The spectrum of magnetic resonance imaging proton density fat fraction (MRI-PDFF), magnetic resonance spectroscopy (MRS), and two different histopathologic methods (artificial intelligence vs. pathologist) in quantifying hepatic steatosis
Introduction
Nonalcoholic fatty liver disease (NAFLD) is one of the most common causes of chronic liver disease, and its prevalence has been increasing due to the increasing number of people with obesity and type 2 diabetes mellitus (1). NAFLD is defined as the presence of steatosis in >5% of hepatocytes without secondary causes of hepatic fat accumulation (e.g., significant alcohol consumption and steatogenic drugs) (2). In NAFLD, the steatosis is primarily macrovesicular steatosis, which is characterized by large fat droplets occupying the cytoplasm of hepatocytes and displacing the nucleus to the periphery (3). In contrast, in microvesicular steatosis, hepatocytes are filled with numerous smaller fat droplets (foamy appearance) with centrally located nucleus (3). NAFLD represents a spectrum of liver diseases, ranging from simple steatosis to nonalcoholic steatohepatitis (NASH), fibrosis, and cirrhosis (4). NASH is characterized by steatosis, lobular inflammation, and hepatocyte ballooning with or without fibrosis (4). The degrees of NASH components are graded according to the NASH Clinical Research Network (NASH-CRN) scoring system (5). The degree of steatosis is assessed semiquantitatively and visually by estimating the percentage of hepatocytes containing macrovesicular fat droplets (5).
Although assessments by pathologists using the NASH-CRN scoring system are widely used in grading hepatic steatosis, it has some drawbacks (6,7). First, steatosis grades are expressed as discrete values, not continuous values. Second, there could be inter- and intra-observer variability in the pathologists’ grading. Therefore, several studies automatically measured the hepatic steatosis on liver biopsy specimens using machine learning and expressed it as a continuous value to reduce the variability (6-8). However, the deep learning method also requires a percutaneous biopsy to obtain hepatic tissues, and ultimately has the disadvantage of involving an invasive procedure.
Among various noninvasive imaging modalities used for the hepatic fat assessment, magnetic resonance imaging (MRI) and MR spectroscopy (MRS) are the most accurate methods, as they directly measure the proton signals in water and fat (9). MRS is considered as the method of choice to measure hepatic fat noninvasively (10-12). Several MRI-based methods (e.g., Dixon technique) have been introduced for the measurement of proton density fat fraction (PDFF) and are more widely available than MRS (13-17).
In some previous studies using MRS and MRI-PDFF, hepatic fat vacuoles on liver biopsy specimens were segmented in a semiquantitative method to measure fat fraction (FF) (18-20). The FFs measured by this method demonstrated a good correlation with MRS and MRI-PDFF as well as the conventional method by pathologists in which the percentages of hepatocytes including fat were assessed (18-20). In daily clinical practice, we found that there was no perfect agreement between the FF measured by MRS (FFMRS) and MRI-PDFF for quantifying the hepatic steatosis. Therefore, in this study, we planned to measure FF using a fully automated deep learning method and systemically compare MRS, MRI-PDFF, and two difference histopathologic methods.
This study aimed (I) to provide a spectrum of values of MRI-PDFF, FF measured by MRS (FFMRS), and FFs measured by two different histopathologic methods [artificial intelligence (AI) and pathologist], (II) to evaluate the correlation among them, and (III) to evaluate the diagnostic performance of MRI-PDFF and MRS in grading hepatic steatosis. We present the following article in accordance with the STARD reporting checklist (available at https://qims.amegroups.com/article/view/10.21037/qims-22-393/rc).
Methods
Patients
The study was conducted in accordance with Declaration of Helsinki (as revised in 2013). This retrospective Health Insurance Portability and Accountability Act (HIPAA)-compliant study was approved by the institutional review board (IRB) of the Korea University Guro Hospital (Approval No. KUGH16184), and the requirement for an informed consent was waived. This study included 47 consecutively recruited patients diagnosed with NAFLD/NASH by liver biopsy in our previous study (21). All patients did not have any other known causes of chronic liver disease such as chronic hepatitis B or C, autoimmune hepatitis, and primary sclerosing cholangitis. Patients with use of steatogenic medications within the past 6 months, significant alcohol consumption (more than 70 g/week for women and 140 g/week for men), history of hepatocellular carcinoma, pregnancy, and contraindications to perform MRI were not included. All patients underwent MRI including MRI-PDFF and MRS. Patients with an interval of more than 30 days between liver biopsy and MRI were excluded.
