, Arai-Okuda H, Murota M, Ibuki E, Kanaji N, Nishiyama Y. Correlation of epidermal growth factor receptor mutation status and PD-L1 expression with 18FFDG PET using volume-based parameters in non-small cell lung cancer. Nucl Med Commun 2022;43:304-9. [Crossref] [PubMed]
- Chen L, Zhou Y, Tang X, Yang C, Tian Y, Xie R, Chen T, Yang J, Jing M, Chen F, Wang C, Sun H, Huang Y. EGFR mutation decreases FDG uptake in non-small cell lung cancer via the NOX4/ROS/GLUT1 axis. Int J Oncol 2019;54:370-80. [PubMed]
- Dong X, Sun X, Sun L, Maxim PG, Xing L, Huang Y, Li W, Wan H, Zhao X, Xing L, Yu J. Early Change in Metabolic Tumor Heterogeneity during Chemoradiotherapy and Its Prognostic Value for Patients with Locally Advanced Non-Small Cell Lung Cancer. PLoS One 2016;11:e0157836. [Crossref] [PubMed]
- Rizzo S, Petrella F, Buscarino V, De Maria F, Raimondi S, Barberis M, Fumagalli C, Spitaleri G, Rampinelli C, De Marinis F, Spaggiari L, Bellomi M. CT Radiogenomic Characterization of EGFR, K-RAS, and ALK Mutations in Non-Small Cell Lung Cancer. Eur Radiol 2016;26:32-42. [Crossref] [PubMed]
- Yip SS, Kim J, Coroller TP, Parmar C, Velazquez ER, Huynh E, Mak RH, Aerts HJ. Associations Between Somatic Mutations and Metabolic Imaging Phenotypes in Non-Small Cell Lung Cancer. J Nucl Med 2017;58:569-76. [Crossref] [PubMed]
- Ma W, Wang M, Li X, Huang H, Zhu Y, Song X, Dai D, Xu W. Quantitative 18F-FDG PET analysis in survival rate prediction of patients with non-small cell lung cancer. Oncol Lett 2018;16:4129-36. [Crossref] [PubMed]