Differentiation of predominantly osteolytic from osteoblastic spinal metastases based on standard magnetic resonance imaging sequences: a comparison of radiomics model versus semantic features logistic regression model findings
Introduction
The spine is the third most common site for distant metastases of malignant tumors, second only to the lungs and liver. A considerable percentage of patients with malignant tumors (40–70%) develop spinal metastases (1,2). Spinal metastases often cause pain, pathological fractures, hypercalcemia, nerve root compression, and spinal cord compression symptoms, thereby severely reducing patients’ quality of life (3). When spinal metastases occur, the dynamic balance between bone formation and bone destruction is destroyed through an interaction between tumor cells and the bone microenvironment (4). Spinal metastases usually lead to the development of 2 types of lesions: osteolytic and osteoblastic. Osteolytic bone metastases are usually noted in lung, kidney, thyroid, and breast cancer (5-8), while osteoblastic bone metastases are commonly seen in prostate and breast cancer (9). The differentiation between osteolytic and osteoblastic metastases is clinically significant for the diagnosis, efficacy evaluation, and prognosis prediction of spinal metastases. The clinical significance includes the following: (I) narrowing the search for primary tumor sites in patients with spinal metastases with unknown primary tumors (10); (II) planning the treatment, including surgery and molecular targeted drugs (9,11); (III) assessing the therapeutic response, as sclerotic transformation in formerly osteolytic lesions generally suggests a response to treatment (12); and (IV) factoring in the higher incidence of vertebral body pathologic fractures with osteolytic metastases compared to osteoblastic metastases, as osteolytic lesions have the highest impact on bone strength (13).
The most frequent modalities to visualize and characterize spinal metastases are computed tomography (CT), magnetic resonance imaging (MRI), and nuclear examination. The current gold standard for differentiating osteolytic from osteoblastic lesions is CT-based changes in Hounsfield units (HUs). However, MRI provides detailed information on the bone marrow and offers excellent soft-tissue contrast. Therefore, it is one of the modalities of choice in the detection, diagnosis, and assessment of treatment response of spinal metastases. Moreover, standard pulse sequences, including T1-weighted (T1W), T2-weighted (T2W), and fat-suppressed T2-weighted (FS-T2W) sequences can achieve high detection and diagnostic accuracy (12,14,15). The signal intensity of spinal metastases is almost invariably of iso low signal intensity on T1W MRIs, but its variability on T2W MRIs is related to osteoblastic or osteolytic characteristics. Osteolytic lesions are more likely to be of high signal intensity, while osteoblastic lesions are more likely to be of iso low signal intensity on T2W MRIs (16,17). In addition, compared with osteoblastic lesions, osteolytic lesions are often accompanied by soft masses and pathological fractures (18,19). These qualitative semantic imaging features are often used to differentiate between predominantly osteolytic and osteoblastic spinal metastases.
However, due to the heterogeneity of osteolytic and osteoblastic lesions on T2W MRIs, there are limitations to standard MRI for differentiating between osteolytic and osteoblastic spinal metastases (10). As a new medical image analysis method, radiomics enables the extraction and selection of a large number of more sophisticated quantitative imaging features from medical images with high throughput, based on which descriptive and predictive models can be constructed (20). Some studies have shown that radiomics has potential for application in differentiation, diagnosis, genetic analysis, treatment response assessment, and prognosis in cases of spinal metastases (21-25). However, few previous studies have compared the performance of the semantic features and radiomics models in differentiating between osteolytic and osteoblastic spinal metastases based on standard MRI to evaluate the potential value of radiomics features.
Therefore, in this study, we developed a radiomics model and a semantic features logistic regression model for differentiation of osteolytic and osteoblastic spinal metastases and comparatively assessed the performance of these 2 models. We present the following article in accordance with the STARD (Standards for Reporting Diagnostic accuracy studies) reporting checklist (available at https://qims.amegroups.com/article/view/10.21037/qims-22-267/rc).
Methods
Study population
The study was conducted in accordance with the Declaration of Helsinki (as revised in 2013). This study was approved by and registered with the Peking University Third Hospital Medical Science Research Ethics Committee, and individual consent for this retrospective analysis was waived. CT images and MRIs of patients with suspected spinal metastases after magnetic resonance (MR) examinations at Peking University Third Hospital between August 2006 and August 2019 (Figure 1) were retrospectively analyzed. The inclusion criteria were as follows: (I) patients with pathologically confirmed spinal metastases and (II) patients with CT images of the same site as the MRIs of the spine and without any therapy administered between the CT and MR scans. The exclusion criteria were as follows: (I) surgery performed for the lesion of the spinal metastases before MR and CT examinations; (II) lesions on CT not being the manifestation of predominantly osteolytic or osteoblastic spinal metastases; (III) and missing sagittal T1W, T2W, or FS-T2W sequences of the spine.