Histopathological evaluation
Ultrasound (US)-guided percutaneous liver biopsy was performed at the right hepatic lobe (segment 5/6) using an 18-gauge semi-automatic needle (TSK Laboratory, Tochigi, Japan). At least two cores of hepatic tissue, each at least 2 cm in length, were obtained. The hepatic tissues were fixed in formalin, embedded in paraffin, and stained with hematoxylin and eosin. The biopsy specimens were reviewed by a pathologist (B.K., with >15 years of experience) who was blinded to the patients’ radiologic and clinical data. Steatosis was graded according to the NAFLD Activity Score (NAS) system (5). For steatosis grades, the percentage of hepatocytes with macrovesicular fat droplets was first determined by visual assessment (FFpathologist). Then, the steatosis grade was assigned as S0 (<5%), S1 (5–33%), S2 (33–66%), and S3 (>66%). In this study, the pathologist separately recorded the percentage of hepatocytes containing macrovesicular fat droplets as a continuous value in units of 5%.
Automatic fat vacuole segmentation on histopathologic slides of liver biopsy samples using a deep learning method
Entire microscope slides of liver biopsy specimens were scanned using an Aperio/Leica CS2 scanner (Leica Microsystems, Wetzlar, Germany). At ×200 magnification, five representative images were selected from each slide by an experienced pathologist who was blinded to the patient’s clinical data and MRI data. These images were used as input data to calculate the percentage of fat vacuoles in the whole area. The characteristics of fat vacuoles were determined using in-house developed processing method. The fat area determination model in pathology image dataset was designed with deep learning-based model with using conventional UNET architecture with minor variations, combined with traditional image processing method. Additional fully-connected layer was adopted in final layer for binarized probability and categorized determination, and custom-designed loss function was adopted for avoiding the false determination of the vessel area. While in the training process, the result of the model was filtered with hessian function to emphasize anisotropic objects such as vessels hessian filtering, and additional post-processing such as analysis using long-axis detection and convex hull algorithm was done. After then, the image results were used to calculate the accuracy and loss for next iteration of the training. The intersection of union (IoU) was used as base loss function.
For initial training of the proposed model, about 32,000 fat area labeled partial pathology image dataset from the liver biopsy samples of NAFLD/NASH patients were used, which was the machine-learning dedicated dataset formerly acquired from the department of pathology, Korea University Guro Hospital. The dataset was retrospectively gathered from the hospital, and the vessels, contaminated tissues, fat area, and major disease category of the biopsy samples were labeled from experienced pathologists. Since the resolution of the pathology image was too high, the images were split into smaller sized tile images. The dataset was separated randomly into training and validation dataset with having a ratio of 8:2, and the augmentation of the dataset was done with random rotation, mirroring, elastic deformation, scaling, etc.
The deep learning models were designed with using Tensorflow library version 1.13.1 (Google, Mountain View, CA, USA), in decent hardware settings with NVIDIA RTX2080Ti GPU card. The training of the models was repeated with 200 epochs, with 0.3 dropout ratio. The accuracy and loss value of the training and validation of the model showed decent result, which were 0.932/0.176 and 0.867/0.266, respectively.
Proposed model was developed for analyzing the characteristics of fat area of the pathology image data, and determine from the input image whether the area includes the fat area or not, and the area includes unnecessary vessel area. During the training of the model, artifactual areas, such as sinusoids (blood vessels), were excluded based on the pathologist’s feedback from the draft result of the base model.
In the image processing phase, a binary image was generated with using gaussian mixture model from the grayscale pixel distribution of the image intensity. With generating a histogram of all grayscale pixel values of the input image, a binary image was obtained by analyzing the distribution of pixels and setting an appropriate threshold between white and black pixels.
The fat vacuole areas of the pathology image were segmented using both methods, additional post-processing was performed through spherical shape analysis using long-axis detection and convex hull algorithm (Figure 1A).
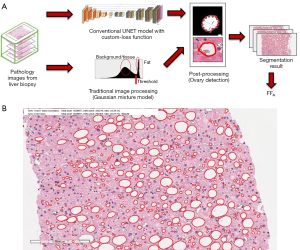
The mean FF values measured in five selected images per patient were used as representative values (Figure 1B). The FFs measured by this automatic fat vacuole segmentation (FFAI) were compared and correlated with FFMRS.
MR examination
All patients underwent MR imaging using a 3 T MR scanner (MAGNETOM Skyra, Siemens Healthineers, Erlangen, Germany). T2-weighted half-Fourier acquisition single-shot turbo spin-echo (HASTE) sequence, three-dimensional T1-weighted gradient-recalled echo volumetric interpolated breath-hold examination (VIBE) sequence, multi-echo (six-echo) modified Dixon (mDixon) gradient echo sequence, MRS, MR elastography, and T1 mapping were performed.