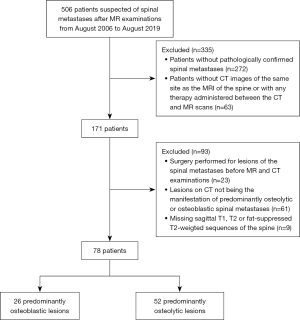
Imaging acquisition
Sagittal T1W, T2W, and FS-T2W sequences of all patients were acquired with a 1.5-T or 3.0-T MR scanner. Table S1 shows the model information of the different MR scanners. Scanning parameters of the cervical, thoracic, lumbar, and sacral vertebrae are shown in Table S2. CT images of the same sites as those examined by MRI were acquired using different scanners with a tube voltage of 120 kV and a tube current of between 137 and 543 mAs. The collimator width was 0.625 or 0.60 mm; the pitch was 1.0; and the slice thickness of reconstruction was 3 mm. For further observation, the acquired images were reformatted to a sagittal view (3-mm thickness). Table S3 shows the model information of the different CT scanners.
For all identified patients, we exported MR and CT scans in Digital Imaging and Communications in Medicine (DICOM) format from the picture archiving and communication system (PACS). If multiple CT or MR scans meeting the inclusion and exclusion criteria were performed at the site of the patient’s lesion, we selected the MRIs and CT images with the shortest interval.
Assessment of CT imaging
With CT as the gold standard, lesions with a higher mean CT HU than those of the adjacent normal vertebrae were considered predominantly osteoblastic, while lesions with a lower mean CT HU were considered predominantly osteolytic. The biggest lesion in the field of view (FOV) was then selected. A radiologist with 4 years (Q.W.) of experience in the diagnosis of musculoskeletal diseases determined whether each lesion was predominantly osteoblastic or osteolytic. The radiologist was blinded to histopathological diagnosis, MRIs, and radiological reports of the MR examinations.
Assessment of semantic features
A radiologist with 5 years (Y.C.) of experience evaluated 5 semantic features (Table 1) of each lesion on sagittal T1W, T2W, and FS-T2W sequences of the spine. For lesions where Y.C. was not confident in his evaluation, a senior musculoskeletal radiologist with 18 years (N.L.) of experience reviewed the MRI scans together with Y.C. to arrive at a mutual consensus. Both radiologists were blinded to histopathological diagnosis, CT images, and radiological reports of the MR examinations.
Table 1
Semantic features | Descriptions |
---|---|
SI on T1WS | Described as low, iso-, or high signal intensity |
SI on T2WS | Described as low, iso-, or high signal intensity |
SI on FS-T2WS | Described as low, iso-, or high signal intensity |
Soft tissue masses | Including intraspinal soft tissue masses and paravertebral soft tissue masses |
Severe compression fractures | Compression height exceeds 50% of the vertebral body |
Low signal intensity, the signal intensity of the lesion is lower than that of the spinal cord at the same slice; high signal intensity, the signal intensity of the lesion is higher than that of the spinal cord at the same slice; iso-signal intensity, the signal intensity of the lesion is approximately equal to that of the spinal cord at the same slice. SI, signal intensity; T1WS, T1-weighted sequence; T2WS, T2-weighted sequence; FS-T2WS, fat-suppressed T2-weighted sequence.
Radiomic analysis
Using ImageJ software (version 1.53a, https://imagej.nih.gov; National Institutes of Health, Bethesda, MD, USA), a radiologist with 3 years (S.Q.) of experience delineated the edge of the region of interest (ROI) of the lesion layer by layer on the spine sagittal T1W, T2W, and FS-T2W sequences. Another radiologist with 18 years (N.L.) of experience in diagnosing musculoskeletal diseases checked each outline. All radiologists were blinded to histopathological diagnosis, CT images, and radiological reports of the MR examinations.
A total of 107 features were extracted from the ROI on each modality. In this study, 4 textual parametric matrices were applied for feature extraction. In particular, 24 features were extracted with a gray-level co-occurrence matrix (GLCM), 16 features with a gray-level size zone matrix (GLSZM), 16 features with a gray-level run length matrix (GLRLM), 14 features with a gray-level dependence matrix (GLDM), and 5 features with a neighboring gray-tone difference matrix (NGTDM). Besides texture features, we also used 18 first-order features and 14 shape features. All features are summarized in Table S4. Tumor segmentation was performed on each 2-dimensional slice, and 2-dimensional slices were rendered into a 3-dimensional space with isotropic voxel resolution for extracting the 3-dimensional texture features.
After the features for all cases were extracted, they were properly normalized to a mean of 0 and a standard deviation of 1. The feature selection was a sequential feature selection process, in which multiple support vector machine (SVM) classifiers were trained and validated. SVM is a supervised machine learning algorithm that occupies different kernel functions, which can map the data to kernel space to optimize the classification performance. During the feature selection process, SVM with a Gaussian kernel served as the cost function to evaluate the performance of models, which were trained from a subset of extracted features. At the start of the selection process, we used an empty candidate feature set. The selected features were then sequentially added to this feature set. To evaluate the model performance, we used 10-fold cross-validation to continuously evaluate the importance of the selected features. To test the robustness of the features, training processes were repeated 1,000 times, and then the feature that provided the best performance was added to the candidate set. When the additional feature could no longer improve the model performance, the feature selection was terminated. In this study, 10−6 worked as the termination tolerance for the cost function value.
The analysis was performed using programs written in MATLAB 2019b (MathWorks, Natick, MA, USA) and Python 3.7 (Python Software Foundation, Wilmington, DE, USA). The radiomics analysis procedures are illustrated in Figure 2.
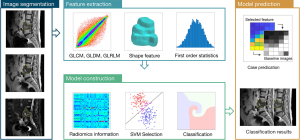
Correlation analysis of radiomics feature values and metastases-to-normal vertebral body CT HU ratio
CT HU units can effectively differentiate predominantly osteolytic and osteoblastic metastases. We used metastases-to-normal vertebral body CT HU ratio (MVR) to describe the extent of bone formation or destruction of the lesions. This was performed to correct the differences in the degree of vertebral bone mineralization between the different patients due to nonmetastatic factors such as osteoporosis. Our aim was to demonstrate that these radiomics features performed as well as CT HUs in differentiating between osteolytic and osteoblastic spinal metastases. Therefore, we further analyzed the correlation between MVRs and the values of radiomics features, which were selected to construct the radiomics model. CT HUs were measured using a built-in calculation tool in the hospital’s PACS (Centricity RIS CE v. 3.0; Chicago, IL, USA). The oval ROI of metastases and normal vertebral bodies were placed at the largest area of the lesion and adjacent normal vertebral bodies on the most representative sagittal slice to measure the CT HUs. The following MVR was calculated:
Pearson correlation coefficients were calculated between MVR and the value of each radiomics feature that was used to construct the radiomics model.
Model development and validation
The radiomics features and semantic features selected by the SVM were evaluated for their ability to predict predominantly osteolytic and osteoblastic lesions using both an SVM model and a logistic regression mode. A detailed description of SVM and logistic regression is included in the Supplementary Materials (Appendix 1). The performance of the 2 models was assessed using 10-fold cross-validation. While 90% of the cases were used for training the model, the performance was evaluated using the remaining 10% cases.
Statistical analysis
Statistical analyses were performed using SPSS 26.0 (IBM Corp., Armonk, NY, USA). Chi-square tests were used for categorical and ranked data to evaluate semantic features. A receiver operating characteristic (ROC) curve was generated to show the predictive performance. Sensitivity, specificity, accuracy, positive predictive value (PPV), negative predictive value (NPV), and area under the ROC curve (AUC) were calculated. P values <0.05 were considered statistically significant. The difference in the AUC between the radiomics model and the semantic model was analyzed using the DeLong test.
Results
Demographics and clinical characteristics
Among 78 lesions of spinal metastases, 26 lesions were predominantly osteoblastic, while 52 lesions were predominantly osteolytic. The mean patient age (standard deviation) was 59.2±10.6 years (range, 39–84 years). The study population included 51 men with a mean age of 60.3±11.2 years (range, 39–84 years) and 27 women with a mean age of 57.0±9.0 years (range, 44–70 years). The average scan interval between MRIs and CT images was 6 days. The primary tumors and anatomic locations of all lesions are shown in Table 2.
Table 2
Characteristics | All lesions (n=78) | Osteoblastic lesions (n=26) | Osteolytic lesions (n=52) |
---|---|---|---|
Primary tumors | |||
Lung cancer | 24 | 8 | 16 |
Renal carcinoma | 11 | 0 | 11 |
Breast carcinoma | 8 | 5 | 3 |
Prostate carcinoma | 7 | 5 | 2 |
Cancer of unknown primary | 7 | 5 | 2 |
Thyroid carcinoma | 7 | 1 | 6 |
Colorectal carcinoma | 5 | 2 | 3 |
Hepatocellular carcinoma | 5 | 0 | 5 |
Cholangiocellular carcinoma | 2 | 0 | 2 |
Rhabdomyosarcoma | 1 | 0 | 1 |
Leiomyosarcoma of uterus | 1 | 0 | 1 |
Locations | |||
Cervical spine | 25 | 3 | 22 |
Thoracic spine | 22 | 9 | 13 |
Lumbar spine | 28 | 14 | 14 |
Sacral spine | 3 | 0 | 3 |
Evaluation results of semantic features
The evaluation results of the 5 semantic features by a radiology resident with 5 years (Y.C.) of experience are shown in Table 3. Except for severe compression fractures, the signal intensities of T1W, T2W, and FS-T2W sequences and the existence of soft tissue masses were significantly different between the predominantly osteolytic and osteoblastic groups (P≤0.001).