MRS
Single-voxel MRS was performed using a prototypical high-speed T2-corrected multi-echo (HISTO) MR spectroscopic technique, which is a modified stimulated echo acquisition mode (STEAM) sequence. MRS spectra were obtained using the same method described in a previous study (22). HISTO MR spectroscopic technique allows the rapid and simultaneous acquisition of multiple echoes to assess hepatic fat within a single breath hold. This technique uses signal integrals from water and lipid spectrum fits to estimate T2 decay and assess the equilibrium signal at TE of 0 ms (23). A single voxel (20×20×20 mm) was placed in the right hepatic lobe (segment 5/6) by an experienced technologist (>10 years of experience in MRS) while avoiding large bile ducts, vessels, and focal hepatic lesions. The technologist tried to locate the voxel at the same position of the liver biopsy site (segment 5/6). The parameters included five echo times (TEs) (12, 24, 36, 48, and 72 ms); repetition time (TR), 3,000 ms; mixing time, 10 ms; and flip angle (FA), 90°. Each MRS acquisition was performed within 15 s during one breath hold. This process was repeated three times. MRS data post-processing was performed using an inline software of the MR vendor.
MRI-PDFF
An axial three-dimensional multi-echo (six-echo) mDixon gradient echo sequence was also acquired for hepatic PDFF measurement within 16 s during one breath hold. The parameters included six TEs (1.23, 2.46, 3.69, 4.92, 6.15, and 7.38 ms); TR, 9.0 ms; FA, 4°; field of view, 350 mm; matrix size, 95×160; slice thickness, 3.5 mm; parallel imaging factor of 2×2; and spatial resolution of 2×2×2 mm3. The short TR and small FA were used to minimize the T1 bias and T2* effect. A Levenberg Marquardt nonlinear fitting was used to fit the complex signal magnitudes of multiple echo data. Inline reconstruction was performed by addressing confounding factors, including field inhomogeneity, eddy currents, T1 bias, T2* decay, and spectral complexity. MRI-PDFF, water fraction, R2* map, T2* map, and goodness-of-fit images were automatically generated based on pixel-by-pixel fitting (24).
Image analysis
- FF measurement on MRS: liver FFs were calculated automatically and displayed as a percentage (%). Mean FF values were used as representative values (Figure 2A).
- PDFF measurement on mDixon gradient echo sequence: two radiologists (JWK and CHL, with 9 and 28 years of experience in abdominal radiology, respectively) who were blinded to the pathologic results performed PDFF measurement. A circular region of interest (ROI) was drawn in the right hepatic lobe (segment 5/6) at the same location as that in MRS while avoiding large bile ducts, vessels, and focal hepatic lesions. The circular ROIs were colocalized to the MRS voxel locations on three consecutive MRI-PDFF images. The two radiologists measured the ROI three times each, and the mean values were used as representative values (Figure 2B).
Statistical analysis
The interobserver agreement of the two radiologists for PDFF measurement was assessed using κ-statistics, with κ-values graded as excellent (0.81–1.0), good (0.61–0.80), moderate (0.41–0.60), fair (0.21–0.40), and poor (0–0.20).
The mean FF values are presented as mean ± standard deviation (SD). The mean values of FFMRS, MRI-PDFF, FFAI, and FFpathologist were compared using paired t-tests. Correlations between MRI-PDFF and MRS, between two different histopathologic methods, and between MRI-PDFF/MRS and each histopathologic method were assessed using Pearson correlation coefficient (r), which was graded as very weak (0.1–0.19), weak (0.20–0.39), moderate (0.40–0.59), strong (0.60–0.79), and very strong (0.80–1.00). The agreement between MRI-PDFF and MRS was assessed by Bland Altman analysis, and the 95% limit of agreement (LOA) was calculated.
To evaluate the diagnostic performance of MRI-PDFF and MRS for grading hepatic steatosis, receiver operating characteristic curve analyses were performed and the areas under the curve (AUCs) were obtained with their 95% confidence intervals (CIs). The optimal cutoff values representing to the maximal sum of the sensitivity and specificity were also determined. The DeLong test was used to compare AUCs of MRI-PDFF and MRS.
A P value <0.05 was considered statistically significant. All statistical analyses were performed using commercially available software programs, SPSS version 27.0 (IBM Corp., Armonk, NY, USA) and MedCalc version 20.009 (MedCalc Software, Ostend, Belgium).
Results
Patients
Forty-seven patients (16 men and 31 women; mean age, 51.0±12.7 years; range, 19–75 years) were included. Based on histopathological evaluation, steatosis was graded as S0 (n=0), S1 (n=25), S2 (n=18), or S3 (n=4). The clinical and laboratory data are summarized in Table 1 and the distribution of histopathologic grade is presented in Table 2. The mean interval between liver biopsy and MRI was 21.0±4.5 days (range, 1–27 days).