Table 3
Semantic features | Osteoblastic (n=26), n (%) | Osteolytic (n=52), n (%) | χ2 value | P value |
---|---|---|---|---|
SI on T1WS | 15.169 | 0.001 | ||
Low | 23 (88.5) | 22 (42.3)a | ||
Iso- | 2 (7.7) | 17 (32.7)a | ||
High | 1 (3.8) | 13 (25)a | ||
SI on T2WS | 17.016 | <0.001 | ||
Low | 19 (73.1) | 13 (25)a | ||
Iso- | 0 (0) | 4 (7.7) | ||
High | 7 (16.7) | 35 (67.3)a | ||
SI on FS-T2WS | 11.446 | 0.001 | ||
Low | 8 (30.8) | 1 (1.9)a | ||
High | 18 (69.2) | 51 (98.1)a | ||
Soft tissue mass | 12 (46.2) | 47 (90.4)a | 18.404 | <0.001 |
Severe compression fracture | 9 (34.6) | 22 (42.3) | 0.428 | 0.513 |
a, comparison with osteoblastic lesions, P<0.05. SI, signal intensity; T2WS, T2-weighted sequence; T1WS, T1-weighted sequence; FS-T2WS, fat-suppressed T2-weighted sequence.
Features to construct the radiomics model
For radiomics analysis, 6 features were considered to show the high importance in the feature selection process, including 2 features from T1W sequences and 4 features from T2W sequences. T1W features included skewness and GLCM max probability. T2W features included GLCM difference entropy, NGTDM busyness, surface area/volume ratio, and GLRLM long run low gray-level emphasis (LRLGLE). The final classification results were generated using the selected features using SVM.
The Pearson correlation between MVR and T1W GLCM maximum probability was 0.88 (Figure 3). For other features, the Pearson correlation values based on MVR were lower than 0.74 (T1W skewness, 0.62; T2W GLCM difference entropy, 0.74; T2W NGTDM busyness, 0.41; T2W GLRLM LRLGLE, 0.41; and T2W surface area/volume ratio, 0.23; Figure 3).
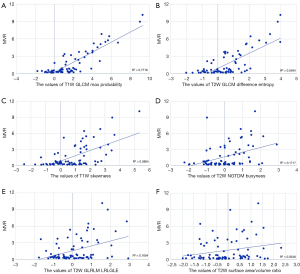
Comparison of semantic features model and radiomics model
For the semantic features logistic regression model and radiomics model, after 10-fold cross-validation, the overall prediction accuracy was 75.6% and 78.2%, respectively. Based on the ROC curve, the corresponding AUCs obtained for the prediction of osteolytic and osteoblastic metastases were 0.79 (95% CI: 0.68–0.91) and 0.82 (95% CI: 0.71–0.93). Based on the DeLong test, the P value was 0.15, and the z value was 0.77. Other diagnostic results are listed in Table 4, and the generated ROC curves are shown in Figure 4. Figure 5 shows 2 examples in which osteolytic and osteoblastic spinal metastases were correctly predicted by the radiomics model and semantic features logistic model. Figure 6 shows 2 examples in which osteolytic and osteoblastic spinal metastases were correctly predicted by the radiomics model only.
Table 4
Models | Sensitivity | Specificity | PPV | NPV | Accuracy | AUC (95% CI) |
---|---|---|---|---|---|---|
Radiomics | 73.1% (19/26) | 80.8% (42/52) | 65.5% (19/29) | 85.7% (42/49) | 78.2% | 0.82 (0.71–0.93) |
Logistic regression with semantic features | 80.8% (21/26) | 73.1% (38/52) | 60.0% (21/35) | 88.4% (38/43) | 75.6% | 0.79 (0.68–0.91) |
PPV, positive predictive value; NPV, negative predictive value; AUC, area under the receiver operating characteristic curve; CI, confidence interval.
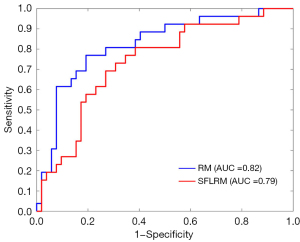
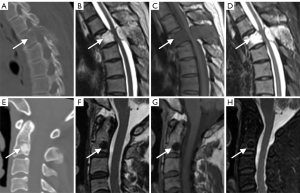
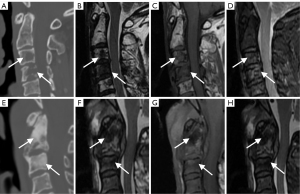
Discussion
Standard MRI has exceptionally high accuracy in the detection of spinal metastases. However, due to the heterogeneity of osteolytic and osteoblastic lesions of T2W MRIs, there are limitations to the application of standard MRI for differentiating between osteolytic and osteoblastic spinal metastases. Emerging radiomics technology can further mine MRI data. This study proved that some semantic features of standard MRI (the signal intensities of T1W, T2W, FS-T2W sequences, and the presence of soft-tissue masses) were significantly different between osteolytic and osteoblastic spinal metastases (P<0.001). CT was used as the reference standard. Constructed using standard MRI semantic features, the logistic regression model used for differentiating osteolytic spinal metastases from osteoblastic metastases had an accuracy of 75.6% and an AUC of 0.79. The accuracy of the radiomics model based on standard MRI was 78.2%, and the AUC was 0.82. T1W GLCM max probability, which was used to construct the radiomics model, was strongly correlated with MVR measurements obtained with CT (r=0.88). Overall, the standard MRI-based radiomics model outperformed the semantic features logistic regression model in differentiating between predominantly osteolytic and osteoblastic spinal metastases.
MRI, CT, whole-body bone scanning (BS), positron emission tomography (PET), and single-photon emission computed tomography (SPECT) are commonly used in the diagnosis of spinal metastases. One meta-analysis suggests that MRI is the best modality and better than other techniques for diagnosing spinal metastases on both a per-patient and a per-lesion basis (26). However, additional CT examinations with extra costs and associated ionizing radiation may be required because osteolytic and osteoblastic metastases cannot always be reliably differentiated with standard MRI sequences. Radiomics is a quantitative approach to medical imaging whose purpose is to enhance the existing data available to clinicians by advanced and sometimes nonintuitive mathematical analysis (27). According to the AUC, the performance of the radiomics model was better than that of the semantic model based on standard MRI; however, as the P value was higher than 0.05, this difference was not significant. However, considering the larger number of osteolytic cases, the radiomics model had a lower number of false positives and improved the specificity. Therefore, the radiomics model can be more robust and generalized when validating other data sets. In addition, some studies have shown that software can automatically identify, segment, and extract radiomics features of tumors (28-30). However, advances in data-sharing technology will help collect data on large cohorts of patients and, thus, improve the performance of radiomics (31). We believe that radiomics can assist with generating more valuable patient information in a single MR exam, thus minimizing the number of required exams and costs in the future.
Böker et al. (10) showed that susceptibility-weighted MR has high sensitivity and specificity for osteolytic and osteoblastic spinal metastases. However, susceptibility is dependent on the magnetic field strength and echo time; thus, the results from this study cannot be directly extrapolated to MR scans, which have magnetic field strengths other than 1.5-T or different echo times. Moreover, MRI scan times are prolonged with an increase in the scan sequence, and patients with spinal metastases often cannot tolerate extended examinations. Therefore, susceptibility-weighted MRI has certain limitations. Nonetheless, adjusting the sequence for individual scanners is not difficult. Multitask radiomics training was recently used in medical imaging for several tasks and modalities (32-34). Therefore, radiomics has a broader range of potential applications than does susceptibility-weighted MR.
Linear regression and logistic regression are widely used due to their simplicity (35). With very few inputs, a relatively general model can be established. SVM is the most popular classification algorithm and typically exhibits the highest performance ranks for many classification problems in radiomics studies, given its advantages of regularization and convex optimization (36-39). Therefore, we selected the above 2 methods to construct models according to the characteristics of semantic features and radiomics features. In our study, the classification performance of the semantic features logistic regression models was lower than that of the radiomics model. One possible explanation was that the texture features (GLCM, GLRLM, NGTDM) to construct the radiomics model contained more information about the distribution of voxel intensities and the intervoxel relationships of lesions compared with MRI signal intensity (40).
The high-dimensionality features used to construct a radiomics model are abstract and difficult to interpret. This reduces the generalizability of the model and also leads to lower trust and acceptance of the model by end users (41). Therefore, we analyzed the correlation between the values of the features used to construct the radiomics model and the MVR. The strong correlation between MVR and T1W GLCM maximum probability (r=0.88) and its relatively strong correlation with T2W GLCM difference entropy (r=0.74) and T1W skewness (r=0.62) enabled reliable differentiation of predominantly osteolytic and osteoblastic spinal metastases. In addition, a novel MR technique using deep learning for synthetic CT (sCT) transformed T1W radiofrequency spoiled multiple gradient-echo-derived MRI properties of tissues to HUs (42,43). This indicates that T1W MR images can estimate HUs to demonstrate bone anatomy. However, further studies are needed to validate the feasibility of generating sCT images using standard MRI radiomics features and comparing them with previous synthesis methods.
Previous studies have shown that it is difficult to differentiate between osteolytic and osteoblastic metastases using MRI due to the heterogeneity of osteolytic and osteoblastic lesions of T2W MRIs (10,16). We found that among the 3 feature values showing a strong correlation with MVR, 2 were from T1W MRIs, while only 1 was from T2W MRIs. These results are consistent with previous studies. However, in image semantic analysis, the signal intensity on T2W MRIs also showed significant differences between osteolytic and osteoblastic metastases (P<0.001), and 4 of the 6 features selected for constructing the radiomics model were from T2W MRIs. Thus, T2W MRIs also play an essential role in differentiating osteolytic from osteoblastic spinal metastases.