Table 1
Variables | Total patients (n=47) |
---|---|
Age (years) | 51.0±12.7 |
Male : female | 16 (34.0) : 31 (66.0) |
Body mass index (kg/m2) | 28.3±6.2 |
ALT (IU/L) | 80.2±43.1 |
AST (IU/L) | 59.6±26.5 |
ALP (IU/L) | 88.2±21.3 |
GGT (IU/L) | 79.0±61.1 |
Total bilirubin (mg/dL) | 0.60±0.29 |
Total cholesterol (mg/dL) | 181.9±36.4 |
Triglycerides (mg/dL) | 154.9±65.3 |
HDL-cholesterol (mg/dL) | 43.5±11.1 |
LDL-cholesterol (mg/dL) | 112.6±33.2 |
Fasting glucose (mg/dL) | 117.0±32.4 |
Albumin (g/dL) | 4.15±0.62 |
Platelet count (×103/L) | 207.8±54.1 |
Type 2 diabetes mellitus | 28 (59.6) |
Continuous variables are presented as mean ± standard deviation and categorical variables are presented as numbers (%). ALT, alanine aminotransferase; AST, aspartate aminotransferase; ALP, alkaline phosphatase; GGT, γ-glutamyltransferase; HDL, high density lipoprotein; LDL, low density lipoprotein.
Table 2
Variables | Grade | Total patients (n=47) |
---|---|---|
Steatosis | S0 | 0 (0) |
S1 | 25 (53.2) | |
S2 | 18 (38.3) | |
S3 | 4 (8.5) | |
Fibrosis | F0 | 13 (27.6) |
F1 | 13 (27.6) | |
F2 | 13 (27.6) | |
F3 | 6 (12.8) | |
F4 | 2 (4.4) | |
Lobular inflammation | L0 | 0 (0) |
L1 | 17 (36.2) | |
L2 | 28 (59.5) | |
L3 | 2 (4.3) | |
Ballooning degeneration | B0 | 26 (55.3) |
B1 | 11 (23.4) | |
B2 | 10 (21.3) |
Variables are presented as numbers (%).
Spectrum of values of MRI-PDFF, FFMRS, and FFs measured by two different histopathologic methods
Interobserver agreement for the measurement of PDFF was excellent (κ=0.998; 95% CI, 0.997–0.999, P<0.001). The means ± SD of MRI-PDFF, FFMRS, FFpathologist, and FFAI were 12.04±6.37, 14.01±6.16, 34.26±19.69, and 6.79±4.37 (%), respectively (Figure 3). The values of MRI-PDFF and FFMRS were significantly higher than those of FFAI and significantly lower than those of FFpathologist. Except for two cases (95.7%, 45/47), FFMRS always showed a higher value than MRI-PDFF. The Bland Altman bias [mean of MRS – (MRI-PDFF) differences] was 2.06% (95% LOA, −0.213%, 4.328%) (Figure 4).
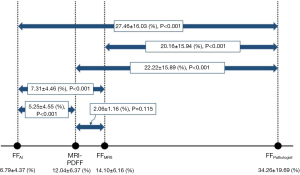
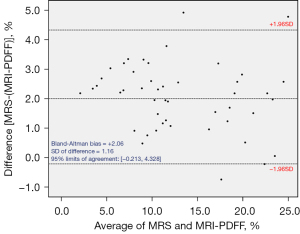
Correlation
MRI-PDFF and MRS showed a very strong correlation (r=0.983, P<0.001). The two different histopathologic methods also showed a very strong correlation (r=0.872, P<0.001). For FFpathologist, both MRI-PDFF and MRS demonstrated a strong correlation (r=0.701, P<0.001 and r=0.709, P<0.001, respectively). For FFAI, both MRI-PDFF and MRS also demonstrated a strong correlation (r=0.700, P<0.001 and r=0.690, P<0.001, respectively) (Figure 5).
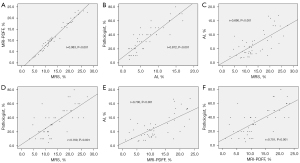
Diagnostic performance of MRS and MRI-PDFF in grading hepatic steatosis
The AUCs of MRS for grading ≥S2 and ≥S3 steatosis were 0.860 and 0.878, respectively. The AUCs of MRI-PDFF for grading ≥S2 and ≥S3 steatosis were 0.846 and 0.855, respectively (Table 3). MRS showed significantly higher diagnostic performance than MRI-PDFF in grading ≥S3 steatosis (P=0.038), while there was no significant difference in grading ≥S2 steatosis (P=0.236).