This study had several limitations. First, as a retrospective study, selection bias was inevitable. Second, compared with osteolytic cases, osteoblastic cases are relatively fewer, thus prolonging the time required to collect cases. Third, ROI segmentation was performed manually, hence increasing the possibility of segmentation errors. Image segmentation methods based on deep learning have attracted attention due to their self-learning and generalization capabilities for big data, and these methods have been applied to image segmentation in radiomics (44). Finally, we did not include an independent test data set since this was a preliminary study. When new data sets become available, the model developed in this work can be tested to determine its external validity.
Conclusions
In this study, we compared the ability of a standard MRI-based radiomics model and semantic features logistic regression model in differentiating osteolytic from osteoblastic spinal metastases. The results showed that the performance of the standard MRI-based radiomics model is better than that of the semantic features logistic regression model. Compared with existing methods for differentiating osteolytic from osteoblastic metastases, the performance of the radiomics model may be improved dynamically with the expansion of the training set. In addition, radiomics may prove to have a broader scope of application with single MR exams in future multitask radiomics studies.
Acknowledgments
Funding: This work was supported by the National Natural Science Foundation of China (Nos. 81971578 and 81871326) and the Beijing Municipal Natural Science Foundation (No. Z190020).
Footnote
Reporting Checklist: The authors have completed the STARD reporting checklist. Available at https://qims.amegroups.com/article/view/10.21037/qims-22-267/rc
Conflicts of Interest: All authors have completed the ICMJE uniform disclosure form (available at https://qims.amegroups.com/article/view/10.21037/qims-22-267/coif). The authors have no conflicts of interest to declare.
Ethical Statement: The authors are accountable for all aspects of the work in ensuring that questions related to the accuracy or integrity of any part of the work are appropriately investigated and resolved. The study was conducted in accordance with the Declaration of Helsinki (as revised in 2013). This study was approved by and registered with the Peking University Third Hospital Medical Science Research Ethics Committee, and individual consent for this retrospective analysis was waived.
Open Access Statement: This is an Open Access article distributed in accordance with the Creative Commons Attribution-NonCommercial-NoDerivs 4.0 International License (CC BY-NC-ND 4.0), which permits the non-commercial replication and distribution of the article with the strict proviso that no changes or edits are made and the original work is properly cited (including links to both the formal publication through the relevant DOI and the license). See: https://creativecommons.org/licenses/by-nc-nd/4.0/.
References
- Zhang HR, Qiao RQ, Yang XG, Hu YC. A multicenter, descriptive epidemiologic survey of the clinical features of spinal metastatic disease in China. Neurol Res 2020;42:749-59. [Crossref] [PubMed]
- Salmon JM, Kilpatrick SE. Pathology of skeletal metastases. Orthop Clin North Am 2000;31:537-44. vii-viii. [Crossref] [PubMed]
- Wewel JT, O'Toole JE. Epidemiology of spinal cord and column tumors. Neurooncol Pract 2020;7:i5-9. [Crossref] [PubMed]
- Guise TA, Mohammad KS, Clines G, Stebbins EG, Wong DH, Higgins LS, Vessella R, Corey E, Padalecki S, Suva L, Chirgwin JM. Basic mechanisms responsible for osteolytic and osteoblastic bone metastases. Clin Cancer Res 2006;12:6213s-6s. [Crossref] [PubMed]
- Gong D, Sun Y, Guo C, Sheu TJ, Zhai W, Zheng J, Chang C. Androgen receptor decreases renal cell carcinoma bone metastases via suppressing the osteolytic formation through altering a novel circEXOC7 regulatory axis. Clin Transl Med 2021;11:e353. [Crossref] [PubMed]
- Iñiguez-Ariza NM, Bible KC, Clarke BL. Bone metastases in thyroid cancer. J Bone Oncol 2020;21:100282. [Crossref] [PubMed]
- Kim H, Kim B, Il Kim S, Kim HJ, Ryu BY, Chung J, Lee ZH, Kim HH. S100A4 released from highly bone-metastatic breast cancer cells plays a critical role in osteolysis. Bone Res 2019;7:30. [Crossref] [PubMed]
- Wu S, Pan Y, Mao Y, Chen Y, He Y. Current progress and mechanisms of bone metastasis in lung cancer: a narrative review. Transl Lung Cancer Res 2021;10:439-51. [Crossref] [PubMed]
- Fang J, Xu Q. Differences of osteoblastic bone metastases and osteolytic bone metastases in clinical features and molecular characteristics. Clin Transl Oncol 2015;17:173-9. [Crossref] [PubMed]
- Böker SM, Adams LC, Bender YY, Fahlenkamp UL, Wagner M, Hamm B, Makowski MR. Differentiation of Predominantly Osteoblastic and Osteolytic Spine Metastases by Using Susceptibility-weighted MRI. Radiology 2019;290:146-54. [Crossref] [PubMed]
- Reddington JA, Mendez GA, Ching A, Kubicky CD, Klimo P Jr, Ragel BT. Imaging characteristic analysis of metastatic spine lesions from breast, prostate, lung, and renal cell carcinomas for surgical planning: Osteolytic versus osteoblastic. Surg Neurol Int 2016;7:S361-5. [Crossref] [PubMed]
- Bäuerle T, Semmler W. Imaging response to systemic therapy for bone metastases. Eur Radiol 2009;19:2495-507. [Crossref] [PubMed]
- Benca E, Patsch JM, Mayr W, Pahr DH, Windhager R. The insufficiencies of risk analysis of impending pathological fractures in patients with femoral metastases: A literature review. Bone Rep 2016;5:51-6. [Crossref] [PubMed]
- Hammon M, Dankerl P, Tsymbal A, Wels M, Kelm M, May M, Suehling M, Uder M, Cavallaro A. Automatic detection of lytic and blastic thoracolumbar spine metastases on computed tomography. Eur Radiol 2013;23:1862-70. [Crossref] [PubMed]
- Lange MB, Nielsen ML, Andersen JD, Lilholt HJ, Vyberg M, Petersen LJ. Diagnostic accuracy of imaging methods for the diagnosis of skeletal malignancies: A retrospective analysis against a pathology-proven reference. Eur J Radiol 2016;85:61-7. [Crossref] [PubMed]
- An C, Lee YH, Kim S, Cho HW, Suh JS, Song HT. Characteristic MRI findings of spinal metastases from various primary cancers: retrospective study of pathologically-confirmed cases. J Korean Soc Magn Reson Med 2013;17:8-18. [Crossref]
- Shah LM, Salzman KL. Imaging of spinal metastatic disease. Int J Surg Oncol 2011;2011:769753. [Crossref] [PubMed]
- Chen HY, Ma XM, Bai YR. Radiographic characteristics of bone metastases from hepatocellular carcinoma. Contemp Oncol (Pozn) 2012;16:424-31. [Crossref] [PubMed]
- Rief H, Bischof M, Bruckner T, Welzel T, Askoxylakis V, Rieken S, Lindel K, Combs S, Debus J. The stability of osseous metastases of the spine in lung cancer--a retrospective analysis of 338 cases. Radiat Oncol 2013;8:200. [Crossref] [PubMed]
- Lambin P, Leijenaar RTH, Deist TM, Peerlings J, de Jong EEC, van Timmeren J, Sanduleanu S, Larue RTHM, Even AJG, Jochems A, van Wijk Y, Woodruff H, van Soest J, Lustberg T, Roelofs E, van Elmpt W, Dekker A, Mottaghy FM, Wildberger JE, Walsh S. Radiomics: the bridge between medical imaging and personalized medicine. Nat Rev Clin Oncol 2017;14:749-62. [Crossref] [PubMed]
- Hinzpeter R, Baumann L, Guggenberger R, Huellner M, Alkadhi H, Baessler B. Radiomics for detecting prostate cancer bone metastases invisible in CT: a proof-of-concept study. Eur Radiol 2022;32:1823-32. [Crossref] [PubMed]
- Liu J, Guo W, Zeng P, Geng Y, Liu Y, Ouyang H, Lang N, Yuan H. Vertebral MRI-based radiomics model to differentiate multiple myeloma from metastases: influence of features number on logistic regression model performance. Eur Radiol 2022;32:572-81. [Crossref] [PubMed]
- Jiang X, Ren M, Shuang X, Yang H, Shi D, Lai Q, Dong Y. Multiparametric MRI-Based Radiomics Approaches for Preoperative Prediction of EGFR Mutation Status in Spinal Bone Metastases in Patients with Lung Adenocarcinoma. J Magn Reson Imaging 2021;54:497-507. [Crossref] [PubMed]
- Chee CG, Yoon MA, Kim KW, Ko Y, Ham SJ, Cho YC, Park B, Chung HW. Combined radiomics-clinical model to predict malignancy of vertebral compression fractures on CT. Eur Radiol 2021;31:6825-34. [Crossref] [PubMed]
- Wakabayashi K, Koide Y, Aoyama T, Shimizu H, Miyauchi R, Tanaka H, Tachibana H, Nakamura K, Kodaira T. A predictive model for pain response following radiotherapy for treatment of spinal metastases. Sci Rep 2021;11:12908. [Crossref] [PubMed]
- Liu T, Wang S, Liu H, Meng B, Zhou F, He F, Shi X, Yang H. Detection of vertebral metastases: a meta-analysis comparing MRI, CT, PET, BS and BS with SPECT. J Cancer Res Clin Oncol 2017;143:457-65. [Crossref] [PubMed]