Table 3
Variables | Grade | AUC | Cut-off value (%) | Sensitivity (%) | Specificity (%) |
---|---|---|---|---|---|
MRS | ≥S2 | 0.860 (0.728–0.944) | 12.7 | 81.8 (59.7–94.8) | 92.0 (74.0–99.0) |
≥S3 | 0.878 (0.749–0.955) | 18.9 | 100.0 (39.8–100.0) | 81.4 (66.6–91.6) | |
MRI-PDFF | ≥S2 | 0.846 (0.711–0.935) | 10.9 | 77.3 (54.6–92.2) | 88.0 (68.8–97.5) |
≥S3 | 0.855 (0.721–0.940) | 16.2 | 100.0 (39.8–100.0) | 76.7 (61.4–88.2) |
Data in brackets are 95% confidence intervals. MRS, magnetic resonance spectroscopy; MRI-PDFF, magnetic resonance imaging-proton density fat fraction; AUC, area under the curve.
Discussion
Our study demonstrated the spectra of FFMRS, MRI-PDFF, FFAI, and FFpathologist. The FFs measured by MRI (FFMRS and MRI-PDFF) were located between the FFs measured by AI and pathologists (FFAI and FFpathologist). FFMRS and MRI-PDFF were significantly higher than FFAI and significantly lower than FFpathologist. These results were thought to be due to different histopathological methods; FFpathologist corresponds to the proportion of hepatocytes including macrovesicular fat, while FFAI corresponds to the area of macrovesicular fat in the entire area. FFAI was significantly lower than FFMRS and MRI-PDFF. This significant difference may be due to the difficulty in measuring microvesicular fat in FFAI measurement (19). Except for two cases, FFMRS always showed a higher value than MRI-PDFF, with a 2.06% difference.
In this study, the FFs measured by MRS and MRI-PDFF showed a very strong correlation with each other (r=0.983). In several previous studies, MRI-PDFF showed high accuracy compared with MRS and histology (13-16,25,26) and excellent correlation with MRS (15,16,25,26). In a study by Vu et al. (16), MRI-PDFF showed an excellent correlation with MRS (r=0.916), and the mean difference between MRI-PDFF and MRS was −1.5%. In another study by Idilman et al. (15), MRI-PDFF and MRS had an excellent correlation for hepatic fat quantification (r=0.986), and the mean difference between MRI-PDFF and MRS was −2.4%. A study by Kang et al. (26) also demonstrated that MRI-PDFF and MRS had an excellent correlation (r=0.961). In the study, the difference between MRS and MRI-PDFF values tended to increase as the average of the two values increased. In contrast, in our study, the difference did not change significantly as the average of the two values increased. In the previous study, although multi-echo (six echo) mDixon technique was used as in our study, a different MRI device from a difference manufacturer (Ingenia; Philips Healthcare, Best, The Netherlands) was used, and various liver diseases other than NAFLD were also included.
MRS is considered as the most accurate modality for a noninvasive hepatic fat quantification (10,11). However, it has several disadvantages in clinical application, including its relatively high cost, requirement of skilled expertise for data collection and analysis, and small sampling volume (27). MRI-PDFF has the advantages of covering the entire volume and being available on any MRI devices (28). As mentioned earlier, MRI-PDFF demonstrated promising results for the accurate quantification of hepatic steatosis, so it is expected to be widely used for hepatic fat detection and monitoring.
In this study, the FFs measured by two different histopathologic methods (AI and pathologist) showed a very strong correlation (r=0.872). In previous studies (18-20), the FF calculated as the area of fat using semiautomatic segmentation and the FF calculated as the percentage of hepatocytes containing fat had a good correlation. In a study by Noworolski et al. (18), the FF determined as a percentage of tissue area [using a high magnification (×200 and ×400)] was lower than the FF determined as a percentage of hepatocytes containing fat [using a standard magnification (×40 and ×100)], and roughly linearly correlated. In a previous study by d'Assignies et al. (19), the FF measured using semiautomatic fat vacuole segmentation and the FF measured by pathologist’s visual assessment had a good correlation (r=0.760). A study by Kukuk et al. (20) also reported an excellent correlation between the FF determined by semiautomatic quantification and the FF determined as the percentage of hepatocytes including fat determined by visual assessment (r=0.929).
Liver biopsy is the reference standard for the diagnosis and staging of NAFLD, and NASH-CRN has been widely used for staging its severity (5,29). However, it has shown poor inter- and intra-observer variability in evaluating the histologic components of NASH (29,30). Therefore, various efforts have been made to measure hepatic fat on histopatholgic slides more accurately (18-20). In the studies mentioned above, a semiautomatic method was used by pathologists to exclude artifacts (e.g., blood vessels) from magnified or digitalized histopathologic slides. With the recent advances in AI, several studies quantified fat using a deep learning algorithm (6-8). In this study, entire histopathologic slides of liver biopsy specimens were scanned as digitalized images; then, the area of fat in the five representative images was automatically measured using the deep learning algorithm. The FFs measured by MRS and MRI-PFF demonstrated a strong correlation with the FF measured by pathologists as well as the FF automatically measured using this deep learning algorithm.
Although, in general, there may be intra- and inter-observer variability in histological grading of hepatic steatosis, the correlation between FFAI and FFpathologist in our study was better than expected. The FFAI and FFpathologist showed a very strong correlation, which is thought to be in due to AI and pathologist evaluating and measuring FFs using the same liver biopsy sample. For the same reason, there was a very strong correlation between FFMRS and MRI-PDFF, as they were measured at the same location as possible. However, both FFMRS and MRI-PDFF demonstrated only a strong correlation with the two different histopathologic methods. This result may be due to the possibility of the biopsy site on US and the location of voxel/ROI on MRS/MRI-PDFF not matching perfectly, despite efforts to measure the FFs at the same location as possible.
This study has several limitations. First, since this was a retrospective study, liver biopsy and MRI were not always performed on the same day. However, patients who had liver biopsy and MRI with an interval of more than 30 days were excluded from this study, and the average interval of 21 days was acceptable. Second, a relatively small number of patients were included, and there were no subjects with grade 0 steatosis. Since this was a retrospective study that included patients with NAFLD who underwent biopsy for NASH evaluation, it is impossible and unethical to perform liver biopsy in healthy people with grade 0 steatosis. Third, the MRS and MRI-PDFF measurements were performed only on one machine in a tertiary hospital. Because different machines from different manufacturers may show different spectra, a larger-scale prospective study that includes several different machines is needed. Fourth, 18-gauge needle may be inadequate compared to 16-gauge needle. A previous study demonstrated that larger gauge needles improved adequacy rate with longer, intact liver biopsy specimens and increased number of portal tracts (31). However, all biopsy specimens in our study were more than 2 cm in length and included more than 11 portal tracts, so they were all adequate for histopathologic diagnosis. Finally, since MRS has an intrinsic problem that FF cannot be measured in the entire liver, to evaluate the correlation between MRI-PDFF and MRS, FF was measured by placing the circular ROI at the same location as MRS in MRI-PDFF.
Conclusions
The spectrum of FF was in the order of FFAI, MRI-PDFF, FFMRS, and FFpathologist. MRS and MRI-PDFF demonstrated a strong correlation with each other and with the two different histopathologic methods. Although it is difficult to measure the amount of real hepatic fat, the results demonstrated that MRI-PDFF and MRS can be used as an alternative noninvasive reference standard in NAFLD patients. However, these preliminary results should be interpreted with caution until they are validated in further studies. Since MRS and MRI-PDFF showed a difference of approximately 2% on average, it is recommended to use one consistent method as a reference standard for treatment monitoring.
Acknowledgments
The authors would like to thank Soon-Young Hwang, an experienced medical statistician at our institution who kindly provided statistical advice for this manuscript. The authors also would like to thank Jong Man Kim who contributed to methodology of the manuscript using a deep learning method.
Funding: None.
Footnote
Reporting Checklist: The authors have completed the STARD reporting checklist. Available at https://qims.amegroups.com/article/view/10.21037/qims-22-393/rc
Conflicts of Interest: All authors have completed the ICMJE uniform disclosure form (available at https://qims.amegroups.com/article/view/10.21037/qims-22-393/coif). CHL serves as an unpaid editorial board member of Quantitative Imaging in Medicine and Surgery. The other authors have no conflicts of interest to declare.
Ethical Statement: The authors are accountable for all aspects of the work in ensuring that questions related to the accuracy or integrity of any part of the work are appropriately investigated and resolved. The study was conducted in accordance with the Declaration of Helsinki (as revised in 2013). This study was reviewed and approved by the institutional review board (IRB) of the Korea University Guro Hospital (Approval No. KUGH16184) and individual consent for this retrospective analysis was waived.
Open Access Statement: This is an Open Access article distributed in accordance with the Creative Commons Attribution-NonCommercial-NoDerivs 4.0 International License (CC BY-NC-ND 4.0), which permits the non-commercial replication and distribution of the article with the strict proviso that no changes or edits are made and the original work is properly cited (including links to both the formal publication through the relevant DOI and the license). See: https://creativecommons.org/licenses/by-nc-nd/4.0/.