- van Timmeren JE, Cester D, Tanadini-Lang S, Alkadhi H, Baessler B. Radiomics in medical imaging-"how-to" guide and critical reflection. Insights Imaging 2020;11:91. [Crossref] [PubMed]
- D'Arnese E, Donato GWD, Sozzo ED, Sollini M, Sciuto D, Santambrogio MD. On the Automation of Radiomics-Based Identification and Characterization of NSCLC. IEEE J Biomed Health Inform 2022;26:2670-9. [Crossref] [PubMed]
- Podgorsak AR, Rava RA, Shiraz Bhurwani MM, Chandra AR, Davies JM, Siddiqui AH, Ionita CN. Automatic radiomic feature extraction using deep learning for angiographic parametric imaging of intracranial aneurysms. J Neurointerv Surg 2020;12:417-21. [Crossref] [PubMed]
- Chen W, Liu B, Peng S, Sun J, Qiao X. Computer-Aided Grading of Gliomas Combining Automatic Segmentation and Radiomics. Int J Biomed Imaging 2018;2018:2512037. [Crossref] [PubMed]
- Nougaret S, Tibermacine H, Tardieu M, Sala E. Radiomics: an Introductory Guide to What It May Foretell. Curr Oncol Rep 2019;21:70. [Crossref] [PubMed]
- Forouzannezhad P, Maes D, Hippe DS, Thammasorn P, Iranzad R, Han J, Duan C, Liu X, Wang S, Chaovalitwongse WA, Zeng J, Bowen SR. Multitask Learning Radiomics on Longitudinal Imaging to Predict Survival Outcomes following Risk-Adaptive Chemoradiation for Non-Small Cell Lung Cancer. Cancers (Basel) 2022;14:1228. [Crossref] [PubMed]
- Fan M, Yuan W, Zhao W, Xu M, Wang S, Gao X, Li L. Joint Prediction of Breast Cancer Histological Grade and Ki-67 Expression Level Based on DCE-MRI and DWI Radiomics. IEEE J Biomed Health Inform 2020;24:1632-42. [Crossref] [PubMed]
- Zhong L, Dong D, Fang X, Zhang F, Zhang N, Zhang L, Fang M, Jiang W, Liang S, Li C, Liu Y, Zhao X, Cao R, Shan H, Hu Z, Ma J, Tang L, Tian J. A deep learning-based radiomic nomogram for prognosis and treatment decision in advanced nasopharyngeal carcinoma: A multicentre study. EBioMedicine 2021;70:103522. [Crossref] [PubMed]
- Nasrabadi NM. Pattern recognition and machine learning. J Electron Imaging 2007;16:049901. [Crossref]
- Xu L, Yang P, Liang W, Liu W, Wang W, Luo C, Wang J, Peng Z, Xing L, Huang M, Zheng S, Niu T. A radiomics approach based on support vector machine using MR images for preoperative lymph node status evaluation in intrahepatic cholangiocarcinoma. Theranostics 2019;9:5374-85. [Crossref] [PubMed]
- Wang J, Wu CJ, Bao ML, Zhang J, Wang XN, Zhang YD. Machine learning-based analysis of MR radiomics can help to improve the diagnostic performance of PI-RADS v2 in clinically relevant prostate cancer. Eur Radiol 2017;27:4082-90. [Crossref] [PubMed]
- Ko CC, Zhang Y, Chen JH, Chang KT, Chen TY, Lim SW, Wu TC, Su MY. Pre-operative MRI Radiomics for the Prediction of Progression and Recurrence in Meningiomas. Front Neurol 2021;12:636235. [Crossref] [PubMed]
- Peng A, Dai H, Duan H, Chen Y, Huang J, Zhou L, Chen L. A machine learning model to precisely immunohistochemically classify pituitary adenoma subtypes with radiomics based on preoperative magnetic resonance imaging. Eur J Radiol 2020;125:108892. [Crossref] [PubMed]
- Parekh V, Jacobs MA. Radiomics: a new application from established techniques. Expert Rev Precis Med Drug Dev 2016;1:207-26. [Crossref] [PubMed]
- Zhong X, Li L, Jiang H, Yin J, Lu B, Han W, Li J, Zhang J. Cervical spine osteoradionecrosis or bone metastasis after radiotherapy for nasopharyngeal carcinoma? The MRI-based radiomics for characterization. BMC Med Imaging 2020;20:104. [Crossref] [PubMed]
- Florkow MC, Zijlstra F, Willemsen K, Maspero M, van den Berg CAT, Kerkmeijer LGW, Castelein RM, Weinans H, Viergever MA, van Stralen M, Seevinck PR. Deep learning-based MR-to-CT synthesis: The influence of varying gradient echo-based MR images as input channels. Magn Reson Med 2020;83:1429-41. [Crossref] [PubMed]
- Jans LBO, Chen M, Elewaut D, Van den Bosch F, Carron P, Jacques P, Wittoek R, Jaremko JL, Herregods N. MRI-based Synthetic CT in the Detection of Structural Lesions in Patients with Suspected Sacroiliitis: Comparison with MRI. Radiology 2021;298:343-9. [Crossref] [PubMed]
- Attanasio S, Forte SM, Restante G, Gabelloni M, Guglielmi G, Neri E. Artificial intelligence, radiomics and other horizons in body composition assessment. Quant Imaging Med Surg 2020;10:1650-60. [Crossref] [PubMed]