References
- Younossi ZM, Koenig AB, Abdelatif D, Fazel Y, Henry L, Wymer M. Global epidemiology of nonalcoholic fatty liver disease-Meta-analytic assessment of prevalence, incidence, and outcomes. Hepatology 2016;64:73-84. [Crossref] [PubMed]
- Chalasani N, Younossi Z, Lavine JE, Charlton M, Cusi K, Rinella M, Harrison SA, Brunt EM, Sanyal AJ. The diagnosis and management of nonalcoholic fatty liver disease: Practice guidance from the American Association for the Study of Liver Diseases. Hepatology 2018;67:328-57. [Crossref] [PubMed]
- Takahashi Y, Fukusato T. Histopathology of nonalcoholic fatty liver disease/nonalcoholic steatohepatitis. World J Gastroenterol 2014;20:15539-48. [Crossref] [PubMed]
- Abd El-Kader SM, El-Den Ashmawy EM. Non-alcoholic fatty liver disease: The diagnosis and management. World J Hepatol 2015;7:846-58. [Crossref] [PubMed]
- Kleiner DE, Brunt EM, Van Natta M, Behling C, Contos MJ, Cummings OW, Ferrell LD, Liu YC, Torbenson MS, Unalp-Arida A, Yeh M, McCullough AJ, Sanyal AJNonalcoholic Steatohepatitis Clinical Research Network. Design and validation of a histological scoring system for nonalcoholic fatty liver disease. Hepatology 2005;41:1313-21. [Crossref] [PubMed]
- Forlano R, Mullish BH, Giannakeas N, Maurice JB, Angkathunyakul N, Lloyd J, Tzallas AT, Tsipouras M, Yee M, Thursz MR, Goldin RD, Manousou P. High-Throughput, Machine Learning-Based Quantification of Steatosis, Inflammation, Ballooning, and Fibrosis in Biopsies From Patients With Nonalcoholic Fatty Liver Disease. Clin Gastroenterol Hepatol 2020;18:2081-2090.e9. [Crossref] [PubMed]
- Ramot Y, Zandani G, Madar Z, Deshmukh S, Nyska A. Utilization of a Deep Learning Algorithm for Microscope-Based Fatty Vacuole Quantification in a Fatty Liver Model in Mice. Toxicol Pathol 2020;48:702-7. [Crossref] [PubMed]
- Heinemann F, Birk G, Stierstorfer B. Deep learning enables pathologist-like scoring of NASH models. Sci Rep 2019;9:18454. [Crossref] [PubMed]
- Lv S, Jiang S, Liu S, Dong Q, Xin Y, Xuan S. Noninvasive Quantitative Detection Methods of Liver Fat Content in Nonalcoholic Fatty Liver Disease. J Clin Transl Hepatol 2018;6:217-21. [Crossref] [PubMed]
- Szczepaniak LS, Nurenberg P, Leonard D, Browning JD, Reingold JS, Grundy S, Hobbs HH, Dobbins RL. Magnetic resonance spectroscopy to measure hepatic triglyceride content: prevalence of hepatic steatosis in the general population. Am J Physiol Endocrinol Metab 2005;288:E462-8. [Crossref] [PubMed]
- Schwenzer NF, Springer F, Schraml C, Stefan N, Machann J, Schick F. Non-invasive assessment and quantification of liver steatosis by ultrasound, computed tomography and magnetic resonance. J Hepatol 2009;51:433-45. [Crossref] [PubMed]
- Zhang QH, Zhao Y, Tian SF, Xie LH, Chen LH, Chen AL, Wang N, Song QW, Zhang HN, Xie LZ, Shen ZW, Liu AL. Hepatic fat quantification of magnetic resonance imaging whole-liver segmentation for assessing the severity of nonalcoholic fatty liver disease: comparison with a region of interest sampling method. Quant Imaging Med Surg 2021;11:2933-42. [Crossref] [PubMed]
- Yokoo T, Shiehmorteza M, Hamilton G, Wolfson T, Schroeder ME, Middleton MS, Bydder M, Gamst AC, Kono Y, Kuo A, Patton HM, Horgan S, Lavine JE, Schwimmer JB, Sirlin CB. Estimation of hepatic proton-density fat fraction by using MR imaging at 3.0 T. Radiology 2011;258:749-59. [Crossref] [PubMed]
- Permutt Z, Le TA, Peterson MR, Seki E, Brenner DA, Sirlin C, Loomba R. Correlation between liver histology and novel magnetic resonance imaging in adult patients with non-alcoholic fatty liver disease - MRI accurately quantifies hepatic steatosis in NAFLD. Aliment Pharmacol Ther 2012;36:22-9. [Crossref] [PubMed]
- Idilman IS, Keskin O, Celik A, Savas B, Elhan AH, Idilman R, Karcaaltincaba M. A comparison of liver fat content as determined by magnetic resonance imaging-proton density fat fraction and MRS versus liver histology in non-alcoholic fatty liver disease. Acta Radiol 2016;57:271-8. [Crossref] [PubMed]
- Vu KN, Gilbert G, Chalut M, Chagnon M, Chartrand G, Tang A. MRI-determined liver proton density fat fraction, with MRS validation: Comparison of regions of interest sampling methods in patients with type 2 diabetes. J Magn Reson Imaging 2016;43:1090-9. [Crossref] [PubMed]
- Syväri J, Junker D, Patzelt L, Kappo K, Al Sadat L, Erfanian S, Makowski MR, Hauner H, Karampinos DC. Longitudinal changes on liver proton density fat fraction differ between liver segments. Quant Imaging Med Surg 2021;11:1701-9. [Crossref] [PubMed]
- Noworolski SM, Lam MM, Merriman RB, Ferrell L, Qayyum A. Liver steatosis: concordance of MR imaging and MR spectroscopic data with histologic grade. Radiology 2012;264:88-96. [Crossref] [PubMed]
- d'Assignies G, Ruel M, Khiat A, Lepanto L, Chagnon M, Kauffmann C, Tang A, Gaboury L, Boulanger Y. Noninvasive quantitation of human liver steatosis using magnetic resonance and bioassay methods. Eur Radiol 2009;19:2033-40. [Crossref] [PubMed]
- Kukuk GM, Hittatiya K, Sprinkart AM, Eggers H, Gieseke J, Block W, Moeller P, Willinek WA, Spengler U, Trebicka J, Fischer HP, Schild HH, Träber F. Comparison between modified Dixon MRI techniques, MR spectroscopic relaxometry, and different histologic quantification methods in the assessment of hepatic steatosis. Eur Radiol 2015;25:2869-79. [Crossref] [PubMed]
- Kim JW, Lee YS, Park YS, Kim BH, Lee SY, Yeon JE, Lee CH, Multiparametric MR. Index for the Diagnosis of Non-Alcoholic Steatohepatitis in Patients with Non-Alcoholic Fatty Liver Disease. Sci Rep 2020;10:2671. [Crossref] [PubMed]
- Park YS, Lee CH, Kim JH, Kim BH, Kim JH, Kim KA, Park CM. Effect of Gd-EOB-DTPA on hepatic fat quantification using high-speed T2-corrected multi-echo acquisition in (1)H MR spectroscopy. Magn Reson Imaging 2014;32:886-90. [Crossref] [PubMed]
- Pineda N, Sharma P, Xu Q, Hu X, Vos M, Martin DR. Measurement of hepatic lipid: high-speed T2-corrected multiecho acquisition at 1H MR spectroscopy--a rapid and accurate technique. Radiology 2009;252:568-76. [Crossref] [PubMed]
- Reeder SB, Cruite I, Hamilton G, Sirlin CB. Quantitative Assessment of Liver Fat with Magnetic Resonance Imaging and Spectroscopy. J Magn Reson Imaging 2011;34:729-49. [Crossref] [PubMed]
- Kramer H, Pickhardt PJ, Kliewer MA, Hernando D, Chen GH, Zagzebski JA, Reeder SB. Accuracy of Liver Fat Quantification With Advanced CT, MRI, and Ultrasound Techniques: Prospective Comparison With MR Spectroscopy. AJR Am J Roentgenol 2017;208:92-100. [Crossref] [PubMed]
- Kang BK, Kim M, Song SY, Jun DW, Jang K. Feasibility of modified Dixon MRI techniques for hepatic fat quantification in hepatic disorders: validation with MRS and histology. Br J Radiol 2018;91:20170378. [PubMed]
- Pasanta D, Htun KT, Pan J, Tungjai M, Kaewjaeng S, Kim H, Kaewkhao J, Kothan S. Magnetic Resonance Spectroscopy of Hepatic Fat from Fundamental to Clinical Applications. Diagnostics (Basel) 2021;11:842. [Crossref] [PubMed]
- Caussy C, Reeder SB, Sirlin CB, Loomba R. Noninvasive, Quantitative Assessment of Liver Fat by MRI-PDFF as an Endpoint in NASH Trials. Hepatology 2018;68:763-72. [Crossref] [PubMed]
- Sumida Y, Nakajima A, Itoh Y. Limitations of liver biopsy and non-invasive diagnostic tests for the diagnosis of nonalcoholic fatty liver disease/nonalcoholic steatohepatitis. World J Gastroenterol 2014;20:475-85. [Crossref] [PubMed]
- Ratziu V, Charlotte F, Heurtier A, Gombert S, Giral P, Bruckert E, Grimaldi A, Capron F, Poynard TLIDO Study Group. Sampling variability of liver biopsy in nonalcoholic fatty liver disease. Gastroenterology 2005;128:1898-906. [Crossref] [PubMed]
- Neuberger J, Patel J, Caldwell H, Davies S, Hebditch V, Hollywood C, Hubscher S, Karkhanis S, Lester W, Roslund N, West R, Wyatt JI, Heydtmann M. Guidelines on the use of liver biopsy in clinical practice from the British Society of Gastroenterology, the Royal College of Radiologists and the Royal College of Pathology. Gut 2020;69:1382-403. [Crossref] [PubMed]